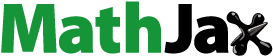
1. Introduction
Musculoskeletal disorders (MSDs) are injuries of the human musculoskeletal system. Occupational diseases represent major problem in the current industry affecting the operator heath, especially the joints and muscles of the upper limb (Zakaria et al. Citation2002). For determining the risk of MSDs and improving the ergonomics of the workplace, one of the important indicators is to analyze the musculo-tendon forces developed by workers. Since direct measurements of forces are difficult, several indirect methods are developed (Buchanan et al. Citation2004). For example, one approach is based on an estimation of the forces from the recording of electrical activity. Another method, inverse dynamics followed by static optimization, uses motion capture data as the only input for the estimation of the muscle forces (Cahouet et al. Citation2002). This method is often used, since the experimental acquisition of kinematic data is possible for most movements. Inverse method followed by static optimization has three main parts: development of a biomechanical model, calculation of joint torques and development of static optimization problem. In this paper, we have developed a static optimization problem with several constraints for estimating the musculo-tendon forces of the human upper limb, which could be used to assist ergonomists to better understand the risk of MSDs. The main advantage of this study is the evaluation of several objective functions by examining their analytical results.
2. Methods
In this work, the estimation of muscle forces during elbow flexion is obtained from inverse dynamics method followed by static optimization technique. During elbow flexion, the equilibrium of one articulation leads only to one mathematical equation with several unknown musculo-tendon forces (EquationEquation (1)(1)
(1) ).
(1)
(1)
Where is the joint torque,
is the matrix of moment arms and
is the vector of musculo-tendon forces. Estimation of individual forces based on the equilibrium equation is a redundant problem. Indeed, elbow joint is activated by six muscle units, which are long Biceps, short Biceps, Brachialis, Brachioradialis, Triceps and Anconeus, implying then a statically underdetermined system. Therefore, the most common solution to this redundant problem involves the optimization of a cost criterion. In this study, we examined two groups of objective functions, which are minimum forces criterion (Group I) and minimum stresses criterion (Group II). The different objective functions are summarized in .
Table 1. The different objective functions evaluated in this study.
Where is the maximum muscle force and
is the physiological cross-sectional area. For a better estimation of individual musculo-tendon forces during elbow flexion, the static optimization problem with constraints is formulated as follows:
(2)
(2)
Under the constraints
Where is varied to product different objective functions and
represents the exponent of the objective function. The equality constraint represents the equilibrium between musculo-tendon forces and joint torque. The inequality constraints represent the physiology of the muscles, where each muscle force is positive, and their physiological limits are not reached.
3. Results and discussion
The aim of the present study is to develop an inverse method followed by static optimization for estimating the musculo-tendon forces from motion data, in order to determine the most reliable performance function. As previously mentioned, we computed the individual muscle forces involved in the elbow joint during flexion movement using several cost functions.
shows the musculo-tendon forces for the long Biceps and Triceps muscles obtained by solving the static optimization problem using different objective functions. Comparing the obtained results with the EMG measurements as described by (Thaut et al. Citation1991) shows clearly that the overall shape of the musculo-tendon forces obtained by nonlinear functions for minimum forces criteria, and for minimum stresses criteria,
was closer to the experimental muscle activation during elbow flexion. It can be seen also that the quadratic function for minimum stresses
gives a more realistic strength for Triceps muscle.
Figure 1. Musculo-tendon forces obtained by different functions for long biceps (a) and triceps (b).
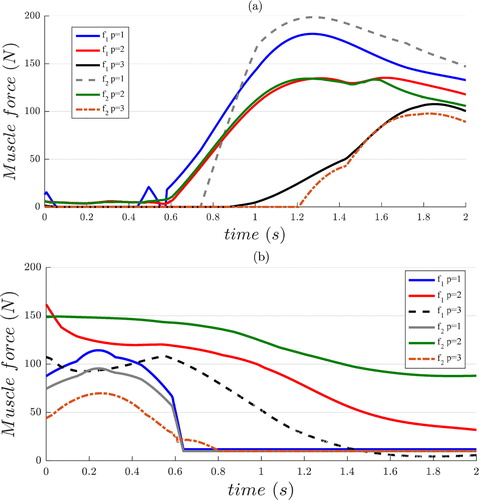
illustrates the forces for six muscles estimated by quadratic function for muscle stresses criterion This objective function does have the advantage that the predicted forces will not exceed maximum physiological limits, which means that the solution will at least lie within the range of physiologically feasible solutions. Thus, musculo-tendon forces are better described by static optimization procedure.
4. Conclusions
The present study presented an inverse method for assessing individual musculo-tendon efforts of upper limb. Due to the high number of muscles involved in the elbow joint, the estimation of forces has been realized by static optimization technique. Several objective functions have been tested. The interpretation of the obtained results shows clearly that the musculo-tendon forces estimated by quadratic function for minimum stresses criterion are much more realistic, close and in agreement with the EMG muscle signals.
Disclosure statement
No potential conflict of interest was reported by the authors.
References
- Buchanan TS, Lloyd DG, Manal K, Besier TF. 2004. Neuromusculoskeletal modeling: estimation of muscle forces and joint moments and movements from measurements of neural command. J Appl Biomech. 20(4):367–395.
- Cahouet V, Luc M, David A. 2002. Static optimal estimation of joint accelerations for inverse dynamics problem solution. J Biomech. 35(11):1507–1513.
- Thaut M, Collins F, Schleiffers S, Davis W. 1991. Analysis of EMG activity in biceps and triceps muscle in an upper extremity gross motor task under the influence of auditory rhythm. J Music Therapy. 28(2):64–88.
- Zakaria D, Robertson J, MacDermid J, Hartford K, Koval J. 2002. Work-related cumulative trauma disorders of the upper extremity: navigating the epidemiologic literature. Am J Ind Med. 42(3):258–269.