ABSTRACT
The objective of this study was to assess the predictive performance of midDRIFTS-PLSR models in quantifying total carbon (TC), total organic carbon (TOC), total inorganic carbon (TIC), total nitrogen (TN), hot water extractable carbon and nitrogen (CHWE, NHWE), pH, and the clay, silt, and sand content of soils. A total of 68 soil samples were taken across an agroecological region in southwest Iran, and analyzed in the laboratory using mid-DRIFTS-PLSR. midDRIFTS-PLSR calibration models were developed, and external validation was performed for each of the soil properties via an independent algorithm. The calibration and validation models allowed for a sufficient prediction of TC, TIC, and TOC with residual prediction deviations ≥3 and R2 values >0.9. The precise prediction ofcarbon fractions, such as TC, TIC, and TOC, in a rapid and inexpensive manner confirmed that midDRIFTS analysis was a rapid-throughput and cost-effective technique for monitoring soil carbon at the regional scale.
1. Introduction
Soil provides a large carbon pool that responds quickly to environmental changes and global warming [Citation1,Citation2]. Soil organic carbon (SOC) has several functions and provides ecosystem services through its crucial role in carbon cycling and greenhouse gas exchanges between the soil and atmosphere. It is also widely acknowledged to be one of the most significant factors affecting soil fertility and plant growth [Citation3]. Therefore, SOC monitoring is given a high priority in sustainable management strategies and environmental assessments [Citation4].
Assessment of soil quality and indicators of soil quality remains challenging [Citation5,Citation6]. Soil characteristics vary spatially and temporally, and soil monitoring is therefore essential for early diagnosis of changes in soil quality [Citation7].
Several studies have proposed programs for monitoring soil quality [Citation8,Citation9]. Most of the proposed monitoring schemes use a data set of the important physical, biological, and chemical properties of soils [Citation10]. Soil characteristics are selected from the data set and normalized to obtain a global soil quality index for a particular soil property or specific ecosystem [Citation8,Citation9]. However, the monitoring of soil quality indicators is very expensive and time consuming because it requires long term sampling (five to ten years) and many soil analyses are required by soil monitoring networks [Citation11] to detect changes in soil quality [Citation7]. Proximal soil sensing (PSS) is a modified sampling design that benefits from the use of cheaper laboratory measurements and portable field spectrometers. Spectroscopic methods, such as portable X-ray fluorescence (PXRF) devices and visible-infrared (Vis-IR) spectroscopy, are used in PSS and can provide rapid measurements of soil contaminants [Citation12,Citation13].
Many studies have shown that both the visible-near infrared (400–700–2500 nm) and mid-infrared (2500–25,000 nm) ranges are suitable for estimating various soil properties such as total carbon (TC), SOC, total nitrogen (TN), and pH values [Citation14,Citation15,Citation16]. However, differentiation of the carbon and nitrogen pools in soils using infrared spectroscopy has not yet been achieved [Citation17,Citation18].
Various studies that have estimated soil properties have shown mid-infrared spectroscopy (MIRS) to be more useful than near-infrared spectroscopy (NIRS). For example, Reeves et al. [Citation19] found that MIRS achieved generally better results than NIRS for different soil carbon pools. McCarty et al. [Citation20] and McCarty and Reeves [Citation21] reported that results of TC, SOC, TN, and pH analyses using vis-NIRS and NIRS were not as good as those obtained using MIRS. Michel et al. [Citation22] reported that MIRS could be a powerful method for estimating carbon and nitrogen using artificial soil mixtures. Vohland et al. [Citation23] predicted soil properties such as TOC, TN, microbial biomass carbon (Cmic), hot water extractable carbon (CHWE), and soil pH by mid-infrared diffuse reflectance Fourier transform spectroscopy (midDRIFTS) in a study of 60 samples using cross-validation models. However, some studies have found that vis-NIRS was better than MIRS for estimating exchangeable aluminum and potassium [Citation12].
Although infrared-based estimations of soil properties may not be as precise as classical laboratory analyses, they are useful for many research goals and applications [Citation16].
midDRIFTS coupled with partial least squares regression (PLSR) is a technique used to predict a variety of soil properties [Citation12,Citation22]. Despite the successful application of infrared spectroscopic analysis for predicting a range of different soil properties, most studies that have used this technique have concentrated on soils from temperate and tropical regions. Few studies have used spectral data to quantify soil properties at different scales and under different land uses in arid and semiarid regions, such as Iran.
The soil in Iran is calcareous and contains a diverse range of carbonate minerals. Interference with spectral bands representing soil organic matter (SOM) functional groups is an important issue in the analysis of calcareous soils. There are several absorbance bands in the MIR range that are influenced by soil carbonate, including the bands at 2686–2460, 1850–1784, 1567–1295, 889–867, 734–719, and 719–708 cm–1 [Citation24,Citation25]. Thus, interference between organic matter and carbonates may affect model accuracy and prevent the use of particular spectral peaks for analysis [Citation26], which may be problematic for monitoring of very heterogeneous soils. Thus, interference by carbonates must be taken into consideration when calcareous soils are studied. The main objective of our study was to verify the ability of midDRIFTS-PLSR models to predict soil carbon fractions in calcareous soil from southwest Iran.
2. Materials and methods
2.1. Study area and soil sampling
Soil samples were collected at 20 locations in Lorestan Province in southwest Iran. All the soils in Lorestan Province are calcareous, with pH values above seven. The area is located in a geomorphological basin between 1000 and 2000 m above sea level. The climate is mild, with a mean annual precipitation and temperature of 350 mm and 17.7°C, respectively. The study area was chosen to represent the variations throughout the study region. Soil samples were taken from three different land uses: forest, range, and crop lands. Various cereals, but predominantly wheat, were cultivated in the crop lands. All selected forest and grassland areas had a southern orientation with slopes <30°. For each land use, we established study plots and divided each plot into four subplots. Four replicated points were chosen in each subplot, and in the last step four replicated samples were mixed into one sample to reduce the number of samples. A total of 68 samples from the surface horizons (0–30 cm) were taken during 2016. At each sampling point, five subsamples were taken and mixed to form a composite sample. The soil samples were stored in plastic bags and kept in cool conditions. Soil samples were oven-dried at 32°C for 48 h and then crushed and sieved (<2 mm) using a mechanical sieve. A 10-g subsample was further ball milled for the midDRIFTS analyses described below.
2.2. Conventional laboratory analysis of soil samples
Soil samples were analyzed to determine their carbon and nitrogen fractions, texture, and pH. The Bouyoucos hydrometer method was applied to determine soil texture. Measurements of soil pH were conducted in 0.01 M CaCl2 with a soil:solution ratio of 1:2.5 (m:v) [Citation27]. Carbon and nitrogen extracted with hot-water were referred to as CHWE and NHWE, respectively, according to Schulz and Körschens [Citation28], and analyzed with a Multi N/C analyzer (Analytik Jena, Jena, Germany). TC and TN were measured using a dry combustion method [Citation29] with a Vario-EL III elemental analyzer (Elementar, Hanau, Germany).
2.3. Determination of Total Organic Carbon (TOC) and Total Inorganic Carbon (TIC) by combustion and gas analysis
All soil samples used in this study contained both calcite and dolomite. Therefore, the VDLUFA-Verlag soil analysis methods [Citation29,Citation30] were used for organic carbon and carbonate measurements.
Ball-milled soil samples were maintained at 32 ºC overnight before analysis. Briefly, around 10 g of each soil sample was weighed into a ceramic crucible which was then placed into a furnace at 500 ºC for 6 h. After 6 h, TIC was measured in samples by dry combustion [Citation29] with a Vario-EL III elemental analyzer (Elementar) and TOC was reported as the difference between soil TC and TIC measured by the dry combustion method [Citation29].
2.4. MidDRIFTS analysis of soil samples
The midDRIFTS analysis was performed according to Demyan et al. [Citation31] and Mirzaeitalarposhti et al. [Citation16]. Ball-milled soil samples were kept at 32 ºC overnight before the analysis. A subsample of approximately 200 mg was scanned using a Tensor-27 mid-infrared spectrometer (Bruker Optik GmbH, Ettlingen, Germany) equipped with a potassium bromide (KBr) beam splitter and a liquid nitrogen-cooled mid-band mercury-cadmium-telluride detector., A praying mantis diffuse reflectance chamber (Harrick Scientific Products Inc., New York, NY, USA) was installed in the spectrometer. A chamber filled with dry air from a compressor (Jun-Air International, Nørresundby, Denmark), with a flow rate of 200 L h–1, was used to reduce moisture and water interference. All subsamples were scanned with three replications in the mid-infrared range (4000–600 cm–1). The spectra were obtained by combining 16 individual scans at a resolution of 4 cm–1 recorded in absorbance units [log(1/reflectance)].
Prior to the analysis, the spectral average of triplicates was determined to generate a single spectrum for PLSR analysis. Dardenne et al. [Citation32] reported that the cross-validation model for a reduced number of samples, as in this study, provides an optimistic prediction of the actual performance.
Through a stratified random selection, a subset of 45 samples was selected for model calibration (calibration subset), while a subset of 23 other samples was selected for independent model validation (validation subset). For model development, the edges of each spectrum (reduced to 3900–700 cm–1) and the CO2 regions (2400–2300 cm–1) were excluded to reduce noise during model optimization.
Before optimization, the entire spectrum, with the exception of CO2 regions, was divided into ten equal portions of 177 cm–1. Optimization of the PLSR models in this study was achieved through the use of a successive projection algorithm, which used different combinations of predefined frequency regions and data preprocessing methods. The most informative and relevant frequency regions in the frequency domain were selected by the successive projection algorithm to develop precise calibration models [Citation33]. Pre-processing transformations of spectral data were used to improve the accuracy of PLSR models through noise reduction and to offset the reduction in raw spectra. We used the following optimization procedures: constant offset elimination (COE), straight line subtraction (SLS), vector normalization (SNV), multiplicative scatter correction (MSC), first derivative (FD), second derivative (SD), FD+SLS, SD+SNV, and FD+MSC [Citation16]. midDRIFTS-PLSR model calibration and model validation were performed for each soil with the OPUS version 7.0 software package (Bruker Optik GmbH, Ettlingen, Germany).
2.5. Data analysis and assessment of model accuracies
Descriptive and multiple regression analyses were conducted with the SPSS (version 14) software [Citation34]. A regression analysis was conducted to test for correlations between the predicted and measured values of each property of interest using SigmaPlot software. The accuracy of midDRIFTS-PLSR models was evaluated by the residual prediction deviation (RPD), the coefficient of determination (R2), and the root mean square error (RMSE) of independent models. In the current study, models with RPDs greater than 5 were considered to be ‘‘excellent”. Models with RPDs between 3 and 5 were considered to be “acceptable”, while RPDs between 1.4 and 3 were considered to be “moderately successful” [Citation35], and models with RPDs less than 1.4 were considered to be ‘unsuccessful’
3. Results
3.1. Soil properties and correlation analysis
As can be seen in , the values of the measured soil properties for forest land were higher (p < 0.01) than for the two other land uses, except for pH, TIC, and silt content. This was also true for CHWE (1168 vs. 493 and 725 mg kg–1, respectively) and TOC (4.31% vs. 1.4% and 1.64%, respectively). For soil texture, higher mean sand contents were observed in range land (56.93%) compared to the forest land (50.74%). By calculating the coefficient of variation (CV), we found that 6 out of 10 soil properties in forest land had a higher variability than in range land and agricultural land (). Of all the soil properties, TN varied the most, with a CV of approximately 104% for forest land.
Table 1. Selected chemical and physical properties of soils under three land use types (n = 68).
shows the correlation between soil properties from all three land uses. TOC and TN were strongly correlated. There were positive correlations among TC, TIC, and TOC (p < 0.001). As an important soil quality indicator, TOC was correlated positively not only with carbon pools, i.e. TC and CHWE (R2 = 0.4 and 0.55, respectively; p < 0.001), but also with nitrogen pools, i.e. TN and NHWE (R2 = 0.9 and 0.47, respectively). The clay content was not significantly correlated with most soil properties. Significant positive correlations with the clay content were only found for silt and pH.
Table 2. Spearman correlation between soil properties (n = 68).
3.2. Spectral features of soil
The baseline corrected and average spectra of all samples from each land use are shown in . Similar shapes but different peak heights in the spectra were observed. As shown in , the soil varied in texture and physicochemical soil properties, which is reflected in the soil spectrum in .
Figure 1. Average mid-infrared diffuse reflectance Fourier transform spectroscopy (midDRIFTS) spectra of bulk soil derived from the three land uses in the Kaka Reza region.
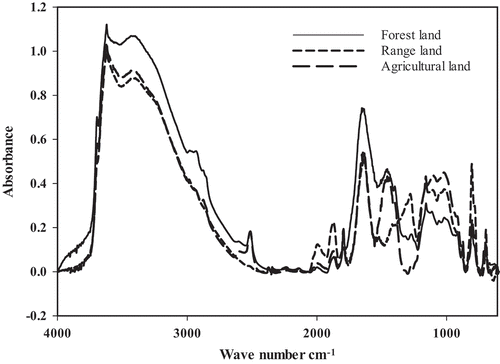
Several peaks throughout the spectrum were assigned to different minerals and organic functional groups [Citation14]. In the 2100–600 cm–1 region, there were several peaks allocated to elemental molecular vibrations (e.g. quartz O–Si–O) and several peaks that were associated with organic compounds [Citation36]. There was a maximum spectral feature in the 3700–3500 cm–1 region, which was mainly due to the presence of hydroxyl stretching vibrations in combination with clay minerals. Dixon and Weed [Citation37] reported that O–H in the 3690–3620 cm–1 region and Si–O stretching near the 1100–1000 cm–1 region might be related to clay minerals. According to Stevenson [Citation38], small peaks between 2960 and 2860 cm–1 could be related to the presence of aliphatic groups. In the forest land spectra, this band was more evident and may be related to higher SOC concentrations. All the soil samples in this study were collected from calcareous soils. Nguyen et al. [Citation39] found that carbonate peaks were mainly present in the region of 2600–2500 cm–1 and quartz produced peaks at 1980 and 1870 cm–1, with higher absorbance in agricultural land. A prominent peak from 2000 to 1500 cm–1 appeared in soils from all three land uses and was related to the double bonds the C = C and C = O groups [Citation40]. The peak near 1160 cm–1 was an easily decomposed organic compound with C–O bonds in poly-alcoholic groups, with the highest peak occurring in agricultural land [Citation36].
3.3. Calibration of midDRIFTS-PLSR models
and show the calibration results for the soil properties. Excellent calibration models were achieved for TC, TIC, and TOC with R2 values ≥0.96 and RPD values ≥3.
Table 3. Calibration results for the investigated soil properties [calibrated mid-infrared diffuse reflectance Fourier transform spectroscopy coupled with partial least square regression (midDRIFTS-PLSR) models; n = 45].
Figure 2. Scatter plots of measurements made by conventional laboratory analyses and values predicted by midDRIFTS-PLSR models for a: total carbon (TC), b: total organic carbon (TOC), and c: total inorganic carbon (TIC). The dashed line represents the 1:1 line. (The model accuracy indicators are shown in and ).
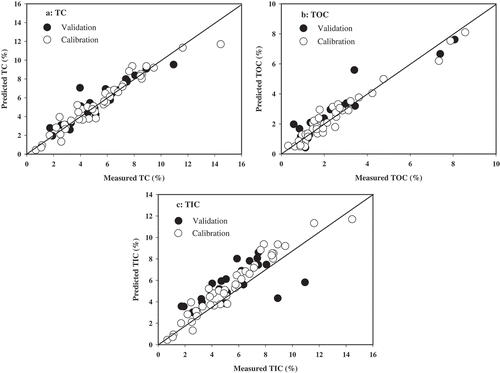
Moderately successful calibration models were found for CHWE with R2 and RPD values of 0.77 and 2.09, respectively.
Moderately successful calibration models were obtained for NHWE, pH, clay, sand, and silt contents with RPDs <3 (). Different PLSR factors were used for the calibration, varying from 2 for pH to 9 for the TIC, TN, and silt content of soils. The smallest RMSECV and largest R2 values corresponded to the optimal number of factors used during calibration. Each soil property had specific absorption bands that corresponded to some of the frequency ranges. Soil carbon fractions such as TC, TIC, TOC, and CHWE had greater frequency ranges than the other measured parameters ().
Model validation is summarized in and . Prediction accuracies were low for all soil properties, with RPDs of only 0.9–3.52. Lower R2 and RPD values were obtained for the sand, silt, and clay contents than for the other parameters (). The prediction results were clearly weaker when the independent model was applied for model validation. The results showed acceptable predictions for TIC, whereas the predictions for other soil properties varied from unsuccessful to moderately successful.
Table 4. Prediction accuracies of calibrated mid-infrared diffuse reflectance Fourier transform spectroscopy coupled with partial least square regression (midDRIFTS-PLSR) models () applied to a validation subset (n = 23).
4. Discussion
4.1. Application of the model
The application of midDRIFTS in this study confirmed its ability to predict physicochemical and biological soil properties, with varying degrees of accuracy [Citation41,Citation42]. However, there were two issues that potentially affected the model performance including the number of samples, similarity or diversity of data sets, soil texture, and the range of the property of interest [Citation43]. A midDRIFTS spectrum contains complex information on soil organic and inorganic compounds. The active vibrations of the molecular bonds of different organic and inorganic compounds may overlay each other and affect the model performance. Although the most informative spectral bands are used by PLSR models to reduce complexity and increase model accuracy, spectral overlap and interference may still prevent a successful model calibration. Therefore, the predictive ability of the midDRIFTS model is based on calibration models that relate spectral data to soil properties.
The results of this study revealed that midDRIFTS-PLSR calibrated models were suitable for the prediction of soil properties. Despite the careful calibration of independent models for SOC, pH, and sand, silt, and clay contents, the prediction accuracies were classified as unsuccessful. Less accurate predictions were obtained for CHWE, NHWE, and TN, while predictions of TC, TOC, and TIC values were good. For pH, the inaccurate prediction was likely due to indirect spectral responses.
Cobo et al. [Citation44] studied soils with various textures from three villages in Zimbabwe. The soils had different sand contents, ranging between 25% and 75%, and an RPD value of 6.8 was found for very sandy soil. This could be related to the overlap of Si–O peaks with SOM peaks [Citation45]. A sufficiently heterogenous sample set is needed to ensure a suitable calibration [Citation46].
Therefore, the inaccurate predictions of the sand, clay, and silt content of soils could be related to differences in the particle size distribution. Inaccurate results also were obtained for soil CHWE and NHWE, which agreed with observations made in other studies [Citation23,Citation45]. The relatively low levels of microbiological carbon in the soil samples (less than 5% of TOC) were poorly represented in the spectral measurements, with peaks overlaid by peaks associated with soil minerals and other organic materials [Citation45,Citation47]. Because of the close relationship with SOM quality, microbiological carbon was expected to be well predicted [Citation48].
As shown in , a significant positive correlation was found for both CHWE and NHWE with TOC. The range of variability, soil type, site, and number of samples may have resulted in the different model performances [Citation49,Citation50]. For the calibration of a reliable PLSR prediction model, a certain amount of heterogeneity is needed [Citation46]. Successful predictions of soil carbon levels in several soils have been achieved using midDRIFTS [Citation16,Citation51,Citation52]. As an example, in the midDRIFTS-PLSR model obtained for TOC, several bands were assigned to organic matter, carbonate, and other soil constituents such as iron oxides and clay minerals. Thus, the model may be constructed based on the bands corresponding to organic compounds or other soil minerals such as iron oxides and clay [Citation53]. Because soils are extremely diverse and variable, making the midDRIFTS-PLSR model useful for predicting soil properties remains a challenging task.
The predictive performance of the PLSR model requires sufficient heterogeneity in a study region. However, if heterogeneity exceeds a certain level there may be adverse effects.
5. Conclusions
This study examined the combination of midDRIFTS with PLSR, with the results confirming that the technique was capable of predicting TC, TOC, and TIC in soils under three different land uses by evaluating the different values of various soil properties. The midDRIFTS-PLSR models developed in this study gave good predictions of the carbon content of soils and were able to make accurate predictions of the soil carbon fractions in ‘unknown’ samples from the study region by analyzing their midDRIFTS spectra without the necessity for any further laboratory measurements. Thus, in situations with an inadequate sample size (<100), as in this study, midDRIFTS-PLSR models can be used to obtain accurate data. The heterogeneity of the data used for calibration has a large effect on calibration and prediction accuracy. For favorable midDRIFTS-PLSR predictive models of soil properties in various regions, the development of accurate predictive models based on a large sample size and adequate heterogeneity is necessary.
In conclusion, our results revealed that the application of midDRIFTS to accurately predict carbon levels in soils was appropriate and the method has a strong potential to make spectrum-based predictions. midDRIFTS-PLSR models can act as a rapid-throughput approach for future spectrum-based predictions in different regions, but large numbers of soil samples are necessary to establish the models because of the unknown soil conditions in many areas.
Acknowledgments
The authors would like to thank the technical staff of the Institute of Crop Science at the University of Hohenheim for providing excellent support services. The corresponding author would like to thank the Ministry of Science, Research, and Technology in Iran for providing expenses to conduct research in Germany.
Disclosure statement
No potential conflict of interest was reported by the authors.
References
- Lary R, Bachelet B, Bellocchi G, Hill DRC. Towards vulnerability minimization of grassland soil organic matter using metamodels. Environ Modell Softw. 2014;52:38–50.
- Luo ZK, Wang EL, Shao QX, et al. Confidence in soil carbon predictions undermined by the uncertainties in observations and model parameterization. Environ Modell Softw. 2016;80:26–32.
- Banitz T, Gras A, Ginovart M. Individual-based modeling of soil organic matter in Netlogo: transparent, user-friendly, and open. Environ Modell Softw. 2015;71:39–45.
- Viscarra-Rossel RA, Behrens T. Using data mining to model and interpret soil diffuse reflectance spectra. Geoderma. 2010;158:46–54.
- Doran JW, Parkin TB. Defining and assessing soil quality. In: Doran JW, Coleman DC, Bzedicek DF, et al., editors. Defining soil quality for a sustainable environment,Vol. 35. Madison (WI): Soil Science Society of America Special Publication. 1994. p. 3–21.
- Doelman P, Eijsackers H. Vital soil: function, value, and properties. Developments in soil science. Amsterdam: Elsevier; 2004. p. 29.
- Morvan X, Saby NPA, Arrouays D, et al. Soil monitoring in Europe: A review of existing systems and requirements for harmonisation. Sci Total Environ. 2008;391:1–12.
- Andrews SS, Karlen DL, Cambardella CA. The soil management assessment framework: a quantitative soil quality evaluation method. Soil Sci Soc Am J. 2004;68:1945–1962.
- Velasquez E, Lavelle P, Barrios E, et al. Evaluating soil quality in tropical agroecosystems of Colombia using NIRS. Soil Biol Biochem. 2005;37:889–898.
- Doran JW, Safley M. Defining and assessing soil health and sustainable productivity. In: Pankhurst CE, Doube BM, Gupta VVSR, editors. Biological indicators of soil health. Wallingford, UK: CAB International; 1997. p. 1–28.
- Jolivet C, Boulonne L, Ratié C. Manuel du Réseau de Mesures de la Qualité des Sols, Edition. France: Unité Infosol; 2006.
- Juang KW, Lee DY, Teng YL. Adaptive sampling based on the cumulative distribution function of order statistics to delineate heavy-metal contaminated soils using kriging. Environ Pollut. 2005;138(2):268–277.
- Juang KW, Liao WJ, Liu TL, et al. Additional sampling based on regulation threshold and kriging variance to reduce the probability of false delineation in a contaminated site. Sci Total Environ. 2008;389(1):20–28.
- Viscarra Rossel RA, Walvoort DJJ, McBratney AB, et al. Visible, near infrared, mid infrared or combined diffuse reflectance spectroscopy for simultaneous assessment of various soil properties. Geoderma. 2006;131:59–75.
- Heinze S, Vohland M, Joergensen RG, et al. Usefulness of near-infrared spectroscopy for the prediction of chemical and biological soil properties in different long-term experiments. J Plant Nutr Soil Sci. 2013;176:520–528.
- Mirzaeitalarposhti R, Demyan MS, Rasche F, et al. MidDRIFTS-PLSR-based quantification of physicochemical soil properties across two agroecological zones in Southwest Germany: generic independent validation surpasses region specific cross-validation. Nutr Cycl Agroecosys. 2015;102:265–283.
- Zimmermann M, Leifeld J, Fuhrer J. Quantifying soil organic carbon fractions by infrared-spectroscopy. Soil Biol Biochem. 2007;39:224–231.
- Ludwig B, Linsler D, Hörper H, et al. Pitfalls in the use of middle-infrared spectroscopy for the estimation of soil properties: representativeness of a sample for a specific population & criteria for ranking infrared estimations as useful. Geoderma. 2016;268:165–175.
- Reeves JB, Follett RF, McCarty GW, et al. Can near or mid- infrared diffuse reflectance spectroscopy be used to determine soil carbon pools? Commun Soil Sci Plant. 2006;37:2307–2325.
- McCarty GW, Reeves JB, Reeves VB, et al. Mid-infrared and near-infrared diffuse reflectance spectroscopy for soil carbon measurement. Soil Sci Soc Am J. 2002;66:640–646.
- McCarty GW, Reeves JB. Comparison of near infrared and mid infrared diffuse reflectance spectroscopy for fieldscale measurement of soil fertility parameters. Soil Sci. 2006;171:94–102.
- Michel K, Terhoeven-Urselmans T, Nitschke R, et al. Use of near- and mid-infrared spectroscopy to distinguish carbon and nitrogen originating from char and forest-floor material in soils. J Plant Nutr Soil Sci. 2009;172:63–70.
- Vohland M, Ludwig M, Thiele-Bruhn S, et al. Determination of soil properties with visible to near- and mid-infrared spectroscopy: effects of spectral variable selection. Geoderma. 2014;223–225:88–96.
- Tatzber M, Mutsch F, Mentler A, et al. Determination of organic and inorganic carbon in forest soil samples by midinfrared spectroscopy and partial least squares regression. Appl Spectrosc. 2010;64:1167–1175.
- Ji J F, Ge Y, Balsam W, et al. Rapid identification of dolomite using a fourier transform infrared spectrophotometer (FTIR): a fast method for identifying Heinrich events in IODP Site U1308. Mar Geol. 2009;258:60–68.
- Calderon FJ, Mikha MM, Vigil MF, et al. Diffuse-reflectance mid-infrared spectral properties of soils under alternative crop rotations in a semi-arid climate. . Commun Soil Sci Plant Anal. 2011a;42:2143–2159.
- Anderson JM, Ingram JSI. Tropical soil biology and fertility: a handbook of methods. Wallingford: CAB International; 1993.
- Schulz E, Körschens M. Characterization of the decomposable part of soil organic matter (SOM) and transformation processes by hot water extraction. Eurasian Soil Sci. 1998;31:809–813.
- ISO 10694. Soil quality. Determination of organic and total carbon after dry combustion (elementary analysis). Wallingford: International Organization for Standardization, Geneva; 1995; 1993.
- Boden, VDLUFA-Verlag, Darmstadt. Determination of organic carbon by combustion and gas analysis. In: The Association of German Agricultural Research Institutes, editor. Handbook of agricultural methods of experimental and investigation (VDLUFA-Methodenbuch). Speyer, German; 2016. p. 1–3.
- Demyan M S, Rasche F, Schulz E, Breulmann M, Müller T, Cadisch G. Use of specific peaks obtained by diffuse reflectance Fourier transform mid-infrared spectroscopy to study the composition of organic matter in a Haplic Chernozem Eur J Soil Sci. 2012;63:189–199.
- Dardenne P, Sinnaeve G, Baeten V. Multivariate calibration and chemometrics for near infrared spectroscopy. Which method? J Near Infrared Spectrosc. 2000;8:229–237.
- Arau´Jo MCU, Saldanha TCB, Galva˜o RKH, et al. The successive projections algorithm for variable selection in spectroscopic multicomponent analysis. Chemometr Intell Lab Syst. 2001;57:65–73.
- Rencher AC. Methods of multivariate analysis. 2th ed. New York (NY): John Wiley & Sons, Inc; 2002.
- Pirie A, Singh B, Islam K. Ultra-violet, visible near-infrared, and mid-infrared diffuse reflectance spectroscopic techniques to predict several soil properties. Aust J Soil Res. 2005;43:713–721.
- Spaccini R, Piccolo A. Molecular characterization of compost at increasing stages of maturity. 1. Chemical fractionation and infrared spectroscopy. J Agric Food Chem. 2007;55:2293–2302.
- Dixon JB, Weed SB. Minerals in soil environments. Madison: Soil Science Society of America; 1989.
- Stevenson FJ. Humus chemistry: genesis, composition, reactions. New York (NY): Wiley; 1994.
- Nguyen TT, Janik LJ, Raupach M. Diffuse reflectance infrared Fourier transform (DRIFT) spectroscopy in soil studies. Aust J Soil Res. 1991;29:49–67.
- Baes AU, Bloom PR. Diffuse reflectance and transmission Fourier transform infrared (DRIFT) spectroscopy of humic and fulvic acids. Soil Sci Soc Am J. 1989;53:695–700.
- Madari BE, Reeves JB III, Machado PLOA, et al. Mid- and near-infrared spectroscopic assessment of soil compositional parameters and structural indices in two Ferralsols. Geoderma. 2006;136:245–259.
- Grinand C, B G B, Brunet D, et al. Prediction of soil organic and inorganic carbon contents at a national scale (France) using mid-infrared reflectance spectroscopy (MIRS). Eur J Soil Sci. 2012;63:141–151.
- Bellon-Maurel V, McBratney A. Near-infrared (NIR) and mid-infrared (MIR) spectroscopic techniques for assessing the amount of carbon stock in soils—critical review and research perspectives. Soil Biol Biochem. 2011;43:1398–1410.
- Cobo J G, Dercon G, Yekeye T, Chapungu L, Kadzere C, Murwira A, Delve RCadisch G. Integration of midinfrared spectroscopy and geostatistics in the assessment of soil spatial variability at landscape level. Geoderma. 2010;158:398–411.
- Janik LJ, Merry RH, Skjemstad JO. Can mid infrared diffuse reflectance analysis replace soil extractions? Aust J Exp Agric. 1998;38:681–696.
- Ce´Cillon L, Barthe`S BG, Gomez C, et al. Assessment and monitoring of soil quality using near-infrared reflectance spectroscopy (NIRS). Eur J Soil Sci. 2009;60:770–784.
- Ludwig B, Nitschke R, Terhoeven-Urselmans T, et al. Use of mid-infrared spectroscopy in the diffuse-reflectance mode for the prediction of the composition of organic matter in soil and litter. J Plant Nutr Soil Sci. 2008;171:384–391.
- Rasche F, Marhan S, Berner D, et al. MidDRIFTS-based partial least square regression analysis allows predicting microbial biomass, enzyme activities and 16S rRNA gene abundance in soils of temperate grasslands. Soil Biol Biochem. 2013;57:504–512.
- McBratney AB, Minasny B, Viscarra Rossel R. Spectral soil analysis and inference systems: a powerful combination for solving the soil data crisis. Geoderma. 2006;136:272–278.
- Kuang B, Mouazen AM. Calibration of visible and near infrared spectroscopy for soil analysis at the field scale on three European farms. Eur J Soil Sci. 2011;62:629–636.
- Linsler D, Geisseler D, Loges R, et al. Temporal dynamics of soil organic matter composition and aggregate distribution in permanent grassland after a single tillage event in a temperate climate. Soil Till Res. 2013;126:90–99.
- Soriano-Disla JM, Janik LJ, Viscarra Rossel RA, et al. The performance of visible, near-, and mid-infrared reflectance spectroscopy for prediction of soil physical, chemical, and biological properties. Appl Spectrosc Rev. 2014;49:139–186.
- Brown DJ, Bricklemyer RS, Miller PR. Validation requirements for diffuse reflectance soil characterization models with a case study of VNIR soil C prediction in Montana. Geoderma. 2005;129:251–267.