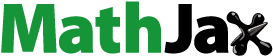
ABSTRACT
Spatial relation extraction is the process of identifying geographic entities from text and determining their corresponding spatial relations. Traditional spatial relation extraction mainly uses rule-based pattern matching, supervised learning-based or unsupervised learning-based methods. However, these methods suffer from poor time-sensitive, high labor cost and high dependence on large-scale data. With the development of pre-trained language models greatly alleviating the shortcomings of traditional methods, supervised learning methods incorporating pre-trained language models have become the mainstream relation extraction methods. Pipeline extraction and joint extraction, as the two most dominant ideas of relation extraction, both have obtained good performance on different datasets, and whether to share the contextual information of entities and relations is the main differences between the two ideas. In this paper, we compare the performance of two ideas oriented to spatial relation extraction based on Chinese corpus data in the field of geography and verify which method based on pre-trained language models is more suitable for Chinese spatial relation extraction. We fine-tuned the hyperparameters of the two models to optimize the extraction accuracy before the comparison experiments. The results of the comparison experiments show that pipeline extraction performs better than joint extraction of spatial relation extraction for Chinese text data with sentence granularity, because different tasks have different focus on contextual information, and it is difficult to take account into the needs of both tasks by sharing contextual information. In addition, we further compare the performance of the two models with the rule-based template approach in extracting topological, directional and distance relations, summarize the shortcomings of this experiment and provide an outlook for future work.
1. Introduction
Spatial relations are the basis of spatial reasoning, inspiring people to reason from known spatial constraints to obtain unknown spatial information and establishing a bridge between semantic space and physical space (Sharma Citation1996; Adibpour, Hochmann, and Papeo Citation2021). Natural language is the most common tool for daily human communication and an important source of spatial information. Spatial relation extraction is the process of identifying geographic entities from text and determining their corresponding spatial relations, and this process is usually divided into two parts: geographic entity recognition and spatial relation classification. Geographic entity recognition is to obtain the name, index and type information of geographic entities in the text, while spatial relation classification determines the spatial relation between any two geographic entities based on the geographic entity recognition results.
Spatial relation extraction is a special kind of relation extraction. The current common extraction methods are rule-based pattern matching, supervised learning methods and unsupervised learning methods. The rule-based pattern matching method obtains geographic entities and determines spatial relations by inducting syntactic patterns and constructing a dictionary of feature words (Du, Wang, and Li Citation2005; Zhang and Lv Citation2007; Zhang et al. Citation2009). The supervised learning method is based on manually annotated corpus data and combines machine learning methods such as random forests, support vector machines and recurrent neural networks to automatically capture the contextual features of text. The method obtains geographic entities and spatial relations based on the captured features (Zhang et al. Citation2011; Du et al. Citation2017). Compared with supervised learning methods, unsupervised learning methods do not require manually annotated data and can extract spatial relations by selecting text features directly from large-scale text data (Loglisci et al. Citation2012; Yu and Lu Citation2015). However, due to the ambiguity and diversity of spatial information expressed in natural language, as well as the drawbacks to the above methods, such as high maintenance cost, excessive manual intervention and over-reliance on large-scale data, the above traditional spatial extraction methods can hardly achieve the expected performance.
Compared with traditional word vector models, pre-trained language models (Devlin et al. Citation2018; Lan et al. Citation2019; Yang et al. Citation2019; Brown et al. Citation2020) capture the implicit linguistic rules and common sense by acquiring a prior knowledge of linguistic expressions from large-scale text data through unsupervised learning methods (Erhan et al. Citation2010; Bengio, Courville, and Vincent Citation2013; Qiu et al. Citation2020). Pre-trained language models have become the dominant choice for natural language processing tasks, as well as for relation extraction tasks. Pipeline and joint extraction are two different strategies of relation extraction implementation. The major difference between the two strategies is whether entity recognition and relation classification are performed in the same model. Pipeline extraction divides the entity recognition and relationship classification tasks into separate entity and relationship models, with the entity model first recognizing entity information in the text, and the relationship model later determining the relationship between any two entities (Zelenko, Aone, and Richardella Citation2003; Chan and Roth Citation2011). However, it is found that entity recognition errors can directly affect relation classification while ignoring the dependency between the two tasks can also have an impact on relation extraction (Miwa and Sasaki Citation2014; Zheng et al. Citation2017; Wang and Lu Citation2020). To better take account into the dependencies between entity recognition and relation classification, and to reduce the impact of the error propagation problem, related studies have proposed the use of a unified encoder to generate joint extraction of contextualized expressions for both tasks (Miwa and Bansal Citation2016; Wadden et al. Citation2019; Nayak and Ng Citation2020). Joint extraction enhances the connection between two tasks through a multi-task learning approach, which leads to better robustness of the model performance on both tasks. Although joint extraction can fully take account into the association information between the two tasks, entity recognition and relationship classification have different information focus on the context, and blindly sharing word vector representations can harm the model extraction performance instead (Zhong and Chen Citation2021). To discover a suitable spatial relation extraction method in geography, this paper selects two relation extraction models based on pre-trained language models and deep learning to conduct comparison experiments, comparing the suitability of pipeline extraction and joint extraction for spatial relationship extraction, and providing a baseline model for future research on spatial relation extraction methods.
The remaining sections of the paper are organized as follows. Section 2 describes in detail the selected pipeline and joint extraction models, meanwhile explained the data sources and processing methods, Section 3 introduces the model evaluation metrics, records the model fine-tuning experiments and comparison experimental results, Section 4 discusses the experimental results, analyses the strengths and limitation of the model based on different spatial relation extraction result, and finally summarizes this study and looks at future research ideas and directions in Section 5.
2. Methods
2.1. Model
PURE and CasREL are representative deep learning models that achieve state-of-the-art performance in pipeline extraction and joint extraction, respectively, and they have achieved excellent results on datasets such as ACE05, SciERC, and NYT. Although the two models belong to two relation extraction strategies, there are similarities in the model architecture and composition, both of which consist of two parts: entity model and relation model. The entity model is used to identify the name, index, and type information of geographic entities in the text; then the relation model uses geographic entities to predict the spatial relations between them. show the overview of the two model architectures.
The CasREL consists of Encoder, Subject Tagger, and Relation-Specific Object Taggers from bottom-up. In order to generate better contextualized representations of Chinese texts, the Chinese-bert-wwm-ext pre-trained language model (Cui et al. Citation2019) is chosen as the Encoder in this paper. Subject Tagger layer is the entity model of CasREL, which uses a Feed Forward Network to predict the start and end position of each geographical entity in the text. More precisely, the Subject Tagger layer consists of two binary classifiers that predict the start position and end position of each geographic entity, respectively. Relation-Specific Object Taggers layer is the relation model of CasREL, which consists of a set of Relation-Specific Object Taggers. Each Relation-Specific Object Tagger is identical to the Subject Tagger in composition, and fuses contextualized representation with subject features to predict the location of objects that have specific spatial relation with the subject.
The two independent encoders in PURE were chosen to be consistent with CasREL’s Chinese-bert-wwm-ext pre-trained language model. The entity model also includes a span enumeration layer and a feedforward neural network layer. Geographic entity recognition usually predicts the BIO label of each character to extract entities, however, predicting geographic entities based only on the labels of discrete characters will have the problem of boundary blur, and this method also does not conform to human reading comprehension habits. To address this problem, the phrase length based on geometric distribution sampling combines continuous tokens into span, and predicting geographic entities based on span can capture the boundaries of geographic entities more accurately and express the potential semantics of geographic entities more realistically (Joshi et al. Citation2020). Span enumeration layer is the combination of discrete character representation into span representation. The feedforward neural network combines with the span representation to predict whether each span is a geographic entity, and the specific geographic entity type. Relation model consists of text marker, encoder, and feedforward neural network layers. Text marker inserts the start and end position markers of each entity based on the result of geographic entity recognition, and the markers also contain geographic entity type information. Encoder generates span representation based on the result of text marker processing. The feedforward neural network layer generates the probability of various spatial relations between two geographic entities, and determines the spatial relationships between geographic entities based on the maximum probability.
2.2. Data
In this paper, we use “Chinese Encyclopedia (Geography)” as the data source, and 188 Chinese articles were manually collated and annotated as the dataset. The dataset contains 1481 Chinese sentences and 368 pairs of spatial relations. The dataset collation and annotation process has been carried out according to the following steps.
The classification of spatial relations specifies the target of spatial relation extraction, and spatial relations are usually classified into topological relations, directional relations, and distance relations (Zhang and Lv Citation2007). Based on the work of (Shen, Zhang, and Jiang Citation2009), this paper subdivides the above three spatial relations into 22 types, for example, topological relations can be further subdivided into Contains, Disjoint, Equals, Intersects, Overlaps, and Touch, etc.
Geographic entities are the basic information for spatial relation classification. In this paper, geographic entities in the text are divided into locations and spatial entity based on ISO:Space. The location refers to inherently grounded spatial entity, such as mountains, cities, rivers, and so on, as well as administrative entities like towns and counties. The spatial entity is used for referring an entity that is not inherently a location, but one which is identified as participating in a spatial relation, such as tall buildings, schools, or geographic phenomena such as typhoons, floods, and so on. As shown in , we list some samples of location and spatial entity (Pustejovsky, Moszkowicz, and Verhagen Citation2011).
A set of data pre-processing procedures were applied to this corpus. Firstly, remove the extra space and meaningless symbols from the sentences. Secondly, convert all punctuation marks in the text into Chinese punctuation marks. Finally, use sentence boundary detection rules based on Chinese punctuation to split Chinese sentences.
The annotation is based on the spatial relations and geographic entity classification formulated in (1) and (2), and then, the annotated sentences that do not have spatial relations and containing less than two geographic entities are eliminated. The annotated corpus was shuffled randomly, and 100 sentences were reserved as the evaluation dataset. The remaining corpus is further divided into training dataset, validation dataset, and test dataset according to bootstrapping method. Firstly, select 100 sentences randomly as test dataset. Secondly, sampling 1281 sentences with replacement as training dataset, and the id of the sentence is recorded in every sample. Finally, the sentences which were not added to the training dataset were selected as the validation dataset. shows the division of the annotated corpus.
Convert the data formats of the training dataset, validation dataset, and test dataset to the requirements of CasREL (Wei et al. Citation2020) and the PURE (Zhong and Chen Citation2021).
Figure 3. Dataset is divided, and the original data set is manually labeled and divided into fine-tune and eval datasets, where the fine-tune data set is divided into training sets, validation sets, and test sets according to the Bootstrapping method.
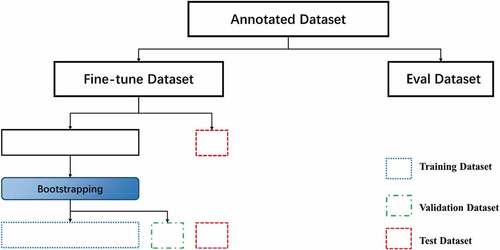
Table 1. Location contains a comparison table of geographic entity types and samples.
Table 2. Spatial entity contains a comparison table of geographic entities with samples.
Chinese sentences encountered in the actual annotating process are not generally as common as examples in a table, and semantic ambiguity often occurs. For example, “Dahong Mountain is located in the northern part of Hubei Province, between the Hanjiang River and the Yunshui River [大洪山位于湖北省境北部, 汉江和涢水之间]”, there is a vague spatial relation between the “Dahong Mountain [大洪山]”, “Hanjiang River [汉江]” and “Yunshui River [涢水]”, touch and overlap may be their spatial relation. This type of situation occurs frequently during manual annotating, and this article gradually adds constraints during annotating to clarify the fuzzy semantics of this class shows an example of annotated records..
Table 3. Example of annotated records and their corresponding English translations.
3. Experiments
The evaluation metrics consist of two parts, one is the evaluation of the geographic entity recognition result for pipeline model, the other is the evaluation of the spatial relation extraction for the two models. The quantitative evaluation metrics used in the experiments are precision, recall, and F1 score. A predicted geographic entity is considered as a correct recognition if the boundaries and type are correct, and a predicted spatial relation is considered as correct includes three aspects :(1) the boundaries and type of geographical entities involved in the spatial relation, (2) the type of spatial relation, and (3) The order of subject and object in the spatial relation triple (Bekoulis et al. Citation2018). Precision, recall, and F1 are calculated as follows:
3.1. Fine-tuning CasREL and PURE
To attain an optimal set of hyperparameters for spatial extraction model, we fine-tuned the CasREL and PURE based on them best predictive result on the validation dataset after 100 epochs of training. Meanwhile, we also focus on the training loss, fluctuations in the scores of the prediction results on the validation dataset and time cost in the training process. The hyperparameters fine-tuning process adjusts only one hyperparameter take value of the model per training. Each hyperparameter value is determined by a set of control variable experiments, judged by the F1 of that set of experiments on the validation dataset. Once the optimal value of the hyperparameter is obtained, the value of this hyperparameter is fixed in subsequent experiments until all hyperparameter values are determined and the fine-tuning is finished. Due to the limited computational resources, each hyperparameter value is set within a certain range.
As shown in , with the max sequence length increased from 50 to 200, the training loss decreased steadily and the F1 score on the validation dataset was close, and shows that CasREL gets the best F1 score when the max sequence length is set to 200. As shown in , the F1 score on the validation dataset was always below 0.1 when the learning rate is set to 0.000001. When the learning rate was 0.0002, the training loss converged the fastest; however, shows that learning rate was set to 0.00002 has a lower F1 score on the test dataset than learning rate was set to 0.00001. As shown in , when the batch size was set to 16, the F1 score on the validation dataset appeared unstable, and the F1 score on the validation dataset fluctuated significantly when the batch size was set to 4.
Figure 4. Training losses (a) and F1 scores (b) at different max sequence length of CaREL on validation dataset.
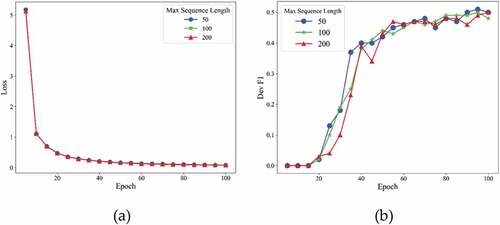
Figure 5. Training losses (a) and F1 scores (b) at different learning rate of CaREL on validation dataset.
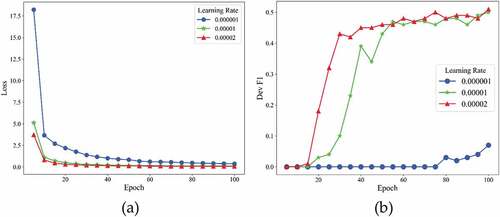
Figure 6. Training losses (a) and F1 scores (b) at different batch size of CaREL on validation dataset.
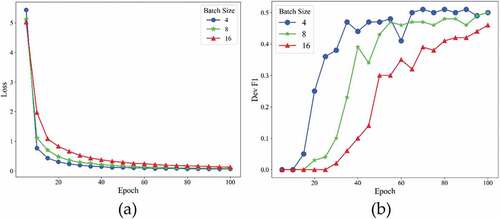
Table 4. Best predictive results of the CasREL and time cost under different hyperparameter settings.
As shown in , when the context window is set to 300, the entity model of PURE got the best F1 score on the validation dataset, while the training time cost increases slightly. When the learning rate was 0.0001 or 0.000001, the precision, recall, and F1 score on the validation dataset decreased significantly, and when the batch size is set to the 8, the model has highest F1 score on the validation dataset.
Table 5. Best predictive results of the PURE entity model and time cost under different hyperparameter settings.
As shown in , with the max sequence length increased from 50 to 200, the training loss of relation model decreased steadily, while the training time cost increased significantly. As shown in , the F1 score on the validation dataset decreased as the batch size increased, and shows that the relation model got best on the test dataset when batch size was set to 16. shows that although the training loss of the model fluctuated as the learning rate increased, the F1 score on the validation dataset gradually rose.
Figure 7. Training losses (a) and F1 scores (b)at different max sequence length of PURE on validation dataset.
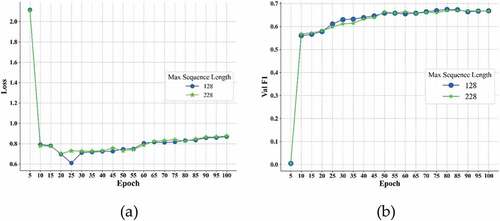
Figure 9. Training losses (a) and F1 scores (b) at different learning rate of PURE on validation dataset.
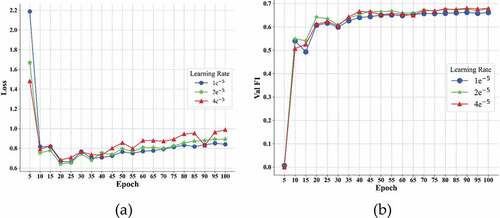
Table 6. Best predictive results of the PURE relation model and time cost under different hyperparameter settings.
3.2. Comparative evaluations of pipeline and joint extraction
To evaluate the spatial relation extraction performance of the pipeline and joint extraction method, PURE and CasREL are compared based on the evaluation dataset. Both PURE and CasREL used the optimal combination of hyperparameters obtained as showed in the previous section. As shown in , PURE performs better than CasREL on the evaluation dataset. Although PURE has better spatial relation extraction performance in evaluation metrics, the F1 score on the evaluation dataset does not reach a high level. To find the reasons why F1 score does not score high, this paper begins to analyze the spatial relation triples from the extraction results of the two models. In the process of analyzing the spatial relation extraction result, we found that some of the wrong extraction results were not due to the content, type of geographic entity, or spatial relation type, but to excessive standards and manual annotating errors. On the one hand, the evaluation criteria determine that the extraction results are the same as the annotated results before to be correct. The judgment includes the name and order of the subject and object, as well as the type of spatial relations. However, some spatial relations are not sensitive to the order of subject and object, it is feasible to swap the order of subject and object in the touch relation. This contradiction drives some correct extraction results to be judged as wrong. For example, “Da tun is located at the northeast of Pei County, Jiangsu Province, the west coast of the west side of the Weishan lake” in the artificial spatial relation is “Da tun”, “touches”, “Weishan lake”, the model extraction results are “Weishan lake”, “touches”, “Da tun”. On the other hand, there are human error in annotation process. The most common mistakes are wrong determination of spatial relation, wrong selection of geographic entity names, and wrong ordering of subject and object. As shown in , we manually judge the spatial relationship extraction results of the two models on the evaluation set and recalculate the scores of the metrics of the two models. The results showed that the metric scores of both models were significantly improved, and CasREL model extracts spatial relations more precisely, while the PURE model extracts spatial relation more comprehensively. Based on the significant difference between the two models, we believe that, compared to sharing, obtaining the contextual representations of two tasks separately captures text features more comprehensively.
Table 7. Comparative evaluation of jointly extraction and pipeline extraction methods.
Table 8. Comparison of extraction results of spatial relationship by manual interpretation.
4. Discussion
only shows the extraction performance of the two models for all spatial relations, is there any difference between the extraction performance of the two models for different spatial relations? To further investigate this question, we analyze the extraction results of the two models for different spatial relations and compare them with the rule-based pattern matching method. shows the different spatial relation extraction results of the two models after manual judgments. The results show that the topological relation extraction performance of the two models is superior compared with the rule-based pattern matching approach, the CasREL and PURE maintain good accuracy and recall rates, respectively. However, the extraction of directional and distance relations by both models showed underfitting compared with the rule-based pattern matching method. Based on this situation, we analyzed the percentages of various spatial relations in the fine-tune dataset and the evaluation dataset on the one hand and further analyzed why the two deep learning models performed poorly in extracting directional and distance spatial relations on the other hand. The statistical results showed that in the fine-tune dataset, topological relations accounted for 87.97% of all spatial relations, directional relations accounted for 11.22%, and distance relations accounted for 0.81%, while in the evaluation dataset, the three spatial relations accounted for 89.95%, 9.51%, and 0.54%. The composition of the three spatial relations in the dataset is extremely unbalanced, deep learning as a data-driven technology, the sample sparsity of the directional and distance relations has an impact on model learning. record typical samples of the wrong extraction results of the direction and distance relations of the PURE and CasREL models. The wrong extraction results consist of both wrong and missed extraction result compared to the labeled results. Among the wrong cases, the order of subject and object in the direction relations is often reversed, which indicates that the model does not really understand the reference target in the Chinese direction relations. In addition, the model does not correctly identify the names of geographic entities, which further leads to the wrong extraction of spatial relations. Distance relations often appear in the missed extraction results, and the model also has difficulty in obtaining complete spatial relations when multiple geographic entities exist in the text, both of which indicate that the model has not yet captured the descriptive features of spatial relations, especially distance relations.
Table 9. Comparative evaluation of spatial relations between joint and pipeline extraction by manual interpretation.
Table 10. The wrong extraction results of PURE.
Table 11. The wrong extraction results of CasREL.
5. Conclusions
In this study, we compare the spatial relation extraction performance between pipeline extraction and joint extraction based on annotated Chinese corpus from “Chinese Encyclopedia (Geography)”. We choose two deep learning models, CasREL and PURE, to represent the joint and pipeline extraction. Firstly, we train the models and obtain the optimal set of hyperparameters for spatial relation extraction. Then, based on the reserved dataset, we compared the spatial relation extraction performance of the two models under optimal hyperparameter settings. According to the experimental results, the F1 score of the pipeline extraction method is higher than that of the joint extraction method. The result shows that it is better to obtain independently rather than share contextual representation for geographic entity recognition and spatial relation classification, geographic entities, and spatial relations have different emphasis on contextual representations. We further analyzed the extraction results of both models for the test set, and we found that some of the results are misjudged due to the strict criterion and the incorrect manual annotating. After manual interpretation, we recalculated the F1 scores for CasREL and PURE to be 0.767 and 0.672, respectively, while CasREL achieves good precision and the PURE achieves a high recall. Further, we analyzed the extraction performance of the two models for different spatial relations, and the results showed CasREL and PURE have a significant improvement in the extraction performance of topological relations compared to the rule-based pattern matching methods, with F1 scores of 0.713 and 0.775.
However, the study has some limitations. For example, two models show an underfit performance on directional and distance relation extraction, because we found that the proportion of topological relation in the material was more than 85%. This extremely unbalanced sample distribution affected the spatial relation extraction performance of the two models. In addition, the materials in this paper comes from a single source, so the coverage of various forms of spatial relation description is limited, and we split the materials into sentences, the experimental results cannot explain the spatial relation extraction performance of the two models in the document. Therefore, in the future work, we plan to combine multi-source text data as corpus and compare the spatial relation extraction performance under different granularity. Moreover, we plan to improve the performance of spatial relation extraction by considering more text features, such as spatial relation feature words.
Acknowledgments
The authors thank Teng Zhong, Yanxu Lu and Shu Wang for their constructive comments.
Disclosure statement
No potential conflict of interest was reported by the author(s).
Data availability statement
The data that support the findings of this study are available from the corresponding author upon reasonable request.
Additional information
Funding
Notes on contributors
Kehan Wu
Kehan Wu received the bachelor’s degree in geographic information science from the Zhejiang A&F University, China, in 2019. He is currently a masters student at Nanjing Normal University. His current research interest is geographic big data mining and GeoAI.
Xueying Zhang
XueYing Zhang is currently a professor and doctoral supervisor of the School of Geographical Sciences of Nanjing Normal University. Her research fields include geographic big data, geographic knowledge map, smart city and humanistic social GIS. She presided over and participated in the 863 Program, the National Key Research and Development Program, and the National Natural Science Foundation of China.
Yulong Dang
Yulong Dang received the bachelor’s degree in geographic information science from the Chang’an University, China, in 2021. He is currently a masters student at Nanjing Normal University. His current research interest is geographic big data mining and knowledge graph.
Peng Ye
Peng Ye received the PhD degree in Cartography and Geographical Information System from Nanjing Normal University, in 2021. He is currently a research assistant professor with the Urban Planning and Development Institute, Yangzhou University. As a member of Smart City Group, his current research interest is geographic big data mining.
References
- Adibpour, P., J. -R. Hochmann, and L. Papeo. 2021. “Spatial Relations Trigger Visual Binding of People.” Journal of Cognitive Neuroscience 33 (7): 1343–1353. doi:10.1162/jocn_a_01724.
- Bekoulis, G., J. Deleu, T. Demeester, and C. Develder. 2018. “Adversarial Training for Multi-Context Joint Entity and Relation Extraction.” arXiv preprint arXiv:1808.06876.
- Bengio, Y., A. Courville, and P. Vincent. 2013. “Representation Learning: A Review and New Perspectives.” IEEE Transactions on Pattern Analysis and Machine Intelligence 35 (8): 1798–1828. doi:10.1109/TPAMI.2013.50.
- Brown, T. B., B. Mann, N. Ryder, M. Subbiah, J. Kaplan, P. Dhariwal, A. Neelakantan, P. Shyam, G. Sastry, and A. Askell. 2020. “Language Models are Few-Shot Learners.” arXiv preprint arXiv:2005.14165.
- Chan, Y. S., and D. Roth. 2011. “Exploiting Syntactico-Semantic Structures for Relation Extraction.” Paper presented at the Proceedings of the 49th Annual Meeting of the Association for Computational Linguistics: Human Language Technologies, Portland, Oregon, USA, June 19–24.
- Cui, Y., W. Che, T. Liu, B. Qin, Z. Yang, S. Wang, and G. Hu. 2019. “Pre-Training with Whole Word Masking for Chinese Bert.” arXiv preprint arXiv:1906.08101.
- Devlin, J., M. -W. Chang, K. Lee, and K. Toutanova. 2018. “BERT: Pre-Training of Deep Bidirectional Transformers for Language Understanding.” arXiv preprint arXiv:1810.04805.
- Du, S., Q. Wang, and Z. Li. 2005. “Definitions of Natural-Language Spatial Relations in GIS.” Geomatics and Information Science of Wuhan University, (30)6: 533–538. doi: 10.3321/j.issn:1671-8860.2005.06.016.
- Du, S., X. Wang, C. -C. Feng, and X. Zhang. 2017. “Classifying Natural-Language Spatial Relation Terms with Random Forest Algorithm.” International Journal of Geographical Information Science 31 (3): 542–568. doi:10.1080/13658816.2016.1212356.
- Erhan, D., A. Courville, Y. Bengio, and P. Vincent. 2010. “Why Does Unsupervised Pre-Training Help Deep Learning?” Paper presented at the Proceedings of the thirteenth international conference on artificial intelligence and statistics, Chia Laguna Resort, Sardinia, Italy, May, 13–15.
- Joshi, M., D. Chen, Y. Liu, D. S. Weld, L. Zettlemoyer, and O. Levy. 2020. “Spanbert: Improving Pre-Training by Representing and Predicting Spans.” Transactions of the Association for Computational Linguistics 8: 64–77. doi:10.1162/tacl_a_00300.
- Lan, Z., M. Chen, S. Goodman, K. Gimpel, P. Sharma, and R. Soricut. 2019. “Albert: A Lite Bert for Self-Supervised Learning of Language Representations.” arXiv preprint arXiv:1909.11942.
- Loglisci, C., D. Ienco, M. Roche, M. Teisseire, and D. Malerba. 2012. “An Unsupervised Framework for Topological Relations Extraction from Geographic Documents.” Paper presented at the International Conference on Database and Expert Systems Applications. Vienna, Austria, September 3–6. doi: 10.1007/978-3-642-32597-7_5.
- Miwa, M., and Y. Sasaki. 2014. “Modeling Joint Entity and Relation Extraction with Table Representation.” Paper presented at the Proceedings of the 2014 Conference on Empirical Methods in Natural Language Processing (EMNLP), Doha, Qatar, October 25–29.
- Miwa, M., and M. Bansal. 2016. “End-To-End Relation Extraction Using Lstms on Sequences and Tree Structures.” arXiv preprint arXiv:1601.00770.
- Nayak, T., and H. T. Ng. 2020. “Effective Modeling of Encoder-Decoder Architecture for Joint Entity and Relation Extraction.” Paper presented at the Proceedings of the AAAI Conference on Artificial Intelligence, New York, USA, February 7–12. doi: 10.1609/aaai.v34i05.6374.
- Pustejovsky, J., J. L. Moszkowicz, and M. Verhagen. 2011. “Using ISO-Space for Annotating Spatial Information.” Paper presented at the Proceedings of the International Conference on Spatial Information Theory. Belfast, Maine, USA, September 12-16.
- Qiu, X., T. Sun, Y. Xu, Y. Shao, N. Dai, and X. Huang. 2020. “Pre-Trained Models for Natural Language Processing: A Survey.” Science China Technological Sciences 63(10): 1872-1897. doi: 10.1007/s11431-020-1647-3.
- Sharma, J. 1996. Integrated Spatial Reasoning in Geographic Information Systems: Combining Topology and Direction. PhD diss. The University of Maine.
- Shen, Q., X. Zhang, and W. Jiang. 2009. “Annotation of Spatial Relations in Natural Language.” Paper presented at the 2009 International Conference on Environmental Science and Information Application Technology, Wuhan, China, July, 4–5. doi: 10.1109/ESIAT.2009.429.
- Wadden, D., U. Wennberg, Y. Luan, and H. Hajishirzi. 2019. “Entity, Relation, and Event Extraction with Contextualized Span Representations.” arXiv preprint arXiv:1909.03546.
- Wang, J., and W. Lu. 2020. “Two are Better Than One: Joint Entity and Relation Extraction with Table-Sequence Encoders.” arXiv preprint arXiv:2010.03851.
- Wei, Z., J. Su, Y. Wang, Y. Tian, and Y. Chang. 2020. “A Novel Cascade Binary Tagging Framework for Relational Triple Extraction.”
- Yang, Z., Z. Dai, Y. Yang, J. Carbonell, R. R. Salakhutdinov, and Q. V. Le. 2019. “Xlnet: Generalized Autoregressive Pretraining for Language Understanding.” Advances in Neural Information Processing Systems 32: Annual Conference on Neural Information Processing Systems,Vancouver, British Columbia, Canada. December, 9-14.
- Yu, L., and F. Lu. 2015. “A Bootstrapping Algorithm for Geo-Entity Relation Extraction from Online Encyclopedia.” Paper presented at the 2015 23rd International Conference on Geoinformatics, Wuhan, China, June 19-21. doi: 10.1109/GEOINFORMATICS.2015.7378569.
- Zelenko, D., C. Aone, and A. Richardella. 2003. “Kernel Methods for Relation Extraction.” Journal of Machine Learning Research 3 (Feb): 1083–1106. doi: 10.3115/1118693.1118703.
- Zhang, X., and G. Lv. 2007. ”Natural-Language Spatial Relations and Their Applications in GIS.” Journal of Geo-Information Science, 9(6): 77–81. doi: 10.3969/j.issn.1560-8999.2007.06.014.
- Zhang, C., X. Zhang, W. Jiang, Q. Shen, and S. Zhang. 2009. “Rule-Based Extraction of Spatial Relations in Natural Language Text.” Paper presented at the 2009 International Conference on Computational Intelligence and Software Engineering, Wuhan, China, December, 11–13. doi: 10.1109/CISE.2009.5363900.
- Zhang, X., C. Zhang, C. Du, and S. Zhu. 2011. “SVM Based Extraction of Spatial Relations in Text.” Paper presented at the Proceedings 2011 IEEE International Conference on Spatial Data Mining and Geographical Knowledge Services, Fuzhou, China, June 29–July 1. doi: 10.1109/ICSDM.2011.5969102.
- Zheng, S., F. Wang, H. Bao, Y. Hao, P. Zhou, and B. Xu. 2017. “Joint Extraction of Entities and Relations Based on a Novel Tagging Scheme.” arXiv preprint arXiv:1706.05075.
- Zhong, Z., and D. Chen. 2021. “A Frustratingly Easy Approach for Entity and Relation Extraction. Paper presented at the 2021 Conference of the North American Chapter of the Association for Computational Linguistics: Human Language Technologies, pages 50–61, Online. Association for Computational Linguistics.”