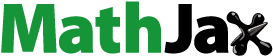
Abstract
Considering the increasing complexity of application scenarios, the evaluation of the intelligence levels for unmanned swarms has attracted scholarly attention. However, the existing evaluation studies cannot suitably reflect the intelligence of unmanned swarms, and they rarely provide a specific evaluation process. By introducing the collective intelligent behavior model for unmanned swarms based on the collective Observe–Orient–Decide–Act (OODA) loop, this study constructs a comprehensive evaluation index system that can systematically reflect the overall intelligence of unmanned swarms in complex scenarios. Considering the fuzziness and randomness in the processes of weight calculation and level evaluation, this study proposes a comprehensive evaluation method of the intelligence levels for unmanned swarms based on a group extension cloud model. This method calculates the weights of various evaluation indexes at different layers by adopting the group extension analytic hierarchy process. Moreover, it obtains the comprehensive evaluation conclusion of the intelligence levels for unmanned swarms by adopting the cloud model. Applying the proposed method, this study evaluates two specific types of unmanned aerial vehicle swarms. The results show that the proposed method can more flexibly and accurately evaluate the intelligence levels of unmanned swarms than the previous fully qualitative methods.
1. Introduction
An unmanned swarm is an artificial swarm system comprising a certain number of homogeneous or heterogeneous unmanned vehicles and other auxiliary units (Bai et al., Citation2016) and has become an important development direction for unmanned systems. As a combination of unmanned systems and swarm intelligence, an unmanned swarm is not a simple aggregation of multiple simple unmanned vehicles. Nonetheless, it makes them cooperate closely to form a self-organised and highly stable distributed system to stimulate individual and swarm intelligence (Sun et al., Citation2020). Owing to their multiple advantages (including intellectualisation, collectivisation, and unmanned operation), unmanned swarms have wide application prospects in complex scenarios, such as cooperative operation, logistics transmission, industrial production, and emergency response (Liang et al., Citation2018). The basic forms of unmanned swarms mainly include unmanned aerial vehicle (UAV), unmanned ground vehicle, unmanned sea vehicle, unmanned underwater vehicle, and some other hybrid unmanned swarms (Liu et al., Citation2017). Currently, cooperative operation is the most important application scenario for unmanned swarms, and the UAV swarm is the primary development form (Jia et al., Citation2019). Countries all over the world are committed to the research and development of UAV swarms, and several representative projects, such as Gremlins (Daniel, Citation2016), Offensive Swarm-Enabled Tactics (DARPA Public Affairs, Citation2016), Perdix (Zhang, Citation2015) and Low-Cost UAV Swarming Technology (Office of Naval Research, Citation2015), have emerged.
Previous studies have demonstrated that the effective evaluation of the capability, effectiveness, or performance for unmanned systems will play a leading role in technology. Recently, the intelligence realisation of unmanned swarms has begun to receive increasing attention (Zhou et al., Citation2020). Every type of intelligence is acquired progressively (Yang, Citation2018). The evaluation of the intelligence levels for unmanned swarms has become an important part of studying and understanding their performance (Dai et al., Citation2020). First, it helps policy makers to scientifically measure the intelligence levels of the existing unmanned swarms and point out the right way to develop and realise their intelligence from low to high when formulating development plans and polices for unmanned swarms and its related industries, respectively. Second, it enables developers and designers to reasonably determine whether the expected intelligence level is achieved. Third, it helps users to systematically describe the intelligence requirements for unmanned swarms and effectively evaluate the intelligence level of multiple similar unmanned swarms.
Although the evaluation of the intelligence levels for unmanned swarms is still a new research problem, there are many alternative methods that can be used to approximately evaluate the intelligence levels of unmanned swarms. However, most previous related studies only provided some fully qualitative evaluation methods based on different evaluation criteria and did not provide a specific evaluation process. A cloud model is a cognitive model that investigates the uncertainties in the transformation between qualitative concepts and quantitative values and is not disturbed by fuzziness and randomness (Harrod, Citation2009; Zhao & Song, Citation2016). Considering the development of collective intelligence, the constructed evaluation index cannot suitably reflect the overall intelligence of unmanned swarms because the previous evaluation model cannot suitably describe the cooperation between individuals in an unmanned swarm. The commonly used weight determination method in previous studies is still the traditional analytic hierarchy process (AHP). This method requires that the elements in the importance judgment matrix are exact numbers, and it does not consider the complexity and uncertainty of each index (Li & Qiu, Citation2019). Moreover, owing to the differences in knowledge structure, preference, and cognitive ability of different decision-makers, the relative importance of the evaluation indexes often deviates from the objective reality. Therefore, it is difficult to obtain more accurate weights using the traditional AHP.
In this study, we propose a quantitative evaluation solution for the intelligence levels of unmanned swarms based on the collective Observe-Orient-Decide-Act (OODA) loop and group extension cloud model. The contributions of this study are enumerated below.
The constructed intelligence evaluation index system covers the collective observation, orientation, decision, and action competencies. It can more suitably and comprehensively reflect the overall intelligence of unmanned swarms owing to the introduction and reference of the collective intelligent behavior model of unmanned swarms based on the collective OODA loop.
The proposed group extension AHP method replaces the exact numbers with interval numbers, integrates the judgments of multiple decision experts, and it does not require a consistency check. It can more flexibly, accurately, and conveniently determine the weights of the constructed intelligence evaluation index system than the traditional AHP method because of the integration of the extension and group decision-making methods.
The designed comprehensive evaluation method based on the cloud model simplifies the measurement and quantification of intelligence evaluation indexes. Furthermore, it fully describes the fuzziness and randomness in the intelligence level evaluation process of unmanned swarms. It can obtain more objective and accurate evaluation results owing to the transformation from a qualitative evaluation to a quantitative evaluation.
The rest of this study is organised as follows. Section 2 reviews the related studies. Considering Section 3, a comprehensive intelligence evaluation index system is constructed for unmanned swarms in complex scenarios by introducing a collective intelligent behavior model based on the collective OODA loop. Section 4 describes the basic principles and detailed design processes of the comprehensive evaluation method for the intelligence levels of unmanned swarms based on the group extension cloud model. To validate the proposed method, a case study is presented in Section 5. Regarding Section 6, the conclusions of this study are presented, and future studies are recommended.
2. Related studies
Researchers have been paying attention to the autonomy level evaluation of unmanned systems for a long time. Because unmanned swarms are also unmanned systems, most of the previous studies included the evaluation of unmanned swarms. Although autonomy and intelligence are two different concepts, autonomy level evaluation methods can also be used to evaluate their intelligence level. This is because the autonomy of an unmanned system is the external manifestation of its intelligence (Duan & Qiu, Citation2018; Fan & Zhang, Citation2017).
Early evaluation studies on the autonomy level of unmanned systems were mainly achieved through a set of extremely simple qualitative languages. To investigate the autonomous operation capability of unmanned systems, the Department of Defense (DoD) of the United States has proposed the definition of autonomy control level (ACL) and has successively released multiple versions of the unmanned system roadmap. Considering the earlier versions (Office of the Secretary of Defense, Citation2000, Citation2002, Citation2005), UAV autonomy is classified into ten levels. These include: remotely guided, real-time health diagnosis, adaptation to failures and flight conditions, onboard route re-planning, group coordination, group tactical re-plan, group tactical goals, distributed control, group strategic goals, and fully autonomous swarms. Regarding this classification, levels one to four describe monomer autonomy, and levels five to ten describe swarm autonomy. Although other research institutions later updated the autonomous capability description of UAVs from different perspectives, they generally did not deviate from the description scope.
Several qualitative evaluation methods are based on multiple evaluation indexes. Draper (Goodrich et al., Citation2003) divided unmanned system autonomy into four levels based on three dimensions: motion control, task planning, and state understanding. The National Aeronautics and Space Administration (NASA) proposed a simplified ACL that classified UAV autonomy into six levels: remote control, simple automatic operation, remote operation, highly automated (semi-autonomous), and fully autonomous and collaboration (Larry et al., Citation2005). One intuitive data is used to show the autonomy level, which indicates the amount of time the person controls during the flight course of the UAV. The Air Force Research Laboratory (AFRL) further refines the evaluation requirements of the ten-level ACL by introducing the classical OODA loop (Clough, Citation2002). Based on the actual development of the UAV autonomy in China, Chen et al. (Citation2011) proposed a more suitable UAV ACL based on four evaluation indexes: autonomous function, autonomous type, intelligent attributes, and communication level.
Some institutions have also proposed few generic evaluation methods. The National Institute of Standard and Technology (NIST) proposed a generic autonomy levels for unmanned systems (ALFUS) framework that comprehensively evaluated the autonomy level based three dimensions: task complexity, environment complexity, and human independence (Huang et al., Citation2005). These three dimensions contain series of influencing factors and a set of measurement methods. The ALFUS framework model can effectively reflect the task execution ability of unmanned systems; nonetheless, the model evaluates unmanned system autonomy completely from an external perspective. Furthermore, there are series of problems that cannot be ignored in practical applications. First, the process of measuring the factors in each dimension and allocation of weights is not provided. Second, the evaluation of the autonomy level is undetermined. The Shenyang Automation Research Institute proposed a universal cobweb model (Wang & Liu, Citation2012) to evaluate the autonomy level of unmanned systems. The model has strong generality; however, it has the same problems as the ALFUS framework model in practical applications. A comparison of these autonomy-level evaluation methods is shown in Table .
Table 1 Comparison of various autonomy level evaluation methods
Recently, few researchers have proposed special evaluation studies on the intelligence levels of unmanned swarms. Considering the development of marine robots and the history of artificial intelligence, Zhang et al. (Citation2017) divided swarm intelligence into six levels: formation control, task planning, cooperation, task re-planning, cooperative exploration, and duty. The level classification standards are common to other fields; however, the study does not provide detailed explanations for each level. Moreover, there is no further study on how to evaluate the intelligence level of unmanned swarms. Wu and Zhou (Citation2020) proposed a generic intelligence level measurement model for unmanned swarms, and the model is composed of three dimensions: scene complexity, system adaptability, and system effectiveness. The model has a strong generality; however, it also has the same problems as the ALFUS framework model in practical applications. In addition, some researchers have proposed some fully quantitative methods (Chmait, Citation2017; Chmait et al., Citation2015; Commuri et al., Citation2004; Dai et al., Citation2020); nonetheless, these methods can only evaluate the intelligence of a certain dimension of unmanned swarms.
In summary, most previous studies are still at the preliminary cognition stage and only provide some qualitative evaluation methods based on different evaluation criteria. However, they do not provide a specific quantitative evaluation process. Moreover, it is difficult for them to reasonably reflect the overall intelligence of unmanned swarms owing to the lack of relation to the corresponding reasonable theoretical models and the over-generalization of evaluation objects. Several researchers have proposed operable evaluation methods for the autonomy level of unmanned systems through mathematical models and quantitative language (Shuai et al., Citation2016; Yan & Tang, Citation2012; Yang & Zhang, Citation2009). These methods can also be used to evaluate the intelligence level of unmanned swarms. However, they still need to be further improved because they all use the traditional AHP when determining the weights of the evaluation indexes. There are recently some emerging related evaluation methods, such as expertise-based bid evaluation with generalised comparative linguistic ELECTRE III (Chen et al., Citation2021a), online review analysis based on large-scale group decision-making (Zsca et al., Citation2021), power-average-operator-based hybrid multi-attribute online recommendation model (Chen et al., Citation2021b), and third-party selection approach based on hybrid-information multi-criteria decision making and cumulative prospect theory (Li et al., Citation2018). Although these advanced methods aim at different objects and have not yet been used in this field, they are not available to improve the comprehensive evaluation process for the intelligence levels of unmanned swarms in the future.
3. Construction of an intelligence evaluation index system for unmanned swarms
3.1. Collective intelligent behavior modelling based on the collective OODA loop
The construction of an intelligence evaluation index system for unmanned swarms should be closely related to the collective intelligent behavior model of unmanned swarms. The traditional collective intelligent behavior modelling methods are realised by simulating the collective intelligent behaviors of simple social biological swarms (Barbara, Citation2002). A typical representative is the Boids model proposed by Reynolds (Citation1987). This modelling method usually needs to make appropriate simplification, abstraction, and assumptions for individual behavior ability and rules in advance (Amanda, Citation2007; Fidel et al., Citation2012). Nonetheless, it is only suitable for some relatively simple scenarios owing to the limitation of individual ability. Considering some complex scenarios, the intelligent behavior of unmanned monomers is often modelled as a classical OODA loop (Boyd, Citation1996). The loop was proposed by Boyd in 1996 and was used to describe the entire process of military system decision-making. However, it only has a good guiding role in analyzing the intelligent behavior of unmanned monomers and is insufficient for describing the cooperation and interaction between multiple unmanned vehicles.
In summary, the traditional collective intelligent behavior modelling method based on simple rules and the classical OODA loop cannot be applied to describe the collective intelligent behavior of unmanned swarms in complex and changeable battlefield environments. Therefore, this study introduces a collective intelligent behavior modelling method for unmanned swarms based on the collective OODA loop as shown in Figure . The collective OODA loop is a type of collective extension for the classical OODA loop. From a micro perspective, each unmanned agent has a highly intelligent behavior, and its intelligent behavior can be regarded as a classical OODA loop. From a macro perspective, the collective intelligent behaviors of an unmanned swarm composed of multiple unmanned agents with highly intelligent behaviors and other agents can be regarded as a collective OODA loop. Therefore, the overall intelligence of unmanned swarms can be realised and analyzed based on the collective OODA loop.
Considering the collective intelligent behavior model based on the collective OODA loop, unmanned swarms complete a given target task through collective observation, orientation, decision, and action functions or competencies between the unmanned agents and between unmanned agents and other agents. These four collective functions support an unmanned swarm having a stronger operational competence in each stage of task execution to promote a significant improvement in the overall task competence. The collective observation competence, for example, can widen the perception range of the whole system. Moreover, the perception types are more diversified, and the individual perception error or loss can be corrected and compensated. When we regard the whole unmanned swarm as a unified whole, it is equivalent to forming a virtual agent from a global perspective. Considering the processes of the collective observation, orientation, decision, and action, the virtual global agent makes a comprehensive trade-off from the overall layer of the swarm based on the global perspective. This ultimately reflects that the behavior of individual unmanned agents in the swarm is not necessarily optimal; however, the effect of the overall behavior of the swarm is optimal. This reflects swarm intelligence. The virtual global agent is not a top-down global control, and its role is not to replace the unmanned agents in the swarm to make decisions. Nonetheless, it is to make individual decision-making with its assistance to have a more macro perspective. Thus, it assists unmanned agents to obtain and process global information and coordinate within the swarm.
3.2. Construction of the evaluation index system based on the collective OODA loop
It is indisputable that the evaluation of the intelligence level of unmanned swarms is a multi-objective and -layer systematic project. To establish a reasonable and comprehensive evaluation index system, we need to consider various factors that have a significant impact on the intelligence level of unmanned swarms as comprehensively as possible using the hierarchical analysis process. Based on the collective intelligent behavior model of unmanned swarms based on the collective OODA loop, it can be concluded that the overall intelligence of unmanned swarms mainly depends on four basic collective competencies: collective observation, orientation, decision, and action. These four basic collective competencies can be described and analyzed from different perspectives. For example, considering the inherent competencies of unmanned swarms, collective observation competence mainly includes radar detection, photoelectric detection, and electronic reconnaissance competencies. However, these inherent competencies are only some basic conditions that generate the intelligence of unmanned swarms, and they do not play a decisive role in evaluating the intelligence level of unmanned swarms.
The intelligence of unmanned swarms can be more reflected in whether it has collective intelligence competencies that are mainly obtained by simulating, extending, and expanding the biological swarm or even human team intelligence (Donna, Citation2017), such as collective perception, navigation, recognition, assessment, diagnosis, prediction, planning, etc. These collective intelligent competencies can distinguish the intelligence level of unmanned swarms, and they may involve more specific classifications. By further sorting out some specific collective intelligent competencies that can reflect the intelligence of unmanned swarms based on the four basic collective competencies described in the collective intelligent behavior model of unmanned swarms based on the collective OODA loop, a multi-dimensional hierarchical intelligence evaluation index system of unmanned swarms can be constructed as shown in Figure .
4. Comprehensive evaluation method based on the group extension cloud model
4.1. Group extension AHP
The extension theory is widely applied to solve comprehensive evaluation problems aimed at multiple objectives (Yang & Cai, Citation2013). The extension set is a quantitative tool used to describe the variability of things in the extension methods. This can determine the degree of things belonging to a certain set based on their quantity values about a certain characteristic. The group extension AHP introduces group decision-making and extension theories into the traditional AHP, replaces exact numbers with interval numbers, and gathers the judgment of multiple experts when constructing the importance judgment matrix of the evaluation indexes. The processes for determining the weights of the evaluation indexes by the group extension AHP are demonstrated below.
Step 1: Construction of the comprehensive extension judgment matrix. The construction of the comprehensive extension judgment matrix is a formal process that transforms various original source data into data that can be directly compared. The general extension AHP (Wu et al., Citation2016; Zhang et al., Citation2014) usually invites a single domain expert to compare the relative importance of the indexes at the -th layer in pairs on a certain index at its upper layer. Thereafter, all the results are combined to form an extension judgment matrix:
. A is a positive reciprocal matrix, which indicates that all the elements obey the conditions
and
. All the elements of A are the interval numbers.
indicates its element in the
-th row and
-th column.
and
indicate the lower and upper endpoints of the element, respectively. The group extension AHP invites
domain experts to construct a comprehensive A using the group decision-making method. To avoid the subjective preference of the invited experts, the value of T should theoretically be as large as possible. This indicates that the invited experts should as many as possible. However, the actual number needs to be determined in combination with other conditions, such as the authority, representativeness, accessibility of experts, and consulting costs. The extension judgment matrix provided by the
-th domain expert is indicated as
and its element in the
-th row and
-th column is indicated as
. Considering the comprehensive A constructed by T domain experts, its element in the
-th row and
-th column can be calculated as:
(1)
(1) Step 2: Calculation of the extension weight vector. The lower and upper endpoint matrices
and
, respectively, can be easily obtained based on these extension interval elements. The processes for calculating the extension weight vector of
satisfy the consistency condition based on the following.
First, the normalised eigenvectors and
with positive components corresponding to the maximum eigenvalues of A+ and A- can be easily calculated.
Second, the values of the two intermediate variables and
can be calculated as:
(2)
(2) where
and
meet condition
. If
, it can be concluded that the constructed A is consistent.
Finally, the extensive weight vector corresponding to matrix A can be calculated as:
(3)
(3) where
indicates the extension interval weight of the
-th element at the
-th layer on a certain factor at its upper layer.
Step 3: Calculation of the single-ordering weights. Considering the hierarchical evaluation index system, each element at the -th layer has a weight expressed by the interval number
, and the relative importance of the two elements
and
at the
-th layer can be calculated as.
(4)
(4) When a fixed number
meets the conditions that
for
,
, and
, the single ordering weights of
elements at the
-th layer on the
-th element at its upper layer can be normalised as:
(5)
(5) Step 4: Calculation of the total ordering weights. Considering the corresponding
of each element at the
-th layer on all elements at its upper layer, an
matrix
can be obtained. If the weight sort of the elements at the
−1th layer on the total objective is indicated as
, then the weight sort of the elements at the
-th layer on the total objective can be defined as:
(6)
(6) Finally, it can be calculated as
.
4.2. Comprehensive evaluation method based the cloud model
(1) Cloud model
The cloud model is a new cognitive model based on the fuzzy set and probability theories (Li et al., Citation2009), and it can effectively reflect the fuzziness and randomness of things or human knowledge. The model skilfully integrates the fuzziness and randomness of things themselves and uses fixed mathematical expressions to describe them uniformly. Currently, the cloud model has developed various forms, such as normal, triangular, and trapezoidal clouds, among which the first model is the most widely used.
The normal cloud model is indicated as triple C(Ex, En, He). Considering the triple, Ex is indicated as the expectation of the membership cloud. This is the central position of the membership cloud, which is the most representative point of a qualitative concept. En (also known as entropy) indicates the width of the membership cloud, which is a measure of the uncertainty of the qualitative concept. He (also known as super entropy) indicates the variance of the membership cloud, which is a measure of the uncertainty of the entropy, and it reflects the dispersion degree of the membership cloud.
(2) Cloud model algorithm
The cloud model algorithm is also referred to as a cloud generator, and it can effectively establish the relationship between qualitative and quantitative attribute values. The cloud generator mainly includes a forward generator, reverse generator, and index approximation method. The algorithm generating two-dimensional points of the cloud is known as a forward generator. One point in the cloud is referred to as a cloud droplet and is indicated as a drop(xi, ui). Considering the three mathematical characteristics of the cloud model, the implementation algorithm for the normal cloud is described below.
Step 1: Generate a normal random number with En as the expectation and He as the variance as expressed below:
(7)
(7) Step 2: Generate a normal random number with Ex as the expectation and
as the variance:
(8)
(8)
Step 3: Calculate the membership degree:
(9)
(9) Obtain a cloud droplet with
as the membership degree.
Step 4: Repeat Steps 1 to 3 until n cloud droplets are generated.
(3) Cloud model operation rules
A new cloud model can be constructed by adopting the fitting operation rules for two cloud models. The fitting operation rules of the mathematical characteristics of the two normal cloud models are shown in Table .
Table 2 Fitting operation rules for two normal clouds
(4) Comprehensive evaluation process of reference levels based on cloud model
Considering actual applications, a comprehensive evaluation of the total objective may be obtained either by single- or multiple-dimensional indexes. The basic processes for establishing a comprehensive evaluation model of objective levels based on the cloud model (Pan et al., Citation2012; Zhang et al., Citation2019) based single-dimensional evaluation indexes are demonstrated below.
Step1: Evaluation index set establishment of the single dimension. The evaluation index set is established by adopting the hierarchical analysis process and is indicated as , where
represents the
-th evaluation factor and
indicates the number of the evaluation indexes.
Step 2: Weight cloud model establishment of the evaluation indexes. The weight set of the evaluation indexes is obtained by adopting the group extension AHP. It is also further transformed into a weight cloud set as:
(10)
(10) where Exw indicates the weight, and
, Ew and Hew indicate the maximum deviation from the expectation and randomness when the expert panel scores.
Step 3: Evaluation cloud model establishment of the evaluation indexes. The evaluation indexes are divided into several qualitative levels based on the actual requirements. The evaluation result set is obtained by adopting the expert rating method and is further transformed into a normal evaluation cloud set as:
(11)
(11) where Exe indicates the score allotted by the expert panel, and Ene = 1/3Ee, Ee and Hee indicate the maximum deviation from the expectation and the randomness when the expert panel scores.
Step 4: Comprehensive evaluation cloud model establishment of the total objective. The comprehensive evaluation cloud model of single-dimension evaluation indexes on the total objective can be obtained by adopting the fitting operation rules on their weight and evaluation clouds as shown in Table .
(12)
(12) Similarly, the comprehensive evaluation cloud model of multiple-dimension evaluation indexes on the total objective can be obtained by adopting the fitting operations rules on the weight and evaluation clouds of multiple sub-objectives. Therefore, a multidimensional hierarchical comprehensive evaluation process based on a cloud model can be realised.
5. Case study
In this study, we mainly consider two specific types of UAV swarms as an example to introduce the process of evaluating the intelligence levels of unmanned swarms by adopting the group extension cloud model. First, the evaluation index weights of all the layers were obtained by adopting the group extension AHP. Second, an intelligence evaluation level classification of unmanned swarms based on the collective OODA loop is presented. Thereafter, the cloud model is used to obtain the comprehensive evaluation results of the intelligence levels of unmanned swarms by fitting it layer-by-layer.
In this section, as shown in Figure , u indicates the overall intelligence of an unmanned swarm, and u1–u4 indicate collective observation, orientation, decision, and action competencies, respectively. Moreover, u11–u13 indicate the environment, target, and self-state perception competencies, respectively. Furthermore, u21 and u22 indicate situation assessment and fault diagnosis and prediction competencies, respectively; u31 and u32 represent the task allocation and path planning competencies, respectively; u41 and u42 indicate the task action competence and human independence, respectively. The interpretation of all the other variables is consistent with the descriptions in Section 4.
5.1. Weight calculation based on group extension AHP
Considering the constructed intelligence evaluation index system of unmanned swarms, three experts who have been engaged in the research and development of unmanned swarms for a long time are invited to construct the extension judgment matrices at all layers by adopting the one-nine scale method proposed by Satty (Liu, Citation2012). A value of 1 indicates that an element is as important as another element, while the values of 3, 5, 7, and 9 indicate that one element is slightly, obviously, strongly, and extremely important than another element, respectively. The relative importance of the primary indexes on the overall intelligence of an unmanned swarm is summarised in Table .
Table 3 Relative importance of the primary indexes on the overall intelligence of an unmanned swarm
The lower and upper endpoint matrices and
of the comprehensive extensive judgment matrix
, respectively, can be obtained using Eq.(1) as shown below:
The normalised eigenvectors and
with positive components corresponding to their maximum eigenvalues can be easily obtained as demonstrated below:
The values of the two intermediate variables can be obtained using Eq.(2), and
. They meet the conditions
, which indicates that the constructed A is consistent.
The weights expressed by the interval numbers can be obtained using Eq.(3) asindicated:
The relative importance between elements and
can be obtained using Eq.(4) as provided below:
Through normalisation, the single ordering weights of the four primary indexes on the overall intelligence of an unmanned swarm can be obtained as shown below:
Similarly, the single ordering weights of the nine secondary indexes on the four primary indexes can be obtained as indicated below:
5.2. Intelligence level classification based on the collective OODA loop
Currently, there is no authoritative standard for classifying the intelligence levels of unmanned swarms. By referring to and combining the classification standard of the intelligence levels of unmanned swarms proposed by Zhang et al. (Citation2017) and the classical ACL distributed by DoD in 2005 (Office of the Secretary of Defense, Citation2005), we divided the intelligence of unmanned swarms into six levels: formation control, task planning, cooperative tasking, task re-planning, cooperative exploration, and fully rational from low to high. This is generally consistent with the intelligent development trend in the two classifications mentioned above; however, they are more reasonable in naming or more suitable for unmanned swarms. The intelligence of unmanned swarms is in the rapid development stage. Moreover, we investigate the existence of one or some specific collective competencies that can reflect the intelligence of unmanned swarms rather than their quantity. Therefore, we have further refined the specific evaluation requirements of different intelligence levels for unmanned swarms on the collective OODA loop as shown in Table . The structure of the classification is similar to the UAV ACL classification based on the OODA loop proposed by AFRL (Clough, Citation2002). Furthermore, it provides comprehensive evaluation levels of the nine secondary indexes on the four primary indexes and the comprehensive evaluation levels of four primary indexes on the overall intelligence of an unmanned swarm. However, the classification is only a type of relative reference because there are some uncertainties in the evaluation results of different evaluation indexes. The impact of different evaluation indexes on the overall evaluation results is also different. To obtain a reasonable intelligence level evaluation result, special methods are required.
Table 4 Classification for the intelligence level of unmanned swarms based on the collective OODA loop
5.3. Comprehensive evaluation of intelligence level based on the cloud model
In this study, we divide the qualitative evaluation conclusions into six intelligence levels as shown in Table . The corresponding expectation can be respectively set to 0.1 -0.6 in the cloud models. The greater the expectation is, the better the evaluation result. The mathematical characteristics of the normal cloud transforming the qualitative evaluation are expressed as Ce(Exe, Ene, Hee), where expectation Exe is the average score allotted by the expert panel, entropy Ene = 1/3Ee, Ee is the maximum deviation of the score provided by each expert relative to the expectation, and super entropy Hee is the randomness covered in the scores provided by the experts. Figure shows a cloud chart of six reference intelligence levels.
The weights of the primary and secondary indexes are converted into the corresponding weight clouds, and their mathematical characteristics are shown in Table and , respectively. Considering the proposed intelligence level classification method and the corresponding scoring standard mentioned above, a special expert panel is invited to evaluate the levels of the secondary indexes for the two specific types of UAV swarms by referring to the available description of system functions and observing its real behaviors. Thereafter, the evaluation results of the secondary indexes for the two UAV swarms are converted into the corresponding evaluation clouds, and their mathematical characteristics are shown in Table . Based on this, the mathematical characteristics of the evaluation clouds of the primary indexes can be obtained by adopting the fitting operation rules as shown in Table on the weight and evaluation clouds of the secondary indexes. The calculation results are shown in Table .
Table 5 Weight and evaluation clouds of the secondary indexes
Table 6 Weight and evaluation clouds of the primary indexes
Similarly, the mathematical characteristics of the comprehensive evaluation cloud models of the intelligence levels for the two UAV swarms A and B can be obtained as C1(0.274,0.0281,0.0035) and C2(0.348,0.0336,0.0042), respectively, by adopting the fitting operation rules as shown in Table on the weight and evaluation clouds of the primary indexes. Their comprehensive evaluation cloud chart is shown in Figure .
5.4. Evaluation results analysis
Considering Figure , the most concentrated cloud drops of UAV swarm A are located between Levels 2 and 3, whereas that of UAV swarm B are located between Levels 3 and 4. This indicates that the intelligence level of UAV swarm B is higher than that of UAV swarm A. The evaluation cloud of UAV swarm B is slightly larger than that of UAV swarm A, considering the span. This demonstrates that the evaluation conclusion of UAV swarm B has a higher fuzziness and randomness than that of UAV swarm A. The evaluation cloud of UAV swarm B is slightly larger than that of UAV swarm A, considering the dispersion degree and thickness. This indicates that the evaluation conclusion of UAV swarm B reached a better consensus than that of UAV swarm A. The evaluation exhibited some fuzziness and randomness. The intelligence of UAV swarm A meets the evaluation requirements of Level 2. Nonetheless, there is still a gap in reaching the evaluation requirements of Level 3. Moreover, the intelligence of UAV swarm B meets the evaluation requirements of Level 3; however, there is still a gap in reaching the evaluation requirements of Level 4. Regarding the perspective of dimension distribution, there is still a certain distance between the current intelligence evaluation results and the most satisfactory intelligence evaluation results. This shows that there is still a great need for further improvement.
Considering Table , the four primary indexes have different effects on the overall intelligence of an unmanned swarm. Furthermore, they are the collective orientation, decision, observation, and action competencies in order of importance. This conclusion is consistent with our general understanding of intelligence. Thinking can best reflect intelligence, perception is the second, and action is the last (Liu et al., Citation2007; Wu & Zhou, Citation2021). There are some inconsistencies in the evaluation results of the four primary evaluation indexes of each evaluated UAV swarm. These inconsistencies effectively meet the actual evaluation requirements of the intelligence levels for unmanned swarms. This is because the actual unmanned swarm cannot be developed completely based on the intelligence level classification method established by the evaluators. Furthermore, it is possible that the intelligence of a certain dimension is slightly higher or lower. The intelligence level of UAV swarm A is lower than that of UAV swarm B mainly because its evaluation results on the four primary indexes are less than or equal to those of UAV swarm B. Regarding Table , the nine secondary indexes have different effects on their superior indexes. There are also some inconsistencies in the evaluation results of the nine secondary indexes of each evaluated UAV swarm. These inconsistencies were also allowed in the evaluation process.
Subsequently, the researchers and developers should continue to work hard in the following aspects. First, they should pay attention to the overall intelligence realisation of unmanned swarms based on the collective intelligent behavior model based on the collective OODA loop. Second, they should continuously improve the intelligence level of unmanned swarms in a progressive manner based on the given intelligence level classification. Moreover, they should regularly evaluate the intelligence level of unmanned swarms in a suitable and feasible evaluation method to prove that its intelligence has been improved.
6. Conclusions and future studies
A comprehensive evaluation of the intelligence levels for unmanned swarms is a complex matter. We propose a suitable evaluation solution. This study combines the collective OODA loop and the group extensive cloud model to achieve this goal. The study has constructed a two-layer evaluation system, covering the collective observation, orientation, decision, and action competencies. The constructed evaluation index system can suitably and comprehensively reflect the overall intelligence of unmanned swarms owing to the introduction and reference of the collective intelligent behavior model of unmanned swarms based on the collective OODA loop. The proposed group extensive AHP method replaces exact numbers with interval numbers when constructing the comparison matrix of the evaluation indexes. It also effectively reduces the influence of individual subjective preferences by synthesising the judgments of multiple experts. In addition, there is no additional consistency check because the obtained weight vector naturally satisfies consistency. Therefore, it can more flexibly, objectively, and conveniently calculate the weights of the evaluation indexes than the traditional AHP used in previous studies. The comprehensive evaluation method based on the cloud model simplified the measurement and quantification of the evaluation indexes. This can fully describe the fuzziness and randomness of the evaluation process. We enabled the transformation of a qualitative evaluation into a quantitative evaluation by rendering results more objective and accurate than the fully qualitative evaluation methods used in previous studies. The limitations of the proposed method are that it is not a fully quantitative evaluation and still needs to rely on the judgment of experts in the evaluation process.
Subsequently, this study can be improved from the following two aspects. Considering the construction of an intelligence evaluation index system for unmanned swarms, it should be designed more detailedly with the continuous opening of relevant parameters. It should also incorporate quantitative evaluation indexes. Moreover, its structure is not necessarily designed strictly based on a hierarchical structure because of the complicated correlation between evaluation indexes. Regarding the evaluation methods, the weight-determining method based on group extension AHP can further consider the authority of different expert judgments rather than a simple geometric average processing. Furthermore, the comprehensive evaluation method based on the cloud model can be further optimised by introducing the extension and group decision-making methods to further improve its flexibility and objectivity.
Acknowledgement
This study was supported in part by the National Defense Science and Technology Innovation Program of China under grant number18-163-11-ZT-003-010-01 and the National Key Research and Development Program of China under grant number 2017YFB1001900. Moreover, we would like to appreciate domain experts for their participation in the case study and the three reviewers for their valued comments on this study.
Disclosure statement
No potential conflict of interest was reported by the author(s).
Additional information
Funding
References
- Amanda, J. C. S. (2007). Swarm robotics and minimalism. Connection Science, 19(3), 245–260. https://doi.org/10.1080/09540090701584970
- Bai, P., Liang, X., & Wang, P. (2016). Research on new air combat system of aircraft swarms. Journal of Air Force Engineering University(Military Science Edition), 16(2), 1–4.
- Barbara Webb. (2002). Swarm intelligence: From natural to artificial systems. Connection Science, 14(2), 163–164. https://doi.org/10.1080/09540090210144948
- Boyd, J. R. (1996). The Essence of Winning and Losing. http://www.defense-and-society.org/fcs/ppt/boyds_ooda-_loop.ppt.
- Chen, Z., Wei, J., Wang, Y., & Zhou, R. (2011). UAV autonomous control levels and system structure. Acta Aeronautica et Astronautica Sinica, 32(6), 1075–1083.
- Chen, Z., Yang, L., Rodríguez, R. M., Xiong, S., Chin, K., & Martínez, L. (2021b). Power-average-operator-based hybrid multi-attribute online product recommendation model for consumer decision-making. International Journal of Intelligent Systems, 36, 2572–2617.
- Chen, Z. S., Zhang, X., Rodríguez, R. M., Pedrycz, W., & Martinez, L. (2021a). Expertise-based bid evaluation for construction-contractor selection with generalized comparative linguistic electre iii. Automation in Construction, 125, 1–18.
- Chmait, N. (2017). Understanding and measuring collective intelligence across different cognitive systems: An information-theoretic approach. Proceedings of twenty-sixth International joint Conference on Artificial intelligence.
- Chmait, N., Lowe, D. L., Green, D. G., & Li, Y. F. (2015). Observation, communication and intelligence in agent-based systems. Proceedings of International Conference on Artificial general intelligence, Springer International Publishing.
- Clough, B. T.. (2002). Metrics, schmetrics! How the heck do you determine a UAV’s autonomy anyway. The Performance Metrics for Intelligent Systems Workshop, Gaithersburg.
- Commuri, S., Li, Y., Hougen, D., & Fierro, R. (2004). Evaluating intelligence in unmanned ground vehicle teams. Proceedings of the Performance Metrics for intelligence systems workshop.
- Dai, A., Zhao, Z., Li, R., Zhang, H., & Zhou, Y. (2020). Evaluation mechanism of collective intelligence for heterogeneous agents group. IEEE Access, 8, 28385–28394. https://doi.org/10.1109/ACCESS.2020.2971278
- Daniel, P. (2016). Gremlins. http://www.darpa.mil/program/gremlins
- DARPA Public Affairs. (2016). OFFSET Envisions Swarm Capabilities for Small Urban Ground Units. http://www.darpa.mil/news-events/2016-12-07
- Donna, S.-M. (2017). Reverse engineering the human: Artificial intelligence and acting theory. Connection Science, 29(1), 64–76. https://doi.org/10.1080/09540091.2016.1271398
- Duan, H., & Qiu, H. (2018). Unmanned aerial vehicle swarm autonomous control based on swarm intelligence. Science Press.
- Fan, B., & Zhang, R. (2017). Unmanned aircraft system and artificial intelligence. Geomatics and Information Science of Wuhan University, 42(11), 1523–1529.
- Fidel, A., Mar, P., & Ramón, R. (2012). Macroscopic definition of distributed swarm morphogenesis. Connection Science, 24(4), 162–192. https://doi.org/10.1080/09540091.2013.795931
- Goodrich, M. A., Crandall, J. W., & Stimpson, J. L. (2003). Neglect tolerant teaming: issues and dilemmas. AAAI Spring Symposium on Human Interaction with Autonomous Systems in Complex Environments.
- Harrod, S. (2009). Capacity factors of a mixed speed railway network. Transportation Research Part E: Logistics and Transportation Review, 45(5), 830–841. https://doi.org/10.1016/j.tre.2009.03.004
- Huang, H., Pavek, K., Novak, B., Albus, J., & Elena, M. (2005). A framework for autonomy levels for unmanned systems(ALFUS). Proceeding of the AUVSI's Unmanned Systems North America, Baltimore, MD.
- Jia, Y., Tian, S., & Li, Q. (2019). Development of unmanned aerial vehicle swarms. Acta Aeronautica et Astronautica Sinica, 41(21), 723–738.
- Larry, A. Y., Jeffrey, A. Y., & Mark, D. G. (2005). System analysis applied to autonomy: Application to high-altitude long-endurance remotely operated aircraft. Proceedings of the AIAA Infotech Aerospace Conference(pp.22-31). Arlington, Virginia: AIAA.
- Li, D., Liu, C., & Gan, W. (2009). A new cognitive model: Cloud model. Wiley Subscription Services, Inc. A Wiley Company, 24(3), 357–375.
- Li, J., & Qiu, C. (2019). A personalised ontology ranking model based on analytic hierarchy process. International Journal of Computational Science and Engineering, 19(4), 518. https://doi.org/10.1504/IJCSE.2019.101882
- Li, Y. L., Ying, C. S., Chin, K. S., Yang, H. T., & Xu, J. (2018). Third-party reverse logistics provider selection approach based on hybrid-information mcdm and cumulative prospect theory. Journal of Cleaner Production, 195, 573–584.
- Liang, X. L., Zhang, J. Q., & Lv, N. (2018). UAV swarms. Northwestern Polytechnical University Press.
- Liu, D., Yin, Y. X., Tu, X. Y., & Dong, J. (2007). Qualitative evaluation on intelligent characteristics of intelligent system. Computer Engineering and Application, 43(11), 1–3.
- Liu, H. X. (2012). Fuzzy mathematics theory and Its application. Science Press.
- Liu, L., Zhao, Y., Zhao, X., Li, Z., & Li, Y. (2017). Design of a simulation platform of unmanned swarm system. Journal of CAEIT, 12((05|5)), 68–74.
- Office of Naval Research. (2015). LOCUST: Autonomous, Swarming UAVs Fly into The Future. http://www.onr.navy.mil/en/Media-Center/Press-Releases/2015/LOCUST-low-cost-UAV-swarms-ONR.aspx
- Office of the Secretary of Defense. (2000). Unmanned aerial vehicles roadmap 2000-2025. Technical Report. Department of Defense, Washington.
- Office of the Secretary of Defense. (2002). Unmanned aerial vehicles roadmap 2002-2027. Technical report. Department of Defense.
- Office of the Secretary of Defense. (2005). Unmanned aircraft system roadmap 2005-2030. Technical Report. Department of Defense, Washington.
- Pan, Q., Zhou, D. Y., & Yu, J. (2012). Effectiveness evaluation of unmanned air vehicle combat system based on cloud model. Command Control and Simulation, 34(6), 68–71.
- Reynolds, C. W. (1987). Flocks, herds, and schools: A distributed behavioral model. ACM SIGGRAPH Computer Graphics, 21(4), 25–34. https://doi.org/10.1145/37402.37406
- Shuai, Z., Xue-Ren, L., Peng, Z., & Qing, G. (2016). UAV autonomy evaluation based on multi-factors fuzzy evaluation. Proceedings of 2016 7th International Conference on Mechanical and Aerospace Engineering, ICMAE 2016: 487-491. doi:10.1109/ICMAE.2016.7549589.
- Sun, J., Wang, J., Chen, J., & Ding, G. (2020). Cooperative communication based on swarm intelligence: Vision, model, and key technology. SCIENTIA SINICA Informationis, 50(3), 307–317. https://doi.org/10.1360/SSI-2019-0186
- Wang, C., & Liu, J. (2012). Evaluation methods for the autonomy of unmanned systems. Chinese Science Bulletin, 57(15), 1290–1299. https://doi.org/10.1360/csb2012-57-15-1290
- Wu, J. Z., Wang, M., & Yuan, M. (2016). Evaluation method and application of mechanical and electrical products’ remanufacture-ability based on fuzzy-eahp. Modular Machine Tool & Automatic Manufacturing Technique, (9), 153–156.
- Wu, W. L., & Zhou, X. S. (2020). An Intelligent Evaluation Method of Application Scenario Complexity Level of Unmanned Swarms. Proceedings of 19th International Conference on Ubiquitous Computing and Communications, Exeter, UK.
- Wu, W. L., & Zhou, X. S. (2021). Description model and qualitative evaluation of intelligence characteristics of unmanned swarms. Journal of Computers, 32(4), 80–93.
- Yan, Y., & Tang, Z. M. (2012). Autonomy evaluation method of ground intelligent robot based on cloud model. Journal of Nanjing University of Science & Technology, 36(3), 420–426.
- Yang, C. Y., & Cai, W. (2013). Extenics: Theory, method and application. Science Press.
- Yang, X. S. (2018). Principles of intelligence. Electronics Industry Press.
- Yang, Z., & Zhang, R. (2009). Fuzzy evaluated method for the autonomy levels of unmanned systems. Journal of Chinese Computer Systems, 2009(10), 125–129.
- Zhang, H. F., Han, F. L., & Pan, C. P. (2019). Operational effectiveness evaluation of uav to sea assault based on cloud model. Ordnance Industry Automation, 038(004), 57–61.
- Zhang, T., Li, Q., Zhang, C., Lang, H., Li, P., Wang, T., Li, S., Zhu, Y., & Wu, C. (2017). Current trends in the development of intelligent unmanned autonomous systems. Frontiers of Information Technology & Electronic Engineering, 18(1), 68–85. https://doi.org/10.1631/FITEE.1601650
- Zhang, Y. (2015). U.S. Air-Launched Drone Swarm Moving towards Real Combat. http://www.myzaker.com/article/58d6ade51bc8e047080000026.
- Zhang, Y. N., Liu, X. W., Du, Y., & Liu, Y. (2014). Durability evaluation of concrete structure based on fuzzy extension ahp. Applied Mechanics & Materials, 454, 179–182.
- Zhao, J., & Song, Y. (2016). Slope stability evaluation based on improved entropy weighted-cloud model. Water Resources and Power, 34(4), 120–122 + 165.
- Zhou, Y., Rao, B., & Wang, W. (2020). UAV swarm intelligence: Recent advances and future trends. IEEE Access, 8, 183856–183878. https://doi.org/10.1109/ACCESS.2020.3028865
- Zsca, B., Xll, A., Ksc, C., Wp, D., Kltb, C., Miroslaw, J., & Skibniewski, e. f. g. h. (2021). Online-review analysis based large-scale group decision-making for determining passenger demands and evaluating passenger satisfaction: Case study of high-speed rail system in china. Information Fusion, 69, 22–39. doi:10.1016/j.inffus.2020.11.010