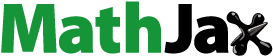
Abstract
This article investigates the demand for military expenditure for a sample of key Asia-Pacific countries. Spatial panel demand estimates are presented for three joined spatial units using a fixed-coefficient spatial lag model based on a two-step efficient GMM estimator. Spatial autoregression estimates are next presented for 1991–2015, founded on alternative kinds of country connectivities, such as contiguity, inverse distance, discrete distance, and power-projection considerations. Finally, 11 select countries’ demands for defense equations are estimated using seemingly unrelated regressions. From alternative perspectives, these estimated models indicate how Asia-Pacific countries respond to the defense spending of other countries. In the spatial runs, free riding is prevalent despite the growing military might of China, which apparently is not generally viewed as a threat. For the sample period, the projection of Chinese or American power is a relevant spatial factor. The main threat is reflected in non-U.S. allies’ reaction to U.S. allies’ defense spending during 1991–2015 and to Chinese defense spending after 2002.
Introduction
Since the seminal piece on the economic theory of alliances by Olson and Zeckhauser (Citation1966), the response of a country’s defense spending to that of its allies or enemies has been an important research topic (see e.g. Smith Citation1980, Citation1995; Murdoch and Sandler Citation1982, Citation1984; McGuire and Groth Citation1985; Bruce Citation1990). This theory of alliances highlights the purely public benefits of allies’ defense spending, as one ally’s defense provides nonrival and nonexcludable benefits to other allies that confront the same enemy states. Given the substitutability of allies’ defense spending, an ally’s defense demand is generally hypothesized to respond negatively to the collective defense spending (or defense spillovers) of its allies owing to free riding (Hilton and Vu Citation1991; Murdoch Citation1995; Sandler and Hartley Citation1995; Douch and Solomon Citation2014). If, however, an ally derives jointly produced country-specific and alliance-wide outputs from its defense spending, then a positive response to allies’ defense spillovers may characterize an ally’s defense demand (Sandler Citation1977; Sandler and Murdoch Citation1990; Sandler and Hartley Citation2001). This positive response follows because increased spillovers may raise an ally’s interest in the jointly produced complementary country-specific benefits that can only be obtained by augmenting the country’s own military expenditure (ME). Thus, the existence of ally-specific private benefits may limit the substitutability of allies’ defense spending. When jointly produced country-specific benefits do not exist or are not complementary, then the standard negative response to spillovers is anticipated. Increases in a perceived enemy’s defense spending or threat are anticipated to augment a country’s defense spending (Bruce Citation1990).
In the literature, estimated defense demand specifications assume many forms (e.g. cross-sectional, panel, dynamic panel, and autoregressive distributed lag), where some measureFootnote1 of ME responds to economic, strategic, and socio-political controls (see e.g. Dunne and Perlo-Freeman Citation2003; Nikolaidou Citation2008; Wang Citation2013; Markowski, Chand, and Wylie Citation2017). Economic factors may include gross domestic product (GDP) or trade openness, while socio-political considerations may involve population or political institutions. For instance, the nature of countries’ regimes – democratic or autocratic – may be an important influence on ME if more democratic countries are less apt to go to war and, thus, spend less on defense (Wang Citation2013). Strategic influences include allies’ or neighbors’ defense spending or perceived threat from an enemy’s defense spending. Smith (Citation1995) provides an excellent survey on the demand for defense spending.
Two recent major developments characterize the empirical estimation of defense demand. First, panel estimates are used extensively during last 20 years and have replaced cross-sectional studies (see e.g. Douch and Solomon Citation2014). Second, spatial methods are applied to defense spillovers based on some measure of propinquity or connectivity, such as contiguity or nearness in distance (Flores Citation2011). In an initial spatial study of defense spending, Goldsmith (Citation2007) investigates a global sample of 120 countries for just 1991, and finds that a country’s military burden, ME/GDP, is positively correlated with those of its neighbors. In a follow-up study, Skogstad (Citation2016) examines alternative spatial weight matrices for 124 countries during 1993–2008 to show that defense burdens of neighboring countries are positively correlated. He also indicates that a country’s regional location may influence its defense spending. Subsequent spatial panel defense studies include alternative connectivity measures for the North Atlantic Treaty Organization (NATO) (George and Sandler Citationforthcoming) and a global sample (Yesilyurt and Elhorst Citation2017). The inclusion of spatial considerations means that relative location matters because, for example, nearer allies’ forces can come to one another’s assistance more rapidly, thus allowing for greater substitutability of forces and thus free riding. If, however, a major country can rapidly project its power, then spatial and locational considerations assume less importance.
The primary purpose of this paper is to present an up-to-date spatial panel analysis of the demand for defense for an Asia-Pacific sample of countries. Our fixed-coefficient spatial lag (FCSL) model allows the spatial lag ME coefficient to differ across spatial units (Elhorst Citation2003). In particular, we estimate separate equations for three joined spatial units using a two-step efficient generalized method of moments (GMM) estimator. In addition, various spatial autoregression (SAR) models are also estimated where spatial connectivity is based on regional membership, contiguity, inverse distance, contiguity augmented with the United States, and contiguity augmented with China. Additionally, we augment contiguity spatial connectivity with the defense spending from nearby, but not contiguous, countries within 2000 and 4000 km of the sample countries. This discrete-distance spatial connectivity allows for broader defense spillovers than contiguity (Flores Citation2011). Again, a two-step efficient GMM estimator is employed for all of our spatial demand estimates. A secondary purpose is to present separate demands for ME estimates for 11 important Asia-Pacific countries, based on seemingly unrelated regressions (SUR) using alterative representations of defense spillovers. U.S. defense spending is included in the spillover term because it has an important military Asia-Pacific presence and is allied with some key countries in the region. One set of SUR ME demand equations has an aggregate defense spillover term, while the alternative set of ME demand equations distinguishes among three kinds of defense spillovers. These spillovers involve: (i) the United States and its six regional allies’ defense spending, (ii) Chinese defense spending, and (iii) the collective of other sample countries’ defense spending. For 1991–2015, these SUR estimates indicate how a sample of Asia-Pacific countries views the growing military presence of China and the United States.
Many findings stem from our estimates. As separate groups, U.S. allies and non-U.S. allies responded negatively to Chinese ME, indicative of a lack of perceived threat during the 1991–2015 period. This is especially surprising because China and some sample countries have territorial disputes. By contrast, China reacted positively to non-U.S. allies’ ME, consistent with China’s goal for regional dominance. Next, we turn to spatial connectivity. Five alternative spatial measures of spillovers led to a significant free-riding response during 1991–2015, indicative of Asia-Pacific countries not viewing their immediate neighbors or China as a threat. Four discrete-distance-augmented contiguity measures provide consistent and further evidence of free riding and that countries are not viewing their adjacent and nearby neighbors as a threat. Our spatial estimates suggest that China and the United States have a defense presence in the Asia-Pacific region. The SUR estimates provide a more nuanced picture of free-riding, complementarity, and threat responses by the Asia-Pacific sample.
Why Study Asia-Pacific Defense Spending?
There are numerous studies of NATO and European Union (EU) countries’ demand for ME, which apply a variety of empirical specifications (e.g. Smith Citation1980, 1990; Murdoch and Sandler Citation1984; Kollias Citation2008; Nikolaidou Citation2008; Christie Citationforthcoming; George and Sandler Citationforthcoming). There are far fewer studies of defense demand of Asia-Pacific countries. Some notable studies of Asia-Pacific defense demand include Markowski, Chand, and Wylie (Citation2017), Murdoch and Sandler (Citation1985), and Wang (Citation2013). There are a number of reasons why the study of defense spending in this region needs more attention. Since 1991, there has been a sustained increase in Asia-Pacific ME as shown in . This region includes the World Bank classifications of South Asia, East Asia, and Pacific. Asia-Pacific defense spending overtook that of Europe and Central Asia in 2014. Moreover, Asia-Pacific ME displayed a steady upward rise and did not have a downturn as in the case of North America. The latter’s downturn primarily resulted from U.S. budget sequestration and deficit-reducing measures after 2009 (Sandler and George Citation2016). Defense spending in Latin America and the Caribbean, as well as in Europe and Central Asia, grew modestly during 1991–2015. Despite a fall in ME in 2015, the Middle East and Africa experienced some ME growth but at a more modest rate than for Asia. The closing gap between Asia-Pacific ME and North America ME is noteworthy and distinguishes Asia-Pacific from the other three regions.
Figure 1. Total military expenditure by region, 1991–2015.
Notes: Asia-Pacific includes World Bank classification of South Asia, East Asia, and Pacific, and Africa includes both sub-Saharan Africa and North Africa.
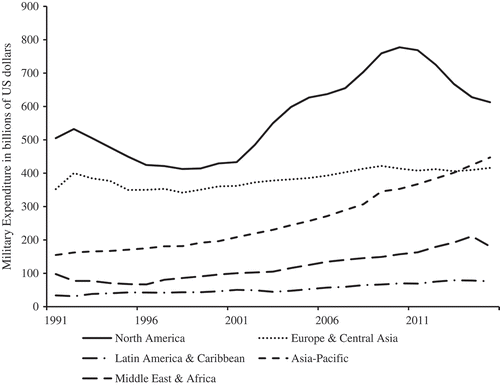
Another rationale for focusing on Asia-Pacific involves China’s rising defense spending since 1991. In constant 2014 U.S. dollars, China’s ME rose from 23.4 billion U.S. dollars in 1991 to 214.5 billion U.S. dollars in 2015, which was over a ninefold increase. This is an average annual real ME growth rate of 9.75%, which is slightly less than China’s annual growth of real GDP of 9.98% during 1991–2015. China’s annual ME growth rate picked up somewhat after 2002 – it was 8.55% for 1991–2001 and 10.70% for 2002–2015.Footnote2 In this latter period, China’s real ME was outpacing its average annual real GDP growth rate of 9.76%. Given China’s prospect for GDP growth, ME growth exceeding GDP growth is an important trend and may represent a worrying threat for the region. Additionally, China’s ME growth greatly exceeded that for the other regional powers. For 1991–2015, ME growth rates were 4.33% for India, 3.47% for South Korea, and 0.33% for Japan. During the same period, U.S. defense spending grew at just 0.49% annually. These ME growth rates are less than these countries’ GDP growth rates of 6.56% for India, 5.02% for South Korea, 1.00% for Japan, and 2.46% for the United States. Russia’s ME growth is notable because it grew at 8% per year after 2002 and represents a driving force in the growth of non-U.S. allies’ ME during 2002–2015 in the Asia-Pacific region.
indicates the changing nature of defense spending in Asia-Pacific by indicating the regional shares of ME for China, six U.S. allies (i.e. Australia, Japan, New Zealand, the Philippines, Pakistan, and South Korea), India, and others (i.e. Bangladesh, Cambodia, Indonesia, Laos, Malaysia, Nepal, Russia, Singapore, Sri Lanka, Thailand, and Vietnam). China and India are highlighted given their GDP growth, their sizable GDP, large population, and significant regional influence. U.S. allies are the six countries with which there is or has been a formal alliance with the United States.Footnote3 depicts four snapshots in time at 1991, 2000, 2010, and 2015. China’s rising regional ME shares and U.S. allies’ falling regional ME shares are readily apparent and suggest that the U.S. allies are not treating China’s military buildup as a threat. Specifically, China’s regional ME share rose from 16.5% in 1991 to 40.9% in 2015, while the six U.S. allies’ regional ME share fell from 59.8% in 1991 to 24.5% in 2015 – almost a reversal in shares between China and the six U.S. allies. Despite India’s improved GDP growth, its regional ME share went from 12.2% in 1991 to 9.8% in 2015, with a short-lived increase to 12.8% in 2000 before declining thereafter. Finally, the other Asia-Pacific countries’ regional ME share rose steadily from 11.5% in 1991 to 24.8% in 2015, primarily due to increases in Russian ME since 2001.
Figure 2. Major Asia-Pacific countries’ regional shares of military expenditure, 1991–2015.
Notes: U.S. allies include Australia, Japan, New Zealand, Pakistan, Philippines and South Korea. Others include Bangladesh, Cambodia, Indonesia, Malaysia, Laos, Nepal, Russia, Singapore, Sri Lanka, Thailand, and Vietnam.
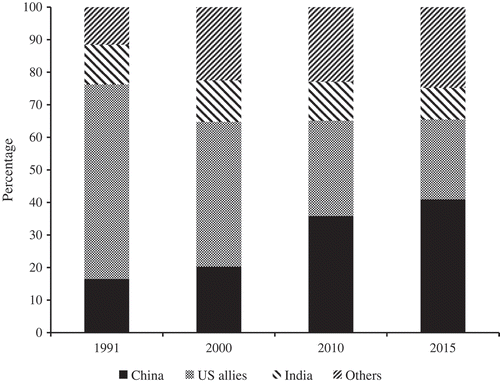
provides yet another vantage of defense spending among key countries in the Asia-Pacific region, ME/GDP values are listed for the list of 19 sample countries and the United States at same four snapshots in time as in . In , missing ME/GDP values are not given in SIPRI (Citation2017). Sixteen of 20 countries in experienced a decline in their military burdens between 1991 and 2015. The most glaring exception is Russia, whose ME/GDP rose from 3.55% in 2000 to 4.90% in 2015. Three countries – Bangladesh, Japan, and Nepal – experienced a small increased military burden between 1991 and 2015. China is also noteworthy because its military burden declined somewhat from 2.35% in 1991 to 1.94% in 2015, despite its rapid growth in ME. This reduced burden follows from China’s GDP growth slightly outpacing its ME growth. Chinese defense spending growth is consistent with many countries using their expanding GDP to fund defense almost proportionately.
Table 1. ME/GDP values for sample countries at four snapshots.
There are at least two other compelling grounds for studying defense demand in the Asia-Pacific region. First, intrastate wars or conflicts plagued this region since the start of 1991 and included one or more conflicts in Bangladesh, India, Indonesia, Malaysia, Nepal, Pakistan, the Philippines, Sri Lanka, and Thailand.Footnote4 According to the Uppsala Conflict Data Program/Peace Research Institute Oslo (Citation2017), an intrastate war involves two or more domestic parties, one of which is the domestic government. The other party (parties) is (are) the domestic opposition group(s). Moreover, an intrastate conflict must surpass a threshold of 25 battle-related deaths annually. During the sample period, India and the Philippines endured the most intrastate wars: India experienced an average of 4.6 conflicts annually and the Philippines experienced an average of 1.8 conflicts annually. Bangladesh, Cambodia, Indonesia, Malaysia, Nepal, Pakistan, Sri Lanka, and Thailand never endured more than one intrastate conflict during a year. For example, Indonesia had a single intrastate war in 1991 and an ongoing conflict during 1997–2005, while Nepal had a single intrastate war during 1996–2015. Thailand had a single ongoing intrastate conflict during 2003–2004 and 2006–2015. Russia experienced an average of one intrastate war per year during 1991–2005. Cambodia had one intrastate war during 1991–1998 and an interstate war in 2011. Laos had no wars during the sample period.
Second, territorial disputes between China and other countries in the region characterize 1991–2015 and raise interest in how defense demands were potentially affected. Noteworthy territorial disputes between India and China date back to British colonial rule. China also has territorial issues with Indonesia, Malaysia, the Philippines, Russia, and Vietnam during the sample period (Council of Foreign Relations Citation2017). In addition, Japan and China disputed the sovereignty of the Diaoyu (Senkaku) Islands (Lee Citation2002), while South Korea and China disputed a submerged reef, the Socotra Rock since 1995 (Horesh et al. Citation2014).Footnote5 Agreements settling Chinese–Russian border disputes were signed in 1991, 1994, 1998, and 2004 (Dmochowski Citation2015). As of 2004, there are no remaining territorial disputes between the two countries.
Theoretical Model
We present a generic demand for ME model capable of capturing spatial and nonspatial defense spillovers among a regional cohort of countries as well as other countries with a military presence in the region. For the Asia-Pacific region, the latter would include the United States that has military bases in the region and strong alliance linkages to Japan, South Korea, and Australia, respectively. There are weaker U.S. alliance linkages to Pakistan, the Philippines, and New Zealand (Correlates of War (COW) Project Citation2013). Spatial connectivity may stem from spatial propinquity (contiguity or nearness in distance) or power projection by dominant countries, such as China and the United States (Skogstad Citation2016; Yesilyurt and Elhorst Citation2017; George and Sandler Citationforthcoming).Footnote6
We assume an N-country scenario in which a unitary actor (e.g. an executive decision-maker) in each country maximizes social welfare, U i , subject to the country’s budget or resource constraint and the defense spending decisions of other relevant countries. Relevancy may be based on some measure of spatial propinquity, power projection, alliance, or adversarial relationships. The strictly quasi-concave social welfare function is
where denotes the ith country’s private consumption good. The public defense good, Q, is the sum of the country’s own defense, q
i
, and defense spillovers, Q
−i
, that it receives from other countries. The vector
includes other relevant arguments such as trade openness, population, and threat factors that may influence social welfare, which increases with larger values of the private and public defense goods.
Defense spillovers for relevant countries can be written as
where δ k denotes the weight applied to the kth country’s defense spending. If, for example, contiguity determines defense spillovers, then δ k = 1 for countries that have a land or a water border with country i, and δ k = 0 for countries that are not contiguous with country i. If contiguity measures account for countries that can project their power, then adjoining countries to country i and countries that can project power are assigned δ k = 1. When spatial propinquity, but not contiguity, defines defense spillovers, the inverse distance between country i’s capital and that of country k defines δ k as a continuous weight. Inverse distance places greater spillover weights on the defense spending of nearer countries. In the case of allies, greater proximity allows the forces of one country to substitute more fully for the forces of another country. The Asia-Pacific region constitutes a large area so that spatial considerations surely matter in how readily one country’s forces may assist or challenge those of another country. If, however, spatial location of countries is not a concern, then δ k equals 1 for all included countries in the spillover term, so that all sample countries in the region are treated identically. An alternative spillover relationship is to distinguish between the defense spending of different countries cohorts. Suppose, for instance, that group 1 denotes allied countries and group 2 represents non-allied countries, then we have:
where the second subscript on Q −i and the second superscript on q k indicates the respective group. N 1 and N 2 denote the number of countries in the respective group.Footnote7
Each country is constrained by
in which the unit price of the private good is one, the unit price of the defense good is p, and national income or gross domestic product (GDP) is I. If the budget constraint and the spillover relationship in Equation (2) are substituted into the social welfare function in Equation (1), we can express each country’s optimization problem as
We remind the reader that the spillover term can assume various forms depending on how connectivity among countries is defined. This optimization problem implies the following reduced-form equation for country i’s demand for defense,
If defense is a normal good, then defense demand responds positively to GDP. Moreover, defense demand falls as the relative price of defense increases. By necessity, this relative price variable is generally dropped in empirical studies of defense demand because of a lack of data (Murdoch and Sandler Citation1984; Smith Citation1995). There is no bias in doing so provided the prices of defense goods inflate at the same rate as those of civilian goods. This is shown to be the case in a SIPRI (Citation1983) study. However, Solomon (Citation2005) finds that military goods inflate at a higher rate than civilian goods for Canada.Footnote8 A country’s defense demand responds negatively to spillovers from allies or friendly nearby states. By contrast, a country’s defense demand is anticipated to rise in response to larger defense spending by a collective of adversarial states owing to enhanced perceived threat. In Equation (6), the vector of other factors includes the country’s population and its trade openness. If population raises the ability to finance defense as more people can carry this burden, then defense demand may increase with a larger population (Dudley and Montmarquette Citation1981). By contrast, a larger population may negatively impact defense demand if a greater population base allows the country to substitute relatively cheap manpower for expensive capital defense equipment (Wang Citation2013). Greater population may also create a smaller demand for defense when there results a larger competing demand for civilian goods (Dunne and Perlo-Freeman Citation2003). The effect of trade openness on defense demand is also unpredictable. Trade openness reduces defense demand if countries in a region become more dependent on one another; however, trade openness increases defense demand if countries must protect trade routes.
Data and Empirical Methodology
Data
We estimate the demand for ME for 19 Asia-Pacific countries during 1991–2015. Sample countries include Australia, Bangladesh, Cambodia, China, India, Indonesia, Japan, Laos, Malaysia, Nepal, New Zealand, Pakistan, Philippines, Russia, Singapore, South Korea, Sri Lanka, Thailand, and Vietnam. A map of the sample countries is displayed in , where these countries are shaded in gray.
Figure 3. A map of sample countries.
Notes: The sample countries include Australia, Bangladesh, Cambodia, China, India, Indonesia, Japan, Laos, Malaysia, Nepal, New Zealand, Pakistan, Philippines, Russia, Singapore, South Korea, Sri Lanka, Thailand, and Vietnam.
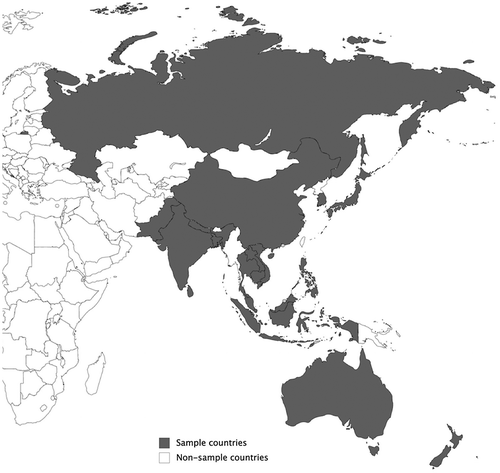
A main consideration in our choice of sample countries is the availability of panel data on ME, extracted from the SIPRI (Citation2017) military expenditure database. SIPRI’s recently updated database combines information from government documents, international statistics, journals, and news reports to obtain reliable and consistent country-level estimates of defense spending (SIPRI Citation2017). Bhutan is left out of our sample because there are no ME data for this country in SIPRI (Citation2017). SIPRI ME data on North Korea, measured in local currency, are reported by the North Korean Government and exclude key defense spending components, such as investment in the arms industry and R & D in dual-use technology. These North Korean ME amounts cannot be converted into constant U.S. dollars, thereby making them noncomparable to the ME spending of other sample countries. Moreover, there are no data on GDP or trade openness for North Korea. These data problems pose insurmountable problems that lead Skogstad (Citation2016), Wang (Citation2013), and others to leave out North Korea from their sample. Additionally, there are missing values of the economic data for Myanmar (formerly Burma) and Papua New Guinea. Thus, these two countries are also left out of our sample. Taiwan is not recognized by the World Bank as a country; hence, Taiwanese economic variables are not in World Bank (Citation2016). If we use Penn World Tables for Taiwanese data, we cannot convert its data to constant 2014 U.S. dollars, consistent with our other sample countries. Consequently, we do not include Taiwan. We acknowledge that our sample choice may result in some omitted variable bias, but this is true of all defense demand studies because data limitations and focus always necessitate dropping countries.
Our sample period commences with China’s emergence as a major military power and the subsequent shift in the regional defense balance away from U.S. Asia-Pacific allies – see .Footnote9 Our dependent variable, annual ME, is measured in 2014 constant U.S. dollars. Data on GDP in constant 2014 U.S. dollars and Population (POP) are extracted from World Bank’s (Citation2016) World Development Indicators along with the Trade Openness variable, measured as the sum of total exports and imports as a percentage of GDP.
For constructing spatial weights matrices, we use multiple alternate measures of distance-based connectivity. The binary contiguity matrix, indicating whether a country shares land or water borders with another country, is obtained from COW Direct Contiguity Data, which records all direct contiguity relationships by land or water (Correlates of War (COW) Project Citation2006; Stinnett et al. Citation2002). The weights matrix representing inverse distance between capital cities is taken from Gleditsch and Ward (Citation2001). Using the same data-set, we also construct two distance-based binary contiguity matrices. Classification of countries into U.S. allies and non-U.S. allies is based on the COW Formal Alliances data-set, which records all formal alliances among states between 1816 and 2012, including mutual defense pacts, non-aggression treaties, and ententes (Gibler Citation2009; Correlates of War (COW) Project Citation2013).
Empirical Methodology
Based on our explicit theoretical model, the dependent variable is a country’s level of ME and not its defense burden (i.e. ME/GDP). As such, we adhere to a deductive approach for which the theoretical antecedent model determines the reduced-form estimating equation (Hartley and Sandler Citation1990; Sandler and Hartley Citation1995). Moreover, the defense spillover variable is a weighted sum of the sample countries’ defense spending levels, where the weights depend on some measure of connectivity, such as contiguity or nearness. This ME approach characterizes defense demand estimates by Douch and Solomon (Citation2014), George and Sandler (Citationforthcoming), Murdoch and Sandler (Citation1984), Solomon (Citation2005), and many others. Some recent spatial panel estimates of defense demand take an inductive approach and use military burdens as the dependent variable. These studies compute spillovers in terms of a weighted sum of other countries’ military defense burdens (Goldsmith Citation2007; Skogstad Citation2016; Yesilyurt and Elhorst Citation2017). This practice troubles us on three grounds. First, there is no theoretical model to justify an estimating equation based on military burdens. Second, the use of military burden spillovers loses the scale of defense spending by a country’s neighbors. In 2015, China and Australia had almost identical military burdens (see ), but China greatly outspent Australia and should have had a larger impact on the perceived spillovers or threat of its neighbors. Third, there is much less variation in ME/GDP than in ME to identify the defense demand estimations – again, refer back to . Most sample countries’ military burdens are within one percent of one another.
In our main set of estimates, we use a static model and do not lag spillovers in keeping with our theoretical model. Thus, our empirical representation is more interested in how Asia-Pacific countries respond to various measures of spatial spillovers, while accounting for their endogeneity. Alternatively, some studies focus on bureaucratic inertia by lagging the dependent variable – see, especially, Wang (Citation2013) for his study of South-East Asian military spending.
We express the log of country i’s demand for ME in year t as follows:
where i = 1, 2, … , N; t = 1, 2, … ,T; and k = 1, 2, …, N. In Equation (7), ρ represents the spatial autoregressive parameter that measures the effect of other countries’ collective ME or defense spillovers on . In particular,
denotes the spillover term for country i in period t, which corresponds to the ME of other countries in the region during the period. GDP
it
denotes country i’s gross domestic product in period t, POP
it
indicates its population in period t, and TradeOpenness
it
represents the trade openness for country i during period t. μ
i
denotes country fixed effects, while ε
it
is an identically and independently distributed (i.i.d) error term. Due to the unavailability of panel data on the relative price of defense, we drop the price variable from the estimating regressions, as previously mentioned and as is common in the literature (see e.g. Murdoch and Sandler Citation1984; Smith Citation1995; Douch and Solomon Citation2014).
The strategic interdependence between countries in deciding their respective MEs raises endogeneity issues with respect to ME as a dependent variable and the spillover terms as an independent variable. However, the spillover term in Equation (7) can be derived from the reduced form of a spatial econometric model, where the ME of country i depends on the MEs of other countries (George and Sandler Citationforthcoming). In the current study, we use two different types of spatial econometric models to estimate the demand for ME: A Fixed Coefficient Spatial Lag (FCSL) model and a Spatial Autoregressive (SAR) model. In both models, the spillover term is treated as a spatially lagged dependent variable, measured by a weighted sum of other countries’ MEs. In general, spatial weights could be any measure of connectivity such as geographical proximity or even alliance membership.
An FCSL model allows the spatial autoregressive parameter, ρ, to vary across spatial units or homogeneous groups of spatial units (Elhorst Citation2003). In this study, we use an FCSL model, where the spillover term is split into three variables; U
.
S
. allies ME, non-U
.
S
. allies ME, and Chinese ME, and the corresponding are estimated for each term. By allowing SAR coefficients to vary, we expect countries in the region to respond differently to U
.
S
. allies ME, non-U
.
S
. allies ME, and Chinese ME. The resulting empirical model can be written as:
where ρ
1 and ρ
2 denotes spatial autoregressive coefficients corresponding to U
.
S
. allies ME and non-U.S
. allies ME, respectively. denotes the weighted sum of U.S. allies’ ME, and
denotes the weighted sum of non-U.S. allies’ ME. Moreover,
and
capture the relative connectivity between countries i and k
1 (U.S. allies), and i and k
2 (non-U.S. allies), respectively.
In an SAR model, we assume that the spatial relations between all sample units can be represented using a single coefficient, ρ. Hence, the empirical equation for an SAR model becomes:
where is now the spatially lagged ME variable. While in our FCSL model, we use a single spatial weighting scheme namely a country’s alliance with the United States; in our SAR model, we use several spatial weighting or connectivity schemes. First, we apply a standard spillover representation, where w
ikt
= 1, k ≠ i, for all sample countries. We also use a binary contiguity weight scheme based on land or maritime borders, and we also apply a distance-weighting scheme based on inverse distance between capital cities. To account for the ability of the United States to project its military power throughout the Asia-Pacific region regardless of its geographical proximity to a country, we employ a contiguity plus U
.
S
. weight matrix, where w
ikt
= 1 if country k is contiguous to country i or country k is the United States, otherwise w
ikt
= 0. Similarly, a contiguity plus China matrix places a weight of one if country k is contiguous to country i or country k is China. Sometimes, a country might respond to the MEs of more distant countries, beyond their immediate neighbors. Hence, we apply weighting matrices, where w
ikt
= 1 when the distance between capital cities of countries i and k falls below a certain cut-off level, and w
ikt
= 0 otherwise. Given our list of sample countries, we use 2000 and 4000 km as the cut-off distances following the lead of Flores (Citation2011).Footnote10
A final set of weight matrices adds Chinese ME or U.S. ME to the cut-off distance-based contiguity matrices. We row-normalize all the weight matrices except the inverse-distance matrix, as is standard in the spatial econometric literature.
All the models are estimated using the two-step efficient GMM estimators with standard errors robust to heteroscedasticity and autocorrelation (Kelejian and Prucha Citation1998). The GMM estimators for SAR and FCSL models use spatial lags of the independent variables as external instruments for the spatial lag of the ME variable. The regressions pass the standard checks for model identification and instrument strength. Statistically significant Kleibergen-Paap LM test statistics suggest that the models are identified. Cragg-Donald Wald F-statistic values are significantly large, ruling out the weak instruments problem (Baum, Schaffer, and Stillman Citation2003, 2007).
We, then, estimate separate defense demand equations for 11 sample countries in the Asia-Pacific region using the Seemingly Unrelated Regression (SUR) technique.Footnote11
SUR regressions, proposed by Zellner (Citation1962), were first used in defense literature by Murdoch and Sandler (Citation1984) to estimate the ME demand equations for a sample of NATO countries for the years 1961–1979. The SUR model accounts for the fact that the variances of the error terms are different across each equation and allows for contemporaneous correlation between the error terms. Or in other words, if we represent ε
i
as a vector of error terms for country i, then the SUR model assumes that and
, where I is the identity matrix.
We use three different spillover specifications to capture a country’s response to ME of other countries in the region. The Spillover variable denotes the sum of MEs of all sample countries minus the country’s own ME. The Spillover1 variable includes the MEs of Bangladesh, Cambodia, India, Indonesia, Laos, Malaysia, Nepal, Russia, Singapore, Sri Lanka, Thailand, and Vietnam, while the Spillover2 variable combines the MEs of Australia, Japan, New Zealand, Pakistan, Philippines, South Korea, and United States. The first SUR estimates include the inclusive Spillover variable. In the second SUR estimates, we include Spillover2 in order to distinguish U.S. allies’ spillovers from those of the other sample countries, included in Spillover1. As discussed earlier, the inclusion of United States signifies its ability to influence the defense policies in the region individually, and through its allies. In individual demand for ME SUR equations, non-spillover variables include Chinese ME, log (GDP), and Trade Openness. The estimates are calculated using feasible generalized least-squares estimation procedures with small-sample adjustments (Greene Citation2012).
Empirical Results
For , we begin with estimated defense demands for China, U.S. Asia-Pacific allies, and non-U.S. Asia-Pacific allies. The Chinese defense demand equation is simple by design because limited degrees of freedom restrict the choice of independent variables to the basic ones. Given China’s important position in the Asia-Pacific region, a separate Chinese FCSL defense demand equation is desirable and offers another vantage to the response to spillovers. In Model 1, Chinese defense spending responds positively to non-U . S . allies ME, but this response is only marginally significant. China’s positive response may be driven by territorial disputes with some of these countries and China’s drive for regional ME dominance. U.S. allies react negatively and significantly to Chinese ME in Model 2, indicative of the absence of a perceived threat. In Model 3, non-U.S. allies raise their ME as U.S. allies, but not China, increase their defense spending. These spillover coefficients are elasticities since the dependent variable in each model is the natural log of ME for China, U.S. allies, or non-U.S. allies, respectively. The basic message is that the latter two collectives are not viewing Chinese ME buildup as a threat, consistent with Wang’s (Citation2013) result for South-East Asia. This finding is not so surprising because it comes during a time when financial crises and peace dividend pursuits meant that governments redirected some of their spending away from defense into civilian concerns. The declining military burdens since 1991 for 16 countries in are consistent with this redirection. During its post-2001 military buildup, China has not threatened its neighbors and maintained trade with many of them.
Table 2. China, U.S. allies and non-U.S. allies demand for ME, 1991–2015.
GDP is associated with a positive and significant income elasticity for China and U.S. allies that is elastic for China. U.S. allies and non-U.S. allies respond positively to Trade Openness, but the response is only marginally significant for the latter collective. Apparently enhanced trade augmented U.S. allies’ and non-U.S. allies’ perceived need for defense. POP has no impact on defense demand for U.S. and non-U.S. allies. The R squares are reasonably high for the three models. In the online Appendix, Table 2A allows U.S. allies and non-U.S. allies to respond to spillovers from their respective cohorts, only the response of U.S. allies to other U.S. allies is significant and positive, indicative of spillover complementarity that will reappear in one set of SUR runs. This online table adds a fourth model for the defense demand for the collective of all sample countries. A negative, but insignificant, reaction to Chinese ME holds for this collective, along with a positive and marginally significant reaction to defense spillovers from U.S. allies.
divides our estimates of U.S. allies’ and non-U.S. allies’ defense demand into two periods: 1991–2001 and 2002–2015. This division is chosen because our earlier discussion in Section ‘Why Study Asia-Pacific Defense Spending?’ indicates that China’s defense spending increased markedly after 2001 and began to approach China’s considerable growth in GDP. Thus, we are curious whether there is a greater threat response by these two cohorts after 2001. For 1991–2001, only non-U.S. allies react negatively and significantly to Chinese ME; however, non-U.S. allies respond positively to Chinese enhanced ME buildup after 2002. This latter response is indicative of a threat reaction. The U.S. allies still respond in a negative fashion after 2001 to Chinese ME, consistent with these allies’ very modest rise in ME when compared to a larger than 10% annual rise in Chinese ME during 2002–2015. GDP continues to be a positive influence on defense demand for both cohorts, but is not significant for non-U.S. allies in the latter period. When the periods are split, Trade Openness is a positive influence on defense demand for U.S. allies in both periods and is a significant positive influence for non-U.S. allies in the post-2001 period. This suggests that trade encourages some greater defense, which might be due to factors not captured in the data such as a rising piracy threat. For 2002–2015, POP is associated with a positive defense response in U.S. allies and a negative response in non-U.S. allies. The U.S. allies’ response suggests that rising population is facilitating defense financing, while non-U.S. allies’ response may indicate population-induced increases in civilian goods demand. Once again, the R squares are high, indicative of a good fit for all four models.
Table 3. U.S. allies and non-U.S. allies demand for ME, 1991–2001 and 2002–2015.
In our intent to view Asia-Pacific defense demand from alternative perspectives, we present five SAR country fixed-effect estimates in . For the Spillovers representation in Model 1, each sample regional country is assigned a weight of 1 applied to its ME. A country’s own defense spending is excluded from the Spillovers term. As explained in the methods section, Spatial Lag (contiguity) corresponds to δ k equal to 1 for only countries that share a land or water border. Spatial Lag (inverse distance) has δ k set equal to the reciprocal of the distance between capital cities, so that closer countries’ ME receives a greater weight. The last two contiguity spatial weighted spillover expressions apply a weight of 1 to the ME of the United States and China, respectively, along with a weight of 1 applied to the ME of contiguous neighbors. As such, contiguity and power projection determine spillovers for our last two spatial spillover measures, consistent with George and Sandler (Citationforthcoming), Skogstad (Citation2016), and Yesilyurt and Elhorst (Citation2017).
Table 4. Asia-Pacific military expenditure, 1991–2015.
In , the Spillovers coefficient is negative and significant, indicating some regional free riding and no overall perceived threat, consistent with Wang (Citation2013) and our . Free riding also characterizes the other four measures of spatial connectivity and indicates that sample countries are not viewing their various neighbors or the United States and China as a threat during the sample period. The significant negative coefficients of contiguity, contiguity + U . S ., and contiguity + China display very similar values. The contiguity + U . S . coefficient indicates that the U.S. ability to project power affords a free ride to many countries in the region. In addition, the contiguity + China coefficient strongly suggests that sample countries are not viewing China as a threat. The inverse-distance measure of spillovers is also a negative and significant influence on ME demand, consistent with neighboring and nearer countries not being viewed as a threat. In , the inverse-distance variable’s coefficient is much larger in absolute value compared to the other spatial measures because the former scales down the spatial lag variable relative to other spatial weighting schemes. This scaling-down factor results in a larger spillover response (George and Sandler Citationforthcoming). GDP is positive and significant for all five spatial models with income elasticities near or somewhat larger than unity. Trade Openness is not significant, while POP is negative and significant. The latter is consistent with larger populations causing competing demand for civilian goods to rise.
The results in suggest six alternative SAR representations involving contiguity2000, contiguity2000 + China, contiguity2000 + U . S . , contiguity4000, contiguity4000 + China, and contiguity4000 + U . S ., given the significance of contiguity, contiguity + China, and contiguity + U . S. We do not expand the inverse-distance SAR representation because it does not lend itself to a discrete-distance augmentation. In , adding defense spending from countries within 2000 km of the sample country to its contiguous neighbor’s defense spending yields significant negative spillovers at the 0.10 level. Extending this discrete augmentation of contiguity to 4000 km is consistent with significant negative spillovers at the 0.05 level. When these two discrete-distance contiguity measures are further enhanced by Chinese ME, the spatial spillovers coefficient is negative and significant at the .05 level for contiguity4000 + China. Discrete-augmented contiguity SAR measures for the United States are negative and significant for 2000 and 4000 km at the 0.10 and 0.05 level, respectively. Thus, there is convincing evidence that spatial spillovers matter, even when contiguity is augmented by discrete-distance measures. In five of six cases, free riding is evident. GDP elasticity remains near unity in value and significant, and POP is negative and significant. The latter is consistent with the competing demands for civilian goods tied to expanding population during a relatively peaceful period in the region (Dunne and Perlo-Freeman Citation2003). Trade Openness is not significant in the six models in .
Table 5. Asia-Pacific military expenditure, expanded contiguity runs, 1991–2015.
presents a much different disaggregated viewpoint in terms of 11 sample countries’ defense demands, estimated in an SUR framework. The Spillovers variable accounts for the ME of these 11 sample countries, augmented by the ME of Cambodia, Indonesia, Laos, Nepal, Russia, Singapore, Thailand, the United States, and Vietnam. When we include separate defense demand equations for all 19 sample countries, the variance-covariance matrix for errors is singular and, hence, the system of equations cannot be jointly estimated within an SUR framework (see footnote 11). In a couple of cases – e.g. Cambodia and Laos – the demand equations are not estimated owing to incomplete data that would further limit our degrees of freedom if these years are dropped. However, all sample countries’ defense spending along with that of the United States is kept for the Spillovers expression so as not to underestimate ME spillovers. When countries’ demands for ME are estimated, some heterogeneity becomes apparent and informs our earlier aggregate representations. China, Pakistan, Malaysia, and Australia respond positively to Spillovers, where regional spillovers are augmented by U.S. defense spending. These positive coefficients on Spillovers are consistent with either a perceived threat or a complementarity between country-specific defense outputs and the regionwide defense gain.Footnote12 In particular, Australia is viewing U.S. defense spending in a complementary way to its own defense outputs, consistent with the findings in Murdoch and Sandler (Citation1985) for a much earlier period. Malaysia may be responding to its territorial dispute with China, while China is seeking regional dominance and Pakistan has internal (i.e. intrastate wars) and external security concerns during the sample period. Free riding is marginally apparent for Japan and is likely due to the inclusion of U.S. defense spending. GDP is a positive and significant influence on each of these countries’ ME with the exception of Japan, which is constrained by the 1%-of-GDP rule for its defense spending. Trade Openness is a positive determinant of ME for South Korea and Malaysia, while it is a negative determinant of ME for Bangladesh, Australia, and New Zealand. Otherwise, Trade Openness is insignificant in affecting Asia-Pacific defense spending. Except for GDP, the SUR representation implies heterogeneous ME responses at the country level. In the case of the SUR estimates, our earlier free-riding results is less comparable because the SUR estimates has the considerable ME of the United States in the spillover term, unlike all of the representations (except for the contiguity + U . S . specification) in Tables –.
Table 6. Estimated coefficients from the SUR method for the demand for military expenditures, 1991–2015.
In , we separate defense spending spillovers into three components: Chinese ME, Spillover1, and Spillover2. Spillover1 consists of the defense spending of 12 Asia-Pacific countries (see the note to ), while Spillover2 includes the defense spending of the United States and its six Asia-Pacific allies. In , Japan, India, and Australia react positively and significantly to Chinese ME, so that most Asia-Pacific countries do not view China as a threat, consistent with most findings in Tables –. Bangladesh, Sri Lanka, and the Philippines respond negatively and significantly to Chinese ME, while South Korea, Pakistan, Malaysia, and New Zealand do not respond significantly to Chinese ME. Notably, South Korea, Australia, New Zealand, and Sri Lanka respond positively and significantly to Spillover2. The first three countries’ reactions are consistent with these three U.S. allies displaying a defense complementarity reaction. Japan’s negative response to these U.S. allies’ spillovers denotes free riding, noted in a different form in . The remaining Spillover2 coefficients are insignificant. In terms of Spillover1, only China, Pakistan, and Malaysia display the significant positive responses, which are marginally significant. South Korea, Sri Lanka, and New Zealand respond negatively to Spillover1, the defense spending of most of the other regional countries. In summary, with a few exceptions, a negative or insignificant ME response holds in most individual countries, indicative as before of free riding or a lack of concern for the ME spending of others in the region. In , five sample countries display positive income elasticities, while four (two) sample countries reduce (increase) their defense spending as Trade Openness increases. For these four countries, enhanced trade makes conflict and, thus, the need for arming less desirable. Income and trade openness reactions are affected by the disaggregation of spillovers. Tables and indicate heterogeneity at the country level.
Table 7. Estimated coefficients from the SUR method for the demand for military expenditures, 1991–2015.
We tried other specifications without success in the models in Tables – and –. Following Wang (Citation2013), we included a debt-to-reserves ratio variable from World Development Indicators (WDI) data-set (World Bank Citation2016), defined as the value of total external debt to the value of total reserves. This variable was added to determine if high leverage served as a constraint on ME. The variable did not yield significant results. Also following Wang (Citation2013), we added polity2 variable in our estimations to account for regime type. The polity2 index captures a country’s adherence to democratic principles on a 21-point scale ranging from –10 (autocracy) to +10 (democracy) (Center for Systemic Peace Citation2017). We anticipated that higher polity2 values would decrease defense spending, because democracies are viewed as trying to avoid conflict. However, the regime variable was insignificant in our runs. In addition, we interacted our spatial lag terms in Tables – with year dummies for 1997, 1998, and 1999, separately and together, to spot any structural change because of the Asian financial crisis. No such change was uncovered during our sample period that exceeded that of Wang (Citation2013).
As a final exercise, we estimate ME demand with lagged ME and lagged Spillovers as additional independent variables. Not surprising, the lagged ME is positive and significant, indicative of bureaucratic inertia. Moreover, Spillovers and lagged Spillovers are not significant. When we lag ME and the spatial contiguity measure, lagged ME is positive and significant, but current and lagged contiguity measures are not significant. These results are in Table 4A in the online Appendix. In an earlier version of the paper, we were reluctant to estimate such dynamic models, because we correctly suspected that lagged ME eliminates the researcher’s ability to identify spatial influences.
Concluding Remarks
Although European demand for defense have received a lot of research attention, very little attention has been paid to Asia-Pacific demand for defense, with the exception of two interesting recent articles (Wang Citation2013; Markowski, Chand, and Wylie Citation2017). This is unfortunate because the Asia-Pacific region has many interesting security features since 1991 that include the rise of China as a major economic and military power, the growing regional presence of the United States, the fighting of frequent intrastate wars, a sustained growth of regional defense spending, and numerous territorial disputes with China. To explore these features and others, we view Asia-Pacific defense demand from alternative perspectives that differ from recent articles. Unfortunately, the lack of reliable ME and economic data on North Korea keeps us from including it in the analysis. In particular, we include a spatial-based analysis of defense spillovers and a SUR representation of individual countries’ demand for ME. Both of these perspectives are new to the analysis of Asia-Pacific and offer interesting results.
Despite the many formulations for defense spending that are presented, some consistent insights follow. First, despite China’s rising ME, most countries in the region do not view China as a threat during 1991–2015. The main exception is the response of the non-U.S. allies after 2001. Our SAR estimated models find strong evidence of free riding. This free riding also characterizes five contiguity-augmented spatial spillover measures. Apparently, this free riding extends up to 4000 km of the country and includes the ME of contiguous countries, near non-contiguous neighbors, China, and the United States. This is a novel result and adds more context to the important findings of Wang (Citation2013). Second, defense is a normal good with positive income elasticity. Third, the influence of Trade Openness on defense demand hinges on the way defense spillovers are displayed. Fourth, there is a marked heterogeneous response of individual countries’ ME demand to standard influences. This is in contrast to some recent and past research findings with respect to European countries (e.g. Murdoch and Sandler Citation1984; Nikolaidou Citation2008; Douch and Solomon Citation2014). Fifth, there is a large response to inverse-distance-based spatial spillovers of defense, consistent with the findings in a recent NATO study (George and Sandler Citationforthcoming). In Tables and , alternative contiguity spillover models identify free riding and a lack of threat among immediate and nearby neighbors. Sixth, for the spatial models, population is a negative determinant of defense spending, consistent with population-driven competing civilian goods demand. Seventh, the United States presence in the Asia-Pacific region is providing free-riding opportunities, except for select U.S. allies who are treating their ME as complementary to that of the United States in the SUR representations.
If data for North Korea become available, then an obvious extension is to include it in the spatial analysis. Additional countries – e.g. Afghanistan and Iran – can be included in future studies. In all regional analyses, there is always the question as to where to draw the line in terms of included countries.
Disclosure statement
No potential conflict of interest was reported by the authors.
Funding
This work was supported by the Vibhooti Shukla Endowment.
Supplemental data
Supplemental data for this article can be accessed here https://doi.org/10.1080/10242694.2018.1434375.
Supplemental_material.docx
Download MS Word (18.3 KB)Acknowledgments
The authors gratefully acknowledge extremely helpful comments from two anonymous reviewers and Binyam Solomon on an earlier draft.
Notes
1. Primary measures are ME or the share of GDP devoted to ME (i.e. ME/GDP).
2. In this paragraph, the ME data are from Stockholm International Peace Research Institute (SIPRI Citation2017), while the GDP data are from World Bank (Citation2016).
3. The U.S. alliance with New Zealand ended in 1986. The United States is still formally allied with the other five countries.
4. These facts come from UCDP/PRIO (2017).
6. Skogstad (Citation2016) puts forward a novel power-projection connectivity matrix based on countries’ air, land, and sea capabilities surpassing set thresholds.
7. With this alternative representative for spillovers, the social welfare function could be written as , or some variant, where q
i
and Q
−i1 are summed if country i is allied with group 1 but not with group 2, i.e.
.
8. Nevertheless, Solomon’s (Citation2005) inclusion of relative prices has a minimal effect on his long-run demand estimates.
9. Also, the fact that SIPRI does not publish Chinese ME data before 1989 due to methodological reasons contributed to the selection of this particular period. For more details on the calculation of Chinese ME, see ‘SIPRI Military Expenditure Database Sources and Methods,’ available at https://www.sipri.org/databases/milex/sources-and-methods#sipri-estimates-for-china.
10. Flores (Citation2011) also use 8000 km as cut-off distances for his global sample. However, since we use a much smaller set of sample countries for our analysis, employing such a cut-off point means a very sparse weight matrix leading to estimation problems.
11. Out of nineteen sample countries used in the SAR and FCSL models, eight were omitted from the SUR regressions due to singularities in the variance-covariance matrix or incomplete data. In SUR regressions, a singular variance error matrix is typical of some systems containing a large number of individual equations. A common procedure for handling the singularity problem is to drop troublesome equations and estimate the remaining equations (Takada, Ullah, and Chen Citation1995).
12. See Murdoch and Sandler (Citation1984) and Sandler (Citation1977) on complementarity and spillovers.
References
- Baum, Christopher F., Mark E. Schaffer, and Steven Stillman. 2003. “Instrumental Variables and GMM: Estimation and Testing.” The Stata Journal 3 (1): 1–31.
- Baum, Christopher F., Mark E. Schaffer, and Steven Stillman. 2007. “Enhanced Routines for Instrumental Variables/Generalized Method of Moments Estimation and Testing.” The Stata Journal 7 (4): 465–506.
- Bruce, Neil. 1990. “Defence Expenditures by Countries in Allied and Adversarial Relationships.” Defence Economics 1 (3): 179–195.10.1080/10430719008404661
- Center for Systemic Peace. 2017. The Polity Project. Accessed 1 October 2017. www.systemicpeace.org/polityproject.html.
- Christie, Edward Hunter. Forthcoming. “The Demand for Military Expenditure in Europe: The Role of Fiscal Space in the Context of a Resurgent Russia.” Defence and Peace Economics. doi:10.1080/10242694.2017.1373542.
- Correlates of War (COW) Project. 2006. Direct Contiguity Data, 1816–2006, Version 3.1. Accessed 30 September 2017. http://www.correlatesofwar.org.
- Correlates of War (COW) Project. 2013. Formal Alliances Data, 1816–2012, Version V4.1. Accessed 30 September 2017. http://www.correlatesofwar.org.
- Council of Foreign Relations. 2017. Global Conflict Tracker. Accessed 30 September 2017. https://www.cfr.org/interactives/global-conflict-tracker#1/conflict/.
- Dmochowski, Tadeusz. 2015. “The Settlement of the Russian–Chinese Border Dispute.” Polish Political Science Yearbook 44: 56–74.
- Douch, Mohamed, and Binyam Solomon. 2014. “Middle Powers and the Demand for Military Expenditures.” Defence and Peace Economics 25 (6): 605–618.10.1080/10242694.2013.861652
- Dudley, Leonard, and Claude Montmarquette. 1981. “The Demand for Military Expenditures: An International Comparison.” Public Choice 37 (1): 5–31.10.1007/BF00124229
- Dunne, J. Paul, and Sam Perlo-Freeman. 2003. “The Demand for Military Spending in Developing Countries.” International Review of Applied Economics 17 (1): 23–48.10.1080/713673166
- Elhorst, J. Paul. 2003. “Specification and Estimation of Spatial Panel Data Models.” International Regional Science Review 26 (3): 244–268.10.1177/0160017603253791
- Flores, Alexandro Q. 2011. “Alliances as Contiguity in Spatial Models of Military Expenditures.” Conflict Management and Peace Science 28 (4): 402–418.10.1177/0738894211413064
- George, Justin, and Todd Sandler. Forthcoming. “Demand for Military Spending in NATO, 1968–2015: A Spatial Panel Approach.” European Journal of Political Economy. doi:10.1016/j.ejpoleco.2017.09.002.
- Gibler, Douglas M. 2009. International Military Alliances, 1648–2008. Thousand Oaks, CA: CQ Press.10.4135/9781604265781
- Gleditsch, Kristian S., and Michael D. Ward. 2001. “Measuring Space: A Minimum-distance Database and Applications to International Studies.” Journal of Peace Research 38 (6): 739–758.10.1177/0022343301038006006
- Goldsmith, Benjamin E. 2007. “Arms Racing in ‘Space’: Spatial Modelling of Military Spending Around the World.” Australian Journal of Political Science 42 (3): 419–440.10.1080/10361140701513562
- Greene, William H. 2012. Econometric Analysis. 7th ed. Upper Saddle River, NJ: Prentice Hall.
- Hartley, Keith, and Todd Sandler, eds. 1990. The Economics of Defence: An International Survey. London: Routledge.
- Hilton, Brian, and Anh Vu. 1991. “The McGuire Model and the Economics of the NATO Alliance.” Defence Economics 2 (2): 105–121.10.1080/10430719108404684
- Horesh, Niv, Hyunjin Kim, Peter Mauch, and Jonathan Sullivan. 2014. “Is My Rival’s Rival a Friend? Popular Third-party Perceptions of Territorial Disputes in East Asia.” The Copenhagen Journal of Asian Studies 32 (1): 5–25.10.22439/cjas.v32i1.4594
- Kelejian, Harry H., and Ingmar R. Prucha. 1998. “A Generalized Spatial Two-stage Least Squares Procedure for Estimating a Spatial Autoregressive Disturbance Model with Autoregressive Disturbances.” The Journal of Real Estate Finance and Economics 17 (1): 99–121.10.1023/A:1007707430416
- Kollias, Christos. 2008. “A Preliminary Investigation of the Burden Sharing Aspects of a European Union Common Defence Policy.” Defence and Peace Economics 19 (4): 253–263.10.1080/10242690802164777
- Lee, Seokwoo. 2002. Territorial Disputes among Japan, China and Taiwan Concerning the Senkaku Islands. Vol. 3. Durham: International Boundaries Research Unit, Boundary and Territorial Briefings. Accessed 30 September 2017. https://www.dur.ac.uk/ibru/publications/view/?id=222.
- Markowski, Stefan, Satish Chand, and Robert Wylie. 2017. “Economic Growth and Demand for Military Expenditure in the Indo-Pacific Asia Region.” Defence and Peace Economics 28 (4): 473–490.10.1080/10242694.2016.1274059
- McGuire, Martin C., and Carl H. Groth. 1985. “A Method for Identifying the Public Good Allocation Process within a Group.” The Quarterly Journal of Economics 100 (Supplement): 915–934.10.1093/qje/100.Supplement.915
- Murdoch, James C. 1995. “Military Alliances: Theory and Empirics.” In Handbook of Defense Economics, vol. 1, edited by Keith Hartley, and Todd Sandler, 89–108. Amsterdam: North-Holland.10.1016/S1574-0013(05)80007-9
- Murdoch, James C., and Todd Sandler. 1982. “A Theoretical and Empirical Analysis of NATO.” Journal of Conflict Resolution 26 (2): 237–263.10.1177/0022002782026002003
- Murdoch, James C., and Todd Sandler. 1984. “Complementarity, Free Riding, and the Military Expenditures of NATO Allies.” Journal of Public Economics 25 (1–2): 83–101.10.1016/0047-2727(84)90045-8
- Murdoch, James C., and Todd Sandler. 1985. “Australian Demand For Military Expenditures: 1961–1979.” Australian Economic Papers 24 (44): 142–153.10.1111/j.1467-8454.1985.tb00101.x
- Nikolaidou, Eftychia. 2008. “The Demand for Military Expenditure: Evidence From The EU15 (1961–2005).” Defence and Peace Economics 19 (4): 273–292.10.1080/10242690802166533
- Olson, Mancur, and Richard Zeckhauser. 1966. “An Economic Theory of Alliances.” The Review of Economics and Statistics 48 (3): 266–279.10.2307/1927082
- Sandler, Todd. 1977. “Impurity of Defense: An Application to the Economics of Alliances.” Kyklos 30 (3): 443–460.10.1111/kykl.1977.30.issue-3
- Sandler, Todd, and Justin George. 2016. “Military Expenditure Trends for 1960–2014 and What They Reveal.” Global Policy 7 (2): 174–184.10.1111/1758-5899.12328
- Sandler, Todd, and Keith Hartley. 1995. The Economics of Defense. Cambridge: Cambridge University Press.
- Sandler, Todd, and Keith Hartley. 2001. “Economics of Alliances: The Lessons for Collective Action.” Journal of Economic Literature 39 (3): 869–896.10.1257/jel.39.3.869
- Sandler, Todd, and James C. Murdoch. 1990. “Nash-Cournot or Lindahl Behavior? An Empirical Test for the Nato Allies.” The Quarterly Journal of Economics 105 (4): 875–894.10.2307/2937877
- Skogstad, Karl. 2016. “Defence Budgets in the Post-cold War Era: A Spatial Econometrics Approach.” Defence and Peace Economics 27 (3): 323–352.10.1080/10242694.2015.1034911
- Smith, Ron. 1980. “The Demand for Military Expenditure.” The Economic Journal 90 (360): 811–820.10.2307/2231744
- Smith, Ron. 1990. “Defence Spending in the United Kingdom.” In The Economics of Defense Spending, edited by Keith Hartley, and Todd Sandler, 76–92. London: Routledge.
- Smith, Ron. 1995. “The Demand for Military Expenditure.” In Handbook of Defense Economics, vol. 1, edited by Keith Hartley, and Todd Sandler, 69–87. Amsterdam: North-Holland.10.1016/S1574-0013(05)80006-7
- Solomon, Binyam. 2005. “The Demand for Canadian Defence Expenditures.” Defence and Peace Economics 16 (3): 171–189.10.1080/10242690500123380
- Stinnett, Douglas M., Jaroslav Tir, Philip Schafer, Paul F. Diehl, and Charles Gochman. 2002. “The Correlates of War Project Direct Contiguity Data, Version 3.” Conflict Management and Peace Science 19 (2): 58–66.
- Stockholm International Peace Research Institute. 1983. SIPRI Yearbook, Stockholm International Peace Research Institute. New York: Oxford University Press.
- Stockholm International Peace Research Institute. 2017. SIPRI Extended Military Expenditure Database. Accessed 30 September 2017. http://www.sipri.org/databases/milex.
- Takada, Hirokazu, Aman Ullah, and Yu-Min Chen. 1995. “Estimation of the Seemingly Unrelated Regression Model When the Error Covariance Matrix is Singular.” Journal of Applied Statistics 22 (4): 517–530.10.1080/757584788
- Uppsala Conflict Data Program/Peace Research Institute Oslo. 2017. Armed Conflict Dataset, Version 17.1. Accessed 30 September 2017. http://ucdp.uu.se/downloads/.
- Wang, Yu. 2013. “Determinants of Southeast Asian Military Spending in the Post-cold War Era: A Dynamic Panel Analysis.” Defence and Peace Economics 24 (1): 73–87.10.1080/10242694.2012.656944
- World Bank. 2016. World Development Indicators (WDI). Accessed 30 September 2017. http://data.worldbank.org/data-catalog/world-development-indicators.
- Yesilyurt, M. Ensar, and J. Paul Elhorst. 2017. “Impacts of Neighboring Countries on Military Expenditures: A Dynamic Spatial Panel Approach.” Journal of Peace Research 54 (6): 777–790.10.1177/0022343317707569
- Zellner, Arnold. 1962. “An Efficient Method of Estimating Seemingly Unrelated Regressions and Tests for Aggregation Bias.” Journal of the American Statistical Association 57 (298): 348–368.10.1080/01621459.1962.10480664