Abstract
We investigated how native and fluent users of English segment continuous speech and to what extent they use sound-related and structure-related cues. As suggested by the notion of multi-competence, L1 users are not seen as ideal models with perfect command of English, and L2 users not as lacking in competence. We wanted to see how language experience affects speech segmentation. We had participants listen to extracts of spontaneous spoken English and asked them to mark boundaries between speech segments. We found that in chunking authentic speech, both groups made the most use of prosody, with L1 users relying slightly more on it. However, the groups did not differ in segmentation strategies and performed alike in efficiency and agreement. Results show that in line with multi-competence, native speakers do not have an advantage over fluent speakers in higher-level speech processes, and that the outcome of natural speech comprehension does not significantly depend on the different language experiences at high levels of fluency. We suggest that research on speech segmentation should take natural continuous speech on board and investigate fluent users independently of their L1s to gain a more holistic view of processes and consequences of speech segmentation.
Introduction
A person’s aggregated experience of a language affects many—perhaps all—aspects of their command of that language. It is therefore generally assumed that fluent L2 speakers (henceforth referred to as ‘fluent speakers’) and native speakers of a given language are fundamentally different. However proficient fluent speakers become, they remain forever ‘near-native’ (Ringbom, Citation1993) at best, if measured by the same criteria of proficiency. There is a wealth of research supporting this assumption, notably in studies of learner language from a variety of angles. However, if we think of fluent speakers not solely in terms of learners, but embrace Cook’s (Citation2007, Citation2016) notion of multi-competence, we see that native speakers are not necessarily perfect performers, and that fluent L2 speakers can often have higher metalinguistic abilities (Barac & Bialystok, Citation2012).
Within the vast domain of second language acquisition (SLA) research, the line of research that is directly relevant to our present interest is that of speech segmentation by native and other fluent speakers. In most cases, this means contrasting L1 speakers with L2 learners. Although many such studies have shown that language processing is affected by language experience (Cutler et al., Citation1983, Citation1986; Johnson & Newport, Citation1991; Sanders & Neville, Citation2000; Tremblay et al., Citation2016), their research designs have typically focused on the processing of linguistic artefacts or elements separated from their natural contexts (i.e. phones or words), which is a poor representation of the spontaneous speech encountered in everyday life. The question arises: would the same results also hold for more naturalistic speech processing, which more closely represents real-life language use?
We set out to explore the question of how, if at all, L1 users and L2 users differ in processing continuous spontaneous speech as they hear it. We asked L1 users and fluent L2 users to segment, or chunk up, extracts of continuous authentic speech. We invited them to do this intuitively, without specifying what a ‘chunk’ was. We specifically looked at one higher-order factor (syntax) and one lower-order factor (prosody) involved in assigning chunk boundaries.
The framework
Perspectives on language competence
Experience of a language varies widely among users with some command of it, with consequences for the way it is processed. Broadly speaking, it is possible to distinguish three groups among users of a given language: those who learned it as their first language (‘L1 users’), those who learned it as a later language but are competent users (‘L2 users’) and those who are in the process of acquiring it (‘learners’). All three groups have some command of the language; however, while ‘learners’ are relatively easy to identify as a separate group, discrimination between L1 and L2 users is still a matter of debate in linguistic research (Dewaele, Citation2018).
There are two main views on this issue. One—typical of SLA research—is the traditional monolingual perspective, which assumes that L1 users have essentially perfect knowledge of a language, and that while L2 users are able to learn it, they never reach the same level of proficiency (Ellis & Ellis, Citation1994; Towell & Hawkins, Citation1994). The other is the bilingual (or multilingual) perspective. This conceptualisation acknowledges that speakers do not necessarily possess perfect competence in their L1 (i.e. real speakers are not ideal speakers), and therefore it is pointless for L2 users to strive for such perfection either. Cook (Citation2016) uses the term multi-competence to describe the latter case. Multi-competence challenges the perception of native speakers representing the ‘gold standard’, as is typical of the monolingual approach. Instead, an L2 user’s different languages are seen not as so many deficient systems, but rather as one connected system. This bilingual repertoire is, in effect, advantageous to the L2 user: the benefits of multi-competence have been proposed by studies in numerous fields, from classroom teaching and learning (multi-competence can make learning additional languages easier, Hofer, Citation2017) to language identity (multilingual speakers tend to better maintain a sense of self-esteem in challenging situations, Dörnyei, Citation2009). Therefore, while experience undoubtedly differs between L1 and L2 users in both quantity and quality, the former are neither homogeneous nor perfect, and the latter can be more aware of the language and have higher metalinguistic abilities (Barac & Bialystok, Citation2012; Jessner, Citation1999).
The current study investigates speech processing in adult individuals with a very strong command of English (‘L1 users’ and ‘L2 users’). In line with Cook (Citation2007), we take L1 users to be native speakers of English belonging to the English-speaking community, and L2 users to be static speakers, not learners, who use their L2 as part of their repertoire for their own purposes. We wanted to step away from testing performance primarily in relation to language proficiency, and instead find out how divergent language exposure in terms of L1 and L2 experience may affect speech perception, specifically in speech segmentation. We focused on how L1 and L2 users intuitively segment continuous speech.
Cues in speech segmentation
The relationship between speech perception and language experience has been investigated before. One way to explore how speech perception is affected by language experience is a segmentation task (e.g. Cole et al., Citation2017; Mattys et al., Citation2010; Sanders et al., Citation2002). During the task, listeners are instructed to segment spoken input, typically by reporting or marking segment boundaries. Participants then use their knowledge of the language structure and certain heuristics to make quick decisions regarding what they consider to be a segment. This task comes in different shapes and forms depending on the experimental purpose: from syllables (e.g. Goyet et al., Citation2010; Otake et al., Citation1993) to meaningful segments (Anurova et al., Citation2022; Vetchinnikova et al., Citation2022).
Segmentation of smaller linguistic units, such as phones or words, is strongly affected by the quality of the signal; studies therefore often present such stimuli in adverse conditions, e.g. with volume variation, background noise and time compression. These intentional task complications have an expected effect on performance: L1 users clearly outperform L2 users when perceiving speech in noise (Cooke & Scharenborg, Citation2008; Mayo et al., Citation1997; Pinet et al., Citation2011) and when experiencing reverberation (Takata & Nábělek, Citation1990). One explanation is suggested by Lecumberri et al. (Citation2010): while L1 users are able to fall back upon their extensive experience to combine all linguistic means when dealing with imperfect signals, L2 users may either have insufficient skills or be slower in exploiting their repertoire.
Yet when we move to higher levels of linguistic units, such as sentences, the situation is far less clear. Contrary to our intuitive impression, free-flowing speech is not reliably divided by acoustic gaps. The listener is therefore forced to use other linguistic and metalinguistic cues to segment spontaneous spoken input into chunks that would be linguistically and psychologically meaningful. The findings so far agree that users with different experiences rely on different segmentation cues (e.g. Sanders & Neville, Citation2000; Tremblay et al., Citation2016). Mattys et al. (Citation2005) roughly divide such cues into three tiers: Tier I consists of lexical or structural cues (syntactic and semantic), Tier II of segmental cues (distributional and phonotactic), and Tier III of metrical cues. Their model suggests that in normal conditions, L1 users have the strongest reliance on Tier I, since these cues are maximally informative for identifying upcoming information. The tiers are therefore viewed as a gradient: from lower-order cues that relate to sound (prosodic and metrical cues, Tiers II and III) to higher-order cues that relate to structure (syntactic cues, Tier I). All of these cues affect L1 segmentation as well as L2 segmentation; therefore, studies often choose to compare L1 and L2 users.
Prosodic pattern is one of the earliest linguistic characteristics that infants learn; it naturally follows that prosodic cues are sensitive to a speaker’s L1. Studies have repeatedly shown that although both L1 and L2 users rely on prosodic patterns during speech recognition, the extent to which they do this depends on their L1 (Sanders et al., Citation2002). The most studied patterns are stress and rhythm. Adult speakers of languages with different stress patterns have been shown to use their native language stress cues to segment speech in English (while Spanish speakers rely on metrical stress, Japanese speakers rely on tone, Archibald, Citation1997). Rhythm and syllable structure influence segmentation in L1 users as well. Rhythm has been shown to influence Japanese speech segmentation by native Japanese speakers, but not by native English speakers listening to either English or Japanese (Cutler & Otake, Citation1994; Otake et al., Citation1993). Syllable structure has been found to influence segmentation of syllable-timed French by native speakers (Cutler et al., Citation1983, Citation1986), but the same effect was not demonstrated in native English speakers with fluent French (Cutler et al., Citation1989; Cutler & Butterfield, Citation1992). These findings suggest that L2 users do not acquire prosodic segmentation cues beyond those in their L1, or acquire them to a lesser degree than L1 users. Thus, the prosodic characteristics of a language are important in determining how L1 users of that language will segment speech in any other language.
However, it is worth bearing in mind that many prosodic cues are not language-specific, but rather universal. Among strong universal cues we find, for example, final lengthening (syllables at the end of a prosodic unit are lengthened in many languages), phonemes at the beginning of prosodic units (phonemes are more strongly articulated at the beginning than in other positions within the units), and transitions between prosodic units such as intonational phrases (signalled by an abrupt change in pitch) (for a review, see Endress & Hauser, Citation2010; Barbosa & Raso, Citation2018; Mello & Raso, Citation2019). To make use of universal cues in spontaneous speech, often no knowledge of the language is required: when you listen to a dialogue in an unknown language, you probably have no problem detecting the beginning and the end of an utterance. In the present study, we investigate adult individuals with extensive knowledge of English (see 3.1, Participants). While they undoubtedly make use of universal cues, we are interested in the degree to which language-specific prosodic cues influence L2 segmentation.
There are fewer studies on the use of syntactic cues during speech segmentation, mainly on account of the difficulties in experimental design: syntactic structure is often not obvious until the onset of the next word. The evidence so far suggests that even though both L1 and L2 users rely on syntax in speech perception (Tremblay et al., Citation2018), L1 users do so to a greater extent (Johnson & Newport, Citation1991). Different language experience (e.g. age of acquisition, characteristics of L1, learning conditions, exposure) has been found to affect L2 syntactic processing, while having very little effect on semantic processing (Hahne & Friederici, Citation2001; Weber-Fox & Neville, Citation1996). As in the case of prosody, syntactic characteristics of a speaker’s L1 have been found to be important in that they directly affect speech segmentation of another language.
Thus, segmentation cues are invaluable for studying how users with different language experience comprehend speech: findings suggest that some systems are flexible and largely independent of the L1 (e.g. semantics), while others are relatively fixed and rarely change during L2 acquisition (e.g. syntax and prosody). In the multi-competence paradigm, we can regard these findings as helping us understand how the different languages spoken by an L2 user can function as one connected system, and how speech perception is organised in general.
Despite abundant studies on speech segmentation cues, we hitherto know little about how they are used beyond sentences. This knowledge gap is evident in, for example, the persistent difficulties in developing automatic speech recognition systems, in which speech comprehension requires the segmentation of continuous streams of unpolished sound into recognisable units.
The present study
Prosodic and syntactic segmentation at the levels of phones and words have been extensively studied, but are still not well understood in spontaneous speech. The problem seems to stem largely from commonly-used experimental designs. Firstly, the data is usually artificially constructed (from sounds, words, sentences, or text paragraphs), which is not an ecologically valid way to capture the normal speech segmentation process. Secondly, the participants are often given specific tasks (e.g. click when they hear a certain word) which are unlikely to reflect natural, effortless speech segmentation.
In our study, we address both problems. To tackle the first, we use speech data recorded in natural settings. Using such spontaneous speech extracts allows us to capture the intuitive process of segmenting continuous speech in real time (Mauranen, Citation2018; Sinclair & Mauranen, Citation2006). The second problem is best obviated by aiming for as little instruction as possible, in order to maintain spontaneity in the segmentation process. This has been achieved in some studies (i.e. Cole et al., Citation2017; Ventsov & Kasevich, Citation1994; Vetchinnikova et al., Citation2017), which have shown that when L2 users spontaneously segment speech into manageable units, they tend to converge on their segmentation choices (Vetchinnikova et al., Citation2022); that is, they show significant agreement on the size and frequency of those units.
However, to our knowledge, there are no studies that compare speakers with different language experience in terms of the degree to which they may converge in spontaneous segmentation of natural speech.
The current exploratory study investigates segmentation cues that are used by native and fluent L2 speakers of English as they segment continuous speech. We are interested in whether L1 and L2 users chunk speech in the same way and what strategies they use to make segmentation choices. The research question is: how much, if at all, do native and fluent L2 speakers of English differ in segmenting natural spontaneous speech?
Previous research has suggested that during sentence segmentation, L1 users exploit cues of Tier I and Tier II (syntax and prosody) to a greater extent than L2 users (Sanders et al., Citation2002). In this study, we investigate the extent to which L1 and L2 users’ reliance on segmentation cues of Tier I and Tier II might differ during natural speech comprehension beyond sentence or utterance level. Therefore, we hypothesise that
when listening to continuous speech, L1 users will rely on syntactic and prosodic cues more than L2 users.
However, looking at the bigger picture, when listening to continuous speech with the purpose of extracting meaning, all speakers of a language are likely to employ the full range of cues they have at hand (Sanders & Neville, Citation2000) – lexical, segmental, and metrical. Therefore, we hypothesise that
when listening to continuous speech, differences in segmentation strategies will level out, resulting in equal levels of agreement on chunk boundaries and chunks of equal size among L1 and L2 users.
We predict that even if L1 and L2 users employ different strategies to segment continuous speech, this will not affect the outcome. In agreement with the multi-competence perspective, we expect results to show that the ultimate outcome of natural speech comprehension does not depend on language experience, and that native speakers do not have an advantage over fluent L2 speakers in speech segmentation.
Design and method
Participants
Two groups of participants took part in this study: 27 L1 users and 29 L2 users. We used Prolific,Footnote1 an online participant recruitment service, to invite L1 users, and recruited L2 users in person in Helsinki, Finland. The difference is due to limitations imposed by the COVID-19 pandemic: we were not able to interact with participants in person during the data collection process for the L1 group; therefore, the experiment took place online. A detailed description of both groups is provided in .
Table 1. Description of participant groups.Footnote8
Research shows that L1 affects L2’s speech perception (e.g. Kartushina & Frauenfelder, Citation2014), especially when it comes to prosodic patterns (e.g. Goad & White, Citation2004). In this study, we did not intend to explore individual languages or the intonation effects of all the L1s involved. Instead, we treated L2 participants as a multilingual group with various backgrounds, a ‘collective L2 user’, and purposely varied their native languages as much as possible for a more balanced experimental design. We looked for a general L2 effect, to compare it to an L1 group.
All participants were naïve to the purpose of the experiment and were asked to sign an informed consent form prior to the study. None of them reported having a background in linguistics and their level of education varied from upper secondary school to a university degree. Everybody received a reward for their participation: L1 users received an experimental fee recommended by Prolific, and L2 users a movie ticket. Compensation fees for both groups were comparable.
Stimuli
We selected 50 speech extracts from two corpora of academic spoken English: the MICASE CorpusFootnote2 (Simpson et al., Citation2002) and the ELFA CorpusFootnote3 (Mauranen & Ranta, Citation2008). We chose continuous speech extracts that were 20–35 seconds long, with few hesitations, few technical terms, and few proper names. The extracts were chosen so that each would contain a meaningful passage. We also selected one training extract and three practice extracts with the same criteria as the actual extracts. These extracts were not included in the analysis.
After selecting the extracts, we re-recorded them: a fluent speaker of English read them aloud in a professional studio, replicating the intonation patterns from the original data. We understand that imitation is a problematic task since, for instance, in American English, speakers who perform imitation often change the nature of the phonetic cues (e.g. pause duration) (Cole & Shattuck-Hufnagel, Citation2011). In our case, imitation was essential in order to perform the automatic prosodic annotation (see Section 4.2). This type of annotation reduces the subjective judgement involved in prosodic analysis performed manually by listeners (as in Cole et al., Citation2017), but requires high sound quality. Our original data was spontaneous speech recorded in authentic settings, which meant that there were multiple speakers and occasionally considerable background noise. Imitation was the best way to eliminate noise and to engage only one speaker’s voice in the experiment.Footnote4
To eliminate intensity differences, all final audio files were scaled to the same root-mean-square (RMS) amplitude before the experiment. They were then resampled to 22 kHz to decrease the weight of the files.
Procedure
The experimental procedure for both groups followed three steps, which are described in more detail below: (i) background questionnaires, (ii) chunking task, (iii) Elicited Imitation Task.
Before and after the experiment, participants filled in a background questionnaire and a feedback form respectively. In the former, we asked about their education, age, gender, language use, and possible hearing problems; in the latter, we asked them to self-assess their performance and strategies used during the main task. No personal data was collected: instead, each participant was assigned a random combination of letters and numbers as an identifier.
For the chunking task, we used a web-based application: the ChunkitApp (Vetchinnikova et al., Citation2017). It is designed to play speech extracts through headphones while displaying their transcripts on a tablet screen (). Individual words in the transcripts are separated by interactive tilde symbols (∼) that allow participants to physically mark their segmentation choices. The instruction for participants read as follows:
Figure 1. Extract from ChunkitApp. The ‘∼’ sign marks potential boundaries between each orthographic word. If tapped, a boundary ( ‘|’ ) appears.
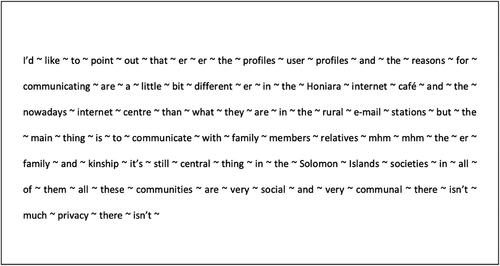
Humans process information constantly. When we take in information, we tend to break it up quickly into small bits or chunks. We ask you to work intuitively. Your task is to mark boundaries between chunks by clicking ‘∼’ symbols. One click makes the boundary appear. If you click the symbol again, the boundary will disappear. If you lose the line in the text, stay with the speaker and do not try to go back.
No explanation was provided as to what ‘chunks’ meant. Each extract was followed by a yes/no comprehension question.
To assess the participants’ proficiency in English, we adopted an Elicited Imitation Task (EIT, Ortega et al., Citation2002) that has proved to be an effective measure of implicit L2 grammatical knowledge (Erlam, Citation2006; Spada et al., Citation2015). This task requires participants to listen to a number of sentences, one by one, and write them down as precisely as possible. As the task proceeds, the sentences increase in both length and syntactic complexity. Since we have adopted the multi-competence framework, we tested both L1 and L2 users to capture any difference in performance between the groups. We used a slightly modified version of the EIT for participant convenience (24 sentences in total). For L1 users collected online, the EIT was implemented via the ChunkitApp; for L2 users collected offline, it was implemented using LimeSurvey.
To collect L1 data, we integrated the ChunkitApp with the Prolific platform. Only participants with tablets and headphones could proceed with the experiment. For L2 data collection, we used fifth-generation iPads and JBL Tune600BTNC headphones with an active noise cancellation feature. The whole experimental session took about an hour, with the chunking task itself taking about 30 minutes.
The L1 group took the test online within Prolific, a crowd-sourcing platform for running online studies. The researcher was not present during the task, but completion could only be approved if a participant used the correct devices and progressed through all three stages (background questionnaires, chunking task, Elicited Imitation Task).
The L2 group took the test offline, in a classroom at the University of Helsinki, Finland. One of the researchers was present during the task.
Analysis and results
Outliers
We excluded participants from the analysis based on undesired language experience, technical issues, low proficiency in English or misunderstanding of the task.
Out of 48 participants in the L1 group, 21 were excluded. Three of the respondents turned out to be linguists, and three reported having hearing problems. Two participants performed significantly more poorly than the others in the Elicited Imitation Task (see Section 4.3, threshold based on IQR), and eight participants’ data was missing due to technical error. Another five participants were excluded based on interquartile range, where any observations that are more than 1.5 IQR below Q1 or more than 1.5 IQR above Q3 are considered outliers. A possible explanation for this exclusion could be misunderstanding of the task—those participants chunked almost every single word.
Out of 34 participants in the L2 group, five were excluded. One was an L1 user who accidentally took part in the experiment (we did not include him in the L1 group; L1 speakers completed the experiment online and L2 speakers offline, and it was important to maintain uniformity in experimental settings); two performed significantly more poorly than others in the Elicited Imitation Task (see Section 4.3, threshold based on IQR), and data for two other participants was missing due to technical error.
In total, 27 L1 users and 29 L2 users were included in the analysis.
Variables
Data pre-processing included preparation of variables that we later used for the analysis of segmentation strategies and agreement.
Our dependent variable was segmentation choice at the participant level; that is, whether a participant marked (1) or did not mark (0) a boundary in every between-word space in the transcripts.
Our independent variables were prosodic and syntactic cues, which were defined independently of boundary placement and annotated in a similar way to Anurova et al. (Citation2022).
For prosodic cues, we used predicted boundary strength – a variable that predicts the prominence of the boundary between two orthographic words. Like Anurova et al. (Citation2022), we utilised the Wavelet Prosody Toolkit (Suni, Citation2017; Suni et al., Citation2017), an unsupervised program that calculates fundamental frequency, energy envelope and duration features from a given speech extract and performs continuous wavelet transform (CWT) on them. During CWT, each word boundary is checked for energy minima, fundamental frequency minima and the minima of differences between durations of adjacent words. Minima across all those scales in the resulting scalograms constitutes a prosodic boundary. As a result, the program assigns a numerical value to each prosodic boundary across the extract. The strength of a prosodic boundary is a decimal number that ranges from 0.000 (no boundary) to infinity. The higher the number, the stronger the prosodic boundary.
For syntactic cues, we annotated each potential boundary between all orthographic words as clausal or non-clausal, drawing on several recent descriptive English grammars (Biber et al., Citation1999; Huddleston & Pullum, Citation2002; Kaltenböck et al., Citation2011; Lohmann & Koops, Citation2016). Our annotation resulted in four types of boundaries:
C/C – where one clause ends and another begins;
C/NC – where a clause ends but neighbours non-clausal material;
NC/C – where non-clausal material ends and a clause begins;
NC/NC – inside a clause, or between two semantically-unrelated non-clausal elements.
An example of an extract featuring all four annotation types is shown below in :
Language proficiency and comprehension accuracy
All of the following analyses were carried out using programming language R version 4.0.5 (2021-03-31).
We first ensured that the participants had approximately the same level of English and that they were able to answer more than half of the comprehension questions.
To calculate language proficiency, we compared participants’ answers to the 24 sentences of the Elicited Imitation Task using Levenshtein Distance, with 0 being the best score and 63 being the worst score (number of all characters in all sentences divided by the number of sentences). Here, we had to exclude two L1 and two L2 users due to low performance in the EIT (threshold based on IQR; those with a score superior to 30 in ).
Figure 3. EIT scores for L1 and L2 users (two outliers in each group).Footnote9
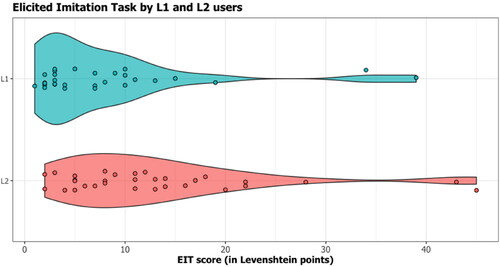
To calculate comprehension rate, we looked at the percentage of correctly answered questions among all participants. Since we had two possible answers to each question (yes/no), anyone who answered more than 50% of the questions (above chance) correctly was considered successful. Participants in both groups performed considerably above chance (L1: mean comprehension rate = 92.9%; L2: mean comprehension rate = 93.0%).
Unpaired two-samples Wilcoxon rank sum test with continuity correction showed a significant difference between EIT results for the groups (W = 210, p < .05 with a moderate effect size, Shapiro test showed non-normality of distribution). According to the results, L1 users performed significantly better in the Elicited Imitation Task than L2 users (see ). By contrast, we did not reveal any statistically significant differences between the groups based on mean comprehension rate (W = 381.5, p > .05). EIT results had a slight correlation with comprehension questions for both groups (L1 Spearman r = −0,46, p < .05; L2 Spearman r = −0.54, p < .05; ), meaning that participants with the best performance in answering comprehension questions had a higher estimated level of English.
shows the analysis of comprehension questions (y axis, with 0% being the worst score) and language proficiency (x axis, with 0 being the best score). Although L2 users performed slightly less well in proficiency (, y axis), this did not affect their success in answering the questions (, x axis). In both groups, the highest scores in comprehension rate (y axis) corresponded to the lowest EIT scores (y axis, close to 0), meaning participants with a high level of English answered the questions well.
Segmentation cues
Based on previous research (Sanders et al., Citation2002), we hypothesised that L1 users would rely on syntactic and prosodic cues to a greater extent than L2 users.
In this analysis, we wanted to understand which factors contributed to the segmentation choices participants made in the experiment. Our goal was to model boundary placement at the individual level; that is, whether a participant marked a boundary in a particular place. To that end, we fitted prosodic and syntactic variables (IVs, see Section 4.2) into a binomial logistic regression with mixed effects (fixed + random intercepts [extract and participant]). The reason for choosing beta-binomial regression was that the DV distribution is considerably skewed (64.8% of zeroes).
The full model included three fixed effects: prosodic boundary as a continuous variable, clausal boundary as a factor with four levels, and condition (L1 vs L2); two- and three-way full interactions between those factors, and extract and participant as random intercepts. We fitted the model using the lme4 package (Bates et al., Citation2015). The prosodic boundary variable was centred. We used L1 as the reference level for condition and the absence of clausal boundary as the reference level for factor variables. We applied the dummy coding scheme for factor variables because we aimed to compare each level of both predictors to the reference levels.
The backward model selection approach indicated that the three-way interaction did not improve the model. We then dropped it, continuing forward with the model with two-way interactions. Residual diagnostics with the DHARMa package (Hartig & Hartig, Citation2017) confirmed the goodness of fit, but collinearity and variance inflation factor (VIF) analysis confirmed that prosodic boundary strength and clausal boundaries were highly collinear, with a VIF of 99 for the clausal boundaries.Footnote5
Linguistic attributes are often unavoidably collinear (Tomaschek et al., Citation2018). To combat this issue in a way that still made theoretical sense, we transformed the clausal boundary variable into an ordinal one with the following levels: 0 to indicate the absence of a clausal boundary, 1 to indicate a clause ending or beginning, and 2 to indicate the presence of at least two clausal boundaries. We also centred the transformed variable and fitted the same full model with three fixed effects, their two-way interactions and participant and extract as random intercepts. The three-way interaction was again found to be insignificant through backward model selection. The residual diagnostics confirmed the validity of the model. VIFs for all variables and their interactions were below 5, indicating that we could interpret the model coefficients ().
Table 2. Logistic regression coefficients. Significant effects are marked in bold.
Prosodic boundary strength and clausal boundary strength, as well the interaction between the prosodic boundary strength and condition were statistically significant. The condition and the interaction between condition and clausal boundary strength were not. The R2 (Marginal 0.49/Conditional 0.59) values demonstrated a moderate effect size and a significant degree of individual variability, as reflected by the random effects’ contribution to the variance. However, this must be interpreted with caution, since there is no consensus in the statistical community regarding how this measure should be calculated for generalised mixed effects models (Piepho, Citation2019).
Based on the intercept coefficient and the fact that we did not find a statistically significant effect of condition, it can be said that the probability of both L1 and L2 users of English marking a boundary when both prosodic and clausal boundary strength are at their mean—that is, in the absence of both these cues—is just 2%. Due to the nature of the task, boundaries could not be marked after every word, or even after every second word. In fact, if participants had behaved in this way, it would have indicated that they either did not understand the task or perhaps did not understand the language in which the task was presented. The same goes for clausal and prosodic boundaries: a clause or a prosodic unit usually consists of several words. Therefore, both the dependent and independent variables contain a lot of zeroes, and their means are skewed towards 0 as well.
shows the visualisation of the model and features the probability of marking a boundary depending on the type of cue, the condition, and their interaction. Both the graph and the model coefficients for all the predictors and their interactions were positive, which means that the stronger any of the linguistic cues (or their combination), the higher the probability of a boundary appearing.
The graph shows that, in general, the stronger the prosodic boundary, the higher the probability of it being marked—for both groups. The strength of a prosodic boundary is a decimal value from 0 to infinity, and all boundaries with CWT values larger than 0 are ‘prosodic’. In our speech extracts, the CWT value did not originally exceed 3. After centring, boundaries with values closer to 0 were weaker, and those closer to 5 were stronger.
When a strong prosodic boundary was also a clausal boundary, the probability of it being marked increased further. The significant interaction between condition and prosodic boundary strength indicated that L2 users of English might be more sensitive to prosodic cues than L1 users. If a prosodic boundary was strong but there was no clausal boundary, they were less likely to mark the end of the chunk (although the likelihood of a boundary being marked was still very high).
To investigate this result further, we fitted a series of logistic regression models identifying cue importance for both groups and compared them using Akaike Information Criterion (the lower the AIC is, the better the model fits). For both groups and for each group individually, we first fitted the baseline model with the random intercepts only, then added clausal boundary strength, then subsequently dropped this predictor and added the prosodic boundary strength. The AIC comparison (shown in below) demonstrates that the order of the predictors was the same for both groups, with prosodic boundaries making a more significant contribution to the variance than syntactic ones.Footnote6
Table 3. AIC comparison for predictor importance evaluation.
Therefore, our hypothesis was not confirmed; L1 users did not differ from L2 users in terms of which cues they used for speech segmentation. However, prosodic cues were more important than syntactic for both groups, with native speakers relying on prosody slightly more than fluent speakers when the syntactic boundary was not realised.
Agreement and chunk length
We hypothesised that the chunks produced by both L1 and L2 users would be essentially similar, i.e. that participants would converge on the chunk boundaries they marked, and the resulting chunks would not differ in size.
Probably the simplest way to assess inter-participant agreement in chunking behaviour is to look at observed agreement; that is, the number of raters in agreement as a proportion of the total possible number of raters. However, as argued by Cohen (Citation1960), it is possible that under uncertainty raters simply guess and some agreement arises due to chance. We therefore chose to use Fleiss’ kappa (Fleiss et al., Citation2003), as it accounts for chance agreement. It expresses the degree to which the observed proportion of agreement among raters exceeds what would be expected if all raters made their ratings completely at random. This measure ranges from 0 to 1, with 0 indicating no agreement and 1 indicating absolute agreement. Several studies have shown that Fleiss’ kappa values are comparable across populations and experimental conditions: American English recruited offline (κ = 0.51) and American English (κ = 0.43)/Indian English (κ = 0.23) recruited online (Cole et al., Citation2017); fluent English speakers (κ = 0.45) (Vetchinnikova et al., Citation2022). Although it is not an ideal measure to assess inter-dependent choices in language, as imbalance in the number of responses across categories can produce underestimates of consensus (Feinstein & Cicchetti, Citation1990), results from previous studies show that it is conceptually possible to use Fleiss’ kappa to investigate participant agreement rate (for detailed discussion, see Vetchinnikova et al., Citation2022).
To investigate the size of the units that participants came up with, we computed what we call chunk length; that is, the mean number of orthographic words between non-random boundaries. One of the important questions when discussing boundary frequency is how to understand whether people chunked speech on a random basis or not. To that end, we used a Monte Carlo-based permutation test that estimated the probability of our results being random. We set the false discovery rate to 0.05 and found a significance of 8 for L1, and 9 for L2, meaning that if 8/9 participants or more inserted a boundary, it was considered statistically significant. These significant boundaries were used to calculate chunk length for each group.
Results for agreement (Fleiss’ kappa) and for the size of chunks (in words) for both groups are provided in .
Table 4. Agreement and chunk length in words for native and fluent speakers.
The results show that agreement among both L1 speakers and fluent L2 speakers was moderate and is comparable between the groups. Moreover, the mean chunk lengths were equal (approximately 7 words). Therefore, our hypothesis was supported: L1 and L2 users agreed on chunk boundaries to the same extent, and these chunks did not differ in size between the groups.
Discussion
This study investigated segmentation of continuous speech among native and other fluent speakers of English in the light of two main hypotheses: (1) L1 users will rely on syntactic and prosodic cues more than L2 users, but (2) both groups will agree on chunks of the same size and to the same degree.
Results showed that the groups did not differ in the cues they relied on. This contrasts with the findings of Sanders et al. (Citation2002), who found that during sentence segmentation, L1 users exploited cues of Tier I and Tier II (syntactic and prosodic) to a greater extent than L2 users. Our result means that in respect of syntax and prosody, native speakers did not significantly differ from other fluent speakers in the speech segmentation strategies they used. It also transpired that the outcome of speech comprehension—extracting meaning—was not notably affected by language experience. This interpretation is supported by EIT and comprehension questions: although our L1 and L2 users had significantly different levels of English, as measured by EIT, they answered the comprehension questions equally well. This finding supports the interpretation that both groups employed the full range of cues they had available, as suggested by Sanders and Neville (Citation2000).
We also found that prosodic factors were more important than syntax for both L1 and L2 users. This result is in line with e.g. Cresti et al. (Citation2018), who showed that prosody is crucial in spontaneous speech using methodology from the utterance-based Language into Act Theory (L-AcT). However, our data challenges earlier research (Mattys et al., Citation2005) which suggests the greater importance of syntax. The reason for the discrepancy may lie in the nature of the speech material—syntactic cues might be more important in sentence segmentation, as Mattys et al. (Citation2005) suggest; but when segmenting natural continuous speech, lower-order cues, which relate to sound (prosody), outperform higher-order cues, which relate to structure (syntax). The importance of prosodic cues over syntax also speaks for the validity of our method. Even though our participants viewed the text together with the audio, they attended to what they heard more than to what they read. We suggest that ChunkitApp could be used in speech segmentation studies on different language users, because it seems to capture aural speech perception.
The results also showed that native and fluent speakers agreed on chunking to the same extent when segmenting natural speech. Agreement measured by Fleiss’ kappa was comparable between the groups (L1 = 0.58; L2 = 0.54), as were the average lengths of chunks measured in words (L1 = 7.32; L2 = 7.61). The agreement measures correspond to previous studies that used similar methods: native English speakers (0.51; Cole et al., Citation2017) and fluent English speakers (0.45; Vetchinnikova et al., Citation2022). Although our two speaker groups differed in proficiency, the ability to respond to comprehension questions equally well suggests that L2 users are a relevant group in natural speech segmentation studies, instead of the more traditional approach of limiting experimental subjects to native speakers only. The apparent independency of continuous speech perception from language experience also speaks for the inclusion of speakers of different proficiency levels in speech segmentation studies.
In sum, we investigated how native and fluent speakers of English segment continuous speech and found that L1 and L2 groups performed differently in an EIT—native speakers were more proficient than other fluent speakers. This, however, did not affect their performance—they relied on linguistic cues in the same way, so that prosody was more important than syntax for both groups. Moreover, they extracted meaning equally well, and agreed on chunks of the same size to the same degree. Therefore, in a continuous speech segmentation task, L1 and L2 users did not differ. In line with multi-competence, the outcome of speech perception did not depend on experience, and native speakers did not seem to have an advantage over fluent speakers. Our results not only confirm the importance of prosody in speech comprehension, but also suggest that native and fluent speakers are interchangeable in studies of this kind.
This paper is the first exploratory study that compares speakers with different language experience in terms of their degree of convergence in spontaneous speech segmentation. It addressed a gap in research: although studies of speech segmentation abound, most of them use artificially-constructed data (sounds, words, sentences, or text paragraphs) or specific tasks that exclude spontaneity. In our study, we used speech data recorded in natural settings and instructed participants as little as possible, which allowed us to capture an intuitive segmentation process. Our results support the multi-competence paradigm, where L1 users and L2 users are seen as two separate groups, independent and sustainable on their own, but at the same time interdependent. We suggest that studies of speech segmentation should take natural continuous speech on board and investigate fluent users independently of their L1 status, in order to gain a more holistic view of speech segmentation.
Obviously, our study has its limitations. Firstly, the fact that participants had the text on screen prevents us from drawing firm conclusions about purely auditory speech segmentation. Although the results showed that both groups of listeners adhered to prosodic cues, the transcript was likely to play some role in this task. One suggestion for future research would be to develop a method in which participants would not see a transcript but could still mark boundaries in a similar way: for instance, by blurring the text or shadowing it. However, Anurova et al. (Citation2022) studied the brain responses to triggers set at chunk boundaries and non-boundaries obtained from a behavioural experiment with ChunkitApp. The participants of the brain experiment did not see any text, but their responses to triggers at boundaries and non-boundaries were significantly different. This can be seen as validation of the method used in the current study. Another limitation is within the L2 user group: we did not control for specific language levels (e.g. B1, B2, C1), but rather considered them a uniform community of ‘static L2 users’ (following Cook, Citation2007). It would be interesting to see how the language levels of L2 users affect their speech segmentation, if at all.
This was an exploratory study and gives rise to many new questions. We only investigated two independent variables—those most prominent in earlier research—but there may be others that reveal variable strategies in L1 and L2 users, or indeed finer user groupings. What does ‘using all available cues’ really mean? Importantly, as this was a quantitative study, we did not investigate the chunks themselves. Future qualitative studies could fruitfully explore the resulting chunks: the degree of similarity in chunks produced by L1 and L2 users, and what differences there might be. Future research might also consider the potential effects of language proficiency more carefully; for example, by investigating segmentation strategies in L2 learners and L2 users, or by looking for a threshold at which proficiency might affect agreement between chunkers. Furthermore, the types of cues could be investigated more thoroughly, e.g. by separating temporal cues from melodic cues and finding out how they are relied upon in a group of speakers.Footnote7
Supplemental Material
Download MS Word (15.4 KB)Supplemental Material
Download MS Word (15.4 KB)Disclosure statement
No potential conflict of interest was reported by the authors.
Additional information
Funding
Notes on contributors
Aleksandra Dobrego
Aleksandra Dobrego is a PhD candidate at the University of Helsinki, Finland. She has an international academic and research experience with a background in linguistics and cognitive science. Her research interests include online processing of continuous speech, and the linguistic factors that contribute to the listener’s understanding of spoken input, particularly prosodic cues.
Alena Konina
Alena Konina is a PhD candidate at the University of Helsinki, Finland. She has MA in Cognitive Studies and in Translation and Interpreting Studies. Her main research interests lie in online speech processing, reading, and simultaneous interpreting.
Anna Mauranen
Prof. (emer.) Anna Mauranen is Research Director at the University of Helsinki. Her current research focuses on linear modelling of spoken language and English as a lingua franca. Her most relevant publication for the current paper is Linear Unit Grammar (with Sinclair 2006). She is director of the Finnish Cultural Foundation-funded CLUMP project on chunking and the brain, and also director of several projects on spoken and written ELF, Global English, and Changing Englishes. Her recent publications have two foci, LUG and ELF.
Notes
1 https://www.prolific.co last accessed on 10.05.2021.
2 https://quod.lib.umich.edu/m/micase/ last accessed on 17.05.2021.
3 https://www.kielipankki.fi/corpora/elfa/ last accessed on 17.05.2021.
4 We thank an anonymous reviewer for suggesting the inclusion of a note on imitation.
5 We thank an anonymous reviewer for this suggestion.
6 These findings were corroborated by the random forest analysis that we performed to identify the order of cue importance for each of the groups. We do not include this analysis in the main text, because random forests do not work with random effects (Tomaschek et al., Citation2018) and are not particularly well adapted to treat interactions. We grew 1500 trees for each of the participant groups using the party package (Strobl et al., Citation2007).
7 We thank an anonymous reviewer for this suggestion.
8 The table contains information on participants after exclusion of outliers.
9 This and all following graphs were built using ggplot2 package in R (Wickham, Citation2016).
References
- Anurova, I., Vetchinnikova, S., Dobrego, A., Williams, N., Mikusova, N., Suni, A., Mauranen, A., & Palva, S. (2022). Event-related responses reflect chunk boundaries in natural speech. NeuroImage, 255, 119203.
- Archibald, J. (1997). The acquisition of English stress by speakers of nonaccentual languages: Lexical storage versus computation of stress. Linguistics, 35(1), 167–182. https://doi.org/10.1515/ling.1997.35.1.167
- Barac, R., & Bialystok, E. (2012). Bilingual effects on cognitive and linguistic development: Role of language, cultural background, and education. Child Development, 83(2), 413–422.
- Barbosa, P. A., & Raso, T. (2018). A segmentação da fala espontânea: aspectos prosódicos, funcionais e aplicações para a tecnologia [Spontaneous speech segmentation: Functional and prosodic aspects with applications for automatic segmentation]. Revista DE Estudos DA Linguagem, 26(4), 1361–1396. https://doi.org/10.17851/2237-2083.26.4.1361-1396
- Bates, D., Machler, M., Bolker, B., & Walker, S. (2015). Fitting linear mixed-effects models using lme4. Journal of Statistical Software, 67(1), 48. https://doi.org/10.18637/jss.v067.i01
- Biber, D., Johansson, S., Leech, G., Conrad, S., & Finegan, E. (1999). Longman grammar of spoken and written English. Longman.
- Cohen, J. (1960). A coefficient of agreement for nominal scales. Educational and Psychological Measurement, 20(1), 37–46. https://doi.org/10.1177/001316446002000104
- Cole, J., & Shattuck-Hufnagel, S. (2011). The phonology and phonetics of perceived prosody: What do listeners imitate? In 12th Annual Conference of the International Speech Communication Association.
- Cole, J., Mahrt, T., & Roy, J. (2017). Crowd-sourcing prosodic annotation. Computer Speech & Language, 45, 300–325. https://doi.org/10.1016/j.csl.2017.02.008
- Cook, V. (2007). Multi-competence: Black hole or wormhole for second language acquisition research? In Z. H. Han (Ed.), Understanding second language process (pp. 16–26). Multilingual Matters.
- Cook, V. (2016). Where is the native speaker now? TESOL Quarterly, 50(1), 186–189. https://doi.org/10.1002/tesq.286
- Cooke, M. P., & Scharenborg, O. E. (2008). The interspeech 2008 consonant challenge [Paper presentation]. https://doi.org/10.21437/Interspeech.2008-486
- Cresti, E., Gregori, L., Moneglia, M., & Panunzi, A. (2018, May). The Language into Act Theory: A pragmatic approach to speech in real-life. In Proceedings of the Eleventh International Conference on Language Resources and Evaluation (LREC 2018), LB-ILR2018 and MMC2018 Joint Workshop: Language and Body in Real Life Multimodal Corpora (pp. 20–25).
- Cutler, A., & Butterfield, S. (1992). Rhythmic cues to speech segmentation: Evidence from juncture misperception. Journal of Memory and Language, 31(2), 218–236. https://doi.org/10.1016/0749-596X(92)90012-M
- Cutler, A., & Otake, T. (1994). Mora or phoneme? Further evidence for language-specific listening. Journal of Memory and Language, 33(6), 824–844. https://doi.org/10.1006/jmla.1994.1039
- Cutler, A., Mehler, J., Norris, D., & Segui, J. (1983). A language-specific comprehension strategy. Nature, 304(5922), 159–160.
- Cutler, A., Mehler, J., Norris, D., & Segui, J. (1986). The syllable’s differing role in the segmentation of French and English. Journal of Memory and Language, 25(4), 385–400. https://doi.org/10.1016/0749-596X(86)90033-1
- Cutler, A., Mehler, J., Norris, D., & Segui, J. (1989). Limits on bilingualism. Nature, 340(6230), 229–230.
- Dewaele, J. M. (2018). Why the dichotomy ‘L1 versus LX user’ is better than ‘native versus non-native speaker. Applied Linguistics, 39(2), 236–240.
- Dörnyei, Z. (2009). The L2 motivational self system. Motivation, Language Identity and the L2 Self, 36(3), 9–11.
- Ellis, R., & Ellis, R. R. (1994). The study of second language acquisition. Oxford University.
- Endress, A. D., & Hauser, M. D. (2010). Word segmentation with universal prosodic cues. Cognitive Psychology, 61(2), 177–199.
- Erlam, R. (2006). Elicited imitation as a measure of L2 implicit knowledge: An empirical validation study. Applied Linguistics, 27(3), 464–491. https://doi.org/10.1093/applin/aml001
- Feinstein, A. R., & Cicchetti, D. V. (1990). High agreement but low kappa: I. The problems of two paradoxes. Journal of Clinical Epidemiology, 43(6), 543–549.
- Fleiss, J. L., Levin, B., & Paik, M. C. (2003). Statistical methods for rates and proportions. Wiley-Interscience.
- Goad, H., & White, L. (2004). Ultimate attainment of L2 inflection: Effects of L1 prosodic structure. EUROSLA Yearbook, 4(1), 119–145. https://doi.org/10.1075/eurosla.4.07goa
- Goyet, L., de Schonen, S., & Nazzi, T. (2010). Words and syllables in fluent speech segmentation by French-learning infants: An ERP study. Brain Research, 1332, 75–89.
- Hahne, A., & Friederici, A. D. (2001). Processing a second language: Late learners’ comprehension mechanisms as revealed by event-related brain potentials. Bilingualism: Language and Cognition, 4(2), 123–141. https://doi.org/10.1017/S1366728901000232
- Hartig, F., & Hartig, M. F. (2017). Package ‘DHARMa. R package.
- Hofer, B. (2017). Emergent multicompetence at the primary level: a dynamic conception of multicompetence. Language Awareness, 26(2), 96–112. https://doi.org/10.1080/09658416.2017.1351981
- Huddleston, R., & Pullum, G. K. (2002). The Cambridge grammar of English (Vol. 1, p. 23). Cambridge University Press.
- Jessner, U. (1999). Metalinguistic awareness in multilinguals: Cognitive aspects of third language learning. Language Awareness, 8(3–4), 201–209. https://doi.org/10.1080/09658419908667129
- Johnson, J. S., & Newport, E. L. (1991). Critical period effects on universal properties of language: The status of subjacency in the acquisition of a second language. Cognition, 39(3), 215–258. https://doi.org/10.1016/0010-0277(91)90054-8
- Kaltenböck, G., Heine, B., & Kuteva, T. (2011). On thetical grammar. Studies in Language. International Journal Sponsored by the Foundation “Foundations of Language”, 35(4), 852–897.
- Kartushina, N., & Frauenfelder, U. H. (2014). On the effects of L2 perception and of individual differences in L1 production on L2 pronunciation. Frontiers in Psychology, 5, 1246.
- Lecumberri, M. L. G., Cooke, M., & Cutler, A. (2010). Non-native speech perception in adverse conditions: A review. Speech Communication, 52(11–12), 864–886. https://doi.org/10.1016/j.specom.2010.08.014
- Lohmann, A., & Koops, C. (2016). Aspects of discourse marker sequencing. In G. Kaltenböck, E. Keizer, and A. Lohmann (Eds.), Outside the Clause: Form and function of extra-clausal constituents (pp. 417–446). John Benjamins.
- Mattys, S. L., Carroll, L. M., Li, C. K., & Chan, S. L. (2010). Effects of energetic and informational masking on speech segmentation by native and non-native speakers. Speech Communication, 52(11–12), 887–899. https://doi.org/10.1016/j.specom.2010.01.005
- Mattys, S. L., White, L., & Melhorn, J. F. (2005). Integration of multiple speech segmentation cues: a hierarchical framework. Journal of Experimental Psychology: General, 134(4), 477–500. https://doi.org/10.1037/0096-3445.134.4.477
- Mauranen, A. (2018). Linear unit grammar. In J.-O. Östman, and J. Verschueren (Eds.), Handbook of pragmatics: 21st annual installment (Vol. 21, p. 25).
- Mauranen, A., & Ranta, E. (2008). English as an Academic Lingua Franca–the ELFA project. Nordic Journal of English Studies, 7(3), 199–202. https://doi.org/10.35360/njes.108
- Mayo, L. H., Florentine, M., & Buus, S. (1997). Age of second-language acquisition and perception of speech in noise. Journal of Speech, Language, and Hearing Research : JSLHR, 40(3), 686–693.
- Mello, H., & Raso, T. (2019). Speech segmentation in different perspectives: diachrony, synchrony, different domains, different boundaries, corpora applications. Journal of Speech Sciences, 7(2), 01–08. https://doi.org/10.20396/joss.v7i2.14997
- Ortega, L., Iwashita, N., Norris, J. M., & Rabie, S. (2002, October). An investigation of elicited imitation tasks in crosslinguistic SLA research. In Second Language Research Forum, Toronto.
- Otake, T., Hatano, G., Cutler, A., & Mehler, J. (1993). Mora or syllable? Speech segmentation in Japanese. Journal of Memory and Language, 32(2), 258–278. https://doi.org/10.1006/jmla.1993.1014
- Piepho, H. P. (2019). A coefficient of determination (R2) for generalized linear mixed models. Biometrical Journal. Biometrische Zeitschrift, 61(4), 860–872. https://doi.org/10.1002/bimj.201800270
- Pinet, M., Iverson, P., & Huckvale, M. (2011). Second-language experience and speech-in-noise recognition: Effects of talker–listener accent similarity. The Journal of the Acoustical Society of America, 130(3), 1653–1662.
- Ringbom, H. (1993). Near-native proficiency in English. Åbo Akademi University.
- Sanders, L. D., & Neville, H. J. (2000). Lexical, syntactic, and stress-pattern cues for speech segmentation. Journal of Speech, Language, and Hearing Research : JSLHR, 43(6), 1301–1321.
- Sanders, L. D., Neville, H. J., & Woldorff, M. G. (2002). Speech segmentation by native and non-native speakers. The use of lexical, syntactic, and stress-pattern cues. Journal of Speech, Language, and Hearing Research, 45(3), 519–530. https://doi.org/10.1044/1092-4388(2002/041)
- Simpson, R. C., Briggs, S. L., Ovens, J., & Swales, J. M. (2002). The Michigan corpus of academic spoken English. The Regents of the University of Michigan.
- Sinclair, J. M., & Mauranen, A. (2006). Linear unit grammar: Integrating speech and writing (Vol. 25). John Benjamins Publishing.
- Spada, N., Shiu, J. L. J., & Tomita, Y. (2015). Validating an elicited imitation task as a measure of implicit knowledge: Comparisons with other validation studies. Language Learning, 65(3), 723–751. https://doi.org/10.1111/lang.12129
- Strobl, C., Boulesteix, A., Zeileis, A., & Hothorn, T. (2007). Bias in random forest variable importance measures: Illustrations, sources and a solution. BMC Bioinformatics, 8, 25. https://doi.org/10.1186/1471-2105-8-25.
- Suni, A. (2017). Wavelet Prosody Toolkit.
- Suni, A., Šimko, J., Aalto, D., & Vainio, M. (2017). Hierarchical representation and estimation of prosody using continuous wavelet transform. Computer Speech & Language, 45, 123–136. https://doi.org/10.1016/j.csl.2016.11.001
- Takata, Y., & Nábĕlek, A. K. (1990). English consonant recognition in noise and in reverberation by Japanese and American listeners. The Journal of the Acoustical Society of America, 88(2), 663–666.
- Tomaschek, F., Hendrix, P., & Baayen, R. H. (2018). Strategies for addressing collinearity in multivariate linguistic data. Journal of Phonetics, 71, 249–267. https://doi.org/10.1016/j.wocn.2018.09.004
- Towell, R., & Hawkins, R. D. (1994). Approaches to second language acquisition. Multilingual Matters.
- Tremblay, A., Broersma, M., Coughlin, C. E., & Choi, J. (2016). Effects of the native language on the learning of fundamental frequency in second-language speech segmentation. Frontiers in Psychology, 7, 985.
- Tremblay, A., Spinelli, E., Coughlin, C. E., & Namjoshi, J. (2018). Syntactic cues take precedence over distributional cues in native and non-native speech segmentation. Language and Speech, 61(4), 615–631.
- Ventsov, A., & Kasevich, V. (1994). Problemy vospriyatiya rechi. Izdatelstvo Sankt-Peterburgskogo universiteta, 64. [Problems in speech perception, Saint Petersburg University Press, 64].
- Vetchinnikova, S., Konina, A., Williams, N., Mikušová, N., & Mauranen, A. (2022). Perceptual chunking of spontaneous speech: Validating a new method with non-native listeners. Research Methods in Applied Linguistics, 1(2), 100012. https://doi.org/10.1016/j.rmal.2022.100012
- Vetchinnikova, S., Mauranen, A., & Mikusova, N. (2017). ChunkitApp: Investigating the relevant units of online speech processing. In INTERSPEECH 2017 18th Annual Conference of the International Speech Communication Association.
- Weber-Fox, C. M., & Neville, H. J. (1996). Maturational constraints on functional specializations for language processing: ERP and behavioral evidence in bilingual speakers. Journal of Cognitive Neuroscience, 8(3), 231–256.
- Wickham, H. (2016). ggplot2: Elegant graphics for data analysis. Springer-Verlag.