ABSTRACT
Introduction: Several studies have evaluated the association between the multidrug resistance 1 (MDR1) polymorphism (rs1045642 C > T) and multiple myeloma (MM). However, the results were not consistent. Therefore, to reach a comprehensive and reliable answer we determined the association of the MDR1 (rs1045642 C > T) polymorphism and MM in the context of meta-analysis.
Methods: All eligible studies published in EMBASE, PubMed, and Web of Science databases before July 2017 were reviewed. Subsequently, to assess the strength of association in the dominant model, recessive model, allelic model, homozygotes contrast, and heterozygotes contrast, pooled odds ratios and 95% confidence intervals (CIs) were calculated by the fixed effects model.
Results: A total of four case–control studies with 395 MM cases and 418 healthy controls were included in the meta-analysis. The overall results showed no significant association between the MDR1 (rs1045642 C > T) polymorphism and the risk of MM in genetic models (dominant model: OR = 1.04, 95% CI = 0.78–1.38; recessive model: OR = 0.74, 95% CI = 0.52–1.06; allelic model: OR = 0.90, 95% CI = 0.73–1.11; TT vs. CC: OR = 0.80, 95% CI = 0.51–1.25; and CT vs. CC: OR = 1.12, 95% CI = 0.77–1.62). No evidence of publication bias was detected except for the analysis of the recessive model.
Conclusion: This meta-analysis suggests that the MDR1 C > T polymorphism was not associated with the risk of MM. To confirm these findings, further comprehensive and well-designed studies are needed.
1. Introduction
Multiple myeloma (MM) is a lymphoproliferative disease of B lymphocyte origin, which is known as plasma cell myeloma, myelomatosis, or Kahler disease [Citation1]. MM is characterized by high-speed growth of monoclonal plasma cells, which leads to mass production of malfunction immunoglobulin (Ig) chains. Subsequently, accumulation and interaction of these abnormal immunoglobulins and monoclonal plasma cells with other cells in bone marrow culminated in major pathologic problems including bone lesion, infection, and kidney failure [Citation2,Citation3]. Several risk factors increase the incidence of MM, such as older age, male sex, positive family history, black race, and genetic factors [Citation4,Citation5]. From an epidemiologic point of view, approximately 10% of hematologic malignancies and 1% of all malignant diseases can be classified as MM [Citation6,Citation7]. Regarding MM treatment, common drugs including alkylating agents, monoclonal antibodies, and others have been reported to be promising, but some patients are intrinsically resistant or finally acquire resistance to various anticancer drugs. The most common justification for refractoriness is the multidrug resistance (MDR) phenomenon [Citation8–10]. P-glycoprotein or the ATP-binding cassette transporter B1 (ABCB1) is encoded by the multidrug resistance 1 (MDR1) gene, which is located on chromosome 7q21 [Citation11]. Some members of the human ABC transporters such as P-Glycoprotein simplify the efflux of various chemotherapeutic drugs and confer the MDR phenomenon on cancer cells [Citation12]. Although P-glycoprotein expression on the plasma membrane and Golgi membrane of normal tissues, such as kidney, liver, placenta, and others, is common, it is significantly overexpressed in drug-resistant tumors and results in ineffectiveness of anticancer drugs [Citation13–15].
The importance of genetic factors as predisposition agents for cancers led to conducting of extensive molecular studies, which resulted in the identification of numerous single nucleotide polymorphisms (SNPs) in different cancers. Among the identified polymorphisms in MM, the SNP 3435C > T in exon 26 is well known. This SNP modifies P-glycoprotein expression and function [Citation16,Citation17]. This meta-analysis was conducted to find out whether or not the MDR1 polymorphism 3435C > T affects susceptibility to MM.
2. Methods
This meta-analysis was performed based on the Preferred Reporting Items for Systematic reviews and Meta-Analyses (PRISMA) guidelines, including Searches and data sources, study selection, inclusion and exclusion criteria, data extraction and quality assessment, and statistical analysis [Citation18].
2.1. Searches and data sources
All publications reporting the association between the 3435C > T polymorphism at the MDR1 gene and susceptibility to MM published before July 2017 were retrieved by comprehensive electronic search of EMBASE, PubMed, and Web of Science databases without any restriction of publication language. The following search algorithm was applied: (‘Multiple myeloma’ OR myeloma OR MM OR ‘plasma cell myeloma’ OR myelomatosis OR ‘kahler disease’) AND (‘P-glycoprotein 1’ OR ‘multidrug resistance protein1’ OR MRD1 OR ABCB1 OR CD243) AND (polymorphism OR genotype OR SNP OR ‘single nucleotide polymorphisms’). In addition, the reference lists of eligible studies and related publications were searched manually to identify potential publications that were not covered by the electronic search.
2.2. Study selection
After removing duplicate records, the titles and abstracts of all articles identified in the initial search were independently screened by two reviewers to determine whether to include or exclude the studies. Any disagreements between reviewers were resolved by consensus.
2.3. Inclusion and exclusion criteria
All candidate articles were considered eligible for the meta-analysis if they met following criteria: (1) case–control studies; (2) studies that assessed the association between MRD1 3435C > T polymorphism and risk of MM and; (3) studies with clear reports of genotype frequency for the 3435C > T polymorphism in cases and controls. Book chapters, reviews, and studies that examined the influence of certain drugs on MM patients without control group, letters to the editor, case reports, animal studies, or studies with overlapping data were all excluded. Application of these major criteria yielded four eligible case–control studies for the meta-analysis.
2.4. Data extraction and quality assessment
The pre-designed data extraction form for qualified articles had the following items: the first author’s name, journal and year of publication, ethnicity, country of origin, gender, study design, mean or range of age, method of determining genotype, sample size of cases and controls, and the number of cases and controls for each genotype. Two reviewers independently extracted data; subsequently, any disagreement was discussed and resolved by consensus. To assess the methodological quality of included studies The Newcastle–Ottawa Scale was used [Citation19]. According to the quality assessment tools studies that scored 0–3, 4–6, or 7–9 indicated low-, moderate-, or high-quality studies, respectively.
2.5. Statistical analysis
Deviation from the Hardy–Weinberg equilibrium (HWE) was analyzed by the χ2-test in control groups [Citation20]. The strength of association between the MM susceptibility and the SNP in the MDR1 3435C > T gene was accessed by computing odds ratios (ORs) and 95% confidence intervals (CIs) on forest plots under the dominant model (TT + CT vs. CC), recessive model (TT vs. CT + CC), allelic model (T vs. C), homozygote comparison (TT vs. CC), and heterozygote comparison (CT vs. CC). Details regarding the definition of genetic models have been reported previously [Citation21,Citation22]. The heterogeneity effect was evaluated by applying Cochran Q and the I2 statistics, ranging from 0% to 100% (I2<25%, no heterogeneity; I2 = 25–50%, moderate heterogeneity; I2 = 50–75%, large heterogeneity, I2 > 75%, extreme heterogeneity) [Citation23]. I2 > 50% or Q statistic with P < 0.1 was regarded as the presence of significant heterogeneity among the included studies. In the presence of heterogeneity, the random-effects model (Der Simonian–Laird approach) was utilized. Otherwise, the fixed-effect model (Mantel–Haenszel approach) was performed (Q statistic P > 0.1 or I2<50%). Publication bias was assessed by Begg’s tests (P < 0.05 was considered as statistical significance) [Citation24,Citation25]. The data analysis was performed by STATA (version 14.0; Stata Corporation, College Station, TX, U.S.A.) and SPSS (version 23.0; SPSS, Inc. Chicago, IL, U.S.A.).
3. Results
3.1. Study characteristics
outlines the including/excluding procedure of potential studies in a flowchart based on PRISMA guidelines [Citation26]. A total of 98 studies were examined, and eventually according to the inclusion and exclusion criteria 4 eligible studies with 398 cases and 418 healthy subjects were included in this meta-analysis. The studies were conducted in different countries including Poland [Citation27], North Ireland [Citation28], China [Citation29], and Turkey [Citation30]. All eligible studies had an overall good methodological quality with an overall score ranging from 7 to 9. Except for one study [Citation29], in others, the RFLP (Restriction Fragment Length Polymorphism) genotyping platform was applied for genotyping assessment. The baseline characteristics and the allele and genotype distributions of all included studies are reported in and .
Table 1. Characteristics of studies included in the meta-analysis.
Table 2. Distribution of genotype and allele among MM patients and controls.
3.2. Quantitative synthesis
3.2.1. Meta-analysis for the MDR1 3435 C > T polymorphism and MM
We analyzed all included studies on the association between the MDR1 3435 C > T polymorphism and MM risk. The summarized results of the ORs and 95% CIs in different genetic models and heterogeneity tests are reported in . The statistical analysis revealed a lack of significant association between the MDR1 3435 C > T polymorphism and MM risk in all the genetic models including the dominant model (OR = 1.04, 95% CI = 0.78–1.38); recessive model (OR = 0.74, 95% CI = 0.52–1.06); allelic model (OR = 0.90, 95% CI = 0.73–1.11); TT vs. CC (OR = 0.80, 95% CI = 0.51–1.25); and CT vs. CC (OR = 1.12, 95% CI = 0.77–1.62). The forest plots of the meta-analysis for different genetic models are reported in supplemental –.
Figure 2. Forest plot of association between MDR1 (rs1045642 C > T) SNP and MM risk under the dominant model.
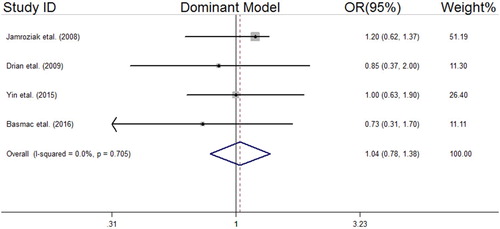
Figure 3. Forest plot of association between MDR1 (rs1045642 C > T) SNP and MM risk under the recessive model.
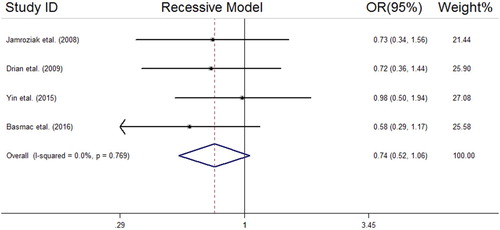
Figure 4. Forest plot of association between MDR1 (rs1045642 C > T) SNP and MM risk under the allelic model.
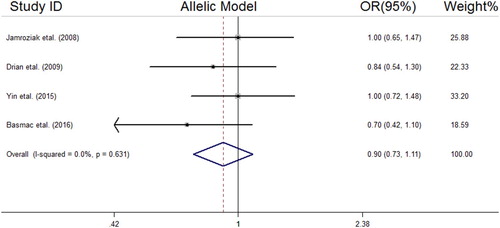
Figure 5. Forest plot of association between MDR1 (rs1045642 C > T) SNP and MM risk under CT vs. CC model.
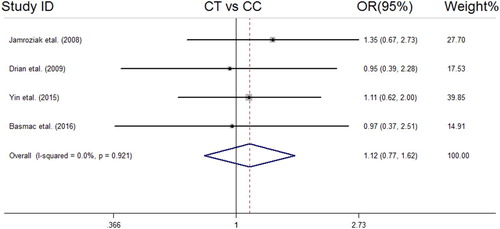
Figure 6. Forest plot of association between MDR1 (rs1045642 C > T) SNP and MM risk under TT vs. CC model.
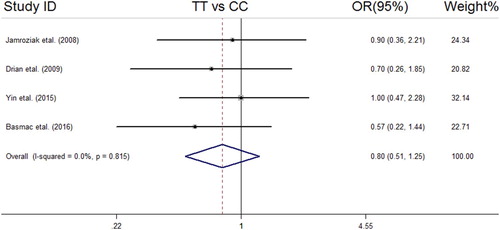
Table 3. Main results of pooled ORs in meta-analysis of MDR1 (rs1045642) polymorphism and MM risk.
3.2.2. Evolution of publication bias and sensitivity analysis
The sensitivity analysis showed reliability of our meta-analysis after removing non-HWE studies [Citation30]. Except for the recessive model (Begg’s test; z = −2.04, P = 0.04), no evidence of publication bias was detected in the other genetics models ().
4. Discussion
The association of the MDR1 3435 C > T polymorphism with MM is a controversial subject. Although recently some studies have investigated this association, published data are still inconclusive. This meta-analysis, which was done on 4 studies with 395 subjects, examined the relationship between the MDR1 3435 C > T polymorphism and susceptibility to MM. Our findings unveiled that there was no significant association between the MDR1 3435 C > T polymorphisms with MM risk in different genetic models.
Since P-glycoprotein acts as an efflux pump that protects cells against endogenous and exogenous harmful compounds, especially environmental carcinogens, any MDR1 SNP might alter the P-glycoprotein expression and protein conformation that subsequently culminated in the development of several cancer types including hematologic malignancy as a result of carcinogens’ accumulation in cells [Citation31,Citation32]. Among three common SNPs in MDR1, including 3435C > T, 1236C > T, and 2677G > T/A, unlike the 2677G > T/A polymorphism which is a non-synonymous SNP and causes an amino acid substitution, the 3435C > T and 1236C > T polymorphisms are synonymous SNPs, not causing a change in the amino acid. Theoretically, synonymous SNPs should not alter the function of the affected protein but the MDR1 3435 C > T polymorphism at codon 1142 (Ile1142Ile) leads to several changes in mRNA level and expression and folding of protein [Citation33]. Elevated expression of MDR1 is a disease-related unfavorable prognostic factor which has a significant impact on complete remission. A distinctive explanation for delayed complete remission is effluxing of chemotherapeutic drugs [Citation34].
Thus, according to the mentioned mechanism, the crucial role of the MDR1 3435 C > T polymorphism in various cancers is obvious, as a number of molecular epidemiological studies have shown increased risk of gastric cancer, breast cancer, colorectal cancer, and hematological cancers [Citation35–37]. Two robust meta-analyses [Citation38,Citation39] about the MDR1 3435 C > T polymorphism and susceptibility to cancers including hematological cancer were performed. Wang et al. [Citation38] reported the elevated risk of ALL in patients with the MDR1 3435 C > T polymorphism for the heterozygote model (TT vs. CC: OR = 1.286, 95% CI = 1.123–1.474), whereas Wang et al. [Citation39] found no association between the MDR1 3435 C > T polymorphism and ALL susceptibility. Furthermore, in these studies, the pooled risks estimate for MM in relation to the MDR1 3435 C > T polymorphism was reported in combination with some other rare cancers as one outcome and the separate pooled risk estimate for MM was not reported as our study did.
Although the present meta-analysis did not find associations of the MDR1 3435 C > T polymorphism and the risk of MM, the results should be interpreted with caution. More than 50 SNPs are defined for the MDR1 gene [Citation30], but only the rs1045642 C > T polymorphism has been examined in MM patients. Accordingly, this meta-analysis was limited to one of the most investigated SNPs, and lack of association of rs1045642 C > T with MM in our analysis could not exclude the possible relationship between other SNPs of the MDR1 gene and MM risk. Thus, further studies are needed to be performed in different ethnicities to find a conclusive result regarding the relation of MDR1 polymorphisms to the risk of MM.
Moreover, a small sample size is an important cause of false-negative associations [Citation40]. A sample size with sufficient statistical power is critical to the success of genetic association studies to detect causal genes of human complex diseases and genome-wide association studies require much larger sample sizes to achieve an adequate statistical power. Hong et al. reported that regardless of disease type, disease prevalence, and minor allele frequency (MAF) of SNPs, the power is too low when the sample size is below 100 cases [Citation41]. The sample size required to achieve 80% statistical power in a case–control study under the assumptions of 5% disease prevalence, 5% MAF, complete linkage disequilibrium (D′ = 1), 1:1 case-to-control ratio, and 5% type I error rate (α) is different according to the genetic models. To achieve 80% statistical power, compared to other genetic models, the dominant model requires the smallest sample size, while the effective sample size to test a single SNP under the recessive model is the largest. It reveals difficulty in detecting a disease allele that follows a recessive mode of inheritance with a moderate sample size. The number of cases for the dominant model to achieve 80% power when the heterozygous odds ratio is equal to 1.3, 1.5, 2, and 2.5 is 606, 258, 90, and 53, respectively, whereas the corresponding cases for the recessive model are 20294, 8390, 2776, and 1536, respectively [Citation41]. The total sample size of the 4 included studies in our meta-analysis was 398 cases and 418 control subjects, and the number of cases in 2 studies were lower than 100, indicating that large epidemiological studies are needed to yield a conclusive result about the association between the rs1045642 C > T polymorphism in the MDR1/ABCB1gene and MM risk.
Some limitations of the current study are worth noting. First, the number of eligible studies was restricted. Thus, we were not able to conduct subgroup analysis to evaluate possible discrepancies caused by sex, age, and ethnicity of the participants. Second, a significant evidence of publication bias was observed in the recessive model, indicating that some small studies might be ignored. Third, the included studies were performed in Caucasians, and studies in other ethnicities were not found, which limits the generalizability of our findings.
In conclusion, the present gene-based meta-analysis suggests that the MDR1 3435 C > T polymorphism might not be associated with the risk of MM. To find a more definitive conclusion, by considering the effect of gene–environment and gene–gene interactions, additional large, well-designed epidemiological studies should be done.
Disclosure statement
No potential conflict of interest was reported by the authors.
ORCID
Gholamreza Anani Sarab http://orcid.org/0000-0003-3844-3643
References
- Sabattini E, Bacci F, Sagramoso O, et al. WHO classification of tumours of haematopoietic and lymphoid tissues in 2008: an overview. Pathologica. 2010;102(3):83–87.
- Gerecke C, Fuhrmann S, Strifler S, et al. The diagnosis and treatment of multiple myeloma. Dtsch Ärztebl Int. 2016;113(27–28):470.
- Brigle K, Rogers B. Pathobiology and diagnosis of multiple myeloma. Semin Oncol Nurs. 2017;33(3):225–236. Elsevier. doi: 10.1016/j.soncn.2017.05.012
- Sergentanis TN, Zagouri F, Tsilimidos G, et al. Risk factors for multiple myeloma: A systematic review of meta-analyses. Cl Lymph Myelom Leuk. 2015;15(10):563–577.e3. doi: 10.1016/j.clml.2015.06.003
- Alexander DD, Mink PJ, Adami HO, et al. Multiple myeloma: a review of the epidemiologic literature. Int J Cancer. 2007;120(S12):40–61. doi: 10.1002/ijc.22718
- Angtuaco EJ, Fassas AB, Walker R, et al. Multiple myeloma: clinical review and diagnostic imaging. Radiology. 2004;231(1):11–23. doi: 10.1148/radiol.2311020452
- Bladé J, Kyle RA, Greipp PR. Multiple myeloma in patients younger than 30 years: report of 10 cases and review of the literature. Arch Intern Med. 1996;156(13):1463–1468. doi: 10.1001/archinte.1996.00440120125014
- Tsuruo T, Naito M, Tomida A, et al. Molecular targeting therapy of cancer: drug resistance, apoptosis and survival signal. Cancer Sci. 2003;94(1):15–21. doi: 10.1111/j.1349-7006.2003.tb01345.x
- Reece DE, Leitch HA, Atkins H, et al. Treatment of relapsed and refractory myeloma. Leuk Lymphoma. 2008;49(8):1470–1485. doi: 10.1080/10428190802064941
- Tsubaki M, Satou T, Itoh T, et al. Overexpression of MDR1 and survivin, and decreased Bim expression mediate multidrug-resistance in multiple myeloma cells. Leuk Res. 2012;36(10):1315–1322. doi: 10.1016/j.leukres.2012.07.003
- Linsenmeyer M, Jefferson S, Wolf M, et al. Levels of expression of the mdr1 gene and glutathione S-transferase genes 2 and 3 and response to chemotherapy in multiple myeloma. Br J Cancer. 1992;65(3):471–475. doi: 10.1038/bjc.1992.95
- Landgren O, Kyle RA, Pfeiffer RM, et al. Monoclonal gammopathy of undetermined significance (MGUS) consistently precedes multiple myeloma: a prospective study. Blood. 2009;113(22):5412–5417. doi: 10.1182/blood-2008-12-194241
- Yunis JJ, Frizzera G, Oken MM, et al. Multiple recurrent genomic defects in follicular lymphoma. N Engl J Med. 1987;316(2):79–84. doi: 10.1056/NEJM198701083160204
- Katayama K, Noguchi K, Sugimoto Y. Regulations of P-glycoprotein/ABCB1/MDR1 in human cancer cells. New J Sci. 2014;2014:1–10. doi: 10.1155/2014/476974
- Yasuhisa K, Shin-ya M, Michinori M, et al. Mechanism of multidrug recognition by MDR1/ABCB1. Cancer Sci. 2007;98(9):1303–1310. doi: 10.1111/j.1349-7006.2007.00538.x
- Chen C-j, Chin JE, Ueda K, et al. Internal duplication and homology with bacterial transport proteins in the mdr1 (P-glycoprotein) gene from multidrug-resistant human cells. Cell. 1986;47(3):381–389. doi: 10.1016/0092-8674(86)90595-7
- Kioka N, Tsubota J, Kakehi Y, et al. P-glycoprotein gene (MDR1) cDNA from human adrenal: normal P-glycoprotein carries Gly185 with an altered pattern of multidrug resistance. Biochem Biophys Res Commun. 1989;162(1):224–231. doi: 10.1016/0006-291X(89)91985-2
- Liberati A, Altman DG, Tetzlaff J, et al. The PRISMA statement for reporting systematic reviews and meta-analyses of studies that evaluate health care interventions: explanation and elaboration. PLoS Med. 2009;6(7):e1000100. doi: 10.1371/journal.pmed.1000100
- Stang A. Critical evaluation of the Newcastle-Ottawa scale for the assessment of the quality of nonrandomized studies in meta-analyses. Eur J Epidemiol. 2010;25(9):603–605. doi: 10.1007/s10654-010-9491-z
- Wigginton JE, Cutler DJ, Abecasis GR. A note on exact tests of Hardy-Weinberg equilibrium. Am J Hum Genet. 2005;76(5):887–893. doi: 10.1086/429864
- Horita N, Kaneko T. Genetic model selection for a case–control study and a meta-analysis. Meta Gene. 2015;5:1–8. doi: 10.1016/j.mgene.2015.04.003
- Clarke GM, Anderson CA, Pettersson FH, et al. Basic statistical analysis in genetic case-control studies. Nat Protoc. 2011;6(2):121–133. doi: 10.1038/nprot.2010.182
- Huedo-Medina TB, Sánchez-Meca J, Marín-Martínez F, et al. Assessing heterogeneity in meta-analysis: Q statistic or I² index? Psychol Methods. 2006;11(2):193–206. doi: 10.1037/1082-989X.11.2.193
- Egger M, Smith GD, Schneider M, et al. Bias in meta-analysis detected by a simple, graphical test. Br Med J. 1997;315(7109):629–634. doi: 10.1136/bmj.315.7109.629
- Begg CB, Mazumdar M. Operating characteristics of a rank correlation test for publication bias. Biometrics. 1994;50:1088–1101. doi: 10.2307/2533446
- Moher D, Liberati A, Tetzlaff J, et al. Preferred reporting items for systematic reviews and meta-analyses: the PRISMA statement. PLoS Med. 2009;6(7):e1000097. doi: 10.1371/journal.pmed.1000097
- Jamroziak K, Balcerczak E, Calka K, et al. Polymorphisms and haplotypes in the multidrug resistance 1 gene (MDR1/ABCB1) and risk of multiple myeloma. Leuk Res. 2009;33(2):332–335. doi: 10.1016/j.leukres.2008.06.008
- Drain S, Catherwood MA, Orr N, et al. ABCB1 (MDR1) rs1045642 is associated with increased overall survival in plasma cell myeloma. Leuk Lymphoma. 2009;50(4):566–570. doi: 10.1080/10428190902853144
- Yin G, Xiao Z, Ni Y, et al. Association of MDR1 single-nucleotide polymorphisms and haplotype variants with multiple myeloma in Chinese Jiangsu Han population. Tumor Biol. 2016;37(7):9549–9554. doi: 10.1007/s13277-015-4574-9
- Basmaci C, Pehlivan M, Tomatir A, et al. Effects of TNFα, NOS3, MDR1 gene polymorphisms on clinical parameters, prognosis and survival of multiple myeloma cases. Asian Pac J Cancer Prev. 2016;17(3):1009–1014. doi: 10.7314/APJCP.2016.17.3.1009
- Yue Q, Xiong B, Chen L, et al. MDR1 c3435 T polymorphism and childhood acute lymphoblastic leukemia susceptibility: an updated meta-analysis. Biomed Pharmacother. 2015;69:76–81. doi: 10.1016/j.biopha.2014.10.015
- Ma L, Liu H, Ruan L, et al. Multidrug resistance gene 1 C1236 T polymorphism and susceptibility to leukemia: a meta-analysis. Biomed Rep. 2015;3(1):83–87. doi: 10.3892/br.2014.387
- Ozdemir S, Uludag A, Silan F, et al. Possible roles of the xenobiotic transporter P-glycoproteins encoded by the MDR1 3435 C > T gene polymorphism in differentiated thyroid cancers. Asian Pac J Cancer Prev. 2013;14(5):3213–3217. doi: 10.7314/APJCP.2013.14.5.3213
- Hartmann G, Kim H, Piquette-Miller M. Regulation of the hepatic multidrug resistance gene expression by endotoxin and inflammatory cytokines in mice. Int Immunopharmacol. 2001;1(2):189–199. doi: 10.1016/S0162-3109(00)00271-X
- Mutoh K, Mitsuhashi J, Kimura Y, et al. A T3587G germ-line mutation of the MDR1 gene encodes a nonfunctional P-glycoprotein. Mol Cancer Ther. 2006;5(4):877–884. doi: 10.1158/1535-7163.MCT-05-0240
- Taheri M, Mahjoubi F, Omranipour R. Effect of MDR1 polymorphism on multidrug resistance expression in breast cancer patients. Genet Mol Res. 2010;9(1):34–40. doi: 10.4238/vol9-1gmr669
- Khedri A, Nejat-Shokouhi A, Salek R, et al. Association of the colorectal cancer and MDR1 gene polymorphism in an Iranian population. Mol Biol Rep. 2011;38(5):2939–2943. doi: 10.1007/s11033-010-9957-9
- Wang L-H, Song Y-B, Zheng W-L, et al. The association between polymorphisms in the MDR1 gene and risk of cancer: a systematic review and pooled analysis of 52 case–control studies. Cancer Cell Int. 2013;13(1):46. doi: 10.1186/1475-2867-13-46
- Wang J, Wang B, Bi J, et al. MDR1 gene C3435 T polymorphism and cancer risk: a meta-analysis of 34 case–control studies. J Cancer Res Clin Oncol. 2012;138(6):979–989. doi: 10.1007/s00432-012-1171-9
- Pruissen DMO, Kappelle LJ, Rosendaal FR, et al. Genetic association studies in ischaemic stroke. Cerebrovasc Dis. 2009;27(3):290–294. doi: 10.1159/000199467
- Hong EP, Park JW. Sample size and statistical power calculation in genetic association studies. Genomics Inform. 2012;10(2):117–122. doi: 10.5808/GI.2012.10.2.117