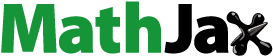
ABSTRACT
Reducing rigidity in labor markets is key to lowering unemployment. Theoretical models suggest that the impact of such reforms depends on the country-specific regulatory framework. We test this hypothesis by estimating the impact of changes in six categories of regulation conditional on the country-specific regulatory environment for 26 OECD countries. We overcome problems of modeling a large set of institutional interdependencies by applying a machine learning type model selection approach. We provide evidence for the existence of higher-order institutional interdependencies. We further document that especially for changes in employment protection and the unemployment benefit system the impact on unemployment is mixed across countries.
1. Introduction
The recent economic crisis has resulted in a dramatic deterioration of economic growth and labor market performance in various industrialized countries. There is a widespread view that economies under pressure associated with high unemployment or low employment rates need to change their labor market institutional environment. This needs to happen by conducting structural labor market reforms in order to improve labor market performance by, for instance, facilitating job reallocation processes or increasing labor market flexibility. Despite a plethora of contributions about the link between labor market institutions and labor market performance, evidence on the impact of labor market rigidity on labor market performance, which take the country-specific institutional framework and potential institutional interactions into account, is still scarce.
Theoretical and empirical evidence suggests that an institutional reform which is successful in one country might not be equally successful in another economy.Footnote1 It is likely that institutions do not work in isolation. The impact of a reform which changes the level of institutional rigidity is likely to depend on the entire labor market institutional environment. This is in line with the rising doubt about best practice solutions across EU member states.
The Danish flexicurity system is a good example. Andersen and Svarer (Citation2007) point out that the relatively low unemployment rate in Denmark since mid-1990 is due to a labor market reform which complements pre-existing low employment protection and generous unemployment benefits with a newly introduced active labor market policy. Caused by this reform, labor is allocated more efficiently through the combination of low employment protection, a suitable safety net (high replacement rates) and adequate activation measures to avoid the loss of job-specific networks and human capital. According to this, labor market institutions have to be matched to each other to work well. While reducing employment protection is likely to result in lower unemployment in countries characterized by a generous unemployment benefit system and pronounced active labor market policies, the reform could have no or even a contradictory effect in countries with low unemployment benefit levels and/or less efforts to bring people back to work.
So far, most empirical macroeconomic studies do not adequately take interdependencies between institutions into account but focus instead on isolated institutional effects or on few subjectively selected interdependencies. Theoretical contributions either focus on a single specific interdependency or deal with a broad but imprecise characterization of interactions. Having a rather limited theoretical foundation in combination with an insufficient number of observations is the main reason for many empirical studies disregarding institutional interactions from a macroeconomic perspective.
This paper aims at closing this gap by introducing model selection methods which are innovative in this literature, namely machine learning type optimization procedures, in order to comprehensively take the impact of institutional interdependencies on labor market performance into account. To be specific, we follow the general theoretical model of Belot and van Ours (Citation2004) in order to select institutional factors which are expected to have (interdependent) effects on the labor market. These include employment protection, unemployment benefits, labor taxes, union density, bargaining coordination, and product market regulation. We then specify a dynamic empirical panel model for 26 OECD countries which explains unemployment, our preferred measure of labor market performance, by institutions, interdependencies between institutions, and a set of control factors. This approach first sheds light on the empirical relevance of institutional interdependencies. Second, the model is able to provide evidence on the substitutability or complementarity of certain institutions. Third, it can be used as a basis for the identification of institutional effects on unemployment. The main advantage of this approach over the previous literature is the comprehensive consideration of interdependencies between institutions by allowing for higher-order interactions without restricting the model space on subjective grounds. Fourth, we focus on OECD participants to benefit from a broader data set that can be taken into account instead of obtaining information on institutional factors at the country-level only.Footnote2
Our results suggest that there are especially substantial quantitative, but also qualitative differences across countries in the labor market impact of institutional changes for nearly all selected institutional indicators. The impact of a change of bargaining power, employment protection, unemployment benefits, labor taxes, and bargaining coordination crucially depends on the country-specific institutional setting. In particular, we show that both complementary and substitutive relationships matter, and that institutional factors interact in a complex way. Thus, we provide evidence for the existence of higher-order institutional interdependencies. These findings provide novel insights for model builders, who have only partially considered interdependencies in theoretical models so far. We further document that especially for changes in employment protection and the unemployment benefit system the impact on unemployment is mixed across countries, thus questioning the relevance of best-practice policies.
The paper is organized as follows. Section 2 provides an overview of existing approaches to identify theoretically and estimate empirically labor market effects of interdependent labor market institutions. Section 3 presents the empirical model specification employed in this paper, while Section 4 introduces the corresponding model selection techniques. Data issues are described in Section 5, results are presented in Section 6, and Section 7 concludes.
2. Literature review
Over the last 20 years, a plethora of empirical contributions sought to identify the direct impact of institutions on unemployment or employment rates. Earlier studies come to the conclusion that rigid labor markets are responsible for weak labor market performance through, for instance, strict employment protection, high union bargaining power, a considerable labor tax burden, or generous unemployment benefit systems.Footnote3 More recent studies benefit from developments in data quality and estimation methods which improve the reliability of the results and question the orthodox view that rigid institutions lead to undesirable labor market outcomes.Footnote4
Yet, the aforementioned studies ignore the institutional environment, hence, the country-specific institutional set-up, of a country as a whole. Belot and van Ours (Citation2004) and Coe and Snower (Citation1997) argue that the same institutional reform does not necessarily need to have the same labor market impact in different countries. A reform might have a distinct effect depending on other country-specific institutional aspects.
The idea of institutional interdependencies has been taken up in some empirical studies differing in terms of which interdependencies are included, see Belot and van Ours (Citation2004), Nickell, Nunziata, and Ochel (Citation2005), Baccaro and Rei (Citation2007), and Bassanini and Duval (Citation2009). In particular, Bassanini and Duval (Citation2009) apply a more comprehensive approach by estimating all possible bivariate interactions between six institutional factors jointly, and by further applying an instrumental variable estimator. They take an important step forward by reducing the risk of an omitted variable bias.
The problem of being faced with an insufficient number of observations is tackled in Sachs (Citation2015) by the application of a Bayesian model averaging approach. The outcomes highlight the importance of institutional interdependencies for a country's labor market performance in the long-run. However, neither higher-order interactions nor a dynamic specification are considered in that contribution.
Instead of constructing interaction terms between individual institutions, Bassanini and Duval (Citation2009) additionally analyse the interaction between a particular institution and the country-specific institutional framework as a whole. This is done by estimating a specification in which the overall country-specific institutional framework is defined by the sum of direct unemployment effects of institutions. In other words, the authors claim that the sum of the estimated marginal effects of institutions approximate the level of regulation. Indeed, results produced with such a model suggest that the impact of an institutional change on unemployment depends on the aggregate institutional setting. The less rigid the overall institutional framework, the more successful are deregulating institutional reforms. According to this, institutions seem to be complementary, i.e. jointly reducing regulation is successful; a finding which is also put forward by Coe and Snower (Citation1997). While this approach is clearly appealing, it suffers from aggregating estimated individual institutional effects on unemployment. This might be seen as a weak proxy for the country-specific regulatory framework. Therefore, we see the approach of Bassanini and Duval (Citation2009) as a first step we can build on by applying a more disaggregated and structural approach.
While both aforementioned studies take a large set of bivariate interactions into account, higher-order interactions are completely neglected. Recalling the example of the successful Danish flexicurity system, such higher-order interactions between more than two institutions can be highly relevant and existing empirical models including merely bivariate interactions might provide only limited evidence. But higher-order interactions also matter from a technical point of view. According to Braumoeller (Citation2004), once a model with two interactions and
is specified, the interactions between
and
must be taken into account as well. Neglecting the trivariate interaction term is equivalent to assuming that the coefficients of the bivariate interaction terms
and
measure the impact on unemployment given that the respective third variable is zero. For instance,
gives the impact of
on unemployment conditional on the level of
and given that
. If this assumption does not hold and the trivariate interaction is a significant explanatory factor, estimates are biased. It is therefore necessary to additionally include
irrespective of its economic relevance, but for pure technical reasons.
3. Empirical specification
Our study seeks to first identify relevant institutional interactions for a given set of institutional factors and second to determine the country-specific marginal effects of institutional changes on the labor market by taking the country-specific institutional environment into account. The machine learning type model selection approach applied in this study is used to generate reliable empirical results. Yet, some theoretical considerations are necessary to define the set of potentially interacting institutions.
To do so, the ‘right-to-manage’ model of Belot and van Ours (Citation2004) is taken as the theoretical basis for the following empirical exercise. In this model, the unemployment benefit system, the labor tax system, employment protection, bargaining coordination, union bargaining power, and product market regulation can theoretically have an interdependent impact in the labor market by affecting the levels and the elasticities of labor supply and demand. More precisely, the labor market impact of a reform that changes the level and/or the elasticity of labor supply depends on the level and the elasticity of labor demand and vice versa. Hence, the labor market effect of a change of an institution can depend on one or more institutions. From a model perspective, both a complementary and a substitutive relationship between institutions is feasible.
The six institutional factors of Belot and van Ours (Citation2004) add up to a set of 63 variables (six individual institutional indicators plus 57 bivariate and higher-order interaction terms) which might be relevant explanatory factors. While product market regulation is not a labor market institution in classical terms, we include it for the following reasons. The literature identified it both as an important conditioning factor for the impact of labor market institutions, and also as a relevant determinant for unemployment itself.
Most econometric studies focusing on the link between labor market performance and institutions use static models. Fiori, Nicoletti, Scarpetta, and Schiantarelli (Citation2012) argue that a static model is only reasonable if a cointegration relationship between unemployment and the institutional factors is given. Furthermore, as pointed out by Nickell et al. (Citation2005) unemployment is probably exposed to some degree of endogenous persistence. Thus, explanatory factors may have an influence on unemployment which lasts longer than one year. A static model would not be able to adequately capture this kind of persistence and reform recommendations would be inadequate. Finally, neglecting the lagged dependent variable from the model can lead to endogeneity due to an omitted variable bias. Hence, allowing for a dynamic model structure to some extent controls for endogeneity. Following these arguments, we specify a dynamic model which entails that marginal institutional effects explain a short-term movement of the unemployment rate, since long-term adjustments are captured by the lagged dependent variable.
Accordingly, the model explaining labor market performance reads
(1)
(1) The dependent variable
is given by the unemployment rate, i refers to the country and t to the time dimension.
is the lagged dependent variable,
is a
vector containing the level indicators of institutional factors,
is a
vector of bivariate and higher-order multiplicative interaction terms between the six variables,
is a
matrix of control variables, δ is a scalar, and β, θ and γ are the corresponding coefficient vectors.
The error term is specified as
(2)
(2) Here,
is the country-specific effect which captures time-invariant unobservable determinants of unemployment. Such unobservables could comprise cultural or social differences across countries like the attitude to work.
is the time-specific effect which takes global events affecting all countries equally into account. An example for this might be a global recession which has an impact on the labor markets of all countries.
In general, we are interested in the coefficient vectors β and θ. The marginal effect of an institution can be derived from these vectors. The coefficients contained in θ additionally identify whether a specific interaction is complementary or substitutive. A positive sign indicates a substitutive relationship, while a negative sign implies that the interacting institutions are complements.
Estimating a dynamic two-way error component panel data model with fixed effects can yield biased and inconsistent estimates since the lagged dependent variable is correlated with which is part of the error term (see Baltagi, Citation2003). Especially for a short time-series, the error could be large. A solution to this is the application of the Arellano and Bond (Citation1991) difference GMM estimator. This estimator transforms the model in differences and uses lagged levels of the dependent variable as instruments. While this estimator performs well when the instruments are appropriate, it is biased for weak instruments. Thus, Blundell and Bond (Citation1998) suggested additional moment restrictions by setting up a system GMM estimator. Here, the system consists of the model equation in differences with lagged levels as instruments, and the model equation in levels with lagged differences as instruments. This estimator is theoretically suitable in particular for persistent dependent variables as they are in our set-up.
While the system GMM estimator is theoretically superior to a fixed effects estimator, the practical implementation is often hampered by weak instruments for both the difference and the level equation, however. This is true specifically when the country- and time dimensions are small and the time series are persistent (see Bun & Windmeijer, Citation2010). Hence, we first apply a simple fixed effects estimator and subsequently check the findings by applying the model selection method to the system GMM estimator.
As will be explained in the following section, a penalty is added to the target function in the model selection if serial correlation is detected. In doing so, we avoid to select models which are misspecified, indicated by serially correlated error terms. For the fixed effects estimator, we perform a recently developed LM-test for serial correlation which is especially adequate for panels with small T (Born & Breitung, Citation2014). Validity of the system GMM estimator requires the verification of the following three assumptions: validity of the instruments, covariance-stationarity of the endogenous variable, and no second order serial correlation in the residuals. The first assumption is tested by the Hansen test of overidentifying restrictions. The second assumption implies that the coefficient of the lagged dependent variable should converge to a steady-state and consequently be smaller than unity (Roodman, Citation2009). This can be tested with a Difference Sargan test of the full instrument set against the instrument set of the first-differenced GMM estimator (Blundell & Bond, Citation2000). Finally, there should be no second order serial correlation in the residuals. This assumption can be tested with the Arellano-Bond serial correlation test (Arellano & Bond, Citation1991). In principle, only if all three tests confirm the validity of the system GMM estimator, it should be applied.
4. Selecting interactions using machine learning optimization techniques
As theoretical models do not offer explicit guidance which institutional interdependencies are crucial for the well-functioning of the labor market, we seek to identify relevant institutional interactions empirically. Besides the ambiguity of the theory, a key methodological problem of model selection in a multiple regression when the relevant variables are not known a priori is the trade-off between consistency and efficiency. Taking a large number of regressors into account increases the variance, whereas including too few variables leads to inconsistent estimates. In our application, allowing for all possible interactions of k=6 institutional variables leaves us with models to be estimated. It becomes infeasible to estimate all potential combinations even when efficient methods are used (Gatu, Kontoghiorghes, Gilli, & Winker, Citation2008). There exist, however, several approaches providing an efficient way to obtain an optimal outcome by estimating only a subset of all potential models in the model selection literature.
Recently, Acosta-González and Fernández-Rodríguez (Citation2007), Kapetanios (Citation2007) and Savin and Winker (Citation2012) have shown that heuristic optimization techniques based on information criteria as objective function yield promising results in selecting regressors in different model selection set-ups of multiple regression models. Kapetanios (Citation2007) documents that Simulated Annealing (SA) and Genetic Algorithms (GA) as two classical heuristic methods perform better than PcGets. The closest to our approach is the model selection set-up by Savin and Winker (Citation2012) who apply heuristics to a dynamic panel model and identify a genetic algorithm as the best model selection strategy for this type of model. Consequently, we apply a genetic algorithm to select relevant institutional interactions.
Let us consider our empirical model explaining unemployment:
(3)
(3) Equation (Equation3
(3)
(3) ) is equal to Equation (Equation1
(1)
(1) ) except for factor ϕ. In other words,
denotes all candidate regression variables, that is all multiplicative interaction terms up to order six. ϕ is a
matrix of zero and ones on the diagonal, depending on which interactions are selected. As a constraint, the lagged dependent variable
, the levels of the institutional variables contained in
and the control factors
are forced to be included in each selected model.
Brambor, Clark, and Golder (Citation2006) point out that even if a constitutive termFootnote5 of an interaction is insignificant in statistical terms (applying the usual t-test) this is not sufficient to leave out this constitutive term from the equation. According to them, there are two conditions which should be fulfilled before a constitutive term can be discarded. First, '··· the analyst should estimate the fully specified model [··· ] and find that the [coefficient of the constitutive term] is zero' (p. 69). This is not possible in our application as we do not know our model structure in advance. Second, the researcher 'must have a strong theoretical expectation that the omitted variable [··· ] has no effect on the dependent variable in the absence of the other modifying variable[··· ]' (p. 68). As theory neither provides arguments for the exclusion nor for the inclusion of specific constitutive interaction terms, the second condition cannot be satisfied. Therefore, we a priori allow all constitutive terms to be potentially included in the model.
In line with Hendry and Krolzig (Citation2004) and Acosta-González and Fernández-Rodríguez (Citation2007), we check the general unrestricted model (GUM) for validity by assessing our model set-up carefully. As long as theoretical, data-measurement, and model specification considerations are conducted appropriately, the econometric set-up is not prone to the criticism of data-mining (Hendry & Krolzig, Citation2004). We tackle these issues thoroughly. As the theoretical literature is not particularly detailed about the link between unemployment and institutional interactions, we rely on the variables for which the selection is theoretically justified. Therefore, we select the potentially interacting institutional variables by taking the theoretical model of Belot and van Ours (Citation2004) as basis. Second, we use recently published panel data of institutional indicators which is superior to previously used data in order to have a reliable data basis. Finally, depending on the specific estimator, the model specification is evaluated either by performing tests of the final model or by directly incorporating the test decisions into the model selection algorithm.
Based on an objective function, we seek to identify the relevant institutional variables for explaining unemployment. We choose an information criterion as loss function in order to derive a high model fit by simultaneously penalizing for overparametrization. As the Bayesian Information Criterion (BIC) is consistent and appears to yield superior results in model selection as pointed out by Kapetanios (Citation2007) and Savin and Winker (Citation2012), we select the BIC as target function. To avoid model misspecifications, we add a penalty to the objective function if the selected model has serially correlated errors based on the test developed by Born and Breitung (Citation2014) for the fixed effects estimation. The test has a null hypothesis of ‘no serial correlation of order one’. The objective function f looks as follows:
(4)
(4) where
denotes the residual variance, m the number of estimated parameters. The penalty applies only if there is serial correlation in the errors. If the null cannot be rejected, the penalty is zero:
The genetic algorithm – the preferred model selection approach – is presented in more detail.Footnote6 More details of the algorithm can be found in the Appendix.
5. Data
The analysis is based on a balanced data set with annual observations for 26 OECD countries from 2001 to 2008.Footnote7 It holds a set of six institutional variables, 57 institutional interactions, and four control factors. Summary statistics for the variables are given in the Appendix in Table . The estimation of a model with more than one interaction term is often blamed to suffer from multicollinearity. This is especially a risk if the constitutive terms, that is, the six institutional indicators, are highly correlated. For our data sample, multicollinearity issues seem to play a minor role as 0.5 is the strongest correlation observed between two individual institutions; most of of them are closer to zero or even negative. The complete correlation matrix is given in the Appendix in Table .
In principle, the fixed effects absorb all time-invariant influences on the dependent variable. Estimating the impact of rarely changing variables on unemployment is difficult in such a set-up. However, while employment protection or bargaining coordination do not change much over time, interacting these rarely changing variables provides substantial exogenous variation over time and enables the precise identification of interaction term coefficients. Thus, when applying a fixed-effects estimator, the problem of imprecise estimates through rarely changing explanatory factors over time is alleviated.
5.1. Labor market performance
Several indicators like the unemployment rate, the employment rate, joblessness, or inactivity can serve as a proxy for labor market performance. The most prominent indicator in econometric studies has been the unemployment rate. Nickell and Layard (Citation1999) argue that this is the best measure for labor market performance 'because it is probably the least voluntary' (p. 3031). The lower the unemployment rate is, the less persons are actively searching for a job and, consequently the better the state of the labor market is. The measurement of unemployment, however, also has some drawbacks. It is difficult to compare unemployment rates across countries since national concepts differ.Footnote8 In order to tackle this problem, the OECD constructs harmonized unemployment rates calculated according to international standards. These series are better suited for international comparisons of labor market performance than pure national numbers. Thus, our dependent variable is the harmonized unemployment rate provided by the OECD.Footnote9
5.2. Institutional variables
On the basis of the theoretical model of Belot and van Ours (Citation2004), six institutional factors are supposed to show an interdependent impact on unemployment.
The net replacement rate (NRR) for an average production worker averaged over different family situations is used as an indicator for the generosity of the unemployment benefit system. It measures the unemployment benefits as a percentage of the last job wage. Note that this indicator has recently been published by the OECD and represents a substantial improvement over the gross replacement rates. This latter factor has been used in nearly all previous studies focusing on institutions. While gross replacement rates relate income during unemployment to the gross last job wage, net replacement rates refer to net last job wages. Comparisons both across countries as well as over time are much more reliable by using the net indicator since differences or changes in the tax system do not have an effect on the replacement rate. Interestingly, Howell and Rehm (Citation2009) report only a small correlation between the OECD gross and net replacement rates. This emphasizes the importance of considering the net measure to take the unemployment benefit system adequately into account.
The labor tax system (LT) is approximated by the tax wedge delivered by the OECD. This indicator measures the amount of income taxes and social security contributions paid by the employee, and payroll taxes and social security contributions paid by the employer, as well as family benefits received by the employee for an average production worker for different family situations as a percentage of the total labor compensation.
Employment protection (EP) can be well described by an OECD indicator which comprises information from different dimensions of employment protection. The values of this indicator, a metric summary variable of various sub-categories of protection, can lie within the range from 0 to 6, and are increasing in the degree of protection.
The degree of coordination between employers and employees in the wage bargaining process gives the level of bargaining coordination (BC). It can take the values 1–5, where higher values indicate a higher degree of coordination. A value of 5 means economy-wide bargaining while a value of 1 expresses fragmented bargaining which takes place mostly at the company level. Note that this measure includes both the formal and the informal dimension of coordination. Soskice (Citation1990) argues that bargaining centralization only measures the level at which bargaining takes place. Bargaining coordination, in turn, is a more general concept which comprises, besides bargaining centralization, other forms of centralization. Even if a country exhibits rather decentralized bargaining, coordination could be high with a considerable impact on the wage bargaining process. Soskice (Citation1990) takes Switzerland and Japan, both with highly decentralized, company-level bargaining, as examples to illustrate such distinctions between centralization and coordination measures. Coordination comes in through higher-level employer organizations in Switzerland and informal wage cartels in Japan.
For union bargaining power, the union density (UD), which measures the percentage of employees organized in unions in relation to all employees, is provided by Visser (Citation2011), and is our preferred indicator. Recently, the union coverage has gained importance as an indicator for union bargaining power since it covers not only all employees organized in unions but all employees affected by union wage agreements. This indicator cannot be considered here due to incomplete coverage over time. Nevertheless, the union coverage is often close to the union density values which is why we consider it as an appropriate indicator for bargaining power.
An indicator for product market regulation (PMR) is available from the Fraser Institute which publishes the Economic Freedom of the World Index (Gwartney, Lawson, & Hall, Citation2012). This measure lies in the range from 0 to 10 and comprises information on business regulations stemming from seven sources: price controls, administrative requirements, bureaucracy costs, starting a business, extra payments/bribes/favoritism, licensing restrictions, and cost of tax compliance. In the original data source, the value is decreasing in the degree of regulation. In order to ensure that deregulation is linked to decreasing indicator values, we multiply the product market regulation value with .
5.3. Control factors
A set of macroeconomic variables which have been proven to be relevant drivers of labor market performance in the literature is taken into account. Similar to Fiori et al. (Citation2012), the output gap, which gives the percentage deviation of actual from trend output, is used to control for cyclical fluctuations in (un)employment. Trend growth is calculated on the basis of a production function. Following Amable, Demmou, and Gatti (Citation2011), three additional factors, the first difference of the real exchange rate, the structural trade balance, and the average labor productivity are used as control factors. The data series are provided by the OECD via its Economic Outlook.Footnote10
6. Results
6.1. Model selection
The basic estimation approach applies the genetic algorithm as model selection tool in combination with a simple dynamic fixed effects estimator. Theoretically, system GMM is efficient but might be plagued by difficulties in determining the optimal instrumental variable set. We later run the model selection approach with the system GMM estimator as a robustness check.
The selected model based on the fixed effects estimation looks as follows:
(5)
(5) As explained in Section 3,
is a vector containing the individual institutional factors EP, NRR, LT, UD, BC and PMR and
represents a vector of control factors. The genetic algorithm identifies seven interaction terms as crucial determinants of unemployment in the fixed effects estimation. More specifically, bivariate interactions between employment protection (EP) and the net replacement rate (NRR), labor taxes (LT) and the net replacement rate, union density (UD) and the net replacement rate, union density and bargaining coordination (BC) are identified as significant. Furthermore, two trivariate interactions, first between employment protection, labor taxes and bargaining coordination, and second between the net replacement rate, bargaining coordination and union density also show an interdependent relationship which is relevant for unemployment. Finally, an interaction between four institutional factors, employment protection, the net replacement rate, labor taxes and bargaining coordination influences unemployment.
The following Table reports whether the institutions of a selected interaction term are complements or substitutes. A complement exists if the coefficient sign of the respective interaction term is negative, while a positive interaction term coefficient is associated with substitutive institutions. It is important to mention that the paper does not aim at explaining good or bad labor market policies, but rather wants to shed light on interacting institutions and their mode of action.
Table 1. Results of model selection.
Overall, 3 interaction terms have a negative sign and therefore exhibit a complementary structure, while 4 interaction terms consist of substitutive institutions. More concretely, employment protection and net replacement rates () as well as net replacement rates and labor taxes (
) are substitutes. Deregulating one of the factors is more beneficial for the labor market when the remaining factor is regulated. In contrast, net replacement rates and union density (
) as well as bargaining coordination and union density (
) are complements. Consequently, it is more beneficial to deregulate one institution when the other is already deregulated or to deregulate the institutions jointly. Considering the higher-order interaction terms complicates the interpretation, however. While net replacement rates and union density (
) are complements, net replacement rates and the product of union density and bargaining coordination (
) are substitutes. Similarly, employment protection and net replacement rates (
) are substitutes, but employment protection and the product of net replacement rates, labor taxes and bargaining coordination (
) are complements. Hence, in our context, we cannot derive from the sign of the interaction term whether jointly deregulating two or more factors is beneficial since there are various, sometimes countervailing, effects at work. We can, however, conclude that both complementary and substitutive relations exist. Additionally, the signs will facilitate the interpretation of marginal effects. This exercise will be carried out in the following subsection.
The model selection procedure selects all five labor market institutions in at least one interaction term. Only the product market regulation variable does not interact with another institutional factor. In other words, the impact of a change in any of the five labor market institutions on unemployment does not depend on the level of product market regulation. Additionally, the impact of a change in product market regulation on unemployment is independent of the level of regulation of the five labor market institutions. This is contrary to recent evidence on an interdependent relation between labor and product market regulation on the labor market (Fiori et al., Citation2012). The interaction between product and labor market regulation documented in the literature could be driven by an omitted variable bias due to neglecting further relevant higher-order interactions. Alternatively, we cannot rule out that there simply has not been an interdependent relationship between labor and product market regulation for the specific country sample and time period in this study. The insignificant coefficient of product market regulation points toward this direction (see Table ).
In summary, there is a conditioning effect stemming from other institutions for a change in the five institutional factors. This highlights the importance of interdependent institutional influences on the labor market and the need for considering the country-specific institutional set-up when conducting labor market reforms. Furthermore, the appearance of higher-order interaction terms in the final model emphasizes the complexity of the interplay between different institutional factors. This is of particular relevance for theoretical model builders who should not restrict their models to bivariate or subjectively selected interactions.
6.2. Marginal institutional effects
While the statistical identification of relevant interaction terms can be reliably done with the model selection approach applied in this paper, a further step is needed to provide a more elaborate economic interpretation. This is done by calculating marginal effects of the institutional factors. Assume the following model where the dependent variable y is explained by three factors ,
, and
and all possible interactions between these factors:
(6)
(6) The marginal effect of a factor
on y is then calculated as the first derivative of y with respect to
(7)
(7) Hence, the marginal effect of
on y depends on the estimated coefficients as well as on the level of
and
for a specific cross-section and a specific point in time.Footnote11 Based on this example, marginal effects can be calculated for all countries in our sample for a given year. The marginal effect then shows what would happen to the unemployment rate if the value of an institutional indicator changes. A positive (negative) marginal effect means that deregulation would lower (raise) unemployment. To interpret our findings, we calculate the marginal effects for the institutional level in 2008 since this is the latest available observation.
Following Greene (Citation2003, p.124), we also calculate the standard errors for the marginal effects. This is more complex than for estimates without conditioning variables since we have to take into account covariances between interacting factors. More specifically, for the model given above, the standard error for the marginal effect of for instance, is given by
(8)
(8)
This standard error provides information on the precision of estimating the marginal effect for given values of the conditioning factors (,
and
in the example given above). Hence, similar to the marginal effect, the standard errors depend on the country-specific institutional framework and can be calculated for each country separately.
In the following Table , we present results of the marginal effects. Deregulating reforms of labor taxes, product market regulation, bargaining coordination and union density have the potential to reduce unemployment in the majority of countries. Most of the marginal effects of these institutions are positive. Moreover, lowering taxation of labor reduces unemployment in 19 countries out of 26 of the sample, while lowering the workers' bargaining power through decreasing union density as well as increasing competition in the product market would be successful in all 26 countries. In contrast, reducing the level of employment protection and the replacement rate level would have detrimental labor market effects in the majority of countries.
Table 2. Marginal institutional effects (fixed effects).
Next, we compare labor market effects through institutional changes across countries. We investigate whether certain regulatory environments explain the success of specific reforms. Thereby, we focus on employment protection, the replacement rate, and labor taxes since these factors show largely heterogeneous marginal effects across countries. First, a ranking is constructed which allows to classify countries according to their level of regulation. More specifically, we rank all 26 countries regarding their level of regulation for the six institutional factors, which are employment protection, net replacement rates, union density, bargaining coordination, labor taxes and product market regulation. Then, we sum up the individual ranks per country and construct an overall ranking of institutional regulation. The lower the indicator value is, the better the rank and the less regulated the labor market is. According to this procedure, the United States is ranked first indicating a low degree of regulation, while Belgium is at the bottom of the ranking. The complete ranking is shown in Table .
Table 3. Ranking of institutional regulation.
Table 4. Detailed marginal institutional effects.
Additionally, Table provides the full list of marginal effects for all countries and each indicator. The countries are listed according to the ranking of Table . Hence, the first country is the least regulated, while the last shows the highest degree of regulation. The marginal effects in Table reveal that mostly Central and Southern European countries have the potential to improve labor market performance by deregulating job protection. Switzerland, Austria, Portugal, Italy, Germany, Greece and Belgium show positive marginal effects for EP. The interconnectedness of institutions can help to explain why especially these countries would benefit from deregulating job protection. Employment protection has a substitutive relationship with the net replacement rate, as well as with the product of labor taxes and bargaining coordination (see Table ). This indicates that reducing employment protection would be more beneficial when the indicator values for the net replacement rate and the product of labor taxes and bargaining coordination are high. However, job protection has also a complementary relation to the product of the net replacement rate, labor taxes and bargaining coordination. Consequently, reducing job protection is more beneficial when the product of the indicator values of net replacement rate, labor taxes and bargaining coordination is low. Which of these countervailing effects prevails depends on the specific magnitude of a regulation and will be discussed in more detail in the following.
Simulations by means of different indicator values of the net replacement rate, the labor taxes and the bargaining coordination show that there are two regulatory environments which particularly promote a successful employment protection deregulation: First, if the net replacement rate is low and the product of labor taxes and bargaining coordination is high; second, if the net replacement rate is high and the product of labor taxes and bargaining coordination is low.
In line with this, a positive marginal effect of employment protection can be observed for two regulatory environments: First, in countries showing a combination of a rather deregulated unemployment benefit system (low replacement rate) and high labor taxes in combination with coordinated wage bargaining (Austria, Germany, Greece, Italy and Belgium); and second, in countries with generous unemployment benefits and low labor taxes in combination with uncoordinated bargaining (Switzerland and Portugal, see Tables and ). Stimulating labor demand through a reduction in job protection should be combined with sufficient labor supply (caused by a low replacement rate) or with a tax system that does not expand hiring costs. Combining reduced job protection with a low replacement rate, limited taxes on labor and uncoordinated bargaining is, however, not a promising way to go. We assume that in this case the firms' market power is above a certain limit which allows sensible wage setting.
A further implication evolves from this finding. While reducing job protection is beneficial in the previously described environments, countries could even amplify the reform effect by jointly reforming specific interconnected institutions. We explain this using Belgium as an example. In 2008, Belgium is ranked 7th regarding its net replacement rate, and 26th and 25th regarding labor taxes and bargaining coordination, respectively. A reduction in the strictness of job protection by one indicator point would be linked to a drop in unemployment by approximately 1.2 percentage points (see the marginal effect of job protection for Belgium). Since such a reform is particularly successful in regulatory environments with a low replacement rate in combination with high taxes and coordinated bargaining, Belgium could benefit from a joint reduction of job protection and the replacement rate. A replacement rate which would be decreased by five percentage points compared to the value 2008 in combination with the 2008 status quo of labor taxes and bargaining coordination would amplify a deregulatory job protection reform. More specifically, jointly reducing the replacement rate by five percentage points and job protection by one indicator point would lead to an unemployment rate which is 1.9 percentage points lower.
Our results suggest furthermore that a replacement rate reduction would be promising in Eastern European economies. Positive marginal effects are reported for Poland, the Czech Republic, Hungary and Slovakia. According to the model selection procedure, replacement rates have a substitutive relation with labor taxes, employment protection, and the product of bargaining coordination and union density, as well as a complementary relation with union density and the product of employment protection, labor taxes and bargaining coordination. This complex network of relationships makes it difficult to pin down which combination of regulations are best for reforming the unemployment benefit system. It is, however, striking that the Eastern European economies have low union participation rates in combination with an uncoordinated bargaining, moderate labor taxes, and a rather flexible job protection.Footnote12 Nevertheless, other countries with positive marginal effects regarding replacement rates exhibit heterogeneous regulatory systems. Hence, there are different specific labor market environments which promote a successful deregulatory reform of the unemployment benefits system.
Finally, a deregulating reform of the tax system would be beneficial in most countries, but not in the least regulated Anglo-Saxon economies. Our estimations show that a reduction in labor taxes would increase unemployment in the United States, New Zealand, Australia, the United Kingdom and Canada. Additionally, Poland and Portugal would also suffer from such a reform. Labor taxes are substitutively linked to net replacement rates and to the product of employment protection and bargaining coordination. Furthermore, labor taxes have a complementary relation with the product of employment protection, net replacement rates and bargaining coordination. Similar to a reform deregulating job protection, reducing taxes on labor is especially beneficial for two regulatory environments. First, countries benefit from a tax reduction if they have a generous unemployment benefit system and a combination of loose job protection and uncoordinated bargaining. Second, economies in which the replacement rate is low, but job protection is strict and bargaining is coordinated benefit from a tax reduction.
Given these combinations we conclude that a tax reduction which is most likely associated with a rise in labor demand, should be carried out in a regulatory environment which allows a sufficient level of labor supply and, at the same time, protects to some extent against labor market risk (becoming unemployed). In other words, stimulated labor demand should be matched by sufficient labor supply. This can be achieved by restricting unemployment benefits, for instance. However, limited financial governmental support for unemployed increases labor market risk. Workers run the risk of substantial income losses when becoming unemployed. Strict job protection can to some extent counterbalance this effect by providing job security and by reducing labor market risk for workers.Footnote13 Strict job protection additionally favors incumbents over job seekers. A wage-setting behavior of incumbents neglecting the interests of job seekers is likely. Our findings indicate that coordinated wage bargaining avoids such a detrimental wage setting behavior associated with excessive wage claims and dampened labor demand, thus balancing interests of employers, employees and job seekers. In the following, we will have a closer look at marginal effects of labor taxes by giving an example.
In 2006, New Zealand and the Netherlands carried out reforms which reduced the tax wedge level considerably. More concretely, the OECD tax wedge indicator dropped by approximately 4 percentage points in New Zealand and the Netherlands. At first sight, one would assume that the same reform leads to the same outcome. However, from 2006 to 2008, unemployment increased slightly in New Zealand, and decreased substantially in the Netherlands. According to the findings of our paper, this difference stems, to some extent, from heterogeneous regulatory structures in both countries. Differences in the level of employment protection, the generosity of the unemployment benefit system and the wage bargaining system are assumed to be responsible for the heterogeneous impact on unemployment. More concisely, a generous unemployment benefit system in combination with a moderately coordinated wage bargaining system and relatively low job protection has likely led to the beneficial impact of a tax cut in the Netherlands. In contrast, uncoordinated bargaining, low job protection as well as a low replacement rate in New Zealand suggest that the potential benefits of a tax reduction are absorbed by an environment which is unable to create additional employment. Missing insurance of unemployed caused by low benefits as well as high transition rates into and out of unemployment through a lack of job protection seem to influence the detrimental labor market impact of the tax cut.
Heterogeneous regulatory structures not only lead to different reform effects in statistical, but also in economic terms. In Table , which is based on 2008 values, we can see that a reduction in the employment protection indicator (ranging from 0 to 6) by one point would change unemployment by between 4.7 and -1.2 percentage points depending on the country. A reduction in the net replacement rate by 1 percentage point would be related to an unemployment rate which is 0.26 percentage points lower in France, and around 0.26 percentage points higher in Canada. Similarly, marginal effects from a labor tax cut by one percentage point range from 0.12 percentage points in the United Kingdom to -0.15 in Greece. Even larger effects apply for changes in the bargaining power (proxied by union density). A reduction in union density by 1 percentage point is linked to a reduction in the unemployment rate between 0.13 and 0.41 percentage points.
A word on significance is appropriate at this point. Note that the standard errors refer to the reliability of the marginal effect given the indicator values for the conditioning factors. Hence, significance depends on the specific year for which marginal effects are calculated (2008 in our example). The estimated marginal effects are significant for several countries and reforms. In particular, the specific reform effects on unemployment discussed previously are statistically significant. However, especially marginal effects for labor taxes are plagued by high standard errors. This also affects the reform example from New Zealand and the Netherlands given above. Hence, some doubts remain caused by insignificant marginal effects.
6.3. Results of GMM estimation
The fixed effects estimates could be biased due to the correlation of the lagged dependent variable with the error term through the country-specific fixed effects. We therefore run the genetic algorithm with the theoretically efficient system GMM estimator. Overall, the findings are similar to the results produced with the fixed effects estimator. Seven interactions terms are still identified as relevant for explaining unemployment. However, the interactions between employment protection and the replacement rate as well as between the replacement rate and labor taxes are not selected by means of the GMM estimation. In contrast, the interactions between employment protection and labor taxes as well as between employment protection, the replacement rate and labor taxes are chosen by the genetic algorithm. These changes in the selected model result in a slightly different pattern of positive marginal effects across countries. The number of positive marginal effects, illustrated in the following Table , increases for employment protection from 7 to 14, and for labor taxes from 19 to 20, while it decreases for the replacement rate from 9 to 8. The remaining numbers do not change.
Table 5. Marginal institutional effects (GMM).
The superiority of the GMM over the fixed effects estimator, however, hinges essentially on assumptions which can be tested (see Section 3 for a brief discussion). Unfortunately, already the Hansen test for overidentifying restrictions of the first-step estimator performs rather dubious. The p-value of this test equals 1 for virtually all models. This is a well-documented shortcoming of the Hansen test in case of instrument proliferation (Roodman, Citation2009). Roodman suggests to apply the Hansen test to the GMM estimator with a collapsed instrument set. Doing this, the Hansen test still performs poorly, however. Furthermore, a comparison of the consistent one-step system GMM estimates and the asymptotically more efficient two-step estimates show that coefficients are highly unstable. We therefore have to assume that the selected instruments are invalid, and that the system GMM estimator does not provide reliable results for our model set-up. Therefore, we trust the fixed effects findings more. Consequently, we accept the consequences of the potential Nickell-bias -- potential correlation between explanatory variables and the residuals -- due to the dynamic structure of our model. Nevertheless, the similarity for the fixed-effects and the GMM estimation results suggests that the Nickell-bias may not be of central importance for our findings.
7. Conclusion
This paper analyses the impact of interdependencies between institutional factors for the evolution of unemployment. Based on an innovative model selection approach, which is combined with a classical dynamic fixed-effect estimator for a two-way error component model, higher-order institutional interdependencies are identified for a panel of 26 OECD countries ranging from 2001 to 2008. We do so by applying a machine learning type optimization method, namely a genetic algorithm, which has not been used in the unemployment-institution literature so far.
In contrast to the previous literature, this paper is the first to focus on the impact of higher-order institutional interactions on unemployment and one of the first to consider a dynamic model specification in the context of institutional interactions. It thereby enables a more precise and detailed analysis of the impact of interdependencies between different labor market institutions on labor market performance on a cross-country level.
The results suggest that there are substantial differences across countries in the labor market impact of institutional changes for nearly all selected institutional indicators. The impact of a reform of employment protection, unemployment benefits, labor taxes, bargaining power, and bargaining coordination crucially depends on the country-specific institutional setting.
In particular, reductions in labor taxes, bargaining power, product market regulation, and bargaining coordination seem to reduce unemployment in the majority of countries. In contrast, lowering employment protection and unemployment benefits are much less likely to have the trivially expected consequences that deregulation is the road to success, although such reforms would be beneficial in some countries.
To be specific, deregulating job protection is likely to be successful in two regulatory environments: first, in countries like Switzerland or Portugal which exhibit a generous unemployment benefit system in combination with rather low labor taxation and comparably uncoordinated wage bargaining; second, in countries with low or moderate unemployment benefits in combination with high labor taxes and coordinated bargaining, for instance Austria, Greece or Italy. Similarly, reducing taxes on labor is particularly beneficial for two regulatory environments: first, in countries with a generous unemployment benefit system and a combination of loose job protection and uncoordinated bargaining; second, in economies with low unemployment benefits, but strict job protection and coordinated bargaining. Such combinations are present in most countries in our sample except for the Anglo-Saxon economies; thus, their labor market would not benefit from lowering taxation.
It further stands out that five of the six institutional categories matter as conditioning factors. This is particularly relevant for theoretical model builders by providing, first, empirical evidence that institutions are linked in a more sophisticated way than considered before, and, second, empirical guidance which institutional factors should be included in such interdependencies.
Due to the lack of adequate instruments and the inability of the system GMM estimator to provide reliable results, no causal relationship between institutions and unemployment can be set up. Furthermore, while the selection of institutional factors is derived directly from theory, additional institutional factors like active labor market policies or family policies might influence the results.
Nevertheless, our findings provide robust evidence that (i) interdependencies are crucial for the labor market effect of an institutional reform, (ii) the order of interactions is rather high suggesting complex links between institutions, and (iii) reform recommendations should not be based on success stories from specific countries, but on an accurate evaluation of the country-specific institutional setting.
Having shown the importance of institutional interdependencies, future research could deal with interrelations of labor market institutions and reforms in different states of the economy. In particular, it would be worth to examine the aftermath of the financial and economic crisis, analyze the effects of the crisis, and compare effects of labor market reforms in different states of the economy, e.g. by means of a (Smooth Transition) Threshold Model.
Although results are valid for OECD, some main messages can be taken away also for developing countries. We expect that the country-specific set-up is crucial when assessing labor market reforms in developing countries as well. More precisely, implementing labor market reforms in developing countries is even more critical than in OECD countries. When conducting reforms, developing countries should focus on those reforms that promise to be most successful. To find critical institutions or reforms, it is essential to take also the country-specific set-up into account. A potential further step forward is perform analysis by using a different country set based on developing countries, in order to identify critical and most promising approaches to reform.
Acknowledgments
We are grateful to Leonhard Brinster, Lisardo Erman and, in particular, Daniel Maas for excellent research assistance. We are especially indebted to Peter Winker for helpful comments and suggestions. We also thank Jan Hogrefe for valuable comments. All remaining errors are our own.
Disclosure statement
No potential conflict of interest was reported by the authors.
Additional information
Funding
Notes on contributors
Andreas Sachs
Andreas Sachs is an Economic Advisor at Prognos AG. He obtained his PhD in Economics from Ulm University in 2014. He was a researcher at the Centre for European Economic Research.
Frauke Schleer
Frauke Schleer-van Gellecom is a Senior Manager at PricewaterhouseCoopers WGP GmbH. She obtained his PhD in Economics from Justus Liebig University of Giessen in 2014. She was a researcher at the Centre for European Economic Research.
Notes
1 See Coe and Snower (Citation1997) and Belot and van Ours (Citation2004) for a theoretical treatment, and Bassanini and Duval (Citation2009) and Sachs (Citation2015) for an empirical investigation of this topic.
2 When using only country-level data, we neglect important data points which we believe are important to receive a more powerful and accurate estimation results, especially when modeling a high number of interdependencies.
3 See, inter alia, OECD (Citation1994), Scarpetta (Citation1996), Elmeskov, Martin, and Scarpetta (Citation1998), Blanchard and Wolfers (Citation2000), IMF (Citation2003).
4 See Howell, Baker, Glyn, and Schmitt (Citation2007), Baccaro and Rei (Citation2007), Bassanini and Duval (Citation2009) and Sachs (Citation2012).
5 ,
,
,
,
and
are the constitutive terms of the interaction
.
6 We also checked a local search heuristics, the Threshold Accepting (TA) algorithm, as model selection technique. Since TA yields worse results in terms of model fit, we stick to the genetic algorithm.
7 We thank an anonymous referee for his or her comment to the sample period. We chose this sample period to explicitly exclude the influence of the financial and economic crisis and refer to the conclusion on a more extensive discussion.
8 The problem of comparability across countries applies to employment rates as well.
9 For Switzerland, no annual harmonized unemployment rate is available. However, the OECD reports the unemployment rate for the second quarter of a year. This value is taken to approximate the annual unemployment rate for Switzerland.
10 Interactions between institutions and controls and control variables may also display relevant interdependencies which cannot be examined within the scope of this paper. We thank an anonymous referee for this remark.
11 For simplification, we abstract from using subscripts for the cross-section and time in this example.
12 The Czech Republic is ranked 18th, but its indicator level is close to that of the remaining Eastern European countries.
13 See Arpaia and Mourre (Citation2012) on the impact of unemployment benefits and employment protection on labor market risk.
14 The size of the initial set of the generated model structure (population) should be sufficiently large to allow for diversification such that a broad range of the search space is covered. Yet, it should not be too large to efficiently search through the search space finding the best solution.
15 The number of generations () amounts to 500 (250,000 iterations divided by population size p=500) for each restart. We set the number of restarts to 10.
References
- Acosta-González E., & Fernández-Rodríguez F. (2007). Model selection via genetic algorithms illustrated with cross-country growth data. Empirical Economics, 33(2), 313–337.
- Amable B., Demmou L., & Gatti D. (2011). The effect of employment protection and product market regulation on labour market performance: Substitution or complementarity?. Applied Economics, 43(4), 449–464.
- Andersen T. M., & Svarer M. (2007). Flexicurity: Labour market performance in denmark. CESifo Economic Studies, 53(3), 389–429.
- Arellano M., & Bond S. (1991). Some tests of specification for panel data: Monte carlo evidence and an application to employment equations. Review of Economic Studies, 58(2), 277–97.
- Arpaia A., & Mourre G. (2012). Institutions and performance in european labour markets: Taking a fresh look at evidence. Journal of Economic Surveys, 26(1), 1–41.
- Baccaro L., & Rei D. (2007). Institutional determinants of unemployment in oecd countries: Does the deregulatory view hold water? International Organization, 61(03), 527–569.
- Baltagi, B. H. (2003). Econometric analysis of panel data (3rd ed.). West Sussex, England: Wiley.
- Bassanini A., & Duval R. (2009). Unemployment, institutions, and reform complementarities: re-assessing the aggregate evidence for oecd countries. Oxford Review of Economic Policy, 25(1), 40–59.
- Belot M., & van Ours J. C. (2004). Does the recent success of some oecd countries in lowering their unemployment rates lie in the clever design of their labor market reforms?. Oxford Economic Papers, 56(4), 621–642.
- Blanchard O., & Wolfers J. (2000). The role of shocks and institutions in the rise of european unemployment: The aggregate evidence. Economic Journal, 110(462), C1–C33.
- Blundell R., & Bond S. (1998). Initial conditions and moment restrictions in dynamic panel data models. Journal of Econometrics, 87(1), 115–143.
- Blundell R., & Bond S. (2000). Gmm estimation with persistent panel data: an application to production functions. Econometric Reviews, 19(3), 321–340.
- Born B., & Breitung J. (2014). Testing for serial correlation in fixed-effects panel data models. Econometric Reviews, forthcoming.
- Brambor T., Clark W., & Golder M. (2006). Understanding interaction models: Improving empirical analyses. Political Analysis, 14, 63–82.
- Braumoeller B. F. (2004). Hypothesis testing and multiplicative interaction terms. International Organization, 58(4), 807–820.
- Bun M. J. G., & Windmeijer F. (2010). The weak instrument problem of the system gmm estimator in dynamic panel data models. Econometrics Journal, 13(1), 95–126.
- Coe D. T., & Snower D. J. (1997). Policy complementarities: The case for fundamental labor market reform. IMF Staff Papers, 44(1), 1–35.
- Elmeskov J., Martin J., & Scarpetta S. (1998). Key lessons for labour market reforms: Evidence from oecd countries' experiences. Swedish Economic Policy Review, 5, 205–252.
- OECD (1994). Evidence and explanations, part II – The adjustment potential of the labour market (Technical report). OECD Job Study.
- Fiori G., Nicoletti G., Scarpetta S., & Schiantarelli F. (2012). Employment effects of product and labour market reforms: Are there synergies?. Economic Journal, 122(558), F79–F104.
- Gatu C., Kontoghiorghes E. J., Gilli M., & Winker P. (2008). An efficient branch-and-bound strategy for subset vector autoregressive model selection. Journal of Economic Dynamics and Control, 32(6), 1949–1963.
- Greene, W. H. (2003). Econometric analysis (5th ed.). New Jersey: Prentice Hall.
- Gwartney J., Lawson R., & Hall J. (2012). Economic freedom of the world 2012 annual report (Technical report). Fraser Institute.
- Hendry D. F., & Krolzig H.-M. (2004). We ran one regression. Oxford Bulletin of Economics and Statistics, 66(5), 799–810.
- Howell D. R., Baker D., Glyn A., & Schmitt J. (2007). Are protective labor market institutions at the root of unemployment? A critical review of the evidence. Capitalism and Society, 2(1), 1–73.
- Howell D. R., & Rehm M. (2009). Unemployment compensation and high european unemployment: A reassessment with new benefit indicators. Oxford Review of Economic Policy, 25(1), 60–93.
- IMF (2003). World economic outlook (Technical report).
- Kapetanios G. (2007). Variable selection in regression models using nonstandard optimisation of information criteria. Computational Statistics & Data Analysis, 52(1), 4–15.
- Nickell, S., & Layard, R. (1999). Labor market institutions and economic performance. In O. Ashenfelter & D. Card (Eds.), Handbook of labor economics (Vol. 3, chapter 46, pp. 3029–3084). Amsterdam: Elsevier.
- Nickell S., Nunziata L., & Ochel W. (2005). Unemployment in the oecd since the 1960s. What do we know?. Economic Journal, 115(500), 1–27.
- Roodman D. (2009). A note on the theme of too many instruments. Oxford Bulletin of Economics and Statistics, 71(1), 135–158.
- Sachs A. (2012). What really drives unemployment? A bayesian approach to determine the impact of institutions on the unemployment rate. Economics Bulletin, 32(1), 1008–1019.
- Sachs A. (2015). IUnemployment, institutions, and interdependencies: Identifying successful reforms. Journal of Economic Policy Reform, 18(1), 34–50.
- Savin I., & Winker P. (2012). Heuristic optimization methods for dynamic panel data model selection: Application on the russian innovative performance. Computational Economics, 39(4), 337–363.
- Scarpetta S. (1996). Assessing the role of labour market policies and institutional settings of unemployment: A cross-country study (Technical Report 26). OECD Economic Studies.
- Soskice D. (1990). Wage determination: The changing role of institutions in advanced industrialized countries. Oxford Review of Economic Policy, 6(4), 36–61.
- Visser J. (2011). The ictwss database: Database on institutional characteristics of trade unions, wage setting, state intervention and social pacts 1960–2010 (ICTWSS) (Technical report). Amsterdam Institute for Advanced Labour Studies AIAS.
- Winker P., & Fang K.-T. (1997). Application of threshold-accepting to the evaluation of the discrepancy of a set of points. SIAM Journal of Numerical Analysis, 34, 2028–2042.
Appendix
Genetic algorithms (GA) update the whole set of solutions simultaneously, see also Algorithm 1 for a pseudocode. Solutions are defined as models with a differing set of interaction terms. Genetic algorithms rely on the principle of replicating the evolutionary process such that superior model set-ups have a higher chance to survive. To initialize the algorithm, we select an initial population (K) of 500 solutions (randomly generated different model structures) as advocated by Savin and Winker (Citation2012).Footnote14 The members of the population are called chromosomes (ϕ). These are randomly generated binary strings (genes) representing the model structure: 0 means excluding the respective interaction term, 1 means including the interaction term; thus, each member of the population represents a specific model structure. For all initial solutions the model is estimated, the information criterion calculated and the elitist (=best among all candidate solutions) reported.
After the initialization, the generations ()Footnote15 start by taking the best half of the originally generated model structures. The best half are called parents, and denoted
. First, those are directly transferred to the new population and second, they are used to generate further solutions (children). This works as follows. Based on 100 randomly selected pairs of parents, 200 children (=new model structures) are formed by crossing them over: Certain regressors (interactions terms) with probability 0.5, are used from the first parent (model structure) and the other from the second. Moreover, the forty best parents – 40 models with best value of objective function – generate another 40 children. This leaves us with overall 240 (C) solutions generated by the uniform crossover mechanism.
To get 10 further solutions, we use the ten best solutions () and change them at one random gene. In other words, we delete or add one interaction term. Having formed a new population, the information criterion is calculated for each model structure based on the estimation outcome. After sorting the new model structures (population) by their objective function value, a random mutation, which refers to a small (if any) change of the model structure, is done to prevent the algorithm to trap in a local minimum. This mutation is applied to the new population except the ten best model structures (
) and the ten children generated from
. Thereby, eight random interaction terms (genes) are changed with probability 0.5 over all elements of the population. Again, at this step the elitist value and its associated model structure are stored. The algorithm runs either a predefined number of generations (
) or stops if all elements of the population have converged to be identical, indicating that all 500 model structures are equal. This implies that the algorithm has reached an optimum.
Threshold Accepting (TA): The model structure is initialized randomly with a binary string of zero and ones (). Based on the randomly initialized model structure, the estimation is performed and our objective function is calculated. This value and the model structure are stored. After the initialization, the iterations start. In each iteration step a new solution (
) which is a neighbor to the current solution is derived. Two regressors out of all potential regressors are selected randomly. The following rule applies: The first regressor is included if it was excluded before and the second vice versa. This corresponds to a Hamming Distance of two. Based on this new structure, the new information criterion is calculated. Then, the difference between the previous and the new value of the loss function is calculated. If the difference is smaller than the corresponding value of the threshold sequence (τ) the new structure is accepted else the previous combination is restored. We use a data-driven threshold sequence as advocated by Winker and Fang (Citation1997) which is based on differences of the objective function. These are generated by running the algorithm without the acceptance criterion and taking the difference of the initial value and new objective function. The threshold sequence for the threshold accepting algorithm gets linearly lowered to zero within 60% as recommended by Savin and Winker (Citation2012). In each iteration step the elitist is preserved to account for potential impairments of the objective function. The next iteration step follows until the predefined number of iterations (
) is done.