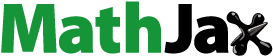
1. Introduction
The biomechanical study of muscle forces is one of the major topics in several fields such as ergonomics, medicine, sports and rehabilitation. In general, two traditional approaches are used to estimating the muscle forces: inverse dynamics and forward dynamics (Erdemir et al. Citation2007). Inverse dynamics is an approach that utilized known kinematic data and external forces to calculate the muscle forces (Davoudabadi et al. Citation2015). Direct dynamics is an approach, which uses the EMG muscular signals to estimate muscle forces and joints moments(Bennour et al. Citation2013). However, the human body is a redundant system where the number of muscles used to actuate each joint usually higher than its degrees of freedom of freedom. Another challenge comes from the difficulty to quantify the muscle forces due to the variability of the geometric parameters required to describe the biomechanical model of the body. In this work, we are developed a robust and computationally efficient methodology to estimate musculotendon forces from kinematic data and EMG measurements during dynamic contraction to take into consideration the variability of the geometric parametersof muscles. Specifically, this approach consists to study the influence of properties muscles, e.g. tendon length, on musculotendon forces.
2. Methods
In this work, the prediction of forces exerted by muscles during dynamic contraction is obtained based on direct dynamics approach. This approach consists of three main steps: signal process, dynamic activation and dynamic contraction. First, the EMG signals of the muscles and the motion capture data were used as inputs of this approach. The first step is the signal process consisting to transform raw signal to filtered excitation signal. Then, the second step is the dynamic activation, where neural signal is transformed to muscle activation. In the third step, musculotendon forces are calculated using the dynamics contraction based in musculoskeletal model.
(1)
(1)
To calculate musculotendon forces, two nonlinear differential equations were used. The first equation is a first order differential equation, which relates muscle excitation to muscle activation(Lloyd and Besier Citation2003). The second equation transforms the obtained muscle activation into a mechanical force. It is a first order differential equation (EquationEquation 1
(1)
(1) ) that will be integrated numerically to ensure the calculation of the musculotendon force. The calculation methodology is implemented in .
Where is the musculotendon force,
is the muscle force,
is the tendon stiffness,
the pennation angle,
is the muscle fiber velocity and
is the musculotendon velocity. Unlike previous studies, the proposed computational model is based on a number of parameters used to characterize the properties of muscles, e.g. musculotendon length
tendon length
muscle force-length relationship
force-velocity relationship
Accurately, the proposed methodology is based in the influence of tendon slack length
in muscle contraction force.
3. Results and discussion
In this paper, the results will be present and analyse in order to validate the influence of tendon in the muscle force during elbow flexion-extension. For this reason, a three-dimensional biomechanical model of the human arm is developed in OpenSim. The model consists of two skeletal segments, two joints including the shoulder and the elbow, with four musculotendon actuators. Each musculotendon unit is an assembly in series of a Hill-type muscle model with a tendon.
As shown in , BRA and BRD are monoarticular muscles, but BIC and TRIC are biarticular muscles. The musculotendon lengths are functions of the joint angle.
illustrates the muscle forces obtained using Hill’s muscle model and musculotendon model. The results obtained show the influence of the tendon in the movement of elbow flexion-extension. The major difference is the development of passive force in the muscle model. Based on the force-length muscle relationship, the presence of a passive force means that the muscle length is longer compared with the isometric muscle length. Contrariwise, the variation of tendon length, in the musculotendon model, enables the muscle to contract at a more optimal velocity and optimal length. Consequently, when muscle working in this optimal zone, the activation needed to developed the musculotendon forces will be lower than the present in the muscle model.
4. Conclusion
The goal of this article was to develop and implement a computationally efficient method to calculate muscle forces of the upper limb using a process based on kinematic data and EMG measurement during dynamic contraction. The method was shown to be efficient in calculating the forces in the muscles during the elbow flexion extension. The results showed the influence of the tendon length in estimating the muscle forces. The variation on tendon length as function in time allow the muscle to contract or elongate with a length and velocity more close to the optimal one, decreasing then the activation needed to produce the force to realize a certain movement (Schoner Citation1990).
References
- Bennour S, Zarrouk N, Dogui M, Romdhane L, Merlet J-P. 2013. Validation of optimisation model that estimates the musculotendinous forces during an isometric extension of knee. Computer Method Biomech Biomed Eng. 16(suppl 1):167–169.
- Davoudabadi S, Skipper M, Zee MD, Rasmussen J. 2015. Human arm posture prediction in response to isometric endpoint forces. J Biomech. 48(15):4178–4184.
- Erdemir A, Mclean S, Herzog W, Bogert AJVD. 2007. Model-based estimation of muscle forces exerted during movements. Clin Biomech (Bristol, Avon). 22:131–154.
- Lloyd DG, Besier TF. 2003. An EMG-driven musculoskeletal model to estimate muscle forces and knee joint moments in vivo. J Biomech. 36(6):765–776.
- Schoner G. 1990. A dynamic theory of coordination of discrete movement. Biol Cybenet. 270:257–270.