Abstract
The population dynamics from 1991 to 2006 for the seven-county Twin Cities Metropolitan Area (TCMA), Minnesota, USA, was analysed in this study. Per cent impervious surface areas (%ISA) for 1991, 1999 and 2006 were derived from Landsat Thematic Mapper (TM) images and were modified using two different masking methods. The modified %ISA images of 1991 and 1999 were correlated with 1990 and 2000 census block group data of the ‘two highly developed counties’, ‘five suburban counties’ and ‘all seven counties.’ Populations of both years were then modelled, assessed and compared. Next, the statistical models based on the 1999 %ISA and 2000 census data were applied to the 2006 residential %ISA image to estimate the 2006 population. These 2006 estimates were compared with census county-level population projections for 2006. In comparison to Method A, which uses ‘adjusted %ISA images’ by masking out highway centrelines and areas that have greater than 75% imperviousness, Method B based on ‘pure residential %ISA image’ has higher coefficient of determination (R 2) and much lower, consistent mean absolute relative errors (MARE). For both methods, the strongest R 2 and lowest MARE values between modelled population density and true density were found in the five-county model, followed by the seven-county model. The two-county model ranks last in terms of model performance for both years. In general, populations for the two highly developed counties were underestimated whereas the opposite was true for the five suburban counties. Population was most accurately estimated based on data from counties with the same or similar characteristics. By comparing the 1990/1991 and 1999/2000 models, we also found that the rate of population density per unit of impervious surface declined from 1991 to 1999. High accuracy was achieved when applying the 1999/2000 model to predict the 2006 population, suggesting that the relationship between per cent imperviousness and population density were relatively stable between 1999 and 2006.
1. Introduction
Population growth is a dynamic phenomenon with an all-encompassing impact on human and natural environments. Population in the USA has increased by more than 100-million from 1967 to 2006 (US Bureau of the Census Citation2006). The significant increases in population have raised many questions on how to address development while minimizing its environmental impacts at the same time. Adequate knowledge of population dynamics will help planners and policy makers create policy that is beneficial to current and future generations.
Traditional population estimation techniques used by the US Census Bureau are labour-intensive and time-consuming. Although the actual census is taken every 10 years, the US Census Bureau produces estimates of total residential population on an annual basis. State level agencies make the most use of component methods, in which the components of population change since the last census (births, deaths and net migration) are collected, to estimate county residential population (US Bureau of the Census Citation1990, Citation2005). The state population estimates are then produced by summing up county populations (US Bureau of the Census Citation2005). The housing unit method is the most widely used approach for finer level (census tract and census block group) population estimation. Regarding the housing unit method, a current inventory of housing units is usually derived by counting the dwelling structures in the field or using residential building and demolition permits information and housing stock figures (US Bureau of the Census Citation1990). These approaches are based on the assumption that changes in the population can be accurately approximated by combining administrative records of data on the number of occupied housing units. The process of searching through administrative records data and combining them with the old database can be as expensive and time consuming as conducting the actual census (Lu et al. Citation2006). Furthermore, areas designed for census output in the USA are essentially administrative units, which represent an artificial partitioning of socio-economic space into objects of census administration (Martin Citation1998). It is also difficult to identify non-residential units at a fine scale in a direct and precise manner (Harris and Longley Citation2000).
Remote sensing provides a more time-efficient way to estimate residential population, particularly for larger areas. The potential of remote sensing data for population estimation has been investigated since the 1950s when air photos were utilized to count dwelling units (Lo 1986, Wu and Murray Citation2007), whereas satellite remote sensing was first used to study urban populations by Tobler (Citation1969), who measured the radii for a given city using satellite imagery, and found a strong statistical correlation between the radii and populations of various cities (Wu et al. Citation2005). Two other types of population estimation methods using remote sensing – estimates derived from land use classification (Langford et al. Citation1991, Yuan et al. Citation1997, Dobson et al. Citation2000, Lo2003, Mennis Citation2003) and automatic image analysis based on image pixel characteristics or spectral features (Iisaka and Hegedus Citation1982, Lo Citation1995, Citation2001, Harvey Citation2002a, Citation2003, Li and Weng Citation2005) – were also summarized and reviewed by Lo (Citation1986), Harvey (Citation2002b), Li and Weng (Citation2005) and Lu et al. (Citation2006). Many of these methods were coupled with statistical modelling to infer the relationship between population and other variables for the purpose of estimating the total population in an area (Wu et al. Citation2005).
In recent studies, a new population indicator – impervious surface fraction – derived from remote sensing imagery was proposed and tested by (Lu et al. Citation2006, Wu and Murray Citation2007). Impervious surfaces refer to any surfaces that are impenetrable by water, including: tarred and paved roads, paved parking lots, buildings, bridges, etc. All of these features are created by humans and serve as quintessential elements of the urban environment. Lu et al. (Citation2006) estimated residential population in Marion County, Indiana, based on impervious surface coverage derived from a Landsat Enhanced Thematic Mapper (ETM +) image with an overall population estimation error of −0.97%. A strong squared correlation (0.82) and a mean relative error (38%) of estimation were achieved based on the residential impervious surface-based approach. Similarly, Wu and Murray (Citation2007) assessed two groups (zonal and pixel-based) of approaches for estimating the urban population of a portion of Columbus, Ohio. They also compared the performance of three indicators – impervious surface fraction, spectral radiance and land use. Their results showed that the overall performance of the impervious surface fraction model achieved less than 7% relative error for the whole validation area and 31%–33% mean average percentage error at the census block group level.
These previous studies demonstrated that remote sensing provides valuable resources and effective tools for population estimation. Nevertheless, main parts of the past studies were focussed on one time static population estimation. Few studies have been done on estimating population dynamically using multi-temporal remote sensing data. The purpose of this article is to examine residential population dynamics from 1991 to 2006 for the Twin Cities Metropolitan Area (TCMA) of Minnesota using multi-temporal satellite remote sensing. In this research, impervious surface fraction maps for 1991, 1999 and 2006 were derived from Landsat Thematic Mapper (TM) images. Statistical models were then created based on 1990 and 2000 US Census block group data and per cent imperviousness classifications of 1991 and 1999 images. Six model results, based on three groups of data (two highly urbanized counties, five suburban counties and all seven counties) and two impervious surface extraction methods (Method A and Method B), from each year were compared to determine which is better for estimating population density. In particular, Method A masks out major highway centrelines and all areas above 75% imperviousness in the TCMA impervious surface image, whereas Method B extracts all residential land uses out of the impervious surface image. Changes in the relationship between per cent imperviousness and population density from 1991 to 1999 were then analysed. Finally, 2006 population was estimated based on the residential population estimation model created using 1999 %ISA and 2000 census block group data. On the basis of the results, benefits and limitations of the methods and urban planning implications were also discussed.
2 Method
2.1 TCMA study site
The 7700 km2 Twin Cities (Minneapolis and Saint Paul) Metropolitan Area ( ) consists of seven counties in East Central Minnesota: an inner metro comprised of two highly developed counties (Hennepin and Ramsey) and an outer metro with five surrounding counties (Carver, Scott, Dakota, Washington and Anoka). The total population of the seven counties comprising the TCMA increased from 2.29 million to 2.64 million from 1990 to 2000. According to Metropolitan Council (Citation2004), TCMA was home to 2.82 million residents as of April 2006. The metropolitan council predicts that the Metro area will add an additional 0.77 million people by 2030.
Significant population growth and urban development over the past decades has placed increased pressure on developing rural environments along the urban fringe for this region. The metropolitan urban service area is expected to expand beyond its current boundaries to accommodate urban expansion in developing communities. The amount of land needed for development in ‘developing’ communities and the amount of redevelopment that occurs in ‘developed’ communities within the TCMA need to be assessed. To address the implications of population growth and urban expansion in the TCMA, it is imperative to study their relationship and examine their dynamics. Understanding population dynamics in this region is valuable for policy makers and land use planners to designate policies and zoning ordinances that can balance the needs of economic development and environmental sustainability.
2.2 Data and pre-processing
Three Landsat 5 TM images (path 27; row 29) acquired on 4 September 1991, 24 July 1999 and 16 July 2006 were used to extract per cent impervious surface areas (%ISA) for this study. The 1991 and 1999 images were chosen because of their high quality and their temporal proximity with the 1990 and 2000 decennial censuses respectively. The 2006 image was selected because it was the most current data available when the study was started. The three TM images have been rectified using a 2000 Minnesota DOT (Department of Transportation) base map, with the root mean square errors (RMSE) less than 0.25 pixels for all the images. After rectification, the original digital numbers of the six reflective bands of TM data were converted to exo-atmospheric reflectance based on the method provided by Chander and Markham (Citation2003).
Census block group data for 1990 and 2000 for the TCMA were obtained from the US Bureau of the Census. These data were checked carefully for sliver polygon errors before they were used in conjunction with impervious surface maps derived from the Landsat images to create the statistical models for population estimation. In total, 36 sliver polygons were removed from the 1990s dataset and another two were identified for 2000. In particular, the Census block group data were selected because they represent the most disaggregated unit in the census hierarchy of spatial data whose size is population sensitive. Block groups contain a population between 600 and 3000 with an optimal population of 1500 (US Bureau of the Census Citation2001). Boundaries of block groups never cross over state lines, county lines, census tracts or other ‘statistically significant entities’ with the exception of some block group delineations provided by authorities of American Indian Tribes.
Major highways data were created by the Minnesota Department of Transportation and distributed by the Minnesota Department of Natural Resources Data Deli ( http://deli.dnr.state.mn.us). These data were used to mask out pixels that intersect major highway centrelines from the impervious surface images in Method A to create a more accurate model for residential population estimation. In addition, generalized land use data for 1990, 2000 and 2005 based on high-resolution aerial photograph interpretation were provided by the Metropolitan Council GIS group and were used to extract residential areas from impervious surface images in Method B. Residential land use categories have changed significantly from 1990 to 2005. Categories from 1990 were based on original categories defined in 1962 by the Metropolitan Council which only include single family, multi-family residential and farmstead residential categories. Land use categories were refurbished in 2000. Therefore, more explicit residential categories – single family detached, single family attached, multifamily, mixed use residential and farmstead – are included in the 2000 and 2005 generalized land use data sets (Metropolitan Council Citation2006).
2.3 Per cent impervious surface estimation
Impervious surface can be extracted accurately by various analysis techniques using remote sensing (Weng Citation2007). Moderate resolution remote sensing images with a synoptic view and relatively low cost, such as Landsat TM and ETM+ have been utilized as major data sources for impervious surface extraction of relatively large areas (Phinn et al. Citation2002, Wu and Murray Citation2003, Yang et al. Citation2003, Dougherty et al. Citation2004, Lu and Weng Citation2004, Wu Citation2004, Yang et al. Citation2005, Lee and Lathrop Citation2006, Wu and Yuan Citation2007, Yuan et al. Citation2008). In this study, the normalized spectral mixture analysis (NSMA) method originally proposed by Wu (Citation2004) was used to extract the per cent impervious surface areas (%ISA) from the TM images. Spectral mixture analysis (SMA) is an image processing tool that assumes a linear or nonlinear combination between spectra recorded by a sensor and fractions of the components or end-members which make up a given pixel (Roberts et al. Citation1998, Wu and Murray Citation2003, Lu et al. Citation2006). Using SMA, each pixel can be broken down into several parts and then reassigned a value based on pre-selected end-members. The NSMA modifies the typical SMA method by adding a normalization process before ‘unmixing’ image pixels, to reduce within-class radiometric variations (
EquationEquation 1).
In this study, pixels of the normalized TM images whose water surface areas have already been masked out were modelled as a three end-member, linear combination of vegetation, impervious surface and soil (V-I-S) (
EquationEquation 2). The three-component (V-I-S) urban model was first proposed by Ridd (Citation1995) to parameterize the biophysical composition of urban environments. Since then, the V-I-S model has been applied in various studies to quantify the extent or density of urban development.
Detailed information about the three end-member NSMA method and its comparison to four end-member linear SMA (vegetation, soil, high albedo and low albedo) in urban studies can be found in Wu (Citation2004). In addition, per cent impervious surface images with continuous values (0–100%) estimated by NSMA for 1991 and 1999 were post-processed to facilitate dynamic analysis and comparison. The post-processing analysis is based on the assumption that pervious areas with 0% imperviousness in 2006 would also have 0% imperviousness in 1991 and 1999. The justification for this assumption is that developed urban areas with %ISA equal and greater than 1% in 1991 and 1999 are not likely to be converted back to undeveloped rural areas in 2006. Moreover, to assess the accuracy of per cent impervious surface images, the estimated per cent imperviousness values were compared to test samples derived from 1-m black and white digital orthophotographs acquired in 1990 and 2000, and 1-m colour National Agriculture Imagery Program digital orthoimagery of 2003. A hundred samples representing various imperviousness levels were digitized from aforementioned high-resolution digital aerial imagery for each year. To incorporate the desired mix of impervious surface and vegetation cover types, a mean sample size of 30 pixels per AOI were chosen.
Next, two modified copies of %ISA images were made for each year. One copy was created by masking out major highway centrelines and pixels with imperviousness values greater than 75%. The reason to mask out areas above 75% imperviousness is because, by analysing the original %ISA image, we found that the majority of non-residential impervious areas such as commercial and industrial have an average per cent imperviousness greater than 75%. This also concurs with the findings of previous studies by Lu et al. (Citation2006) and Wu and Murray (Citation2003). This group of modified %ISA images for each year was used in Method A to create the statistical model for population estimation. Conversely, another modified copy of %ISA image for each year, the residential impervious surface map, was extracted by overlaying the original %ISA image with the land use map of the corresponding year. This residential impervious surface map was used in Method B for population estimation. The overall accuracy of both Method A and B were tested and their accuracy in relation to one another.
2.4 1991 and 1999 population modelling
Three statistical models – two-county (highly urbanized) model, five-county (suburban) model and seven-county (whole TCMA) model were developed for each of the two methods (Methods A and B). Therefore, a total of 12 models were created for both methods and both years (1991 and 1999). Block groups from 1990 were used to create statistical models with the two modified %ISA images of 1991, whereas 2000 block groups were used with the 1999 modified %ISA images.
For the two-county model, 50% of the block groups in Hennepin and Ramsey Counties were randomly selected for each year. Per cent impervious surface and population density of each block group were then calculated and correlated using the following power equation function:
Scatter diagrams between per cent imperviousness and population density show that, in general, the values of population density increase as the per cent imperviousness increases. The nonlinear power equation function was selected because it provided the best coefficient of determination (R 2) compared with other forms of regression, suggesting an inconsistent rate of change in population density over the domain of per cent imperviousness.
Similarly, the five-county models were created using 50% of the block groups that are randomly selected from the five counties (Anoka, Carver, Dakota, Scott and Washington Counties) that comprises the outer metro, whereas the seven-county model was based on block group data from all seven counties, which includes block groups from highly urbanized areas in the ‘urban core’ cities of Minneapolis and Saint Paul as well as block groups from surrounding suburban and rural areas. The two-, five- and seven-county samples were selected separately.
2.5 Accuracy assessment of the population models
The accuracy of each of the six statistical models created for 1991 and 1999 was assessed using the other half of block groups that were not used for model training and creation. Three statistical variables were calculated and compared, including coefficient of determination (R
2), RMSE and mean absolute relative error (MARE). Both R
2 and RMSE have been extensively used for statistical model assessment in various applications. R
2 is the square of the correlation coefficient and measures the proportion of variability in a data set that is accounted for by a statistical model, whereas RMSE represents the average distance of a data point from the fitted line that measured along a vertical line. For our study, the RMSE was calculated using
EquationEquation (4):
To assess the model performance more thoroughly, an additional variable – MARE – was calculated. Harvey (Citation2002b) proposed to use MARE in preference to RMSE since the robustness of the latter is often affected by extreme outlying values. Lu et al. (Citation2006) also used relative error to evaluate the performance of their population estimation models. Relative error (REk) is the error in the estimate divided by the true measurement, and usually expressed as a percentage (
EquationEquation 5). The MARE can then be calculated using
EquationEquation (6)
.
2.6 Comparison of 1991 and 1999 models and estimation of 2006 population
Each of the population estimation models from 1991 was compared with each 1999 model that has the same model setups. For example, the two-county model based on Method A of 1991 was compared to the two-county model based on Method A of 1999. The power regression equations for the models were graphed together to compare changes in the relationship between per cent impervious surface and population from 1991 to 1999. Furthermore, each of the two-, five- and seven-county models were compared between 1991 and 1999 to see if there were differing trends in the relationship between impervious surface and population for urban and suburban areas.
In addition, although we realized both population growth and urban impervious surface increase are dynamic processes and that the regression equation between population density and per cent imperviousness of 2006 will not be exactly the same as that of 1999/2000 case, the statistical model based on the 1999 image and the 2000 block group data were still used to estimate the 2006 population since the actual census is only taken every 10 years and the 2000 block group data is the most current decennial census data set available. The 2006 model performance was assessed by calculating the sum of the population for each of the seven counties and comparing them to US Census population county-level estimates for 2006.
3 Results
3.1 1991 and 1999 impervious surface images and accuracy assessment results
shows the accuracy assessment results for the per cent impervious classification maps for 1991, 1999 and 2006. Strong correlations between the TM-estimated per cent imperviousness and the measurements from the aerial photography were evident for all three years, with R 2 ranges from 0.86 to 0.91 and standard error ranges from 8.78 to 11.14.
Examples of the further ‘modified impervious surface images’ with continuous percentage of imperviousness are displayed in . In particular, , shows ‘adjusted impervious surfaces’ which include up to 75% imperviousness and with major highway centrelines masked (Method A) for two years (1991 and 1999), whereas , depicts ‘residential impervious surfaces’ (Method B) for the 2 years. In , , several roads and non-residential land use areas are visible. Parts of major highways are also visible if they are greater than 30 m in width. By contrast, roads and non-residential land uses were completely masked out of , .
Figure 3. Per cent impervious surface maps of 1991 (a, b) and 1999 (c, d) for both methods (Method A – adjusted impervious surface map with highway centerline and areas greater than 75% imperviousness masked out; Method B – pure residential impervious surface map with all non-residential areas masked out).
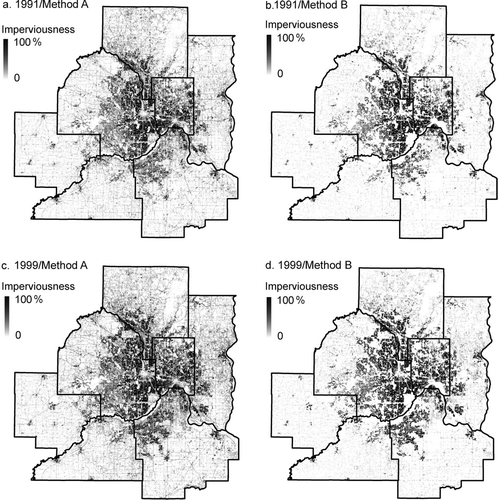
3.2 1991 and 1999 population estimation modelling results
Twelve population estimation models, six for 1991 and six for 1999, were created using a randomly selected 50% training sample of census block groups in each case. The independent (X) variable for each of the population models is the mean per cent impervious surface, while the dependant (Y) variable is population density. Models based on Method A for 1991 and 1999 are shown in . As such, models of Method B are shown in .
The five-county models for Method A have the highest R 2 values of 0.85 for 1991 and 0.92 for 1999 whereas the two-county models have the lowest squared correlation values at 0.68 for 1991 and 0.64 for 1999. Combining block groups from both the five- and two-county models, the seven-county models have intermediate R 2 of 0.71 for 1991 and 0.77 for 1999. Similarly, for Method B models, R 2 values are highest for the five-county models while R 2 values are lowest for the two-county models for both years, and R 2 values for the seven-county models rank in between the values of the two-county and five-county models. In general, Method B proves to have higher R 2 values in comparison to the Method A counterparts.
3.3 Accuracy assessment results of population models
The regression relationships between the census-based population density and model-estimated population density for the 50% validation samples of census block groups are displayed in and , respectively for Method A and Method B. From the figures we can see, the R 2 values are higher for Method B than Method A. For both Methods, the strongest R 2 of the performance assessment models comes with the five-county models, followed by the seven-county models. The two-county model ranks last with the weakest coefficient of determination. On the basis of results obtained, we can see population estimation models created with higher R 2 were more likely to produce higher R 2 in the accuracy assessment models.
Figure 6. Overall performance of two-, five- and seven-county population density estimation models of Method A.
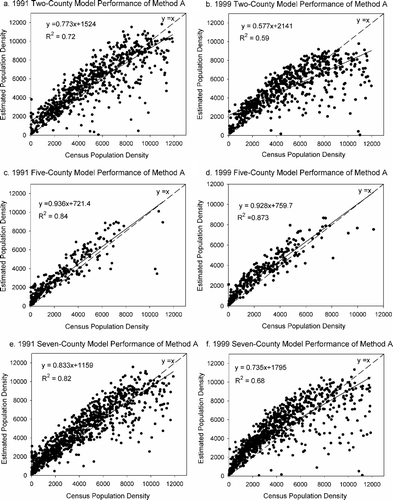
Figure 7. Overall performance of two-, five- and seven-county population density estimation models of Method B.
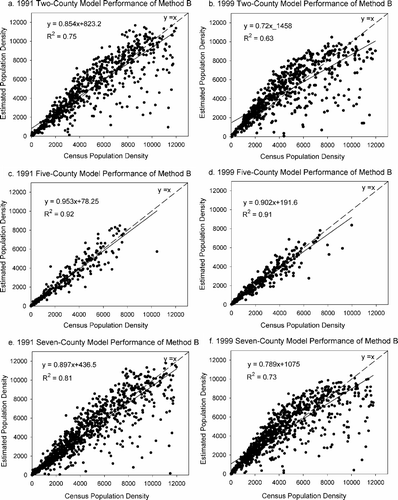
The R 2, RMSE and MARE values for all the accuracy assessment models were summarized in . Using Method A, the five-county models have the lowest RMSE values, but the highest RMSE values are less consistent, occurring in the two-county model in 1991 and the seven-county model in 1999. For Method B, the lowest RMSE values also come from the five-county models, whereas the two-county model has the highest RMSE values for both years. In addition, RMSE values for the five-county model improved from 1991 to 1999 for both methods, but the opposite is true for the seven-county model. For the two-county model, RMSE decreased from 1991 to 1999 using Method A whereas it increased during the same time period using Method B.
Table 1. Performance statistics of population density estimation models.
The MARE values for block groups in all models created using Method A are much higher than all average values in models created using Method B. When using Method B, the five-county model produced the lowest MARE values (25% for 1991 and 22.6% for 1999) whereas the seven-county model produced the highest MARE values (28.6% and 33.1% for 1991 and 1999, respectively). In addition, the MARE values using Method B are much more consistent than values derived from method A.
3.4 Comparison of 1991 and 1999 population dynamics and result of 2006 population estimation
Changes in the relationship between per cent imperviousness and population density from 1991 to 1999 for all two-, five- and seven-county models based on the more accurate method, Method B, were analysed and graphed in . All graphs in demonstrate the same trend – the population density declined from 1991 to 1999 for each per cent of impervious surface. In other words, the results of all three comparisons indicate that the rate of the increase of population density for each 1% increase in per cent impervious surface declined from 1991 to 1999.
The two-, five- and seven-county models of 1999 based on Method B were chosen to estimate 2006 population because they consistently perform better than the Method A models. Population estimates (block group level) from the models were aggregated for each county and compared with 2006 county-level estimates provided by the US Bureau of Census. To facilitate assessment, a comparison index, which is the ratio between aggregated county-level model estimate and census approximation, was constructed. A comparison index value above 1 indicates an overestimation of population. Conversely, a comparison index value below 1 indicates an underestimation of population. lists 2006 county-level population estimates by two-, five- and seven-county models in comparison with the 2006 county-level census projections.
Table 2. 2006 county-level population estimates by two-, five- and seven-county models in comparison to the 2006 census projections.
indicates population was most accurately estimated for Hennepin and Ramsey Counties in the two-county model. On the contrary, for the five-county model, population of the five suburban counties was better estimated than the two highly developed counties. In general, the population for the two highly urbanized counties was underestimated by all models, whereas population for the five suburban counties was overestimated in a majority of the cases. For the five-county model, four of the seven counties in the TCMA (Anoka, Carver, Dakota and Scott) have estimates within 3% of the census projections for 2006. The population of Carver County was underestimated by a population of only 342, making it the most accurately estimated county for this model. In particular, the least accurately estimated county population is Washington County for all three cases. Cloud cover over a section of residential land cover in Washington County produced higher impervious values for that area, which in turn may have amplified the overestimation effect for this county.
4 Discussion
Although extracting impervious surface for residential land uses (Method B) provides more accurate population estimation models than merely masking out major road centrelines and areas above 75% imperviousness (Method A), it can be difficult to incorporate Method B for areas where accurately generalized land use data sets are not available. Although land cover and land use maps can be created by classifying remote sensing images with moderate accuracy, the process takes extra time and effort. Therefore, it may still be beneficial to use Method A to create a population estimation model if accurate land use data are not available. In the two-county (Hennepin and Ramsey) model, population densities were most accurately estimated for the same two highly urbanized counties. On the contrary, for the five-county model, the corresponding five suburban counties were better estimated. This may imply that segregating a large study area into several smaller sub-regions based on their geographic characteristics may improve the model's effort.
For both methods, the strongest R 2 and lowest MARE between estimated density and true density come with the five-county suburban model whereas the two-county highly urbanized model ranks last for both years. Generally, the MAREs are much higher for Method A, demonstrating a much lower overall goodness-of-fit for this model. This may imply that using the 75% imperviousness cut-off threshold to represent residential areas in this method is not ideal, which is reasonable because some non-residential land uses such as industrial, airport and mixed uses may also have imperviousness values less than this threshold. We also found out the large MARE values for Method A of 1991 are caused by ∼10–20 outliers in the dataset. When the outliers are removed, the MARE values decrease dramatically to less than 38, 84 and 56 for the two-, five- and seven-county models respectively. However, the RMSEs are relatively high and not correspondingly reduced for Method B, which may suggest there are some outliers or large errors in the test samples, particularly when the test samples are located in multifamily high-rise residential areas, We can also see the RMSEs are not most useful here in this study because they give a higher weight to outliers or large errors because the errors are squared before they are averaged.
Conforming to findings by Harvey (Citation2002b) and Lu et al. (Citation2006), population density was overestimated for many block groups that are in suburban and/or rural areas whereas population density was almost exclusively underestimated for highly urbanized block groups. One factor leading to overestimation may be overestimation of per cent impervious surface for rural areas because of some degree of confusion between impervious surface and bare soil during the process of spectral mixture analysis. Another possibility might be the presence of more impervious surface per head of population in some types of rural areas, due to agricultural operations (farm buildings and paved areas) or lifestyle differences (larger residence footprints, more associated outbuildings and paved recreational areas). Clouds in the 2006 Landsat TM image also affected the population estimation for Washington County. The main reason for underestimation of highly urbanized areas is that two dimensional (2D) models cannot accurately estimate population for multi-level residential buildings since they cannot detect population vertically.
By comparing the relationship between impervious surface and population density for 1991 and 1999, we found the rate of the increase of population density for each 1% increase in per cent impervious surface declined from 1991 to 1999. This indicates that during the 1990s, there was more extensification of new residential development as compared with intensification of residential development. Although the Metropolitan Council seeks to reinvest in ‘older’ areas throughout the region to ‘ease development pressures on rural land’ as a long-term goal (Metropolitan Council Citation2004), it is not likely that the pressure for development along the urban fringe was eased during the 1990s because of the expansive nature of development in that period. This finding may imply that regional urban planning strategies promoting intensification of development were not implemented very effectively within the TCMA in the decade 1990–1999.
One thing we want to emphasize here is, the census block group data, rather than block, was used in this study because the census block group represents the most disaggregated unit in the census hierarchy of spatial data whose size is population sensitive. And census block group boundaries never cross over state lines, county lines, census tracts or other ‘statistically significant entities’ (US Bureau of the Census Citation2001). Nevertheless, variation may be observed in the obtained estimates of the regression and correlation coefficients when the sizes of the areal units are altered. When data are aggregated above the block group level, there is potential for increasingly accurate estimation of population density that can be used to gain a more detailed understanding of urban population dynamics in the TCMA. For example, when applying the 1999/2000 model to 2006 residential impervious surface image and aggregating the block group level estimation to county-level, four of the seven counties in the TCMA have the estimates within ±3% of the census projection. This result also suggests that the relationship between per cent imperviousness and population density were relatively stable between 1999 and 2006 which explains why the 1999/2000 model provided an accurate estimate of population for the 2006 image.
5 Conclusions
In comparison to conventional population estimation methods, satellite remote sensing provides a more effective way and better visual representation tool to estimate population. Statistics and distribution of population within and surrounding the ‘urban core’ can be generated efficiently. The residential %ISA based on a TM image can be moderately well-correlated to census block group data using a power equation. The methods and models created may be applied to different cities or images with similar geographic characteristics. Comparing population models for different years also provides a better understanding of the dynamic relationship between urban expansion and population growth. With a better understanding of this relationship, urban and regional planners can implement policies in an effort to influence growth patterns in either urban density or population density. Temporal changes in this relationship can also provide an indication of the types of models that urban and regional planners are using in regard to development in their jurisdictions. In future research, the relationship of per cent imperviousness to population density for the TCMA will be monitored continuously based on the availability of Landsat imagery. High resolution IKONOS and Quickbird imagery, coupled with Light Detection and Ranging (LiDAR) data, may be used to build a more accurate three-dimensional (3D) model.
References
- Chander , G. and Markham , B. 2003 . Revised landsat-5 TM radiometric calibration procedures and postcalibration dynamic ranges . IEEE Transactions on Geoscience and Remote Sensing , 41 : 2674 – 2677 .
- Dobson , J. E. 2000 . LandScan: a global population database for estimating populations at risk . Photogrammetric Engineering and Remote Sensing , 66 : 849 – 857 .
- Dougherty , M. 2004 . Evaluation of impervious surface estimates in a rapidly urbanizing watershed . Photogrammetric Engineering and Remote Sensing , 70 : 1275 – 1284 .
- Harris , R. J. and Longley , P. A. 2000 . New data and approaches for urban analysis, modelling residential densities . Transactions in GIS , 3 : 217 – 234 .
- Harvey , J. T. 2002a . Population estimation models based on individual TM pixels . Photogrammetric Engineering and Remote Sensing , 68 : 1181 – 1192 .
- Harvey , J. T. 2002b . Estimating census district populations from satellite imagery: some approaches and limitations . International Journal of Remote Sensing , 23 : 2071 – 2095 .
- Harvey , J. T. 2003 . “ Population estimation at the pixel level: developing the expectation maximisation technique ” . In Remotely sensed cities , Edited by: Mesev , V. 181 – 205 . London : Taylor & Francis .
- Iisaka , J. and Hegedus , E. 1982 . Population estimation from landsat imagery . Remote sensing of environment , 12 : 259 – 272 .
- Langford , M. , Maguire , D. J. and Unwin , D. J. 1991 . “ The areal interpolation problem: estimating population using remote sensing in a GIS framework ” . In Handing geographical information: methodology and potential applications , Edited by: Masser , L. and Blakemore , M. 55 – 77 . New York : Longman Scientific & Technical/John Wiley & Sons .
- Lee , S. and Lathrop , R. G. 2006 . Subpixel analysis of landsat ETM+ using self-organising map (SOM) neural networks for urban land cover characterisation . IEEE Transactions on Geoscience and Remote Sensing , 44 : 1642 – 1654 .
- Li , G. and Weng , Q. 2005 . Using landsat ETM+ imagery to measure population density in Indianapolis, Indiana, USA . Photogrammetric Engineering and Remote Sensing , 71 : 947 – 958 .
- Lo , C. P. 1986 . Applied remote sensing , 393 London : Longman .
- Lo , C. P. 1995 . Automated population and dwelling unit estimation from high-resolution satellite images: a GIS approach . International Journal of Remote Sensing , 16 : 17 – 34 .
- Lo , C. P. 2001 . Modelling the population of China using DMSP operational linescan system nighttime data . Photogrammetric Engineering and Remote Sensing , 67 : 1037 – 1047 .
- Lo , C. P. 2003 . “ Zone-based estimation of population and housing units from satellite generated land use/land cover maps ” . In Remotely sensed cities , Edited by: Mesev , V. 157 – 180 . London : Taylor & Francis .
- Lu , D. and Weng , Q. 2004 . Spectral mixture analysis of the urban landscape in Indianapolis city with landsat ETM+ imagery . Photogrammetric Engineering and Remote Sensing , 70 : 1053 – 1062 .
- Lu , D. , Weng , Q. and Li , G. 2006 . Residential population estimation using remote sensing derived impervious surface . International Journal of Remote Sensing , 27 : 3553 – 3570 .
- Martin , D. 1998 . 2001 Census output areas: from concept to prototype . Population Trends , 94 : 19 – 24 .
- Mennis , J. 2003 . Generating surface models of population using dasymetric mapping . Professional Geographer , 55 : 31 – 42 .
- Metropolitan Council . 2004 . 2030 Regional development framework , Saint Paul, MN : Metropolitan Council .
- Metropolitan Council . 2006 . Generalised land use–historical 1984, 1990, 1997, 2000 and 2005, for the twin cities metropolitan area [online] Available from: http://www.datafinder.org/metadata/landuse_hist.htm [Accessed 13 October 2007]
- Phinn , S. 2002 . Monitoring the composition of urban environments based on the vegetation-impervious surface-soil (VIS) model by subpixel analysis techniques . International Journal of Remote Sensing , 23 : 4131 – 4153 .
- Ridd , M. K. 1995 . Exploring a V-I-S (vegetation-impervious surface-soil) model for urban ecosystems analysis through remote sensing: comparative anatomy for cities . International Journal of Remote Sensing , 16 : 2165 – 2186 .
- Roberts , D. A. 1998 . Mapping chaparral in the Santa Monica mountains using multiple endmember spectral mixture models . Remote Sensing of Environment , 65 : 267 – 279 .
- Tobler , W. R. 1969 . Satellite confirmation of settlement size coefficients . Area , 1 : 30 – 34 .
- U.S. Bureau of the Census . 1990 . State and local agencies preparing population and housing estimates Current population reports, series P-25, No. 1063. Washington, DC: U.S. Government Printing Office
- U.S. Bureau of the Census . 2001 . Census block groups cartographic boundary files descriptions and metadata [online] Available from: http://www.census.gov/geo/www/cob/bg_metadata.html [Accessed 10 June 2007]
- U.S. Bureau of the Census . 2005 . Estimates and projections area documentation state and county total population estimates [online] Available from: http://www.census.gov/popest/topics/methodology/2005_st_co_meth.html [Accessed 22 Dec 2007]
- U.S. Bureau of the Census . 2006 . US census press releases: nation's population to reach 300 million on October 17 [online] Available from: http://www.census.gov/Press-Release/www/releases/archives/population/007616.html [Accessed 22 Dec 2007]
- Weng Q. Remote sensing of impervious surfaces CRC Boca Ratan, FL 2007 454
- Wu , C. 2004 . Normalised spectral mixture analysis for monitoring urban composition using ETM+ imagery . Remote Sensing of Environment , 93 : 480 – 492 .
- Wu , C. and Murray , A. T. 2003 . Estimating impervious surface distribution by spectral mixture analysis . Remote Sensing of Environment , 84 : 493 – 505 .
- Wu , C. and Murray , A. T. 2007 . Population estimation using landsat enhanced thematic mapper imagery . Geographical Analysis , 39 : 26 – 43 .
- Wu , C. and Yuan , F. 2007 . Seasonal sensitivity analysis of impervious surface estimation with satellite imagery . Photogrammetric Engineering and Remote Sensing , 73 : 1393 – 1401 .
- Wu , S. , Qiu , X. and Wang , L. 2005 . Population estimation methods in GIS and remote sensing: a review . GIScience and Remote Sensing , 42 : 58 – 74 .
- Yang , L. 2003 . An approach for mapping large-area impervious surfaces: synergistic use of landsat-7 ETM+ and high spatial resolution imagery . Canadian Journal of Remote Sensing , 29 : 230 – 240 .
- Yuan , F. 2005 . Multi-level land cover mapping of the twin cities (Minnesota) metropolitan area with multi-seasonal landsat TM/ETM+ data . Geocarto International , 20 : 5 – 14 .
- Yuan , F. , Wu , C. and Bauer , M. E. 2008 . Comparison of various spectral analytical techniques for impervious surface estimation using landsat imagery . Photogrammetric Engineering and Remote Sensing , 74 : 1045 – 1055 .
- Yuan , Y. , Smith , R. M. and Limp , W. F. 1997 . Remodeling census population with spatial information from landsat TM imagery . Computers, Environment and Urban Systems , 21 : 245 – 258 .