Abstract
This paper primarily explores whether the transmission of a supplier’s disruption risk along the supply chain exists using a quantitative survey conducted in 31 Chinese automotive-related companies. Two downstream supply chain members are considered: manufacturer and distributor. Structural equation modelling is used. We find that both manufacturer and distributor can be affected by supplier disruptions. In particular, distributors are impacted in two ways: indirectly and directly. On the one hand, indirect transmission of the supplier’s disruption risk to distributors is assumed to be an outcome of interrupted material flows for the production and sales of whole vehicles along the supply chain. Domino effect is used to explain this phenomenon. On the other hand, direct transmission is presumed to originate from the direct business contact between the supplier and distributors in terms of automotive spare parts. Based on primary findings, this paper further investigates strategies used by manufacturers and distributors to mitigate the adverse effects of supplier disruptions through semi-structured interviews. Theoretical and practical implications, as well as limitations, are discussed.
Notes
Disclosure statement
No potential conflict of interest was reported by the authors.
Notes
1 Established in 1994, BCI has positioned itself as a leading membership and certifying organisation for Business Continuity (BC) professionals. It started the Supply Chain Resilience survey in 2009, and has conducted it on a yearly basis. The survey highlights the complexity of current supply chains, the level, range and cost of disruptions to organizations. Interested readers can visit www.thebci.org.
2 Automotive industry is characterized with a high level of modularization, which refers to the process of building a complex product from smaller components that can be designed separately, yet function together as a whole (Doran Citation2004; Lin et al. Citation2009). As a consequence, multi-tiered suppliers are often involved in a single automotive supply chain, increasing its complexity, and also making it an ideal research subject for us to study the domino effect in supply chain. In fact, automotive industry is a very popular research subject in studies on supply chain risk (e.g. Ceryno, Scavarda, and Klingebiel Citation2015).
3 Stata 12.0 was used to realise our structural equation modelling. Dissimilar to other professional SEM tools (e.g. Amos, Lisrel and EQS), Stata is more comprehensive tool that is capable of conducting a variety of quantitative analysis (e.g. regression, clustering and SEM).
4 Response bias is considered here, which relates to cognitive biases that lead responses of participants away from the true response. It is said to be an important issue to control for in self-report surveys (Furnham Citation1986). However, a comprehensive review made by Mortel (Citation2008) demonstrates that response bias is much more likely to happen when participants are asked to self report on their competence or socially sensitive topics, such as history of incarceration and psychiatric conditions. In our survey, these topics were not involved, thus helping response bias to be largely reduced.
5 Common method bias is also concerned. It is pertinent to the variance that is attributable to the measurement method rather than to the constructs the measurements represent, and is claimed to be one of the main sources of measurement error (Podsakoff et al. Citation2003). Podsakoff, MacKenzie, and Podsakoff (Citation2012) further offered several procedural remedies to alleviate this bias, which included obtaining measures from different sources, protecting respondent anonymity and reducing evaluation apprehension, as well as improving scale items. These procedures were strictly followed during the development of our measures, thus helping reduce the common method bias to a great extent.
6 Among supplier risks, disruption risk is a single construct; thus, the problem of multicollinearity does not exist. As a result, we do not need to conduct an EFA for it.
7 It should be noted that the correlations in are the measurement error-adjusted inter-construct correlations, which derive from the SEM model, rather than raw correlations, which derive from the data. Moreover, to perform the AVE-SV comparison, the correlations in must be squared.
8 It should be noted that all of the notes were first taken in Chinese and then translated into English for presentation in this paper.
Additional information
Notes on contributors
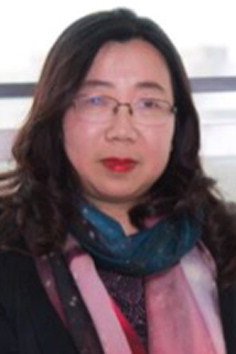
Jiantong Zhang
Jiantong Zhang is a professor and former department chair in the Department of Management Science & Engineering, Tongji University, Shanghai, China. She holds a PhD in Applied Statistics from University Pierre and Marie Curie, and a master's degree in Financial Statistics from Paris Diderot University. Both universities are located in Paris, France. She also received a master’s and bachelor’s degree in Probability Statistics from Jilin University, Changchun, China. Her research interests include supply chain management, applied statistics as well as information systems. She has extensively attended international conferences, such as 2014 INFORMS annual meeting and 2014 POMS international conference. Her publications have appeared in Transportation Research Part E: Logistics and Transportation Review, Maritime Policy & Management, Journal of Cleaner Production and so on.

Xiaodong Chen
Xiaodong Chen is a PhD candidate in the Department of Management Science & Engineering, Tongji University, Shanghai, China. He is also working as CFO in HASCO Auto Parts Group Company, which is a leading auto parts manufacturer in China. He obtained his MBA from Norwegian School of Management in 2005, and received a bachelor’s degree in Financial Management from Fudan University, Shanghai, China in 1999. His researches have been published in international journals, such as International Journal of Systems Science.
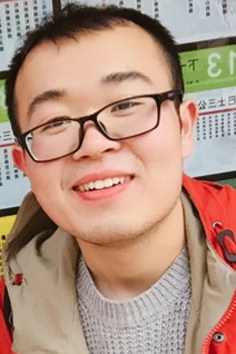
Chencheng Fang
Chencheng Fang is a PhD candidate in the Department of Management Science & Engineering, Tongji University, Shanghai, China. He received his bachelor's degree in Statistics from Zhejiang Gongshang University, Hangzhou, China in 2014. His research interests include information systems, supply chain management and their interface. He has presented his researches in several academic conferences, such as 11th China Summer Workshop on Information Management, 14th International Conference on Services Systems and Services Management, as well as 5th International Asia Conference on Industrial Engineering and Management Innovation. His papers have appeared in Journal of Cleaner Production and Scientometrics.