Abstract
Resting heart rate variability (HRV), a surrogate index of cardiac vagal modulation, is considered a putative biomarker of stress resilience as it reflects the ability to effectively regulate emotions in a changing environment. However, most studies are cross-sectional, precluding longitudinal inferences. The high degree of uncertainty and fear at a global level that characterizes the COVID-19 pandemic offers a unique opportunity to explore the utility of HRV measures as longitudinal predictors of stress resilience. This study examined whether resting measures of HRV prior to the COVID-19 outbreak (i.e. nearly 2 years before; Time 0) could predict emotion regulation strategies and daily affect in healthy adults during the May 2020 lockdown (Time 1). Moreover, we evaluated the association between HRV measures, emotion regulation strategies, subjective perception of COVID-19 risk, and self-reported depressive symptoms at Time 1. Higher resting HRV at Time 0 predicted a stronger engagement in more functional emotion regulation strategies, as well as of higher daily feelings of safeness and reduced daily worry at Time 1. Moreover, depressive symptoms negatively correlated with HRV and positively correlated with the subjective perception of COVID-19 risk at Time 1. Current data support the view that HRV might not only be a marker but also a precursor of resilience under stressful times.
Introduction
On 11 March 2020, the COVID-19 outbreak was declared a global pandemic, and almost all countries in the world have introduced national lockdowns. Psychosocial efforts to resist the lockdown situation first and then overcome the negative consequences of the pandemic have caused mental health problems and continue to challenge vulnerable individuals (Rajkumar, Citation2020). Indeed, the identification of risk, vulnerability, and protective factors for mental health has been recognized as a research priority (Holmes et al., Citation2020).
In this regard, resting vagally-mediated heart rate variability (vmHRV), a surrogate index of cardiac vagal modulation, has been indicated as a putative biomarker of stress resilience, as it reflects the ability to effectively regulate emotions in a changing environment (Carnevali et al., Citation2018; Thayer et al., Citation2012). Unfortunately, the majority of such investigations is cross-sectional and longitudinal studies on vmHRV as a predictor of stress resilience are sparse. For example, preliminary studies have shown that lower HRV before or in the aftermath of a traumatic experience predicts the development of PTSD symptoms in adults (reviewed in (Carnevali et al., Citation2018)). To the best of our knowledge, the association between vmHRV and COVID-19-related stress was examined only in one study, in which changes in HRV parameters during and after the lockdown period appeared to be in line with subjective well-being (Bourdillon et al., Citation2020). Moreover, in a study conducted on U.S. residents, autonomic reactivity measured using a self-report questionnaire mediated the relation between past traumatic experiences and mental health indices during the COVID-19 pandemic (Kolacz et al., Citation2020). However, a recent study in adolescents found that susceptibility to mental health difficulties associated with COVID-19 stress was predicted by higher resting vmHRV assessed four years earlier (Miller et al., Citation2021), which is inconsistent with cross-sectional and longitudinal findings in adults.
The present investigation aimed at extending previous findings, with the hypothesis that higher resting vmHRV, assessed ∼22 months earlier, would predict the use of more functional emotion regulation strategies and daily affective states in healthy adults during COVID-19-related forced lockdown. Furthermore, we tested the cross-sectional relationship between vmHRV, depressive symptomatology, and subjective perception of COVID-19 risk during the lockdown.
Methods
Procedure
One-hundred and seventy-two healthy individuals, whose resting vmHRV was assessed between May 2018 and October 2019 (Time 0; T0) during participation in previous studies (Carnevali et al., Citation2020; Di Bello et al., Citation2021; Sgoifo et al., Citation2021), were contacted. After the exclusion of two participants with current diagnosis of medical disorders, a total of 69 individuals were considered for this investigation, which includes data collected during national lockdowns in Italy and United Kingdom from 1 to 31 May 2020 (Time 1; T1) (ClinicalTrials.gov identification code: NCT04382560). Participants provided informed consent, and the study was approved by the IRBs of King’s College London (LRS-19/20-18429:COVID-19) and Sapienza University of Rome (Prot. 0000653).
Initially, participants filled out online questionnaires including (i) the Perceived Coronavirus Risk scale (PCRS; (Kanovsky & Halamova, Citation2020)), which measures the subjective perception of COVID-19 risk; (ii) the Center for Epidemiological Studies Depression Scale (CES-D; (Radloff, Citation1977)), which assesses self-reported depressive symptoms experienced in the previous week; and (iii) the 10-item Emotion Regulation Questionnaire (ERQ; (Spaapen et al., Citation2014)), which measures the habitual use of several emotion regulation strategies. Among them, this study focused on cognitive reappraisal and emotional suppression as examples of functional and dysfunctional strategies, respectively. Next, participants were asked to download a freely available smartphone application (https://ecg4everybody.com/) and instructed to record vmHRV using finger tip photoplethysmography for 2 min under resting conditions. Specifically, they were asked to refrain from drinking coffee and alcholic and energizing drinks, eating a meal, smoking, and physical exercising for at least two hours prior to the recording, similar to the T0 assessment. Subsequently, participants were asked to rate their current levels of feeling anxious, sad, worried, optimistic, and safe on 5-point Likert scales every 2 h during wake for 2 consecutive days, using electronic diaries delivered by Qualtrics.
Analyses
The root mean square of successive differences between normal heartbeats (RMSSD) was considered as an index of vmHRV (Laborde et al., Citation2017). This time-domain index reflects the beat-to-beat variance in heart rate (or pulse interval) and is less affected by respiratory influences compared to frequency-domain indexes (Laborde et al., Citation2017). At T0, vmHRV was measured from electrocardiographic or plethysmographic signals recorded during 3 − 5 min resting conditions (see Carnevali et al., Citation2020; Di Bello et al., Citation2021; Sgoifo et al., Citation2021 for additional information). In order to obtain comparable measures of vmHRV, all interbeat intervals obtained from the original 3 − 5 min recordings were re-analyzed using the Kubios HRV software (Tarvainen et al., Citation2014). Artifacts and ectopic beats were corrected using a threshold-based correction. At T1, resting vmHRV data were automatically generated by the smartphone application without any further processing.
Initially, Pearson’s correlations and t-tests were performed to investigate the influence of age, sex, lifestyle factors (smoking), and recording procedures on vmHRV values at T0. We accounted for non-normally distributed vmHRV values (p < .01) by calculating their natural logarithm (ln(vmHRV)).
First, linear regressions were performed to evaluate whether ln(vmHRV) values at T0 would predict ERQ reappraisal and suppression scores at T1. Data obtained by daily ecological momentary assessment consist of repeated measures; therefore, random effects mixed regression models were applied to test whether ln(vmHRV) values at T0 would predict momentary mood ratings at T1. The restricted maximum likelihood method was used for model estimation. The covariance model among observations within subject was a random intercept plus autoregressive model. Random slopes were not estimated. To control for potential confounders, months elapsed between the two assessments, sex, age, BMI, and smoking status were included as predictors in all the regression models.
Lastly, Pearson’s correlations were computed between ln(vmHRV) at T0 and T1, and ln(vmHRV), CESD, PCRS, and ERQ scores at T1, controlling for the effects of age and sex. Statistics were performed with SPSS version 25 (SPSS Inc., Chicago, IL) with statistical significance set at p ≤ .05.
Results
The final sample size, excluding incomplete responses (n = 3), was n = 66 (). No significant associations emerged between baseline participants’ demographic, anthropometric, and lifestyle characteristics and ln(vmHRV) at T0.
Table 1. Participants’ characteristics (n = 66).
As shown in and , ln(vmHRV) at T0 positively predicted the use of cognitive reappraisal at T1, controlling for potential confounders. On the other hand, ln(vmHRV) at T0 was not a significant predictor of emotional suppression at T1.
Figure 1. Scatterplot of the relationship between vagally-mediated heart rate variability ln(vmHRV) values at Time 0 (T0) and scores on cognitive reappraisal subscale of the Emotion Regulation Questionnaire (ERQ) at Time 1 (T1).
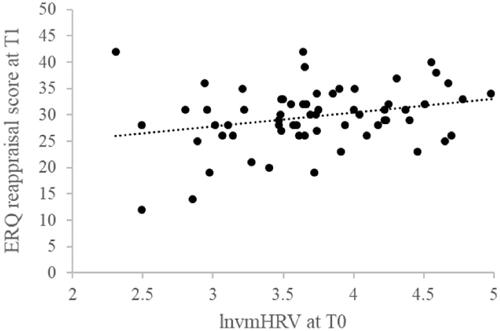
Table 2. Linear regression for the prediction of scores on the cognitive reappraisal subscale of the ERQ at Time 1 based on ln(vmHRV) values at Time 0, controlling for months elapsed between Time 1 and Time 0, sex, age, BMI, and smoking status.
As to momentary mood, the model having “worried” at T1 as outcome yielded a significant role of ln(vmHRV) at T0 and months elapsed between T0 and T1 as predictors (). To better understand the significant interaction between these predictors, we used a median split to divide participants into the Low and High HRV groups and found that only the Low HRV group had higher levels of worry when more months elapsed between T0 and T1 (p < .01).
Table 3. Random effects regression models for the prediction of momentary levels of feeling “worried” and “safe” at Time 1 based on ln(vmHRV) values at Time 0, controlling for months elapsed between Time 1 and Time 0, sex, age, BMI, and smoking status.
In addition, the model having “safe” at T1 as outcome yielded a significant role of ln(vmHRV) as predictor (), also controlling for potential confounders, whereas no significant associations emerged for “anxious”, “sad”, and “optimistic”.
Partial correlation analyses revealed that ln(vmHRV) at T1 was positively associated with ln(vmHRV) at T0 (r = 0.631, p < .001), and negatively associated with CESD (r = −0.261, p = .050) and ERQ suppression (r = −0.255, p = .055) scores. Importantly, a positive significant correlation was found between CESD and PCRS scores (r = 0.391, p < .001). No significant correlations were found between ln(vmHRV) at T1 and PCRS (r = −0.210, p = .117) and ERQ reappraisal (r = 0.179, p = .183) scores.
Discussion
The major and novel findings of this study is that higher resting vmHRV – assessed almost 2 years before – predicted a stronger engagement in more functional emotion regulation strategies, as well as higher daily feelings of safeness and reduced daily worry during COVID-19-related forced lockdown. Present longitudinal findings strengthen previous cross-sectional studies showing that resting vmHRV is positively correlated with functional emotion regulation strategies, such as cognitive reappraisal (e.g. Denson et al., Citation2011). Of note, a negative correlation between vmHRV at T1 and emotional suppression strategies was also found in the present investigation, replicating previous work linking vmHRV with the inability to accept negative emotions and with subsequent emotional suppression (e.g. Visted et al., Citation2017). These results are particularly relevant in the context of stressful situations such as the COVID-19 pandemic, considering that maladaptive emotion regulation has been associated with negative psychological and somatic health outcomes (Hu et al., Citation2014). Relatedly, we found that self-reported depressive symptoms over the forced lockdown were positively correlated with the subjective perception of COVID-19 risk and negatively correlated with concurrent measures of vmHRV. Remarkably, in line with the neurovisceral integration theoretical perspective (Thayer & Lane, Citation2000), higher pre-COVID-19 vmHRV levels predicted reduced worrisome affective state and increased sense of safeness experienced for two consecutive days over the course of the lockdown, suggesting that HRV might not only be a marker but also a precursor of vulnerability/resilience to stress (Carnevali et al., Citation2018). Moreover, the finding that the relationship between vmHRV and worry was stronger when low vmHRV was measured further back in time strengthens the longitudinal predictive power of HRV. We speculate that low resting vmHRV may signal a downward spiraling where impaired vagal tone and psychological wellbeing reciprocally influence one another as a function of time (Kok & Fredrickson, Citation2010). Notably, momentary levels of sadness, anxiety, and optimism were not significantly predicted by HRV at T0, pointing to the specificity of this biomarker for the inner sense of safeness and worry. This is in line with the idea that HRV-related measures index the activity of a core set of neural structures that continuously assess the environment for signs of threat and safety and prepare the organism to adaptively regulate cognition, behavior, and physiology (Thayer & Lane, Citation2000).
In interpreting our findings, we must acknowledge several limitations. First, due to difficulties in recruiting participants with reliable measures of vmHRV before the pandemic, the sample size is modest and should be extended in the future. For example, we did not have a sufficient sample size to elucidate the interaction between vmHRV and worry with the traditional ± 1 standard deviation approach, and used a median split procedure instead. Second, we obtained vmHRV measures at T1 with the use of a smartphone app, which could be suboptimal because smartphone apps cannot discriminate between sinus and non-sinus beats. Nevertheless, we found a significant correlation between vmHRV measures at T1 and T0. Moreover, we do not have measures of emotional regulation strategies at T0; this prevented us to perform a cross-lagged panel analysis, which would have provided stronger evidence of the role of vmHRV as a predictor. Relatedly, it must be noted that the questionnaire used for the assessment of emotion regulation strategies at T1 was not specifically tailored at the COVID-19 pandemic; therefore, our findings should be interpreted more generally in the context of coping styles during stressful situations. Lastly, we did not collect data on physical activity at T0, therefore, we could not control for this potential confounder in the regression models.
Notwithstanding these limitations, the present results might support the implementation of HRV assessment for early identification of individuals who are at higher risk to suffer from stress-related symptoms (Carnevali et al., Citation2018), and encourage the use of interventions, such as transcutaneous vagus nerve stimulation, HRV biofeedback, and compassion-focused therapy to increase vmHRV as a way to foster resilience during stressful pandemic periods and beyond (Dedoncker et al., Citation2021). Considering that the COVID-19 pandemic has led to widespread increases in mental health problems, including anxiety and depression, research aimed at identifying vulnerability risk factors is of clinical relevance.
Disclosure statement
No potential conflict of interest was reported by the author(s).
References
- Bourdillon, N., Yazdani, S., Schmitt, L., & Millet, G. P. (2020). Effects of COVID-19 lockdown on heart rate variability. PLoS One, 15(11), e0242303. https://doi.org/10.1371/journal.pone.0242303
- Carnevali, L., Koenig, J., Sgoifo, A., & Ottaviani, C. (2018). Autonomic and brain morphological predictors of stress resilience. Frontiers in Neuroscience, 12, 228. https://doi.org/10.3389/fnins.2018.00228
- Carnevali, L., Pattini, E., Sgoifo, A., & Ottaviani, C. (2020). Effects of prefrontal transcranial direct current stimulation on autonomic and neuroendocrine responses to psychosocial stress in healthy humans. Stress (Amsterdam, Netherlands), 23(1), 26–36. https://doi.org/10.1080/10253890.2019.1625884
- Dedoncker, J., Vanderhasselt, M. A., Ottaviani, C., & Slavich, G. M. (2021). Mental health during the COVID-19 pandemic and beyond: The importance of the vagus nerve for biopsychosocial resilience. Neuroscience and Biobehavioral Reviews, 125, 1–10. https://doi.org/10.1016/j.neubiorev.2021.02.010
- Denson, T. F., Grisham, J. R., & Moulds, M. L. (2011). Cognitive reappraisal increases heart rate variability in response to an anger provocation. Motivation and Emotion, 35(1), 14–22. https://doi.org/10.1007/s11031-011-9201-5
- Di Bello, M., Ottaviani, C., & Petrocchi, N. (2021). Compassion is not a Benzo: Distinctive associations of heart rate variability with its empathic and action components. Frontiers in Neuroscience, 15, 617443. https://doi.org/10.3389/fnins.2021.617443
- Holmes, E. A., O’Connor, R. C., Perry, V. H., Tracey, I., Wessely, S., Arseneault, L., Ballard, C., Christensen, H., Cohen Silver, R., Everall, I., Ford, T., John, A., Kabir, T., King, K., Madan, I., Michie, S., Przybylski, A. K., Shafran, R., Sweeney, A., … Bullmore, E. (2020). Multidisciplinary research priorities for the COVID-19 pandemic: A call for action for mental health science. The Lancet Psychiatry, 7(6), 547–560. https://doi.org/10.1016/S2215-0366(20)30168-1
- Hu, T., Zhang, D., Wang, J., Mistry, R., Ran, G., & Wang, X. (2014). Relation between emotion regulation and mental health: A meta-analysis review. Psychological Reports, 114(2), 341–362. https://doi.org/10.2466/03.20.PR0.114k22w4
- Kanovsky, M., & Halamova, J. (2020). Perceived threat of the coronavirus and the role of trust in safeguards: A case study in Slovakia. Frontiers in Psychology, 11, 554160. https://doi.org/10.3389/fpsyg.2020.554160
- Kok, B. E., & Fredrickson, B. L. (2010). Upward spirals of the heart: Autonomic flexibility, as indexed by vagal tone, reciprocally and prospectively predicts positive emotions and social connectedness. Biological Psychology, 85(3), 432–436. https://doi.org/10.1016/j.biopsycho.2010.09.005
- Kolacz, J., Dale, L. P., Nix, E. J., Roath, O. K., Lewis, G. F., & Porges, S. W. (2020). Adversity history predicts self-reported autonomic reactivity and mental health in US residents during the COVID-19 pandemic. Frontiers in Psychiatry, 11, 577728. https://doi.org/10.3389/fpsyt.2020.577728
- Laborde, S., Mosley, E., & Thayer, J. F. (2017). Heart rate variability and cardiac vagal tone in psychophysiological research - recommendations for experiment planning, data analysis, and data reporting. Frontiers in Psychology, 8, 213. https://doi.org/10.3389/fpsyg.2017.00213
- Miller, J. G., Chahal, R., Kirshenbaum, J. S., Ho, T. C., Gifuni, A. J., & Gotlib, I. H. (2021). Heart rate variability moderates the effects of COVID-19-related stress and family adversity on emotional problems in adolescents: Testing models of differential susceptibility and diathesis stress. Dev Psychopathol, 1–12. https://doi.org/10.1017/S095457942100033X
- Radloff, L. S. (1977). The CES-D scale: A self-report depression scale for research in the general population. Applied Psychological Measurement, 1(3), 385–401. https://doi.org/10.1177/014662167700100306
- Rajkumar, R. P. (2020). COVID-19 and mental health: A review of the existing literature. Asian Journal of Psychiatry, 52, 102066. https://doi.org/10.1016/j.ajp.2020.102066
- Sgoifo, A., Carnevali, L., Pattini, E., Carandina, A., Tanzi, G., Del Canale, C., Goi, P., De Felici Del Giudice, M. B., De Carne, B., Fornari, M., Gavazzoli, B., Poisa, L., Manzoni, D., & Bollati, D. (2021). Psychobiological evidence of the stress resilience fostering properties of a cosmetic routine. Stress (Amsterdam, Netherlands), 24(1), 53–63. https://doi.org/10.1080/10253890.2020.1750590
- Spaapen, D. L., Waters, F., Brummer, L., Stopa, L., & Bucks, R. S. (2014). The emotion regulation questionnaire: Validation of the ERQ-9 in two community samples. Psychological Assessment, 26(1), 46–54. https://doi.org/10.1037/a0034474
- Tarvainen, M. P., Niskanen, J. P., Lipponen, J. A., Ranta-Aho, P. O., & Karjalainen, P. A. (2014). Kubios HRV–heart rate variability analysis software. Computer Methods and Programs in Biomedicine, 113(1), 210–220. https://doi.org/10.1016/j.cmpb.2013.07.024
- Thayer, J. F., Ahs, F., Fredrikson, M., Sollers, J. J., 3rd., & Wager, T. D. (2012). A meta-analysis of heart rate variability and neuroimaging studies: Implications for heart rate variability as a marker of stress and health. Neuroscience and Biobehavioral Reviews, 36(2), 747–756. https://doi.org/10.1016/j.neubiorev.2011.11.009
- Thayer, J. F., & Lane, R. D. (2000). A model of neurovisceral integration in emotion regulation and dysregulation. Journal of Affective Disorders, 61(3), 201–216. https://doi.org/10.1016/S0165-0327(00)00338-4
- Visted, E., Sorensen, L., Osnes, B., Svendsen, J. L., Binder, P. E., & Schanche, E. (2017). The association between self-reported difficulties in emotion regulation and heart rate variability: The salient role of not accepting negative emotions. Frontiers in Psychology, 8, 328. https://doi.org/10.3389/fpsyg.2017.00328