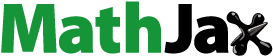
Keywords:
1. Introduction
Classically, inverse dynamics studies of motion are based on a bottom-up approach measuring the Ground Reaction Forces and Moments (GRF&M) with force platforms. The field of study is constrained to the contact zones of these platforms, diminishing the ecological properties of the motion to be studied and constraining drastically the analysis. Such a limitation is critical for sports motion analysis, since motions to be studied can be quite large in amplitude and varied in their contacts with the ground.
GRF&M prediction offers the opportunity to enlarge the field of study without additional measure to be made. Optimization-based GRF&M prediction methods are using a set of contact points defined under the feet to generate forces acting on the body. Motion analysis is used to define at any moment the points in contact with the ground and an optimization routine finds the minimal set of forces to be applied on these points satisfying the dynamical equilibrium of the whole body. If such methods have been validated on daily living motions (Fluit et al. Citation2014) and some classical sports motions (Skals et al. Citation2017) such as running, vertical jump or side-cut motions, their capacity to predict GRF&M on complex sport motions is still to be proved.
Lunge motion is a fundamental attack of modern fencing, asking for a high level of coordination, speed and accuracy. It consists in an explosive extension of the front leg accompanying an extension of the sword arm. In such motions, the direction of action and the way feet are oriented – guard position - are particularly challenging for a GRF&M prediction method. The current study aims at evaluating an optimization based GRF&M prediction method on a large set of fencing lunge data.
2. Methods
2.1. Experimental data
The experimental data used in the current study is extracted from another one dealing with uncertainty during lunge motions (Sorel et al. Citation2019). 10 of 11 male regional to national level fencers (5 right handed, 5 left handed, aged 22 ± 3 y.o., height 1.78 ± 0.06m, weight 75 ± 8kg) participated to this study, giving their informed consent after a presentation of the protocol (validated by the INRIA national ethics comitee). They performed a total of 120 fencing lunges each, under various conditions. 1 fencer of the original cohort was excluded from the present study due to unexploitable force platform data. Whole body motion of the fencers was recorded thanks to a VICON optoelectronic system (24 cameras, 250 Hz). A set of 49 markers derived from the ISB recommendations was used. Each foot was placed under an AMTI Force platform (1000 Hz), enabling the measure of GRF&M under each foot independently during the whole motion. Lunges start-end were extracted with the method proposed in (Sorel et al. Citation2019). A whole body biomechanical model developed in the CusToM library (Muller et al. Citation2019) was used to perform the inverse kinematics analysis and the GRF&M prediction. Geometry of the model was scaled to the subjects (Muller et al. Citation2016) and masses and inertias were obtained from (Dumas et al. Citation2007).
2.2. GRF&M prediction and analysis
For the total of 1200 lunges extracted from the experimentation, a classical GRF&M prediction method was applied. A set of Nf = 14 contact points per foot was defined. At each frame, a Sequential Quadratic Programming (SQP) method found the solution of the following minimization problem:
where, by isolating the whole-body biomechanical model, M(q) is the inertia matrix, C(q, q̇) is the centrifugal and Coriolis force vector, G(q) is the gravity force vector, λ is the generalized internal force vector, E is the generalized external force vector (computed from the contact force at each contact point Fi) and Fimax the vector containing the maximal force available for the contact point i. At each frame, a contact point was considered as active if it was close to the floor and almost without motion. The distance and velocity thresholds were respectively 0.02 m and 0.8 m/s. When a contact point was respecting the thresholds, the associated force was limited to 0.4 Body Weight and had to respect the Coulomb’s law of friction. A friction coefficient of 0.5 was used here (Skals et al. Citation2017). Predicted forces and moments of each foot were compared to the measured ones in terms of RMSE, RMSE normalized to body mass, rRMSE and Pearson’s correlation coefficient.
3. Results and discussion
Results presented are very similar to some of the results presented in (Skals et al. Citation2017). The vertical forces Fz are accurately estimated with a particularly low rRMSE. Most of correlation coefficients are high, excepted for the medio-lateral force Fx and the vertical moment Mz. Fx is particularly low during the whole motion - see RMSE/BM, explaining this issue, whereas Mz is poorly predicted due to the bad estimation of the antero-posterior component Fy. This issue is particularly visible in : at the beginning of the lunge, a static phase in guard position presents an important Fy value of opposite value at each foot. Such a stabilization scheme, ensuring a good balance of the fencer, is not well predicted by the algorithm.
Table 1. Mean ± std RMSE, rRMSE, RMSE/BW and r for all the trials and all the subjects.
Indeed, the minimization tends to find the minimal set of forces ensuring equilibrium, and therefore does not predict forces arising from specific motor control strategies, generally compensated in the whole-body dynamic equilibrium. This issue is a future way of improvement, by adding motor control features in the cost function/the constraints of the optimization scheme, such as balance or stiffness control.
In conclusion, such a method is already quite relevant to study specific and challenging motions in a less constrained field, even if improvements can be made in the definition of the optimization problem to be solved.
References
- Fluit R, Andersen MS, Kolk S, Verdonschot N, Koopman HF. 2014. Prediction of ground reaction forces and moments during various activities of daily living. J Biomech.
- Skals S, Jung MK, Damsgaard M, Andersen MS. 2017. Prediction of ground reaction forces and moments during sports-related movements. Multibody Syst Dyn. 39(3):175–195.
- Sorel A, Plantard P, Bideau N, Pontonnier C. 2019. Studying fencing lunge accuracy and response time in uncertain conditions with an innovative simulator. Plos One. 14(7):e0218959.
- Muller A, Pontonnier C, Puchaud P, Dumont G. 2019. CusToM: a Matlab toolbox for musculoskeletal simulation. JOSS. 4(33):927.
- Muller A, Germain C, Pontonnier C, Dumont G. 2016. A simple method to calibrate kinematical invariants: application to overhead throwing. ISBS conference.
- Dumas R, Cheze L, Verriest JP. 2007. Adjustments to McConville et al. and Young et al. body segment inertial parameters. J Biomec.