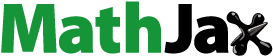
1. Introduction
Simulations of human walking is a current subject of research in different areas. Between the different approaches for the synthesis of human walking (Xiang et al. Citation2010), two clearly distinguish: dynamical synthesis and data motion replay. The first approach allows for the study of the gait itself, without the bias of human data replay that can be found in the second case. This synthesis of walking is commonly found in the humanoid robotics field. However, the majority of robots present flat feet, which is a known drawback in the synthesis of human like gait.
We propose here to compare walking motions generated for human virtual models with and without articulated feet. We make the hypothesis that articulated feet increases the range of possible motions and improves the human likeliness of the generated gait.
2. Methods
2.1 The virtual human
A first model, Model I, composed of 15 rigid segments (head, torso, 2 arms, 2 forearms, 2 hands, 2 legs, 2 shanks and 2 feet) linked together by 14 joints was developed. In order to evaluate the impact of articulated feet in the walking motion, a second model, Model II, is designed, by dividing the foot in two segments, articulated at the metatarsal level, with a one degree of freedom joint. This model is then composed of 17 segments and 16 joints. Body inertial parameters were adapted from (Dumas et al. Citation2007).
2.2 Linear Quadratic Programming Controller
The Linear Quadratic Programming developed by (Salini Citation2012) was used to control the walking motion for both models. The walking motion is generated by combining several objectives to be achieved simultaneously. These objectives are expressed as a task error Ti between a desired acceleration and the system acceleration ai as follows:
The gait generation then consists in finding the system actuation torques, contact efforts and generalised acceleration, , by minimizing a cost function resulting for the weighted ωi sum of all nt squared tasks Ti, as follows:
while respecting the equations of motion and inequality constraints expressed as joint limits, torque limits and non-slipping contact conditions.
2.3 Tasks for the walking motion
The tasks generated for the walking motion are:
• | Left/Right Foot Tasks, controlling the foot frames during foot displacement; | ||||
• | CoM Task, responsible for the control of the Centre of Mass acceleration, following the method of (Wieber Citation2006); | ||||
• | Pelvis Height and Orientation Tasks, controlling the Pelvis Height and Orientation, for stability purposes; | ||||
• | Torso and Head Tasks, responsible for maintaining these segments straight during the entire motion, for stability purposes. |
The main differences between the control of the two models rely on the definition of the foot tasks and the CoM tasks:
• | Model I – Foot displacement is defined with polynomials respecting a zero velocity and acceleration at the beginning and at the end of the displacement in all directions, plus in the vertical direction, maximum height is attained at the middle of the temporal displacement. In this case, the foot leaves and contacts the ground as a whole. | ||||
• | Model II – Foot displacement respects the same zero conditions, but is adapted through B-Splines approximation of foot trajectories of healthy human beings. In this case, feet rockers such as heel rise and toe off are reproduced. |
The CoM Task, which is ZMP based, takes into account the foot segments in contact with the ground at all times.
2.4 Experimental setup
A virtual human is generated using the anthropometric data of a female subject with 51.5 kg and 1.63 m height. Multiple gait patterns are generated with the described controller for different spatial-temporal parameters (SPT) such as step length (varying from 0.2m up to 0.6m), maximum heel height (varying from 0.02m up to 0.22) and walking cycle duration (varying from 1.0s up to 1.4s). A total of 525 simulations are performed.
3. Results and discussion
The ranges of possible spatial-temporal parameters for each model are depicted in Table .
Table 1 Best spatial-temporal parameters achieved for the two models.
They indicate that the introduction of an articulated foot allows to simulate greater range of SPT and especially to reach walking speeds within the range of human ones (Wheelwright et al. Citation1993).
The differences between the two models are the foot modelling and the subsequent adaptations in the gait generation. Naturally, the most impacted joint is the ankle.
To access the principal differences induced in the walking pattern, the correlation factor between the sagittal kinematics of simulation and normal, is used to choose the more human like simulation for both models. The correlation factor between sagittal kinematics of simulation and normal was of 0.52 for Model I and 0.83 for Model II. The kinematics and moments of the right ankle in the sagittal plane for both models can be seen in figure .
Figure 1 Right ankle joint angle (left) and moment (right) in the sagittal plane for Models I (blue) and II (red). Angles are expressed in degrees, and moments are normalized by the product of body mass, height and gravity acceleration (Stansfield et al. Citation2006). Black line represents the healthy human mean and the grey band +/- 2 SD.
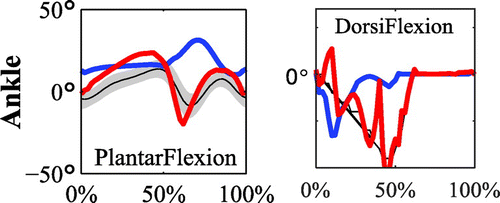
Not only the articulated foot improves ankle kinematics, but moments are also greatly changed. In fact, plantarflexion peak moment occurs near the beginning of the swing phase for Model II, like in human walking, contrarily to the case in Model I.
Videos of the corresponding walking motions can be found in the supplementary electronic data.
4. Conclusions
In this article, the impact of articulated feet in the generated walking motions for a virtual human is accessed.
Walking patterns for different SPT are generated. The gaits generated for Model II increases about 200% the maximum of step lengths and almost 300% the maximum walking speed. Kinematics is greatly improved for the ankle, but also ankle joint moments vary more human likely.
Future work includes inverse optimization techniques to better identify the tasks needed to generate walking patterns, as possible way of improving walking patterns generation.
Acknowledgements
The authors would like to thank the Fondation Ellen Poidatz, the Fondation Bettencourt Schueller, the Association Nationale de la Recherche et de la Technologie (ANRT), and the Region Île de France for their financial support to this work.
References
- Dumas R, Chèze L, Verriest J-P. 2007. Adjustments to McConville et al. and Young et al. body segment inertial parameters. J Biomech. 40:543–553.
- Salini J. 2012. Dynamic control for the task/posture coordination of humanoids: towards synthesis of complex activities. Université Pierre et Marie Curie.
- Stansfield BW, Hillman SJ, Hazlewood ME, Robb JE. 2006. Regression analysis of gait parameters with speed in normal children walking at self-selected speeds. Gait Posture. 23:288–294.
- Wheelwright EF, Minns RA, Law HT, Elton RA. 1993. Temporal and Spatial Parameters of Gait in Children I: Normal Control Data. Dev Med Child Neurol. 35:102–113.
- Wieber P-B. 2006. Trajectory free linear model predictive control for stable walking in the presence of strong perturbations. In: Humanoid Robots 2006 6th IEEE-RAS Int Conf .; p. 137–142.
- Xiang Y, Arora JS, Abdel-Malek K. 2010. Physics-based modeling and simulation of human walking: a review of optimization-based and other approaches. Struct Multidiscip Optim. 42:1–23.