Abstract
Maize is a staple food, but productivity has stagnated due to limited access to advanced farming methods and knowledge. To promote sustainable agriculture, understanding the factors affecting maize yield at the farm level is crucial. This study used panel data on maize yield and agronomic practices in Northern Ghana and Malawi from 2014 to 2020. Satellite-based environmental variables were extracted at household locations, and Random Forest modeling was used to identify factors influencing maize yield variability. The models performance was sub-par with low R2 values (∼0.1 and ∼0.24 for Northern Ghana and Malawi). Fertilizer and precipitation were the most important factors explaining maize yield variability. Spatial maps showed that Malawi’s maize yield can increase with more fertilizer, but rainfall is essential. In Northern Ghana, relying solely on fertilizer may not be enough to boost maize production.
Survey data on maize is limited in making accurate yield predictions.
Fertilizer use can increase maize yield in both Northern Ghana and Malawi.
Fertilizer use intervention strategies should be region-specific.
The efficiency of fertilizer use is dependent on adequate rainfall availability.
KEY POLICY HIGHLIGHTS
1. Introduction
Increased agricultural productivity is critical for Sub-Saharan Africa’s (SSA) economic growth, poverty alleviation, and improved nutrition for the region’s growing population. Maize (Zea mays L) is the second most cultivated and staple crop among SSA families, and it is primarily grown by small-scale farmers (Oluoch et al. Citation2022). Maize yield variance in SSA is influenced by agronomic, biophysical, and socio-economic factors such as variety type, soil fertility, fertilizer application, intercropping, crop rotation, irrigation, farm labour allocation, minimum tillage, input costs, and climatic shifts, among others (Danquah et al. Citation2020). However, the effect of these factors at the field level is lacking in most SSA countries because maize yield data is typically aggregated to larger administrative units, which averages out salient features of spatial and temporal variability in yield data (Vergopolan et al. Citation2021). For example, even in farms with similar environmental conditions, a farmer’s choice of maize cultivar, fertilizer, or pesticides can result in inter-farm yield variability (Muthoni Citation2021). Therefore, characterizing the drivers of crop production at the farm level is crucial for enabling evidence-based scaling out of sustainable agronomic methods that boost maize productivity.
Globally, machine learning algorithms such as Random Forest (RF) have proven to be more accurate in predicting and characterizing crop yield drivers because they can handle large amounts of data and decode complex non-linear relationships between the response variable and the predictor variables (Delerce et al. Citation2016). For example, Lohitha Reddy and Siva Kumar (Citation2023) employed three different machine learning techniques (decision tree classifier, random forest classifier, and gradient boosting) to forecast crop yields using weather and soil properties as predictor variables. Their study revealed that the random forest classifier outperformed other algorithms in accurately predicting yield. Cai et al. (Citation2019) found that ML methods outperformed than Ordinary Least Square regression when predicting wheat yield in Australia and also reported that combining climatic and vegetation indices data improved prediction of wheat yield. Additionally, other studies have utilized RF machine learning techniques to predict crop yield with high precision, such as Charoen-Ung and Mittrapiyanuruk (Citation2019) predicted sugarcane yield using RF and forward feature selection, Jeong et al. (Citation2016) forecasted the yields for maize, wheat, and potato tubers, Everingham et al. (Citation2016) predicted sugarcane yield in Tully, Australia, and Ahmad et al. (Citation2018) who predicted maize yield in Pakistan.
It is commonly assumed that machine learning methods like RF are immune to overfitting. However, including skewed training samples, and irrelevant and redundant predictor variables can significantly overfit the model when extrapolating beyond the areas where the model was trained (Meyer et al. Citation2018, Meyer et al. Citation2019; Meyer and Pebesma Citation2021). Furthermore, most data in nature are geographically dependent. Ignoring spatial dependencies in machine learning models might result in models that perform well on training data but fall short on spatial predictions (Meyer et al. Citation2019). As a result, applying feature selection approaches that incorporate target-oriented cross-validation (CV) processes, such as Leave-Location-Out (LLO), is critical for improving the model’s performance beyond the training area and preventing spatial overfitting (Meyer et al. Citation2019).
In this study, we used a panel household survey data on maize yield and agronomic practices from Ghana and Malawi to 1) identify the target-oriented feature selection and cross-validation strategies that improve the performance of the RF model for predicting maize yield; 2) identify the most important sustainable agriculture intensification practices and socio-economic factors that explain variance in maize yield; and 3) predict the spatial distribution of maize yield under different management practices. The results of this research will provide information on where to scale out specific bundles of sustainable agriculture intensification (SAI) technologies with a low probability of failure.
2. Materials and methods
2.1. Study area
The study area covers two countries in SSA i.e. Ghana and Malawi (). Maize is a crucial crop in both countries, and its growth is heavily dependent on rainfall. Around 90% of smallholder farmers in Ghana and 97% in Malawi rely on maize farming as their primary source of income (Msowoya et al. Citation2016; Scheiterle and Birner Citation2018; White Citation2019). In Ghana, approximately 85% of maize production is consumed by humans, providing about 30% of the combined calorie intake when combined with other cereals such as rice and wheat, while the remaining 15% is used for animal feed to supplement poultry and livestock production (Andam et al. Citation2017; Adu et al. Citation2021). In Malawi, maize makes up more than half of the total calorie intake, with the central region having the largest harvested area, followed by the southern region (Warnatzsch et al. Citation2020). Soil infertility and inadequate use of improved cultivars are the two major obstacles to maize productivity in Ghana (Marfo-Ahenkora Citation2020), while in Malawi, the total family income and off-farm employment are the major determinants of maize yield productivity (Tamene et al. Citation2016). Climate variability has exacerbated maize productivity, resulting in malnutrition, poor human development, and a higher poverty index among small-scale farmers who rely on maize production for a living (Shi and Tao Citation2014; Parkes et al. Citation2018; Ngcamu and Chari Citation2020). As a result, determining the best agronomic strategies for increasing maize yield at the farm level will allow these countries to make data-driven decisions to increase yield.
Figure 1. Map of the study showing the location of the survey households and zones with relatively similar rainfall patterns. The rainfall zones were generated from long-term (2014–2020) aggregation of annual TerraClimate satellite rainfall estimates.
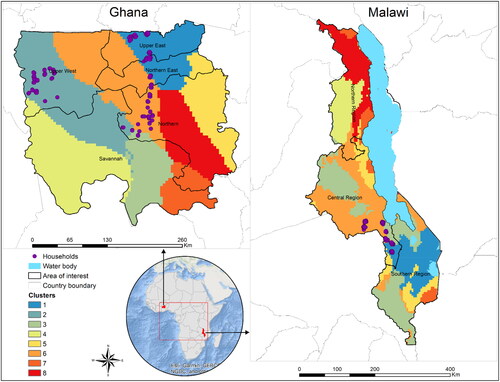
The Palmer Severity Drought Index (PDSI) is a reliable measure used to assess the level of dryness or wetness in comparison to a historical average for a specific time period. In our study we utilised the PDSI (Abatzoglou et al. Citation2018) to evaluate the weather conditions for the two regions and seasons. We observed that the 2018–2019 growing season in Malawi was very wet while rainfall in all other seasons of both regions was below the normal ranges with extreme droughts in Ghana during 2019 season ().
2.2. Agronomic data
A panel household survey was conducted in Ghana and Malawi in 2013 and 2019 under the Africa RISING program (https://africa-rising.net/; Tinonin et al. Citation2016). During the two surveys, respondents provided information on household demographics and production practices ().
Table 1. Variables used in the models.
2.3. Remote sensing variables
The gridded earth observation data were extracted using Google Earth Engine (GEE) cloud computing platform (Gorelick et al. Citation2017). These variables include the vegetation indices, meteorological, topography, socio-economic, hydrological, and soil properties (). Vegetation indices and meteorological data were generated for each month during the respective country’s maize growing season.
Table 2. Gridded environmental and weather data used in the model.
2.4. Model training and evaluation
Eliminating irrelevant and redundant predictor variables in machine learning models is important because their inclusion can reduce the model’s performance. While many feature elimination techniques are available, we used the VSURF feature elimination method, which is included the VSURF package (Genuer et al. Citation2022) in the R programming (R Core Team, Citation2020), to eliminate irrelevant or redundant variables. VSURF eliminates feature in three processes i.e. thresholding, interpretation and predictive step. The first step eliminates irrelevant variables from the dataset. The second step selects all variables related to the response for interpretation purpose. The third step refines the selection by eliminating redundancy in the set of variables selected by the second step, for prediction purpose. We focused on variables that were retained at the thresholding step, which retains or drops variables based on how important they are in explain the response variables. Because most continuous household survey data lacked corresponding raster data, we developed models that included all household survey data and those that only had categorical data to enable spatial predictions under various agronomic scenarios. To elaborate, the categorical household survey data allowed these variables to be converted into dummy variables, which could then be combined with the gridded raster data and toggled on (1) or off (0) to visualize the impact of using or not using the respective agronomic variable. What ‘appending dummy variables to gridded data’ does is create a grided layer of zeros for each pixel (not using agronomic practices), and this layer can be turned on by replacing all values with 1, indicating that all spaces use the agronomic variable. The VSURF elimination method was applied to the two sets of the dataset (all predictors and only categorical household survey data) independently. We used the ‘ranger’ method, as implemented by the train function in the caret R package (Khun Citation2022), to train the maize yield models and used the permutation method to rank the variable importance. Before training the model, we used the CAST package to generate training and testing folds of Leave-Location-Out (LLO) cross-validation. The LLO methodology internally subsets the testing and training set and thus we did not withhold any data for independent testing of the models. We then optimized the model by calculating the best mtry for each dataset separately. The Root Mean Square Error (RMSE) and R-squared (R2) values were used to assess the model’s performance where higher R2 and lower RMSE values indicate a better model performance. We employed the varmImp function in the caret package to determine and rank the significance of the variables. To gain insights into the relationship between maize yield and the predictors, we generated partial dependence plots for the top six predictors using the pdp R package (Greenwell Citation2022). These plots provide a visual representation of the direction of the relationship between the response and the predictor variable.
3. Results
3.1. Descriptive analysis
We investigated the distribution of maize yield for each season and country at different rainfall clusters using box plots (). To annotate the various datasets, we will refer to Ghana data as D1 and D2 for the 2013 and 2019 surveys, respectively, and Malawi data as D3 and D4 for the 2013 and 2019 surveys, respectively.
Figure 3. Boxplots showing the distribution of the maize yield data for Ghana and Malawi with (a) and without (b) outliers were removed. The blue text is the number of households per cluster. The clusters are as described in .
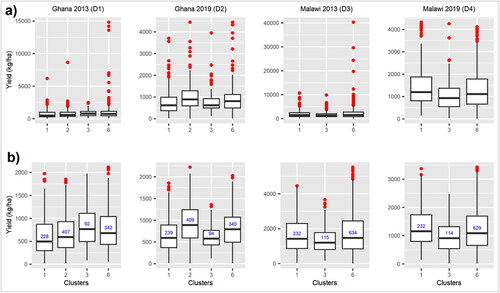
To better understand the distribution of maize yield data based on all the predictor variables, we created histograms (Appendix 1) for various continuous variables for each country and season individually. The total number of predictor variables for D1 and D3 was 43, while D2 had 56 predictors and D4 had 57 predictors.
3.2. Feature elimination
The count of predictor variables that remained after elimination is presented in . Additional information on the actual names of the predictors that were retained can be found in the supplementary information (SS1).
Table 3. The number of retained predictor variables after the VSURF thresholding step.
3.3. Model performances, variable importance, partial dependence plots and spatial predictions
The models only explained a small variability in maize yield with low R2 values across all seasons for each country (). When continuous household data were used, the explained variability was greater (11–15% in Northern Ghana and 24–35% in Malawi) than when only categorical data were used (6–10% in Northern Ghana and 7–14% in MalawiThis implies that the quantity of measurable agronomic practices used explains yield variability better than whether or not that agronomic variable is used. For both countries and seasons, the RMSE values obtained were consistently high, with normalized RMSE values (nRMSE; RMSE/mean yield) exceeding 50%. These values suggest that the model predictions were either overestimating or underestimating the actual yield by a significant margin, often by as much as twice or half the true value. The results underscore the necessity of refining the predictive model to enhance its accuracy and practical applicability.
Table 4. Model performance metrics when all predictors were used and when only the categorical household survey was used.
Previous studies have reported the usefulness of fertilizer application in increasing maize yield in Northern Ghana (Braimoh and Vlek Citation2006; Kanton et al. Citation2016; Buah et al. Citation2017). Our analysis identified the amount of fertilizer used per hectare and total income () as the most significant agronomic practices that positively () influenced maize yield in Ghana in 2013. Interestingly, when considering only the categorical version of the agronomic practices, the importance of these two variables was relatively low (), indicating that the quantity used mattered more than simply their presence or absence. Both datasets showed that the total amount of rainfall experienced in October - which marks the end of the season - had a positive influence on maize yield (). Agronomic practices were found to be poorly correlated with maize yield variability in 2019 (). Instead, the most significant factors influencing yield productivity were August precipitation, which had a positive effect, and temperature, which had a negative impact (). The observed dynamics may be attributed to the exceptionally dry season (), which resulted in reduced soil moisture and likely exacerbated the effects of temperature on yield.
Figure 4. Variable importance and partial dependence plots for Ghana in 2013. (a) and (b) All predictor and categorical variables importance plots respectively. (c) and (d) Partial dependence plots for the top 6 predictors with all predictors and only with categorical variables respectively.
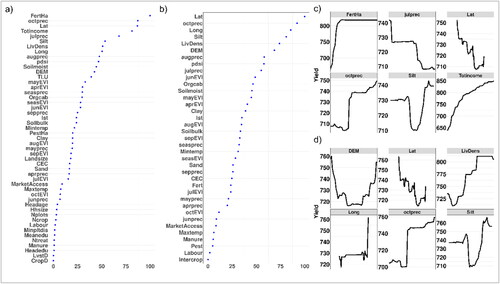
Figure 5. Variable importance and partial dependence plots for Ghana in 2019. (a) and (b) All predictor and categorical variables importance plots respectively. (c) and (d) Partial dependence plots for the top 6 predictors with all predictors and only with categorical variables respectively.
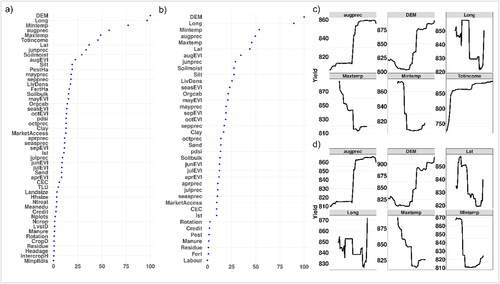
The spatial prediction maps indicated that introducing fertilizer as an agronomic practice resulted in minimal improvements in maize yield in both seasons (). The yield gain observed was relatively low (<50kg/ha) but parts of the upper west and northern region had higher yield gain of more than 50 kg/ha (). The limited yield gain observed may be attributed to two key factors: first, the relatively dry conditions during the two seasons (); and second, the low ranking of fertilizer use (yes/no) as a significant predictor of yield.
Figure 6. The spatial maize yield prediction and yield gain for Ghana in 2013 and 2019 when fertilizer use was incorporated as a useful agronomic practice. (a) When no agronomic practice was used. (b) Fertilizer use and (c) yield gain/loss ( – ).
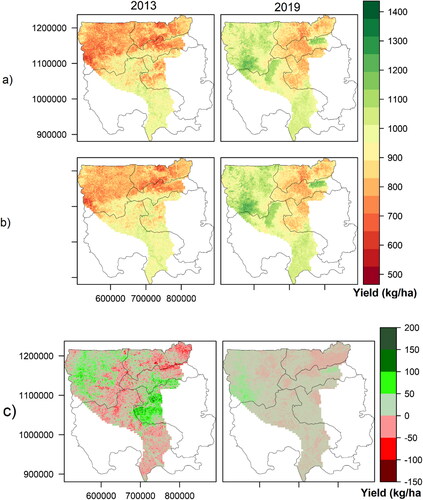
While recent studies have found a limited yield response to fertilizer use in Malawi (Burke et al. Citation2022; De Weerdt and Duchoslav Citation2022), several other studies have demonstrated that applying fertilizer and improving access to it can significantly boost maize yield productivity (Sauer and Tchale Citation2009; Wang et al. Citation2019; Burke and Jayne Citation2021; Cairns et al. Citation2021; Cassim and Pemba Citation2022). According to the 2013 season analysis, fertilizer usage per hectare and the extent of land devoted to maize cultivation were the primary factors accounting for yield variability (), with the former exerting a positive effect and the latter having a negative impact. respectively (). Although soil moisture was identified as the most critical variable affecting maize yield when categorical agronomic practices were employed for predictions (), the observation that yield declined with increasing soil moisture () during a relatively dry season () is perplexing. Fertilizer use (both quantity and yes/no) was an important factor in 2019 () with a positive effect on maize yield (). Total household income and labor were significant factors as continuous variables this season, but labor was less important in categorical analysis (). Livestock density had the most significant positive impact in categorical analysis, likely due to manure use and its positive effect on maize yield (Wang et al. Citation2019).
Figure 7. Variable importance and partial dependence plots for Malawi in 2013. (a) and (b) All predictor and categorical variables importance plots respectively. (c) and (d) Partial dependence plots for the top 6 predictors with all predictors and only with categorical variables respectively.
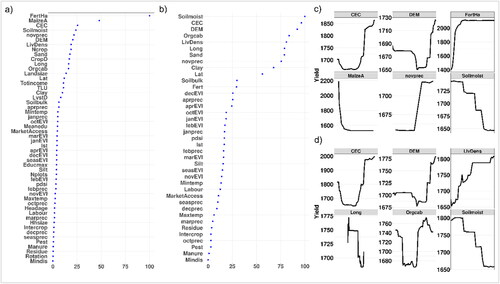
Figure 8. Variable importance and partial dependence plots for Malawi in 2019. (a) and (b) All predictor and categorical variables importance plots respectively. (c) and (d) Partial dependence plots for the top 6 predictors with all predictors and only with categorical variables respectively.
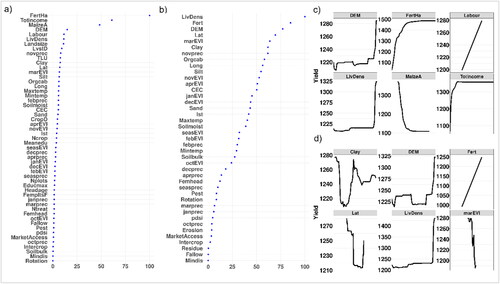
Spatial predictions based on agronomic models demonstrated that the introduction of fertilizer in the 2019 growing season resulted in a significantly greater increase in maize yield as compared to the 2013 season (). This outcome may be attributed to the favorable soil moisture conditions in 2019 (), which allowed for enhanced fertilizer uptake by crops and ultimately contributed to improved yield. Even so, it is important to note that the average maize yield was higher in 2013 as compared to 2019. Two possible reasons could explain this phenomenon: Firstly, the prevalence of extreme floods and soil erosion in Malawi (McCarthy et al. Citation2021) may have reduced crop yield, particularly given the excessively wet weather in 2019. Secondly, excessively moist environments can increase the incidence of corn ear infections (Wang et al. Citation2019), thereby leading to a decline in yield.
4. Discussion
This study examined the factors that affect the maize yield in different regions and periods in northern Ghana and Malawi. To do this, we looked at various biophysical, socio-economic and farm management practices as potential predictors and used a random forest machine learning algorithm with spatial blocking cross-validation. Despite efforts to develop accurate models, the performance was suboptimal, with explained variability ranging from 6 to 15% in Ghana and between 7 to 35% in Malawi over the course of two seasons (). While it is true that spatial blocking cross-validation can lead to reduced R2 values (Meyer et al. Citation2018; Meyer et al. Citation2019; Meyer and Pebesma Citation2021), there may be other factors that may have contributed to the underperformance of the models. For example, farmers reported yields from a different number of plots that were spatially displaced. These imprecise locations of farmer plots could have introduced errors when matching with remote sensing variables (Burke and Lobell Citation2017; Lobell et al. Citation2020). This can be resolved by aggregating the yield data into larger administrative zones although the practice can mask details in heterogeneous farms. Alternatively, we recommend that household surveys should endeavour to precisely map the plot boundaries to enable matching with satellite data. Also, the maize yield data was based on self-reported estimates and numerous studies have shown that self-reported estimates are frequently inaccurate when compared to farm-level estimates derived from actual harvest measurements (Jin et al. Citation2017; Scheiterle et al. Citation2019; Burke et al. Citation2020; Li et al. Citation2022).
The low spatial resolution of the predictor variables used in the models, which were resampled from about 4 to 0.03 km, could also be a contributing factor to the poor performance of the models. Generating reliable satellite-based productivity estimates for smallholder farms in sub-Saharan Africa, which are typically characterized by small land size and intercropping, is unlikely when using low spatial resolution data due to the presence of mixed crops within a single pixel (Jin et al. Citation2017; Li et al. Citation2022). Studies have demonstrated that utilizing higher spatial resolution satellite data, such as those provided by the Sentinel-2 mission (10 m) and PlanetScope (3 m), has resulted in improved model performance (R2>0.5; Li et al. Citation2022). However, the utility of such high-resolution data is limited by frequent cloud cover and requires significant computational resources, particularly when analyzing vast areas. Furthermore, the choice of satellite-based predictor variables used in this study may have been insufficient in explaining the variations in maize yield. According to Jin et al. (Citation2017) and Burke and Lobell (Citation2017) Green Chlorophyll Vegetation Index (GCVI) is more effective at predicting maize yield than other vegetation indices, likely due to its ability to capture nutrient deficiency, which is highly correlated with yield. In addition, factors such as Leaf Area Index (LAI), radiation, and sowing period have been identified as good predictors of maize yield in several studies (Srivastava et al. Citation2017; Lambert et al. Citation2018; Danquah et al. Citation2020; Li et al. Citation2022).
Maize farming in the sub-Saharan Africa region heavily relies on adequate rainfall, which may explain why precipitation and soil moisture emerged as significant factors in explaining the variability of maize yield. Both Malawi and Ghana have made significant investments in fertilizer subsidy programs as part of their efforts to increase maize productivity (Mapila et al. Citation2012; Fearon et al. Citation2015; Ragasa and Chapoto Citation2017; Scheiterle and Birner Citation2018; Andani et al. Citation2020; Cassim and Pemba Citation2022; De Weerdt and Duchoslav Citation2022). There is a debate on the usefulness of fertilizer subsidy programs, with some studies reporting low yield response (Benin et al. Citation2013; Fearon et al. Citation2015; Andani et al. Citation2020; Burke et al. Citation2022), while others suggest that these programs have led to improved maize productivity by making fertilizers more accessible and increasing their usage (Braimoh and Vlek Citation2006; Chibwana et al. Citation2014; Kanton et al. Citation2016; Buah et al. Citation2017; Wang et al. Citation2019). Our results suggest that the application of fertilizer can significantly enhance maize production in both Malawi and Ghana during seasons with adequate soil moisture. This could be attributed to the fact that both countries face challenges of low soil fertility caused by a combination of factors such as low nutrient levels, continuous cropping, overgrazing, deforestation, and poor soil and water management practices (Tittonell and Giller Citation2013; Vuntade et al. Citation2022).
In terms of yield gain/loss, Malawi saw the highest increase in maize yield () when fertilizers were used, while Ghana experienced a much smaller increase (). The high yield gain in Malawi could be because several studies have linked the use of fertilizer, urea, and manure to high maize yield (Snapp et al. Citation2014; Tamene et al. Citation2016; Liu and Basso Citation2017; Wang et al. Citation2019), as well as intercropping, which acts as a soil fertility replenishment (Akinnifesi et al. Citation2006; Silberg et al. Citation2017). The difference in yield gain between Ghana and Malawi in 2019 may be attributed to Ghana’s comparatively dry season and Malawi’s comparatively wet season, which likely explains why Ghana’s yield increase was low (<50 kg/ha) while Malawi’s was high (> 400 kg/ha). Another possible reason why the Ghana season had a lower yield increase could be the limited access to modern agricultural practices, such as mechanization and the use of improved seed varieties, which continue to constrain productivity (Ragasa and Chapoto Citation2017).
The presence of parasitic weeds like Striga (Scheiterle et al. Citation2019; Adu et al. Citation2022; Martey et al. Citation2022) and pests like fall armyworm (Agboyi et al. Citation2020; Nagoshi et al. Citation2021; Yeboah et al. Citation2021) outbreaks in maize farms and increased cost of pesticide that hinders their control could also be a contributing factor to why fertilizer use does not necessarily result in increased yields. Although hand-picking of the striga weed is a commonly used method, it is not sustainable in the long term (Kabambe et al. Citation2008; Wang et al. Citation2019). Therefore, an integrated approach that incorporates different control methods is necessary to effectively manage the weed. Push-Pull technology, which involves planting desmodium and bracharia grass, has been shown to effectively reduce striga weed infestation and ultimately increase maize yield, offering a sustainable and integrated approach to weed control (Niassy et al. Citation2022). To potentially enhance maize yield productivity, factors such as timely fertilizer application, adjusting planting dates to accommodate climate variability (Fosu-Mensah et al. Citation2019; Warnatzsch and Reay Citation2020), educating farmers on the appropriate fertilizer amounts (Addai and Owusu Citation2014; Asante et al. Citation2019; Wang et al. Citation2019; Andani et al. Citation2020; Cairns et al. Citation2021; Setsoafia et al. Citation2022), and promoting the adoption of improved seed varieties may also be beneficial.
Despite the poor performance of the models in this study, we have identified important variables that are consistent with existing knowledge and previous studies on maize yield. To enhance the model performance, we recommend the following: 1) include satellite-based factors like GCVI and LAI, which have shown better performance in predicting yield; 2) integrate a crop classification map that distinguishes maize and non-maize fields; 3) refine yield data using simple thresholds and generate categorical predictive maps rather than actual yield; and 4) explore simple regression models that directly correlate yield data with vegetation indices, as these have been found to better explain variations in maize yield in sub-Saharan African countries (Jin et al. Citation2017; Li et al. Citation2022).
5. Conclusion
The identification of maize yield determinants through the use of household survey data and low spatial resolution satellite-based estimates of the environment has produced a model that performs moderately. Nonetheless, the significant variables identified align with existing knowledge of the factors that affect maize yield variability both at the farm and larger administrative levels. The findings of this study suggest that promoting the use of fertilizers is a viable option for improving maize yield in Ghana and Malawi. Additionally, since precipitation plays a crucial role in determining yield, it is recommended that measures such as rainwater harvesting be promoted to help cushion against the impact of extreme dry seasons.
Acknowledgements
This study was partly funded by USAID through grant number: AID-BFS-G-11-00002 under the Feed the Future initiative to support Africa RISING program. We would like to thank all funders who support the Sustainable Intensification of Mixed Farming Systems Initiative through their contributions to the CGIAR Trust Fund. The authors further acknowledge funding from Bill and Melinda Gates Foundation (BMGF) for grant number INV-005431 in support of Excellence in Agronomy Initiative.
Data availability statement
All the R programming scripts and excel files used in fitting the models are available in github repository (https://github.com/Muthono19/Predicting-Maize-Yield-in-Ghana-and-Malawi.git). The remote sensing data used is open source and can be downloaded through GEE.
Disclosure statement
Authors declare no conflict of interest.
References
- Abatzoglou JT, Dobrowski SZ, Parks SA, Hegewisch KC. 2018. TerraClimate, a high-resolution global dataset of monthly climate and climatic water balance from 1958–2015. Sci Data. 5(1):1–12. doi: 10.1038/sdata.2017.191.
- Addai KN, Owusu V. 2014. Technical efficiency of maize farmers across various agro ecological zones of Ghana. J Agric Environ Sci. 3(1):2334–2412.
- Adu GB, Badu-Apraku B, Akromah R, Amegbor IK, Adogoba DS, Haruna A, Manigben KA, Aboyadana PA, Wiredu AN. 2021. Trait profile of maize varieties preferred by farmers and value chain actors in northern Ghana. Agron Sustain Dev. 41(4):50. doi: 10.1007/s13593-021-00708-w.
- Adu GB, Badu-Apraku B, Akromah R, Awuku FJ. 2022. Combining abilities and heterotic patterns among early maturing maize inbred lines under optimal and striga-infested environments. Genes. 13(12):2289. doi: 10.3390/GENES13122289/S1.
- Agboyi LK, Goergen G, Beseh P, Mensah SA, Clottey VA, Glikpo R, Buddie A, Cafà G, Offord L, Day R, et al. 2020. Parasitoid complex of fall armyworm, Spodoptera frugiperda, in Ghana and Benin. Insects. 11(2):68. doi: 10.3390/insects11020068.
- Ahmad I, Saeed U, Fahad M, Ullah A, Habib Ur Rahman M, Ahmad A, Judge J. 2018. Yield forecasting of spring maize using remote sensing and crop modeling in Faisalabad-Punjab Pakistan. J Indian Soc Remote Sens. 46(10):1701–1711. doi: 10.1007/S12524-018-0825-8/METRICS.
- Akinnifesi FK, Makumba W, Kwesiga FR. 2006. Sustainable maize production using gliricidia/maize intercropping in southern Malawi. Ex Agric. 42(4):441–457. doi: 10.1017/S0014479706003814.
- Andam K, Johnson M, Ragasa C, Kufoalor D, Das Gupta S. 2017. A Chicken and Maize Situation: the Poultry Feed Sector in Ghana, AgriSciRN: Agribusiness (Topic).
- Andani A, Moro A-HB, Issahaku G. 2020. Fertilizer subsidy policy and smallholder farmers’ crop productivity: the case of maize production in North-Eastern Ghana. J Agric Extens Rural Dev. 12(2):18–25. doi: 10.5897/JAERD2020.1138.
- Asante BO, Temoso O, Addai KN, Villano RA. 2019. Evaluating productivity gaps in maize production across different agroecological zones in Ghana. Agric Syst. 176:102650. 102650. doi: 10.1016/j.agsy.2019.102650.
- Benin S, Johnson M, Abokyi E, Ahorbo G, Jimah K, Nasser G, Owusu V, Taabazuing J, Tenga A. 2013. Revisiting agricultural input and farm support subsidies in Africa: the case of Ghana’s mechanization, fertilizer, block farms, and marketing programs. SSRN J. doi: 10.2139/ssrn.2373185.
- Braimoh AK, Vlek PLG. 2006. Soil quality and other factors influencing maize yield in northern Ghana. Soil Use Manage. 22(2):165–171. doi: 10.1111/j.1475-2743.2006.00032.x.
- Buah SSJ, Ibrahim H, Derigubah M, Kuzie M, Segtaa JV, Bayala J, Zougmore R, Ouedraogo M.,. 2017. Tillage and fertilizer effect on maize and soybean yields in the Guinea savanna zone of Ghana. Agric Food Secur. 6(1):1–11. doi: 10.1186/S40066-017-0094-8/TABLES/5.
- Burke M, Lobell DB. 2017. Satellite-based assessment of yield variation and its determinants in smallholder African systems. Proc Natl Acad Sci U S A. 114(9):2189–2194. doi: 10.1073/PNAS.1616919114/SUPPL_FILE/PNAS.201616919SI.PDF.
- Burke WJ, Jayne TS. 2021. Disparate access to quality land and fertilizers explain Malawi’s gender yield gap. Food Policy. 100:102002. doi: 10.1016/j.foodpol.2020.102002.
- Burke WJ, Jayne TS, Snapp SS. 2022. Nitrogen efficiency by soil quality and management regimes on Malawi farms: can fertilizer use remain profitable? World Dev. 152:105792. doi: 10.1016/j.worlddev.2021.105792.
- Burke WJ, Snapp SS, Jayne TS. 2020. An in-depth examination of maize yield response to fertilizer in Central Malawi reveals low profits and too many weeds. Agric Econ. 51(6):923–940. doi: 10.1111/agec.12601.
- Cai Y, Guan K, Lobell D, Potgieter AB, Wang S, Peng J, Xu T, Asseng S, Zhang Y, You L, et al. 2019. Integrating satellite and climate data to predict wheat yield in Australia using machine learning approaches. Agric For Meteorol. 274:144–159. doi: 10.1016/j.agrformet.2019.03.010.
- Cairns JE, Chamberlin J, Rutsaert P, Voss RC, Ndhlela T, Magorokosho C.,. 2021. Challenges for sustainable maize production of smallholder farmers in sub-Saharan Africa. J Cereal Sci. 101:103274. doi: 10.1016/j.jcs.2021.103274.
- Cassim L, Pemba LA. 2022. The interactive effects of farm input subsidy program and agricultural extension eervices on smallholder maize production and Technical Efficiency in Malawi. Malawi J Econ. 2(1):66–84. Available at: https://www.ajol.info/index.php/mje/article/view/228064. (Accessed: 8 March 2023).
- Cattaneo A, Nelson A, McMenomy T. 2021. Global mapping of urban-rural catchment areas reveals unequal access to services. Proc Natl Acad Sci USA. 118(2):e2011990118. doi: 10.1073/PNAS.2011990118/SUPPL_FILE/PNAS.2011990118.SD01.XLSX.
- Charoen-Ung P, Mittrapiyanuruk P. 2019. Sugarcane yield grade prediction using random forest with forward feature selection and hyper-parameter tuning. Adv Intell Syst Comput. 769:33–42. doi: 10.1007/978-3-319-93692-5_4/COVER.
- Chibwana C, et al. 2014. Measuring the impacts of Malawi’s farm input subsidy programme. Afr J Agric Resour Econ. 9(2):132–147. doi: 10.22004/AG.ECON.176511.
- Danquah EO, et al. 2020. Monitoring and modelling analysis of maize of maize (Zea mays L) yield gap in smallholder farming in Ghana. Agriculture. 10(9):420. doi: 10.3390/AGRICULTURE10090420.
- Delerce S, Dorado H, Grillon A, Rebolledo MC, Prager SD, Patiño VH, Garcés Varón G, Jiménez D. 2016. Assessing weather-yield relationships in rice at local scale using data mining approaches. PLoS One. 11(8):e0161620. doi: 10.1371/JOURNAL.PONE.0161620.
- Didan K. 2015. MOD13Q1 MODIS/Terra vegetation indices 16-day L3 global 250m SIN Grid V006 [Dataset]. NASA EOSDIS Land Processes DAAC. accessed 2023-02-22 from doi: 10.5067/MODIS/MOD13Q1.006.
- Everingham Y, Sexton J, Skocaj D, Inman-Bamber G. 2016. Accurate prediction of sugarcane yield using a random forest algorithm. Agron Sustain Dev. 36(2):1–9. doi: 10.1007/S13593-016-0364-Z/FIGURES/3.
- Farr TG, Rosen PA, Caro E, Crippen R, Duren R, Hensley S, Kobrick M, Paller M, Rodriguez E, Roth L, et al. 2007. The shuttle radar topography mission. Rev Geophys. 45(2):2004. doi: 10.1029/2005RG000183.
- Fearon J, Adraki PK, Boateng VF. 2015. ‘Fertilizer subsidy programme in Ghana: evidence of performance after six years of implementation’. 5(21). Available at: www.iiste.org. (Accessed: 8 March 2023).
- Fosu-Mensah BY, Manchadi A, Vlek PLG. 2019. Impacts of climate change and climate variability on maize yield under rainfed conditions in the sub-humid zone of Ghana: a scenario analysis using APSIM. West Afr J Appl Ecol. 27(1):108–126. doi: 10.4314/wajae.v27i1.
- Genuer, R, Jean-Michel, P, Christine, TM,Variable selection using random forests (VSURF), CRAN Repos, 2022
- Gorelick N, Hancher M, Dixon M, Ilyushchenko S, Thau D, Moore R.,. 2017. Google Earth Engine: planetary-scale geospatial analysis for everyone. Remote Sens Environ. 202:18–27. doi: 10.1016/j.rse.2017.06.031.
- Greenwell B. 2022. Package ’pdp’- Partial Dependence Plots. Available at: https://cran.r-project.org/web/packages/pdp/pdp.pdf. (Accessed: 10 February 2023).
- Hengl T, Miller MAE, Križan J, Shepherd KD, Sila A, Kilibarda M, Antonijević O, Glušica L, Dobermann A, Haefele SM, et al. 2021. African soil properties and nutrients mapped at 30 m spatial resolution using two-scale ensemble machine learning. Sci Rep. 11(1):1–18. doi: 10.1038/s41598-021-85639-y.
- Jeong JH, Resop JP, Mueller ND, Fleisher DH, Yun K, Butler EE, Timlin DJ, Shim K-M, Gerber JS, Reddy VR, et al. 2016. Random forests for global and regional crop yield predictions. PLoS One. 11(6):e0156571. doi: 10.1371/JOURNAL.PONE.0156571.
- Jin Z, Azzari G, Burke M, Aston S, Lobell D.,. 2017. Mapping smallholder yield heterogeneity at multiple scales in Eastern Africa. Remote Sens. 9(9):931. doi: 10.3390/rs9090931.
- Kabambe VH, Katunga LA, Kapewa T. 2008. Screening legumes for integrated management of witchweeds (Alectra vogelii and Striga asiatica) in Malawi. Afr J Agric Res. 3:708–715.
- Kanton RAL, Prasad PVV, Mohammed AM, Bidzakin JK, Ansoba EY, Asungre PA, Lamini S, Mahama G, Kusi F, Sugri I, et al. 2016. Organic and inorganic fertilizer effects on the growth and yield of maize in a dry agro-ecology in Northern Ghana. J Crop Improve. 30(1):1–16. doi: 10.1080/15427528.2015.1085939.
- Khun M. 2022. Classification and regression training: package caret. Available at: https://cran.r-project.org/web/packages/caret/caret.pdf. (Accessed: 26 January 2023).
- Lambert M-J, Traoré PCS, Blaes X, Baret P, Defourny P. 2018. Estimating smallholder crops production at village level from Sentinel-2 time series in Mali’s cotton belt. Remote Sens Environ. 216:647–657. doi: 10.1016/j.rse.2018.06.036.
- Li C, Chimimba EG, Kambombe O, Brown LA, Chibarabada TP, Lu Y, Anghileri D, Ngongondo C, Sheffield J, Dash J, et al. 2022. Maize yield estimation in intercropped smallholder fields using satellite data in Southern Malawi. Remote Sens. 14(10):2458. doi: 10.3390/rs14102458.
- Liu L, Basso B. 2017. Spatial evaluation of maize yield in Malawi. Agric Syst. 157:185–192. doi: 10.1016/j.agsy.2017.07.014.
- Lobell DB, Azzari G, Burke M, Gourlay S, Jin Z, Kilic T, Murray S. 2020. Eyes in the sky, boots on the ground: assessing satellite- and ground-based approaches to crop yield measurement and analysis. Am J Agric Econ. 102(1):202–219. doi: 10.1093/ajae/aaz051.
- Lohitha Reddy K, Siva Kumar AP. 2023. Machine learning techniques for weather based crop yield prediction. 2023 Third International Conference on Artificial Intelligence and Smart Energy (ICAIS). pp. 1263–1268. doi: 10.1109/ICAIS56108.2023.10073740.
- Mapila MATJ, Njuki J, Delve RJ, Zingore S, Matibini J. 2012. Determinants of fertiliser use by smallholder maize farmers in the Chinyanja Triangle in Malawi, Mozambique and Zambia. Agric Econ Res Policy Pract Southern Afr. 51(1):21–41. doi: 10.1080/03031853.2012.649534.
- Marfo-Ahenkora E. 2020. Strategies for sustainable productivity of maize (Zea mays L.) - based farming systems of smallholder farmers in Ghana. University of Cape Coast. Available at: http://ir.ucc.edu.gh/jspui/handle/123456789/7197. (Accessed: 28 February 2023).
- Martey E, Etwire PM, Wossen T, Menkir A, Abdoulaye T. 2022. Impact assessment of Striga resistant maize varieties and fertilizer use in Ghana: a panel analysis. Food Energy Secur. 12(2):e432. doi: 10.1002/fes3.432.
- McCarthy N, Kilic T, Brubaker J, Murray S, de la Fuente A. 2021. Droughts and floods in Malawi: impacts on crop production and the performance of sustainable land management practices under weather extremes. Environ Dev Econ. 26(5-6):432–449. doi: 10.1017/S1355770X20000455.
- Meng L, Liu H, Ustin SL, Zhang X. 2021. Predicting maize yield at the plot scale of different fertilizer systems by multi-source data and machine learning methods. Remote Sens. 13(18):3760. doi: 10.3390/rs13183760.
- Meyer H, Reudenbach C, Hengl T, Katurji M, Nauss T. 2018. Improving performance of spatio-temporal machine learning models using forward feature selection and target-oriented validation. Environ Model Softw. 101:1–9. doi: 10.1016/j.envsoft.2017.12.001.
- Meyer H, Reudenbach C, Wöllauer S, Nauss T. 2019. Importance of spatial predictor variable selection in machine learning applications – moving from data reproduction to spatial prediction. Ecol Modell. 411:108815. doi: 10.1016/j.ecolmodel.2019.108815.
- Meyer H, Pebesma E. 2021. Predicting into unknown space? Estimating the area of applicability of spatial prediction models. Methods Ecol Evol. 12(9):1620–1633. doi: 10.1111/2041-210X.13650.
- Msowoya K, Madani K, Davtalab R, Mirchi A, Lund JR. 2016. Climate change impacts on maize production in the warm heart of Africa. Water Resour Manage. 30(14):5299–5312. doi: 10.1007/S11269-016-1487-3/FIGURES/8.
- Muthoni F. 2021. Machine learning model accurately predict maize grain yields in conservation agriculture systems in Southern Africa. 2021 9th International Conference on Agro-Geoinformatics, Agro-Geoinformatics 2021. doi: 10.1109/AGRO-GEOINFORMATICS50104.2021.9530335.
- Nagoshi RN, Koffi D, Agboka K, Adjevi AKM, Meagher RL, Goergen G.,. 2021. The fall armyworm strain associated with most rice, millet, and pasture infestations in the Western Hemisphere is rare or absent in Ghana and Togo. PLoS One. 16(6):e0253528. doi: 10.1371/JOURNAL.PONE.0253528.
- Ngcamu BS, Chari F. 2020. Drought influences on food insecurity in Africa: a systematic literature review. IJERPH. 17(16):5897. doi: 10.3390/ijerph17165897.
- Niassy S, Agbodzavu MK, Mudereri BT, Kamalongo D, Ligowe I, Hailu G, Kimathi E, Jere Z, Ochatum N, Pittchar J, et al. 2022. Performance of push-pull technology in low-fertility soils under conventional and conservation agriculture farming systems in Malawi. Sustainability. 14(4):2162. doi: 10.3390/su14042162.
- Oluoch KO, De Groote H, Gitonga ZM, Jin Z, Davis KF. 2022. A suite of agronomic factors can offset the effects of climate variability on rainfed maize production in Kenya. Sci Rep. 12(1):1–8. doi: 10.1038/s41598-022-19286-2.
- Parkes B, Sultan B, Ciais P. 2018. The impact of future climate change and potential adaptation methods on Maize yields in West Africa. Clim Change. 151(2):205–217. doi: 10.1007/S10584-018-2290-3/METRICS.
- R Core, Team,R: A language and environment for statistical computing [Internet], 2020, http://www.r-project.org/index.html (Accessed: 21 August 2020).
- Ragasa C, Chapoto A. 2017. Moving in the right direction? The role of price subsidies in fertilizer use and maize productivity in Ghana. Food Sec. 9(2):329–353. doi: 10.1007/S12571-017-0661-7/METRICS.
- Sauer J, Tchale H. 2009. The economics of soil fertility management in Malawi. Appl Econ Perspect Policy. 31(3):535–560. doi: 10.1111/j.1467-9353.2009.01452.x.
- Scheiterle L, Häring V, Birner R, Bosch C. 2019. Soil, striga, or subsidies? Determinants of maize productivity in Northern Ghana. Agric Econ. 50(4):479–494. doi: 10.1111/agec.12504.
- Scheiterle L, Birner R. 2018. Assessment of Ghana’s comparative ddvantage in maize production and the role of fertilizers. Sustainability. 10(11):4181. doi: 10.3390/su10114181.
- Setsoafia ED, Ma W, Renwick A. 2022. Effects of sustainable agricultural practices on farm income and food security in Northern Ghana. Agric Econ. 10(1):1–15. doi: 10.1186/S40100-022-00216-9/TABLES/4.
- Shi W, Tao F. 2014. Vulnerability of African maize yield to climate change and variability during 1961-2010. Food Sec. 6(4):471–481. doi: 10.1007/S12571-014-0370-4/METRICS.
- Silberg TR, Richardson RB, Hockett M, Snapp SS. 2017. Sustainability maize-legume intercropping in central Malawi: determinants of practice. Int J Agric. 15(6):662–680. doi: 10.1080/14735903.2017.1375070.
- Snapp S, et al. 2014. Maize yield response to nitrogen in Malawi’s smallholder production systems, MaSSP. 9. Washington, DC. Available at: http://ebrary.ifpri.org/cdm/ref/collection/p15738coll2/id/128436. (Accessed: 20 February 2023).
- Srivastava AK, Mboh CM, Gaiser T, Ewert F. 2017. Impact of climatic variables on the spatial and temporal variability of crop yield and biomass gap in Sub-Saharan Africa- a case study in Central Ghana. Field Crops Research. 203:33–46. doi: 10.1016/j.fcr.2016.11.010.
- Tamene L, Mponela P, Ndengu G, Kihara J. 2016. Assessment of maize yield gap and major determinant factors between smallholder farmers in the Dedza district of Malawi. Nutr Cycl Agroecosyst. 105(3):291–308. doi: 10.1007/s10705-015-9692-7.
- Tinonin C, et al. 2016. Africa RISING Baseline Evaluation Survey (ARBES) report for Ghana. International Food Policy Research Institute. Available at: https://cgspace.cgiar.org/handle/10568/75528. (Accessed: 8 March 2023).
- Tittonell P, Giller KE. 2013. When yield gaps are poverty traps: the paradigm of ecological intensification in African smallholder agriculture. Field Crops Res. 143:76–90. doi: 10.1016/j.fcr.2012.10.007.
- Vergopolan N, Xiong S, Estes L, Wanders N, Chaney NW, Wood EF, Konar M, Caylor K, Beck HE, Gatti N, et al. 2021. Field-scale soil moisture bridges the spatial-scale gap between drought monitoring and agricultural yields. Hydrol Earth Syst Sci. 25(4):1827–1847. doi: 10.5194/hess-25-1827-2021.
- Vuntade D, Mzuza MK, Vuntade D, Mzuza MK. 2022. Factors affecting adoption of Conservation Agriculture practices in Mpatsa extension planning area, Nsanje, Southern Malawi. GEP. 10(03):96–110. doi: 10.4236/gep.2022.103008.
- Wang H, Snapp SS, Fisher M, Viens F. 2019. A Bayesian analysis of longitudinal farm surveys in Central Malawi reveals yield determinants and site-specific management strategies. PLoS One. 14(8):e0219296. doi: 10.1371/journal.pone.0219296.
- Warnatzsch EA, Reay DS, Camardo Leggieri M, Battilani P. 2020. Climate change impact on aflatoxin contamination risk in Malawi’s maize crops. Front Sustain Food Syst. 4:238. doi: 10.3389/FSUFS.2020.591792/BIBTEX.
- Warnatzsch EA, Reay DS. 2020. Assessing climate change projections and impacts on Central Malawi’s maize yield: the risk of maladaptation. Sci Total Environ. 711:134845. doi: 10.1016/j.scitotenv.2019.134845.
- De Weerdt J, Duchoslav J. 2022. Are fertilizer subsidies in Malawi value for money? doi: 10.2499/P15738COLL2.135960.
- White S. 2019. A TEEBAgriFood analysis of the Malawi maize agri-food system. Available at: https://futureoffood.org/wp-content/uploads/2021/01/GA_TEEB_MalawiMaize201903.pdf. (Accessed: 20 February 2023).
- Yeboah S, Ennin SA, Ibrahim A, Oteng-Darko P, Mutyambai D, Khan ZR, Mochiah MB, Ekesi S, Niassy S. 2021. Effect of spatial arrangement of push-pull companion plants on fall armyworm control and agronomic performance of two maize varieties in Ghana. Crop Prot. 145:105612. doi: 10.1016/j.cropro.2021.105612.
- Zhang L, Zhang Z, Luo Y, Cao J, Tao F. 2019. Combining optical, fluorescence, thermal satellite, and environmental data to predict county-level maize yield in China using machine learning approaches. Remote Sens. 12(1):21. doi: 10.3390/rs12010021.