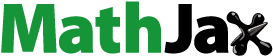
1. Introduction
Neurological lesions cause spasticity, which leads to movement disorders, as in celebrity palsy (CP) or stroke (S). These patients could benefit from the most advanced rehabilitation techniques, such as mechatronic systems and exoskeletons. Unfortunately, these systems face a control problem when dealing with movement disorders. An ideal control strategy is to follow a co-robotic approach where the patient and the exoskeleton collaborate to complete a task. The control should detect the patient intention, capture his variability, ensure the stability, characterize the deviation induced by a change of speed in the movement (Pons Citation2010). To provide this collaboration, the first challenge is to determine the specific admissible range for each joint, then to optimize the movement amplitude that avoids the patient pain. Regarding the two control methods described in the literature, either the control using impedance or using EMG interpretation, both have not studied the influence of the admissible joint range (Yan et al. Citation2015). To overcome this issue, we propose to rely on the direct measurement of bio-kinematic signals, and to find the causes of the movement changes. The activity of muscle co-contraction, around a joint, generates its movement. This activity allows the detection of the patient intention (Shiavi et al. Citation1998) and ensures articular stability (Kellis et al. Citation2003).
Co-contraction indexes (CCI) are not suitable for a proper control strategy since each patient needs different solutions to minimize their movement disorders. We propose a new index, called neuro motor index (NMI), serves to integrate the possibilities and variabilities of patient walking. This index avoids the fatigability while using the technical aids (such as the exoskeleton). We examine the accuracy and efficiency of the NMI under three walking speeds.
2. Methods
2.1. Subjects and data acquisition
Nine healthy subjects, without history of neuromusculoskeletal impairments (five males and four females with age of 45 ± 7 years), were recorded in
Gait Laboratory (Raymond Poincaré Hospital, Garches, France). A bio-kinematics study was conducted in a large room; where the patients walked a straight line for 10 meters. The acquisition system includes:
Video equipment to record the front and the profile of the patient.
An optoelectronic system to measure the segment kinematics and the joint angles of the knee and the hip steps with respect to each foot (left and right).
Ground force platforms to record dynamics (recording of ground reaction forces and the moments).
An electromyographic system to record muscle activity. Electrodes were placed at the level of two biarticular muscle groups (quadriceps and hamstrings) for the knee and the hip on each leg (left and right). Everything was synchronized and connected to a computer for data acquisition. Kinematic measurements and electromyographic data were taken and recorded for three rhythms velocities (fast, normal and slow) and for 11 walking cycles at each velocity.
2.2. Signal processing
The raw EMG signals were band-pass filtered (10–400 Hz), full-wave rectified, low-pass filtered using a Butterworth filter (4th order) from 4 till 6 Hz cut-off frequency according to the subject cadence (Shiavi et al. Citation1998), and normalized by the detected maximal voluntary contraction (MVC) of each muscle on gait trials (Kellis et al. Citation2003).
The kinematic data is filtered using a Butterworth filter (4th order, 6 Hz cut-off frequency) connected to the acquisition system, then they are segmented in 1001 values for knee and hip joints to obtain matrices with fixed dimensions which are equal to those of recorded EMG matrices. Then, their mean and variance are estimated. The CCIs have been continuously computed using a sliding window with an overlap of 10 points and with a single point increment.
2.3. Proposed neuro motor index (NMI)
This index is a sliding evaluation of muscle co-contraction during a gait (Souissi et al. Citation2017) derived from the combination between the CCIs proposed by (Frost et al. Citation1997), (Unnithan et al. Citation1996) denoted CCI1 and by (Hesse et al. Citation2000) denoted CCI2. They are combined together by a nonlinear regression Rm (t), of the quadriceps and hamstring EMGs. The regression is estimated with a Hermitian Polynomial He(t). where: Rm (t) = h1 (t) f0 + h2 (t) p0 + h3(t) f1 + h4 (t) p1
And the peaks are identified with: argmin t | f’(t)|
With: f (t) = EMGantago (t) ∩ EMGago (t); h1, h2, h3, h4He(t); and p0 and p1 are the tangents to f0 and f1.
2.4. Validation with canonical correlation analysis
The canonical correlation (CCA) is a multivariable statistical method used to extract the underlying correlation of two sets of data (Larimore Citation1990). To test the reliability of the NMI, CCA is applied at EMG- joint angles acquired during the gait cycle, at three velocities on the healthy subjects.
3. Results and discussion
On data from healthy subjects, with three velocities (slow, normal, and fast), CCA is applied for knee and hip gait angles (right and left). Regarding CCI1 and CCI2, the correlation points show an obvious variability from one velocity to another, moreover, the percentage of correlation is weak and so far from a linear relation, but regarding NMI the correlation points show strong similarity under the three velocities (). In other words, the percentage of correlation is very high and the correlation is almost linear ().
4. Conclusions
In this article, we have proposed a bio-kinematic strategy to ensure rehabilitation with mechatronic systems. We have investigated the efficiency of a new neuro motor index (NMI) by applying a canonical correlation analysis to extract his relation with the joint walking angles (for hip and knee). Also to ensure its effectiveness and its preference, against the CCIs, by studying it on several speeds. It is an important factor to describe the gait pathology by evaluating the inefficiency of movement. This latest can be a requirement to work with CP and stroke patients, laying a solid ground for the control of rehabilitation exoskeleton. Future work will focus on introducing this index on a control strategy for this type of mechatronic device.
References
- Frost G, Dowling J, Dyson K, Bar-Or O. 1997. Cocontraction in three age groups of children during treadmill locomotion. J Electromyograph Kinesiol. 7(3):179–186.
- Hesse S, Brandl-Hesse B, Seidel U, Doll B, Gregoric M. 2000. Lower limb muscle activity in ambulatory children with cerebral palsy before and after the treatment with Botulinum toxin A. Restorat Neurol Neurosci. 17(1):1–8.
- Kellis E, Arabatzi F, Papadopoulos C. 2003. Muscle co-activation around the knee in drop jumping using the co-contraction index. J Electromyograph Kinesiol. 13(3):229–238.
- Larimore WE. 1990. Canonical variate analysis in identification, filtering, and adaptive control. In: 29th IEEE Conference on Decision and Control. Honolulu, HI: IEEE; pp. 596–604.
- Pons JL. 2010. Rehabilitation exoskeletal robotics. IEEE Eng Med Biol Mag. 29:57–63.
- Shiavi R, Frigo C, Pedotti A. 1998. Electromyographic signals during gait: criteria for envelope filtering and number of strides. Med Biol Eng Comput. 36(2):171–178.
- Souissi H, Zory R, Bredin J, Gerus P. 2017. Comparison of methodologies to assess muscle cocontraction during gait. J Biomech. 57:141–145.
- Unnithan VB, Dowling JJ, Frost G, Volpe BA, Bar-Or O. 1996. Cocontraction and phasic activity during GAIT in children with cerebral palsy. Electromyograph Clin Neurophysiol. 36(8):487–494.
- Yan T, Cempini M, Oddo CM, Vitiello N. 2015. Review of assistive strategies in powered lowerlimb orthoses and exoskeletons. Robot Autonom Syst. 64:120–136.