Abstract
Trends in water quality and quantity were assessed for 11 major reservoirs of the Brazos and Colorado river basins in the southern Great Plains (maximum period of record, 1965–2010). Water quality, major contributing-stream inflow, storage, local precipitation, and basin-wide total water withdrawals were analyzed. Inflow and storage decreased and total phosphorus increased in most reservoirs. The overall, warmest-, or coldest-monthly temperatures increased in 7 reservoirs, decreased in 1 reservoir, and did not significantly change in 3 reservoirs. The most common monotonic trend in salinity-related variables (specific conductance, chloride, sulfate) was one of no change, and when significant change occurred, it was inconsistent among reservoirs. No significant change was detected in monthly sums of local precipitation. Annual water withdrawals increased in both basins, but the increase was significant (P < 0.05) only in the Colorado River and marginally significant (P < 0.1) in the Brazos River. Salinity-related variables dominated spatial variability in water quality data due to the presence of high- and low-salinity reservoirs in both basins. These observations present a landscape in the Brazos and Colorado river basins where, in the last ∼40 years, reservoir inflow and storage generally decreased, eutrophication generally increased, and water temperature generally increased in at least 1 of 3 temperature indicators evaluated. Because local precipitation remained generally stable, observed reductions in reservoir inflow and storage during the study period may be attributable to other proximate factors, including increased water withdrawals (at least in the Colorado River basin) or decreased runoff from contributing watersheds.
The chemical composition of surface waters is the result of a complex interaction among the natural geochemistry of watersheds and aquifers, topography, and climate (Dillon and Kirchner Citation1975). Anthropogenically driven changes in land cover (Foley et al. Citation2005, Kaushal et al. Citation2005, Price and Leigh Citation2006, VanLandeghem et al. Citation2012) and climate (Austin and Colman Citation2007, Tadonleke et al. Citation2009, Bonte and Zwolsman Citation2010, Zhang et al. Citation2010, Sahoo et al. Citation2011, Shrestha et al. Citation2012) can also influence the quality and quantity of surface waters and consequently the ecosystem services they provide.
Constructed reservoirs provide a surface water supply for human consumption, irrigation, hydroelectric power, flood control, and recreational activities. Reservoirs also serve as habitat for aquatic organisms, and many support important subsistence, commercial, and sport fisheries (Downing and Plante Citation1993, Petrere Citation1996, Allen et al. Citation2008). The state of Texas is in the southernmost region of the Great Plains (Wishart Citation2011). While water supply impounded by the more than 100 major reservoirs in the state provides a critical buffer to mitigate the effects of drought, increasing demands for water coupled with decreasing supplies are projected to result in a steadily growing total water deficit for the state through much of the 21st century (TWDB Citation2012). In addition, about 50% of surface waterbodies for which information is available are currently listed as not meeting water quality criteria (TCEQ Citation2011a), and 90% of reservoirs are classified as eutrophic or hypereutrophic (TCEQ Citation2011b). A recent study estimated that climate change could contribute to the further deterioration of the quality and quantity of water in Texas (Nielsen-Gammon Citation2011), due in part to projected changes in the frequency and intensity of drought periods (Melillo et al. Citation2014). Ecological consequences of changes in the quality and quantity of surface waters can include an increased risk of harmful algal blooms (HABs; Paerl et al. Citation2011a, Citation2011b, O’Neil et al. Citation2012). For example, blooms of toxic golden algae (Prymnesium parvum) in reservoirs of the southern Great Plains have been linked to water quality attributes such as high salinity and eutrophic conditions (Hambright et al. Citation2010, Roelke et al. Citation2012, Patiño et al. Citation2014, VanLandeghem et al. Citation2015a, Citation2015b).
Retrospective analyses of reservoir water quality and quantity in the context of long-term trends can help natural resource managers anticipate and plan for future needs. Results of such analyses can also yield information about water quality factors that are associated with or have influenced past distributions of harmful algae and therefore help model and anticipate their future distribution. Studies focusing on individual reservoirs while simultaneously covering wide geographic areas across a variety of landscapes, however, are limited. Therefore, the objective of this study was to assess long-term spatiotemporal characteristics of reservoir water quality and quantity in 2 major river basins of the southern Great Plains: the Brazos River and the Colorado River, Texas. Reservoirs selected for this study include several whose ecological integrity has been severely impacted by toxic blooms of golden algae (Southard et al. Citation2010, VanLandeghem et al. Citation2013, Patiño et al. Citation2014). The intent of this study was not to develop process-based models of reservoir function but to provide a first-order, regional perspective of trends and status of discrete water quality and quantity parameters.
Study area
The portions of the Brazos River and Colorado river basins targeted by this study () are located in the southern Great Plains within Texas (Wishart Citation2011). Their total basin watersheds cover a significant land area of 11.6 (BRA 2012) and 10.4 (Leifeste and Lansford Citation1968) million ha, respectively, and vary spatially in underlying geology, land use, land cover, and precipitation patterns. The section of the Brazos River basin pertinent to this study sequentially includes the semi-arid high plains (Permian age sediments), the Osage plains, and central Texas, such that changes in geology, and hence subsurface water sources and interactions, typically lead to reduced salinity in a downstream direction (Baker et al. Citation1964, Rawson Citation1967, Wurbs et al. Citation1993). The section of the Colorado River basin pertinent to this study begins in the rolling plains and prairies of west-central Texas, running through chloride-rich Permian age sediments before turning southeast across lesser salt-contributing Cretaceous sediments of the Llano uplift and the central Texas Hill Country (Leifeste and Lansford Citation1968). Average annual precipitation in both basins tends to increase along a west-to-east gradient nearly tripling from about 40 to 115 cm (Nielsen-Gammon Citation2011).
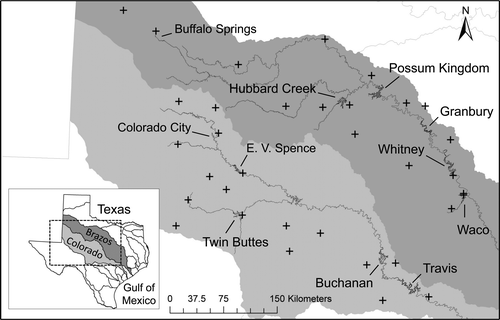
Numerous reservoirs located in the study area primarily serve as water sources for local as well as relatively large metropolitan areas such as Waco (Brazos River) and Austin (Colorado River), and for numerous municipal, agricultural, and industrial users. We selected 11 reservoirs for this study, ordered in a downstream direction: Brazos River reservoirs included Buffalo Springs Lake, Hubbard Creek Reservoir, Possum Kingdom Lake, Lake Granbury, Whitney Lake, and Waco Lake; Colorado River reservoirs included Lake Colorado City, E.V. Spence Reservoir, Twin Buttes Reservoir, Lake Buchanan, and Lake Travis (). Tributary reservoirs included Buffalo Springs Lake, Hubbard Creek Reservoir, and Waco Lake on the Brazos River; and Lake Colorado City and Twin Buttes on the Colorado River; all others are mainstem reservoirs.
The salinity of mainstem reservoirs in both basins tends to decrease in a downstream direction, attributable in part to dilution by discharge from lower-salinity tributaries. Specifically, the salinity of the tributary reservoirs Hubbard Creek, Waco Lake (Brazos), and Twin Buttes (Colorado) is lower than mainstem reservoirs, but that of Buffalo Springs Lake (Brazos) and Lake Colorado City (Colorado) is similar or higher. The relatively high salinity of Buffalo Springs Lake is associated with discharge of treated wastewater effluent and urban runoff from the city of Lubbock, Texas (VanLandeghem et al. Citation2012). Lake Colorado City is on Morgan Creek, a naturally saline tributary in the upper reaches of the Colorado River (Paine et al. Citation2005).
Methods
Data acquisition and preprocessing
The amount of data available over the period of record (POR) relative to the maximum amount of data possible (1965–2010) varied substantially among water variables and reservoirs, especially for water quality variables. This POR, therefore, is not to be inferred as representative for any one variable and (or) reservoir; the beginning of this POR even predates the construction of some reservoirs. This section describes criteria implemented to select and process data so that assessments could be made in time and space for the largest possible number of variables.
Reservoir water-quality and storage data and caveats
Reservoir water quality and storage data for this study were primarily derived from datasets assembled and preprocessed from raw sources by Burley et al. (Citation2011). The original sources for water quality data were the US Geological Survey (USGS) and the Texas Commission on Environmental Quality (TCEQ). Depending on the reservoir and water variable, the maximum POR for the present analyses is as broad as 1965–2010. Water quality data from each source (agency) were kept as distinct “data products” and were temporally and spatially pooled by a simple arithmetic mean by day (when more than one reading per day per reservoir was available) while preserving the depth-specific nature of each water quality record (Burley et al. Citation2011).
Supplemental water quality data for this study were obtained from other sources including the Lower Colorado River Authority (LCRA, online download), the Colorado River Municipal Water District (CRMWD, Big Spring, TX; directly provided by that agency), and the Brazos River Authority (BRA, online download). These supplemental data cover periods of time for selected reservoirs where the primary database (Burley et al. Citation2011) contained little to no USGS or TCEQ data. Inspection of the supplemental data indicated they were distinct from those in Burley et al. (Citation2011). For this study, the supplemental data for each basin were maintained as a separate product from those data collected by Burley et al. (Citation2011).
The following water quality variables were included in this study: chloride (mg/L), dissolved oxygen (mg/L), fluoride (mg/L), total hardness (mg/L in CaCO3 equivalents), nitrogen (mg/L, total [unfiltered] and dissolved [filtered]), nitrate + nitrite (mg/L), pH, phosphorus (mg/L, total and dissolved), potassium (mg/L, total and dissolved), specific conductance (μS/cm), sulfate (mg/L), temperature (C), warmest (by month) temperature (C), and coldest (by month) temperature (C).
The original sources for reservoir storage data (m3) were the USGS, TCEQ, and Texas Water Development Board (TWDB; Burley et al. Citation2011). At the time of this study, the maximum POR for storage data was 1965–2009. The first year included in the analysis of any given reservoir was no earlier than the calendar year after reservoir completion. For example, E.V. Spence Reservoir () was completed in 1969, but the first year for analysis was 1970.
For this study, trend analyses of a water variable require that data be pooled into predefined time intervals, such as months (Hirsch et al. Citation1982, Helsel and Hirsch Citation2002, Migliaccio et al. Citation2011). Trend analysis of reservoir storage was based on monthly median reservoir storage values calculated from daily averages of storage for each reservoir. For analysis of water quality, data from each original source (USGS and TCEQ: Burley et al. Citation2011; LCRA, CRMWD and BRA: supplemental data) with intact depth structures were separately processed by Locally WEighted Scatterplot Smoothing (LOWESS; Cleveland Citation1979), a special form of nonparametric regression-based smoothing, to produce monthly estimates at a specified depth (see Depth-corrected monthly estimates of water quality).
The preprocessing of the Burley et al. (Citation2011) and supplemental datasets differed. A monthly water quality estimate at a given depth derived from Burley et al. (Citation2011) represents the arithmetic mean of all available daily values within a month, which themselves are arithmetic means of available samples on a given day. Data from the supplemental sources were not averaged prior to the LOWESS smoothing; instead, all values available within a given month were used in the LOWESS smoothing process. When compared to other sources of uncertainty, this difference in preprocessing of the Burley et al. (Citation2011) and supplemental data was considered inconsequential for the scope and objectives of the present study.
Other factors limited our opportunity to rigorously assess potential biases. For example, water quality data for reservoirs in Texas have been collected by various agencies under various protocols (including time of day), instrumentation, laboratory processing (or “schedules”), and even perhaps from different spatial locations. While metadata concerning data acquisition are limited and inconsistently available, for the present study we assumed that data from the respective agencies are inherently correct and consistent. Also, the majority of major reservoirs in Texas (including those used in this study) exhibit significant changes in storage, typically in cycles of varying durations and as a result, reservoir elevation (the vertical dimension) changes considerably. For any record of water quality at a given depth, its vertical relation to the larger lake environment and setting is another external factor with unknown ramifications on the analysis of water quality. Although all of these factors may introduce a degree of bias into the data, it is reasonable to assume that any such biases would be randomly distributed and, therefore, that averages derived from trend analyses would become unbiased over the long term. Additional quality assurance–quality control procedures are described later (see Single values, data density, and outliers).
Depth-corrected monthly estimates of water quality
Reservoir water quality attributes can be strongly influenced by position in the water column because of depth-dependent differences in temperature, light levels, and the specific hydrology of each reservoir (Forbes et al. Citation2008). Consequently, analysis based on a consistent depth is critical for temporal (within reservoir) and spatial (among reservoirs) assessments of water quality. For each variable in each reservoir, a LOWESS smoothing (smoother-span = 1/2, iterations = 3) was conducted on data for each available day and separately for each source (previously discussed) to obtain a single estimated monthly value at a depth of 1 m. The depth-corrected monthly estimates were then merged to yield a single series of monthly values. In cases of temporal overlap between data sources, the monthly estimate reported was the median of the estimates for each reservoir.
Single values, data density, and outliers
Some data sources reported a single value (collected at a single depth) for water quality variables in some of the reservoirs. In the vast majority of these situations, the single depth was 1 foot (30.48 cm), which was assumed to be sufficiently close to 1 m for the purpose of the present study.
To account and compensate for the variability in available data for water quality, a water variable for a given reservoir had to contain data for at least 16.6% of months over the POR, which is approximately equivalent to at least 2 months per year. This minimum level was chosen as an ad hoc compromise for maintaining reliability of results for comparative purposes while simultaneously increasing sample sizes. This criterion allows the inclusion of more variables and reservoirs in the analysis than would otherwise be available. Although uneven within-year distribution of data could create temporal biases, we assumed that any such error would occur randomly in any given year and therefore balance out and become unbiased over the long term. In addition, the use of Seasonal Kendall Tau (see Data analyses) for trend analysis can compensate for this type of bias because it compares the same months (or seasons) across years.
To mitigate the influence of potential outliers, a monthly estimate for a given variable was excluded from further analysis if the value met both of the following ad hoc criteria: (1) a value was 5 times higher/lower than the next highest/lowest value in the dataset, and (2) graphical time-series analysis of flanking values on a potential outlier did not heuristically suggest the presence of a short-term fluctuation. This procedure resulted in the identification and removal of only 6 instances of more than 1500 cases available for analyses, including the February 2007 chloride and hardness data points from Hubbard Creek Reservoir, the September 1971 pH datum for Whitney Lake, the July 1982 dissolved oxygen datum from E.V. Spence Reservoir, and the July 1976 and August 2002 sulfate data points from Lake Colorado City.
Inflow, precipitation, and water withdrawal data
Most reservoir inflow data (m3/s) were obtained from USGS streamflow-gaging stations (streamgages) on major streams upstream of reservoirs using the USGS National Water Information System (NWIS) web interface (http://waterdata.usgs.gov). Reservoir inflow data for Lake Travis were obtained from the LCRA. The maximum POR for this analysis is 1970–2010. These streamflow data are observational and represent only major inflow. Several studies of Texas streamflow trends have been conducted previously using similar statistics as for this study (Asquith and Heitmuller Citation2008, Asquith and Barbie 2013, Miyazono et al. Citation2015 and references therein). These studies collectively provide background context to streamflow history in Texas.
Daily precipitation data (mm/d) were obtained from 34 weather stations (National Climatic Data Center, http://www.ncdc.noaa.gov/) located in the vicinity of the study reservoirs (). At least 2 and as many as 4 weather stations were selected for each reservoir to provide spatial representation over its corresponding watershed. The POR for this analysis is 1965–2009.
Data on water withdrawals for the Brazos and Colorado river basins were obtained from TWDB (http://www.twdb.texas.gov/waterplanning/waterusesurvey/estimates/index.asp). Annual water usage information is compiled from surveys of water withdrawals by multiple sectors, including mining, industry, irrigation, electricity generation, and livestock, and includes both ground and surface water withdrawals. The POR for this analysis is 1974–2010; data prior to 1974 are not available. Present analyses were based on total water withdrawals (combined surface and ground).
Data analyses
Kendall tau (standard and seasonal) and principal component analyses (PCA) were conducted with the R statistical programming language (R Development Core Team 2010; v2.12). Quantile regressions were performed using the QUANTREG procedure in SAS (SAS Institute, Cary, NC, USA; v9.3). An overall α of 0.05 was used to assess the statistical significance of tests. Type 1 error rates introduced by conducting multiple hypothesis tests of water quality and storage for reservoirs within each basin were controlled by calculating the q-values of all P-values produced by individual trend analyses using function “q-value” in R (Dabney et al. Citation2011). The q-values can be interpreted similarly to P-values except that a q-value is a measure of significance in relation to the False Discovery Rate (pFDR) of a hypothesis test instead of the rate of false positives (Storey Citation2002, 2003, Storey and Tibshirani Citation2003). Total water withdrawal is a basin-wide attribute, and the significance of trend analysis for this variable was based on actual P-values.
Trend analyses of reservoir water quality and storage, and basin-wide withdrawals
Monthly data for water quality and storage variables in each reservoir were analyzed using the Seasonal Kendall Tau test, which accounts for seasonal variability (Hirsch et al. Citation1982, Helsel and Hirsch Citation2002; custom R-script, Appendix A). Warmest- and coldest-monthly temperatures of the year and total water withdrawals consisted of a single value per year and thus were analyzed using the conventional Kendall tau (Helsel and Hirsch Citation2002). These methods measure the strength and direction of the monotonic relation between values of a given variable and time, represented by the Kendall tau coefficient (τ: range, −1 ≤ τ ≤ 1). Sen slopes (Sen Citation1968), which give the approximate rate of change per year, were also calculated (custom R-script, Appendix A). Kendall tau and Sen slope computations are nonparametric and less sensitive to deviations from normality and the presence of outliers than are parametric methods (Helsel and Hirsch Citation2002).
Quantile regression of inflow and precipitation
Quantile regression facilitates detection of trends in the overall value of a variable (represented by the median, or 50th quantile) as well as in their extremes values (e.g., 10th and 90th quantiles) (Koenker and Bassett Citation1978). Because patterns in hydrological variables have been shown to vary widely at different quantiles (Lins and Slack Citation1999), the consideration of extremes along with the median facilitates a representative picture of overall trends. For reliable estimation of quantiles, this approach to trend analysis requires relatively high density of data distributed evenly in the time axis and therefore could be applied only to inflow and precipitation. For inflow data, separate analyses were conducted for each of the 15 streamgages examined because the watershed area above each streamgage varied from 20254 to 5133121 ha (USGS Citation2013). Distributions of daily streamflow averages were constructed for each year of the POR, and annual changes in the 10th, 50th, and 90th quantiles at each streamgage were computed. Trends were expressed as the estimated annual change at each selected quantile (in m3/yr) as well as the percent change in the respective quantiles calculated for the entire POR. The POR varied among streamgages, but at least 15 years of data were available for each gage.
Table 1 Water-quality and storage variables analyzed in reservoirs of the Brazos River basin for monotonic trends in their monthly values using Seasonal Kendall Tau test. Shown for each variable are the specific period of record (POR) for each analysis, tau, Sen slope (change per year), and minimum, median, and maximum values. Trends found to be significant after q-value correction (q < 0.05) are highlighted in bold, italic type. NA: either data were not available or available data did not meet minimum density criteria (see text); NC: Sen slope not calculated.
Table NaN Water-quality and storage variables analyzed in reservoirs of the Brazos River basin for monotonic trends in their monthly values using Seasonal Kendall Tau test. Shown for each variable are the specific period of record (POR) for each analysis, tau, Sen slope (change per year), and minimum, median, and maximum values. Trends found to be significant after q-value correction (q < 0.05) are highlighted in bold, italic type. NA: either data were not available or available data did not meet minimum density criteria (see text); NC: Sen slope not calculated. (Continued)
Table 2 Water quality and storage variables analyzed in reservoirs of the Colorado River basin for monotonic trends in their monthly values using Seasonal Kendall Tau test. Shown for each variable are the specific period of record (POR) for each analysis, tau, Sen slope (change per year), and minimum, median, and maximum values. Trends found to be significant after q-value correction (q < 0.05) are highlighted in bold, italic type. NA: either data were not available or available data did not meet minimum density criteria (see text); NC: Sen slope not calculated.
Table NaN Water quality and storage variables analyzed in reservoirs of the Colorado River basin for monotonic trends in their monthly values using Seasonal Kendall Tau test. Shown for each variable are the specific period of record (POR) for each analysis, tau, Sen slope (change per year), and minimum, median, and maximum values. Trends found to be significant after q-value correction (q < 0.05) are highlighted in bold, italic type. NA: either data were not available or available data did not meet minimum density criteria (see text); NC: Sen slope not calculated. (Continued)
For each of the 32 selected weather stations, daily precipitation data were used to calculate monthly cumulative precipitation values, and these monthly totals were grouped by calendar year. Because each reservoir had at least 2 and as many as 4 weather stations, monthly cumulative precipitation values for each station were pooled by reservoir prior to analysis. Then, for each reservoir, quantile regression was used to estimate trends in the 10th, 50th, and 90th quantiles of monthly precipitation over the POR (1965–2009). Trends in precipitation were expressed as estimated change in precipitation (in mm/yr) for each quantile over the POR. In addition, the 10th, 50th, and 90th quantiles of monthly cumulative precipitation over the maximum available POR (i.e., all years combined) were estimated, and trends were then also expressed as the percent change relative to their respective overall quantile.
Principal component analysis
PCA reduces complex datasets of potentially correlated, multiple variables to a lower number of orthogonal variables (principal components [PCs]) without much loss of information and is especially useful for pattern exploration (Ringnér Citation2008). In this study, PCA was used to examine geographic and seasonal patterns of reservoir water quality distribution. Because of insufficient data for some variables, PCA was possible with only 10 of the 11 reservoirs and had to be limited to chloride, dissolved oxygen, pH, specific conductance, sulfate, temperature, and total phosphorus. Buffalo Springs Lake could not be included in the analysis because of a general lack of sufficient data for most variables. Approximate normality of these variables was achieved by transformation to either natural log (chloride and sulfate) or square-root (phosphorus and specific conductance) as necessary. Dissolved oxygen, pH, and temperature were not transformed.
The PCAs were performed using function “prcomp” in R. The Kaiser criterion (eigenvalue ≥1.0), Cattell scree test plot (elbow cutoff), and cumulative variance (≥75%) were used to select principal components (PCs) for interpretation. The importance of the contribution of each variable to individual PCs was assessed by their loading factors, and only those with a value ≥ |0.4| are interpreted in this study. To facilitate identification of patterns in data distribution, 95% confidence ellipses (standard error [SE]) were drawn around data clustered according to selected grouping variables. Grouping variables for this study included basin (Colorado River or Brazos River), season (winter, Dec–Feb; spring, Mar–May; summer, Jun–Aug; fall, Sep–Nov), and individual reservoir.
Results
Trends
Results of trend analyses for water quality did not reveal many patterns that were widespread geographically or consistent in the direction of change. Notable exceptions included total phosphorus, which significantly increased during the POR in 7 of 8 reservoirs (∼88% of reservoirs) with sufficient data for analysis. In the 7 reservoirs where change was detected, their Sen slopes were 0.001 mg/L per year ( and ). E.V. Spence Reservoir was the only reservoir analyzed that did not show a significant trend in total phosphorus.
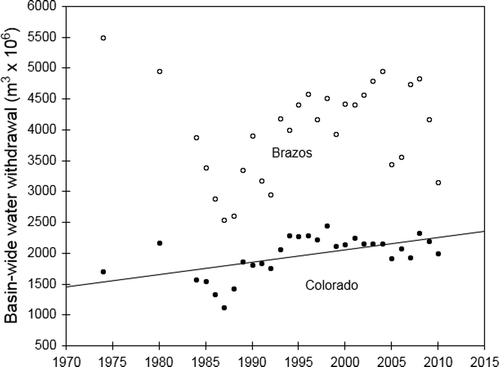
Overall temperature significantly increased in 4 reservoirs (Sen slope, 0.033–0.048 C/yr), significantly decreased in 1 reservoir (Lake Colorado City; Sen slope, −0.086 C/yr), and did not significantly change in 6 other reservoirs. The warmest-monthly temperature of the year significantly increased in 4 reservoirs (Sen slope, 0.029–0.035 C/yr), significantly decreased in 1 reservoir (Lake Colorado City; Sen slope, −0.085 C/yr), and did not significantly change in the other 6 reservoirs. The coldest-monthly temperature significantly increased in 2 reservoirs (Sen slopes, 0.046–0.070 C/yr), but did not significantly change in the other 9 reservoirs ( and ). Overall, 7 of 11 reservoirs (64%) experienced increases in at least one of the temperature attributes examined. Lake Travis was the only reservoir where the median, warmest-, and coldest-monthly temperatures of the year all increased during the POR.
Other water quality variables seemed to show inconsistent trends among reservoirs ( and ). For example, specific conductance significantly decreased in 3 reservoirs, significantly increased in 2, and did not significantly change in 5; Buffalo Springs Lake had insufficient data for specific conductance. Chloride significantly decreased in 3 reservoirs and significantly increased in 3 reservoirs, but no significant change was observed in the other 5 reservoirs. Sulfate showed significant decreases in 7 reservoirs and significant increases in 3 reservoirs, and only 1 reservoir did not exhibit a significant change in sulfate. Dissolved oxygen significantly decreased in 2 reservoirs, significantly increased in 2 reservoirs, and did not significantly change in 6 reservoirs; Buffalo Springs Lake had insufficient data for dissolved oxygen. A significant decrease in pH was detected in only 1 reservoir, whereas pH significantly increased in 4 reservoirs and did not significantly change in 6 others. Other variables analyzed either did not change significantly, or if a variable did change, it did in ≤3 reservoirs ( and ).
Storage significantly decreased in 6 of 9 reservoirs (67%) with sufficient data for analysis. The Sen slope for these decreases ranged from −0.659 × 106 to −3.624 × 106 m3/yr which, based on respective median monthly storage values over the POR, is equivalent to a yearly percent change ranging from −0.1% at Lake Travis to −3.1% at Twin Buttes Reservoir. In the other reservoirs, storage increased in 1 (Lake Colorado City; Sen slope, 0.134 × 106 m3/yr; 0.7% change per year), did not significantly change in 2, and had insufficient data for analysis in 2 ( and ). Although the databases of this study contained insufficient data for storage analysis of Lake Buchanan (), using lake elevation as a proxy (available from http://www.lcra.org/water/river-and-weather/pages/historical-lake-levels.aspx), trend analysis based on either annual monthly means or medians (both calculated from monthly elevation averages) did not reveal significant changes between 1970 and 2010 (data not shown).
Total water withdrawals by year significantly increased in the Colorado River basin (Kendall tau = 0.34, P < 0.05) whereas in the Brazos River basin only a marginally significant increase was detected (Kendall tau = 0.22, 0.05 < P < 0.1; ). The estimated Sen slope for the Colorado River basin was 17.2 million m3/yr (equivalent to 0.8% per year; based on median year values). The estimated Sen slope for the Brazos River basin was 38.1 million m3 (0.9% per year).
Table 3 Results of quantile regression for detection of trends in annual reservoir inflow in the Brazos and Colorado river basins. Flow rate data were obtained from USGS gages on major streams upstream of reservoirs for the period of record (POR), which varied by gage. Distributions of daily average flow rates were constructed for each year of the POR, and annual changes (10−6 m3/s per year) in the 10th, 50th, and 90th quantiles were estimated (Estimated annual change). The 10th, 50th, and 90th quantiles (10−6 m3/s) were also estimated for the entire POR (Estimated for POR), and the estimated annual changes were also expressed as a percentage of this value (Percent annual change). Trends found to be significant after q-value correction (q < 0.05) are highlighted in bold type.
Table 4 Results of quantile regression for detection of trends in annual precipitation in the Brazos and Colorado river basins. Monthly precipitation totals were calculated from daily precipitation data obtained for the period of record (POR, 1965–2009) from the National Climatic Data Center for selected weather stations. Monthly totals were grouped by year, and annual changes (monthly sum in mm/yr) in the 10th, 50th, and 90th quantiles were estimated (Estimated annual change). The 10th, 50th, and 90th quantiles (monthly sum in mm) were also estimated for the entire period of record (Estimated for POR), and the estimated annual changes were also expressed as a percentage of this value (Percent annual change). Trends found to be significant after q-value correction (q < 0.05) are highlighted in bold type.
Quantile regression analysis detected significant trends in reservoir inflow at many of the streams associated with the present study reservoirs (). Inflow trends for the Brazos River reservoirs were mixed between positive and negative for each of the quantiles examined; however, trends in the 50th quantiles were largely negative (). Inflow trends for Colorado River reservoirs were more consistent and mostly negative, especially for reservoirs in the western region (upper reaches) of the basin ().
Generally, median and extreme precipitation quantiles estimated for the entire POR (1965–2009) showed the expected spatial (west-to-east) gradient of increasing precipitation in both basins (; ). In addition, precipitation quantiles were generally of higher value in the Brazos than Colorado river basins (), thus also exhibiting a north-to-south geographic gradient of decreasing precipitation (). However, no significant temporal trends in precipitation were observed at any of the quantiles examined ().
Geographic and seasonal patterns in water quality
The first three PCs were retained for interpretation in the PCA, and together they explained 81% of the variance (). Specific conductance, chloride, and sulfate had the highest loading factors on PC1 and were positively associated with each other (), indicating that most of the water quality variance in the datasets is explained by the variance associated with total dissolved solids (hereafter referred to as salinity). Dissolved oxygen and temperature were the only contributing variables to PC2 and were inversely associated with each other (), which suggests that PC2 captured the variance associated with natural seasonal phenomena. For example, water temperature fluctuates seasonally, and solubility of gases is inversely related to water temperature (see also ). Total phosphorous and pH were inversely associated and had the highest loading factors on PC3 ().
Table 5 Principal component analysis of monthly water quality data. Loading factors are shown for each variable in the first three principle components (PC) retained by the analysis. Variables with loadings ≥ |0.4| are highlighted in bold type. Eigenvalues, and proportional and cumulative variance are shown for each PC.
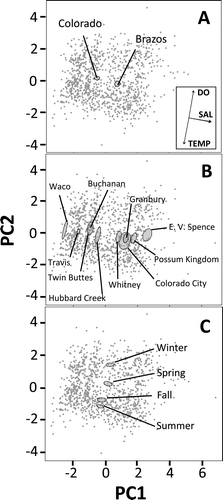
In PC2–PC1 biplots, 95% confidence ellipses of water quality data grouped by basin suggested an overall higher salinity for reservoirs in the Brazos River than those in the Colorado River (). Grouping of data by reservoir, however, indicated that this finding was due to the inclusion in the analysis of a greater number of high-salinity reservoirs for the Brazos River than the Colorado River basin. Possum Kingdom Lake, Lake Granbury, Whitney Lake (Brazos River), E.V. Spence Reservoir, and Lake Colorado City (Colorado River) are located at the higher end of the salinity axis (PC1), and Waco Lake, Hubbard Creek Reservoir (Brazos River), Lake Travis, Lake Buchanan, and Twin Buttes Reservoir (Colorado River) are at the lower end ().
In PC2–PC1 biplots, 95% confidence ellipses of water quality data grouped by season showed clear separation along the temperature–dissolved oxygen axis. Summer and winter ellipses were located at the opposite extremes of the inverse gradients ().
In PC3–PC1 biplots, 95% confidence ellipses of data grouped by season suggest that the inverse association between pH and total phosphorus along PC3 also reflects, at least in part, a seasonal phenomenon (). For example, water tended to have higher pH and lower total phosphorus during spring and summer than during fall and winter. In addition, 95% confidence ellipses of data grouped by reservoir showed that Waco Lake separated from other reservoirs along PC3, toward higher phosphorus (). None of the other grouping variables generated clearly interpretable patterns in the biplots (data not shown).
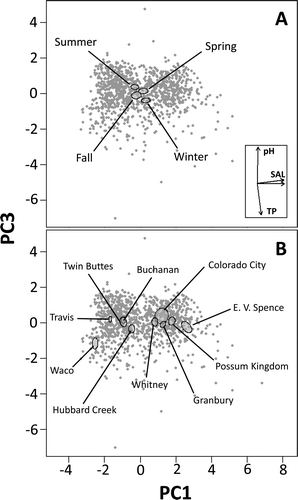
Discussion
Trends
Although the purpose of the present study was not to examine associations between reservoir water attributes and climate variability or change, examination of local precipitation trends was conducted to assist in the interpretation of reservoir water attributes. Generally, no significant trends in median (50th quantile) or extreme (10th and 90th quantiles) levels of precipitation were detected during the present study period (1965–2009) at the selected weather stations. A separate analysis for the same weather stations using a longer POR, 1945–2009, however, yielded significant positive trends in median and extreme quantiles of monthly rainfall near most reservoirs (data not shown). The difference in results between the two PORs could simply be that more data coupled with a longer POR enhances statistical power. Regardless of statistical significance, however, all rainfall trends at the 50th quantiles for the longer POR (1945–2009) (data not shown) and the majority for the shorter POR (1965–2009) were positive. These retrospective observations agree with those of Nielsen-Gammon (Citation2011) and Dixon and Moore (Citation2011) whose analyses, based on different approaches to data transformation and trend analyses, also suggested the occurrence of generally positive precipitation trends in Texas throughout much of the 20th century especially along and east of the north–south corridor from Oklahoma to south Texas.
Because no significant changes in local precipitation were detected that correspond to contemporaneous changes in reservoir inflow or storage, observed decreases in the inflow or storage in the majority of reservoirs of the present study are likely attributable to factors other than precipitation. This study revealed a significant, positive trend in total water withdrawals in the Colorado River basin between 1974 and 2010. In addition, although the trend in water withdrawals was only marginally significant in the Brazos River basin, its direction was also positive and its relative magnitude (0.9% per year) was similar to that observed in the Colorado River basin (0.8% per year). Consistent with these observations, the population of Texas nearly tripled between the 1960s and 2000s, from 9.6 million in 1960 to 24.8 million in 2009 (USCB Citation2013a, Citation2013b). Overall, these observations suggest that anthropogenic withdrawals may have been partly associated with reduced inflow and storage in those reservoirs where negative trends were detected. Factors not directly examined in this study, however, such as changes in reservoir operations, runoff, land use, groundwater-surface water interactions, and evaporation, also may have affected reservoir inflow and storage levels during the study period.
Recent studies of the impacts of global warming in Texas (Nielsen-Gammon Citation2011) indicate that air temperatures generally increased throughout the state between 1900 and 2000, and particularly from about 1960 to 2000. Higher air temperatures can result in higher water temperatures (Tibby and Tiller Citation2007, Gelca et al. Citation2015), higher evaporation rates (Nielsen-Gammon Citation2011), and increased water use by humans, all of which could result in less available inflow and storage. In the present study, 7 reservoirs showed increases in overall, warmest-, or coldest-monthly temperatures of the year whereas only 1 showed a decrease and 3 showed no significant change. This observation is consistent with a scenario where temperature-dependent increases in rates of evaporation and water use may have contributed to decreased storage in at least some of the reservoirs examined. Note that the temperature profile of the only reservoir that showed a decreasing trend in temperature, Lake Colorado City, has been artificially influenced by its past use as source of cooling water for a thermal power plant (Harbeck et al. Citation1958).
Despite the decreased inflows and storage observed in many of the reservoirs, results of the present study did not reveal the existence of long-term, monotonic trends in salinity or other water quality variables that were consistent across reservoirs, except for the generally positive trend in total phosphorus concentrations. Although reservoirs of this study could be clearly classified according to their level of salinity, trend analysis showed that salinity did not monotonically change in 5 reservoirs, decreased in 3 reservoirs, and increased in only 2 reservoirs over the study period. Using a different approach to trend analysis (linear regression), earlier PORs, and monitoring stations downstream of reservoirs, Wurbs et al. (1993) also reported the absence of salinity trends in many reservoirs of the Brazos River basin, including several examined in the present study.
The effects of natural or anthropogenic processes on reservoir salinity are not well understood, and changes in land use and climate may interact to affect lake (reservoir) water chemistry in a nonlinear fashion or even to neutralize each other (Adrian et al. Citation2009). This complex relation makes efforts to identify long-term trends in reservoir water chemistry and their underlying causes difficult; however, transient associations between rainfall, inflow, reservoir level, and salinity can be observed at shorter time scales. Recent studies of reservoirs in the upper reaches of the Colorado River, including some used in the present study, found that storage decreased and salinity increased during the drought of late 2010 through 2011, likely from a combination of low inflows (Winters Citation2013), high evaporation, and anthropogenic withdrawals (VanLandeghem et al. 2015a).
Nutrient concentrations typically increase with reservoir age, and this process can be greatly accelerated by anthropogenic activities (Rast and Holland Citation1988). The positive, monotonic trend in total phosphorus observed in the majority of the reservoirs of this study is therefore consistent with expectations. The only reservoir that showed no trend in total phosphorus was Lake E.V. Spence, which has been the target of a restoration effort to reduce its level of total dissolved solids (TCEQ Citation2011b), a process that may also have reduced nutrient inputs. The widespread positive trend in phosphorus documented in reservoirs of this study is consistent with and explains the present classification of most Texas reservoirs (90%) as eutrophic to hypereutrophic (TCEQ Citation2011b). Anthropogenic nutrient loading into streams and reservoirs varies depending on land-use practices within watersheds, with agricultural activity and wastewater effluent generally being among the most important contributors. The Brazos River and Colorado river basins contain a mix of rural and urban activities within their basins. Previous studies have shown that anthropogenic nutrient loading in some reservoirs of the present study is based on point sources such as wastewater effluent (Lake Granbury: Riebschleager et al. Citation2012; Waco Lake: TCEQ Citation2013) or to nonpoint, upstream drainage (Possum Kingdom Lake: USEPA Citation1977). Total phosphorus in most study reservoirs increased at a rate of about 0.001 mg/L/yr over the study POR, and median concentrations (0.02–0.08 mg/L) generally exceeded the aggregate recommended standards for reservoirs within their corresponding ecoregions (USEPA Citation2000, Citation2001).
Geographic and seasonal patterns in water quality
Variables reflecting or strongly associated with salinity (specific conductance, chloride, and sulfate) defined the variance structure of the first component of the PCA. This observation indicates that salinity is the primary water quality trait that differentiates reservoirs in the Brazos and Colorado river basins and also conforms to the known distribution of streamflow and reservoir salinity in these basins. The PCA also showed that variables strongly associated with season defined the variance of PC2, with temperature and dissolved oxygen being inversely associated with each other. Total phosphorus and pH were the primary variables contributing to the variance structure of PC3; generally, total phosphorus seemed to be lowest and pH highest in the summer and, conversely, total phosphorus was highest and pH lowest in winter. These observations are consistent with well-documented seasonal patterns in these variables associated with annual cycles in algal production (Driscoll and van Dreason Citation1993, Singh et al. Citation2004, Wetzel Citation2001).
At the level of individual reservoirs, most reservoirs did not separate from each other on the basis of total phosphorus or pH except for Waco Lake, which is clearly separated from all other reservoirs by its higher phosphorus concentration. This observation is not unexpected because the main tributary to Waco Lake, the North Bosque River, until recently carried high loads of nutrients into the lake from municipal wastewater effluent and concentrated animal feeding operations (Wagner Citation2010, TCEQ Citation2013).
Overall, the present observations indicate that salinity differences among individual reservoirs as well as seasonal changes in temperature, dissolved oxygen, total phosphorus, and pH are the primary drivers for the geographic and seasonal patterns of water quality observed in reservoirs of the Brazos and Colorado river basins.
Conclusions
Management operations and their changes through time can impact storage and water quality in a reservoir-specific fashion. While the influence of reservoir operations was not assessed in the present study, the finding of spatial and temporal patterns across a majority of reservoirs for some water attributes indicates that other factors are also important drivers of these attributes. Water quality variables that consistently changed in the majority of reservoirs with sufficient data for analyses include total phosphorus (increase) and, to a lesser degree, temperature (increase). The positive trend in total phosphorus is consistent with and explains the high levels of eutrophication observed in the majority of Texas reservoirs, including reservoirs outside the current study basins (TCEQ Citation2011b). This trend may have abated in recent years, however; an analysis based on only the last 20 years of the same database used in the present study did not detect significant trends in total phosphorus (Patiño et al. Citation2014). The most consistent pattern observed for salinity was one of no monotonic change, and when change was detected, its direction was inconsistent among reservoirs. Specifically, increased salinity was observed in only 2 reservoirs. Shorter-term, supra-annual fluctuations in salinity have been documented in the study reservoirs, however, and are likely to continue in the future as a function of climate variability.
The Brazos and Colorado river basins both contain relatively high- and low-salinity reservoirs. Although all of the higher-salinity reservoirs have experienced toxic blooms of golden algae (Southard et al. Citation2010, Roelke et al. Citation2012, VanLandeghem et al. Citation2013, Patiño et al. Citation2014), none of the lower-salinity reservoirs were affected during the study POR. Because salinity is a key indicator of golden algal bloom potential (Patiño et al. Citation2014), it is encouraging that most of the lower-salinity reservoirs examined in this study did not show signs of increasing salinity, and 2 (Lakes Buchanan and Travis) actually showed slight decreases during the study POR.
The human population and corresponding water demand in Texas are projected to increase by 82% and 21%, respectively, between 2010 and 2060, and estimates indicate that existing water supplies are already insufficient to meet water demands during times of drought (TWDB Citation2012). It is therefore of concern that monotonic reductions in reservoir inflow and storage were observed in the majority of study reservoirs in the absence of detectable, contemporaneous trends in precipitation. If current climate-change model projections of overall decreases in precipitation for the southern Great Plains (Melillo et al. Citation2014) prove to be correct, the geographic scope and severity of negative trends in reservoir inflow and storage could increase. Given the complex nature of watershed–reservoir interactions, factors driving the changes in reservoir storage and water quality will likely remain an open research question into the foreseeable future. Results of this study may help reservoir managers identify which factors warrant additional research.
Acknowledgments
The authors acknowledge Dr. Ahm A. Reza for his contributions during the exploratory phases of the project. Useful comments on a draft manuscript were provided by Dr. Steve Miranda, Mississippi Cooperative Fish and Wildlife Research Unit. The authors acknowledge David Lorenz with US Geological Survey Minnesota Water Science Center for an early review of the nonparametric Seasonal Kendall Tau test. John Burch provided waterquality data from the Colorado River Municipal Water District, and Bob Huber and David Walker provided inflow data for Lake Travis from the Lower Colorado River Authority. Weather stations were selected in collaboration with Drs. Katharine Hayhoe and Rodica Gelca, Texas Tech University.
Funding
This study was funded by US Geological Survey National Climate Change and Wildlife Science Center. The Texas Cooperative Fish and Wildlife Research Unit is sponsored by US Geological Survey, Texas Tech University, Texas Parks and Wildlife Department, The Wildlife Management Institute, and US Fish and Wildlife Service. Any use of trade, firm, or product names is for descriptive purposes only and does not imply endorsement by the US Government.
Appendix A: Custom R Scripts
Disclaimer: Although this software program has been used by the US Geological Survey (USGS), no warranty, expressed or implied, is made by the USGS or the US Government as to the accuracy and functioning of the program and related program material nor shall the fact of distribution constitute any such warranty, and no responsibility is assumed by the USGS in connection therewith.
Seasonal Kendall tau function
This script was written by William H. Asquith to conduct Seasonal Kendall Tau analyses using estimated monthly values of water quality variables based on algorithmic methods described by Helsel and Hirsch (2002, p. 338–339). The Seasonal Kendall's Tau through an independently developed code base is available by McLeod (2011). Numerical congruency of the Seasonal Kendall Tau analyses between the code reproduced here and the Kendall R package by McLeod (2011) and to the USGSwsStats R package by David Lorenz (USGS, written communication, 2013) can be shown.
Note: This script is designed to accept tabular data in the following example format:
Listing of R code for Seasonal Kendall's Tau
′′seasonalKendallYearMonth′′ <-
function(YMV, tiefix=FALSE) {
if(! is.data.frame(YMV)) stop(′′YMV needs to be a data frame.′′);
Sk <- N <- sigSk <- tie.correction <- 0;
for(m in sort(unique(YMV$Month))) {
ties <- 0;
YV <- YMV[YMV$Month == m,]; ox <- complete.cases(YV);
YV <- YV[ox,]; X <- YV$Year; Y <- YV$Value;
nm <- length(Y);
if(nm < 2) next; # go to next month
nunique <- nm*(nm-1)/2;
tmpterm <- (nm/18)*(nm-1)*(2*nm+5);
for(i in 1:(nm-1)) {
for(j in (i+1):nm) {
delta <- (Y[j] - Y[i])/(X[j] - X[i]);
shift <- NA;
shift <- ifelse(delta == 0 | ! is.finite(delta), 0,
ifelse(delta > 0, 1, -1));
Sk <- Sk + shift;
}
}
N <- N + nunique;
if(tiefix) {
tie.h <- new.env(hash=TRUE); # initialize
#message(′′month= ′′,m,′′\n′′); print(sort(Y)); print(sort(unique(Y)))
′′dualFOR′′ <- function(AVAR) {
for(avar in unique(AVAR)) {
tie.extent <- length(AVAR[AVAR == avar]);
tie.key <- as.character(tie.extent);
if(exists(tie.key, tie.h)) {
tmp <- get(tie.key, tie.h);
assign(tie.key, tmp + 1, tie.h);
} else {
assign(tie.key, 1, tie.h);
}
}
}
dualFOR(X); dualFOR(Y); # check ties in X and Y
tie.extents <- ls(tie.h); # list of identified tie extents
for(a.tie in tie.extents) {
n.ties <- get(a.tie, tie.h); # how many of each extent found
a.tie <- as.numeric(a.tie); #print(a.tie); print(n.ties);
ties <- ties + n.ties*a.tie*(a.tie-1)*(2*a.tie+5)/18;
}
tie.correction <- tie.correction + ties;
}
sigSk <- sigSk + tmpterm - ties; # ties is ZERO if tiefix=FALSE
}
sigSk <- sqrt(sigSk); # finally compute standard deviation of Sk
shift <- ifelse(Sk == 0, 0, ifelse(Sk > 0, -1, 1));
ZSk <- (Sk + shift)/sigSk;
pval <- 2 * (1 - pnorm(abs(ZSk))); # TWO TAILED 2*
my.mess <- ifelse(tiefix, ′′tie correction made′′,
′′tie correction not made′′);
my.tie <- ifelse(tiefix, tie.correction, NA);
ans <- list(tau=Sk/N, pvalue=pval, Sk=Sk,
Z=ZSk, sigmaSk=sigSk,
tie.correction=my.tie, tiefix=my.mess,
message=′′two-tailed test′′);
return(ans);
}
# Example of useage:
seasonalKendallYearMonth(DATATABLE)
seasonalKendallYearMonth(DATATABLE, tiefix=TRUE)
Sen Slope calculator for data analyzed with Seasonal Kendall Tau
This script, written by Dan Dawson and Matt VanLandeghem, was created by modifying the script presented in A-1. This script computes the Sen Slopes of datasets analyzed with the Seasonal Kendall Tau analysis method. It will run data with the same format as required by A-1. However, to use the script with data in which entire months are missing from the dataset, all references to non-present months must be removed from the script. For example, if a dataset contains no January data, all references to January must be removed from the script prior to running it. The script consists of 2 main parts. The first part employs a function (SenEst) to compute slopes within months across years. The second part arranges the data and computes a median slope for the entire set that is output as an object called SenSlopeEst.
Listing of R code for Sen Slope
′′SenEst′′ <-
function(YMV, tiefix=FALSE) {
if(! is.data.frame(YMV)) stop(′′YMV needs to be a data frame.′′);
Sk <- N <- sigSk <- tie.correction <- 0;
for(m in sort(unique(YMV$Month))) {
ties <- 0;
YV <- YMV[YMV$Month == m,]; ox <- complete.cases(YV);
YV <- YV[ox,]; X <- YV$Year; Y <- YV$Value;
nm <- length(Y);
if(nm < 2) next; # go to next month
nunique <- nm*(nm-1)/2;
tmpterm <- (nm/18)*(nm-1)*(2*nm+5);
if (m == 1) {jan <- matrix(ncol = nm, nrow=nm)}
if (m == 2) {feb <- matrix(ncol = nm, nrow=nm)}
if (m == 3) {mar <- matrix(ncol = nm, nrow=nm)}
if (m == 4) {apr <- matrix(ncol = nm, nrow=nm)}
if (m == 5) {may <- matrix(ncol = nm, nrow=nm)}
if (m == 6) {jun <- matrix(ncol = nm, nrow=nm)}
if (m == 7) {jul <- matrix(ncol = nm, nrow=nm)}
if (m == 8) {aug <- matrix(ncol = nm, nrow=nm)}
if (m == 9) {sep <- matrix(ncol = nm, nrow=nm)}
if (m == 10){oct <- matrix(ncol = nm, nrow=nm)}
if (m == 11){nov <- matrix(ncol = nm, nrow=nm)}
if (m == 12){dec <- matrix(ncol = nm, nrow=nm)}
for(i in 1:(nm-1)) {
for(j in (i+1):nm) {
delta <- (Y[j] - Y[i])/(X[j] - X[i]);
if (m == 1){jan[i,j-1] <-delta}
if (m == 2){feb[i,j-1] <-delta}
if (m == 3){mar[i,j-1] <-delta}
if (m == 4){apr[i,j-1] <-delta}
if (m == 5){may[i,j-1] <-delta}
if (m == 6){jun[i,j-1] <-delta}
if (m == 7){jul[i,j-1] <-delta}
if (m == 8){aug[i,j-1] <-delta}
if (m == 9){sep[i,j-1] <-delta}
if (m == 10){oct[i,j-1] <-delta}
if (m == 11){nov[i,j-1] <-delta}
if (m == 12){dec[i,j-1] <-delta}
shift <- NA;
shift <- ifelse(delta == 0 | ! is.finite(delta), 0,
ifelse(delta > 0, 1, -1));
Sk <- Sk + shift;
} #end of j loop
} #end of i loop
N <- N + nunique;
if(tiefix) {
tie.h <- new.env(hash=TRUE); # initialize
#message(′′month=′′,m,′′\n′′); print(sort(Y)); print(sort(unique(Y)))
′′dualFOR′′ <- function(AVAR) {
for(avar in unique(AVAR)) {
tie.extent <- length(AVAR[AVAR == avar]);
tie.key <- as.character(tie.extent);
if(exists(tie.key, tie.h)) {
tmp <- get(tie.key, tie.h);
assign(tie.key, tmp + 1, tie.h);
} else {
assign(tie.key, 1, tie.h);
}
}
}
dualFOR(X); dualFOR(Y); # check ties in X and Y
tie.extents <- ls(tie.h); # list of identified tie extents
for(a.tie in tie.extents) {
n.ties <- get(a.tie, tie.h); # how many of each extent found
a.tie <- as.numeric(a.tie); #print(a.tie); print(n.ties);
ties <- ties + n.ties*a.tie*(aSe.tie-1)*(2*a.tie+5)/18;
}
tie.correction <- tie.correction + ties;
}
sigSk <- sigSk + tmpterm - ties; # ties is ZERO if tiefix=FALSE
}
sigSk <- sqrt(sigSk); # finally compute standard deviation of Sk
shift <- ifelse(Sk == 0, 0, ifelse(Sk > 0, -1, 1));
ZSk <- (Sk + shift)/sigSk;
pval <- 2 * (1 - pnorm(abs(ZSk))); # TWO TAILED 2*
my.mess <- ifelse(tiefix, ′′tie correction made′′,
′′tie correction not made′′);
my.tie <- ifelse(tiefix, tie.correction, NA);
ans <- list(tau=Sk/N, pvalue=pval, Sk=Sk,
Z=ZSk, sigmaSk=sigSk,
tie.correction=my.tie, tiefix=my.mess,
message=′′two-tailed test′′, JAN = jan, FEB = feb, MAR = mar, APR = apr,
MAY=may, JUNE=jun, JULY=jul,AUG=aug, SEP=sep, OCT=oct, NOV=nov, DEC=dec)
return(ans);
SenEst(TMP)
} #end of first function function
′′SenMedianCalc′′ <- function(x) {
SenMedian <-(SenEst(TMP))
attach(SenMedian)
jan=as.vector(JAN)
feb=as.vector(FEB)
mar=as.vector(MAR)
apr=as.vector(APR)
may=as.vector(MAY)
june=as.vector(JUNE)
july=as.vector(JULY)
aug=as.vector(AUG)
sep=as.vector(SEP)
oct=as.vector(OCT)
nov=as.vector(NOV)
dec=as.vector(DEC)
jan.na.omit<-as.vector(na.omit(jan))
feb.na.omit<-as.vector(na.omit(feb))
mar.na.omit<-as.vector(na.omit(mar))
apr.na.omit<-as.vector(na.omit(apr))
may.na.omit<-as.vector(na.omit(may))
june.na.omit<-as.vector(na.omit(june))
july.na.omit<-as.vector(na.omit(july))
aug.na.omit<-as.vector(na.omit(aug))
sep.na.omit<-as.vector(na.omit(sep))
oct.na.omit<-as.vector(na.omit(oct))
nov.na.omit<-as.vector(na.omit(nov))
dec.na.omit<-as.vector(na.omit(dec))
allslopes<-as.vector(c(jan.na.omit, feb.na.omit, mar.na.omit, apr.na.omit,
may.na.omit, june.na.omit, july.na.omit, aug.na.omit, sep.na.omit, oct.na.omit,
nov.na.omit, dec.na.omit))
ans<-c(allslopes)
return(ans)
}
allslopes<-SenMedianCalc(TMP)
SenSlopeEst<-as.vector(median(allslopes))
SenSlopeEst
References
- Adrian R, O’Reilly CM, Zagarese H, Baines SB, Hessen DO, Keller W, Livingstone DM, Sommaruga R, Straile D, Van Donk E, et al. 2009. Lakes as sentinels of current climate change. Limnol Oceanogr. 54:2283–2297.
- Allen MS, Sammons S, Maceina MJ. 2008. Balancing fisheries management and water uses for impounded river systems: An introduction. In: Allen MS, Sammons S, Maceina MJ, editors. Balancing fisheries management and water uses for impounded river systems. American Fisheries Society Symposium 62, Bethesda, MD, 1–3.
- Asquith WH, Barbie DL. 2014. Trend analysis and selected summary statistics of annual mean streamflow for 38 selected long-term U.S. Geological Survey streamgages in Texas, water years 1916–2012. US Geological Survey Scientific Investigations Report 2013–5230.
- Asquith WH, Heitmuller FT. 2008. Summary of annual mean and annual harmonic mean statistics of daily mean streamflow for 620 U.S. Geological Survey streamgages in Texas through water year 2007. US Geological Survey Data Series 372. 1259 p.
- Austin JA, Colman SM. 2007. Lake Superior summer water temperatures are increasing more rapidly than regional air temperatures: a positive ice-albedo feedback. Geophys Res Lett. 34:L06604.
- Baker RC, Hughes LS, Yost ID. 1964. Natural sources of salinity in the Brazos River, Texas, with particular reference to the Croton and Salt Croton Creek Basins. US Geological Survey Water-Supply Paper 1669-CC. 81 p.
- Bonte M, Zwolsman JJG. 2010. Climate change induced salinisation of artificial lakes in the Netherlands and consequences for drinking water production. Water Res. 44:4411–4424.
- [BRA] Brazos River Authority. 2012. The Brazos River; [cited 9 May 2014]. Available from: http://www.brazos.org/About_the_Brazos_River.asp
- Burley TE, Asquith WH, Brooks DL. 2011. Spatially pooled depth-dependent reservoir storage, elevation, and water-quality data for selected reservoirs in Texas, January 1965–January 2010. US Geological Survey Data Series 594. 13 p.
- Cleveland WS. 1979. Robust locally weighted regression and smoothing scatterplots. J Am Stat Assoc. 74:829–836.
- Dabney A, Storey JD, Warnes GR. 2011. qvalue: q-value estimation for false discovery rate control. R package v1.26.0; [cited 9 May 2014]. Available from: http://www.bioconductor.org/packages/release/bioc/html/qvalue.html
- Dillon PJ, Kirchner WB. 1975. The effects of geology and land use on the export of phosphorus from watersheds. Water Res. 9:125–148.
- Dixon RW, Moore TW. 2011. Trend detection in Texas temperature and precipitation. Southwest Geogr. 15:80–103.
- Downing JA, Plante C. 1993. Production of fish populations in lakes. Can J Fish Aquat Sci. 50:110–120.
- Driscoll CT, van Dreason R. 1993. Seasonal and long-term temporal patterns in the chemistry of Adirondack lakes. Water Air Soil Pollut. 67:319–344.
- Foley JA, DeFries R, Asner GP, Barford C, Bonan G, Carpenter SR, Chapin FS, Coe MT, Daily GC, Gibbs HK, et al. 2005. Global consequences of land use. Science. 309:570–574.
- Forbes MG, Doyle RD, Scott JT, Stanley JK, Huang H, Brooks BW. 2008. Physical factors control phytoplankton production and nitrogen fixation in eight Texas reservoirs. Ecosystems. 11:1181–1197.
- Gelca R, Hayhoe K, Scott-Fleming I, Crow C, Dawson D, Patiño R. 2015. Climate-water quality relationships in Texas reservoirs. Hydrol Process. DOI: 10.1002/hyp.10545
- Hambright KD, Zamor RM, Easton JD, Glenn KL, Remmel EJ, Easton AC, 2010. Temporal and spatial variability of an invasive toxigenic protist in a North American subtropical reservoir. Harmful Algae. 9:568–577.
- Harbeck GE, Koberg GE, Highes GH. 1958. The effect of the addition of heat from a powerplant on the thermal structure and evaporation of Lake Colorado City, Texas. US Geological Survey, Professional Paper 272-B. 51 p. [cited 9 May 2014]. Available from: http://pubs.usgs.gov/pp/0272b/report.pdf
- Helsel DR, Hirsch RM. 2002. Statistical methods in water resources. US Geological Survey techniques of water resources investigations, Book 4, Chapter A3. 522 p.
- Hirsch RM, Slack JR, Smith RA. 1982. Techniques of trend analysis for monthly water-quality data. Water Resourc Res. 18:107–121.
- Kaushal SS, Groffman PM, Likens GE, Belt KT, Stack WP, Kelly VR, Band LE, Fisher GT. 2005. Increased salinization of fresh water in the northeastern United States. P Nat Acad Sci-USA. 102:13517–13520.
- Koenker R, Bassett GW. 1978. Regression quantiles. Econometrica. 46:33–50.
- Leifeste DK, Lansford MW. 1968. Reconnaissance of the chemical quality of the surface water of the Colorado River Basin, Texas. Austin (TX): Texas Water Development Board; [cited 9 May 2014]. Available from: http://www.twdb.state.tx.us/publications/reports/numbered_reports/doc/R71/r71_ColoradoRiver1968.pdf
- Lins HF, Slack JR. 1999. Stream flow trends in the United States. Geophys Res Lett. 26:227–230.
- McLeod AI. 2011. Kendall---Kendall rank correlation and Mann-Kendall trend test, R package version 2.2 dated May 16, 2011: accessed on August 13, 2015 at https://cran.r-project.org/web/packages/Kendall/Kendall.pdf
- Melillo JM, Richmond TTC, Yohe GW, editors. 2014. Climate change impacts in the United States: the third national climate assessment. US Global Change Research Program. 841 p.
- Migliaccio KW, Castro J, Haggard BE. 2011. Water quality statistical analysis. In: Li Y, Migliaccio KW, editors. Water quality concepts, aampling, and Analyses. Boca Raton (FL): CRC Press. p. 241–274.
- Miyazono S, Patiño R, Taylor CA. 2015. Desertification, salinization, and biotic homogenization in a dryland river ecosystem. Sci Total Environ. 511:444–453.
- Nielsen-Gammon JW. 2011. The changing climate of Texas. In: Schmandt J, North GR, Clarkson J, editors, The impact of global warming on Texas. Austin (TX): University of Texas Press. p. 39–68.
- O’Neil JM, Davis TW, Burford MA, Gobler CJ. 2012. The rise of harmful cyanobacteria blooms: the potential roles of eutrophication and climate change. Harmful Algae. 14:313–334.
- Paerl HW, Hall NS, Calandrino ES. 2011a. Controlling harmful cyanobacterial blooms in a world experiencing anthropogenic and climatic-induced change. Sci Total Environ. 409:1739–1745.
- Paerl HW, Xu H, McCarthy MJ, Zhu G, Qin B, Li Y, Gardner WS. 2011b. Controlling harmful cyanobacterial blooms in a hyper-eutrophic lake (Lake Taihu, China): the need for a dual nutrient (N & P) management strategy. Water Res. 45:1973–1983.
- Paine JG, Collins EW, Nance HS. 2005. Geophysical investigations of salinization along the Upper Colorado River between Lake Thomas and Ivie Reservoir, Texas. Texas Bureau of Economic Geology, Draft Report for Texas Commission on Environmental Quality under Contract No. 582-4-56385. 110 p. [cited 25 September 2014]. Available from: http://www.tceq.texas.gov/assets/public/waterquality/tmdl/32colorado/32-beg_colorado_final.pdf
- Patiño R, Dawson D, VanLandeghem MM. 2014. Retrospective analysis of associations between water quality and toxic blooms of golden alga (Prymnesium parvum) in Texas reservoirs: implications for understanding dispersal mechanisms and impacts of climate change. Harmful Algae. 33:1–11.
- Petrere M Jr. 1996. Fisheries in large tropical reservoirs in South America. Lake Reserv Res Manage. 2:111–133.
- Price K, Leigh DS. 2006. Comparative water quality of lightly- and moderately-impacted streams in the southern Blue Ridge Mountains, USA. Environ Monit Assess. 120:269–300.
- R Development Core Team. 2010. R: a language and environment for statistical computing. Vienna (Austria): R Foundation for Statistical Computing; [cited 9 May 2014]. Available from: http://www.R-project.org/
- Rast W, Holland M. 1988. Eutrophication of lakes and reservoirs: a framework for making management decisions. Ambio. 17:2–12.
- Rawson J. 1967. Study and interpretation of the chemical quality of surface waters in the Brazos River Basin, Texas. Austin (TX): Texas Water Development Board. Report 55; [cited 9 May 2014]. Available from: http://www.twdb.state.tx.us/publications/reports/numbered_reports/doc/R55/R55.pdf
- Riebschleager KJ, Karthikeyan R, Srinivasan R, McKee K. 2012. Estimating potential E. coli sources in a watershed using spatially explicit modeling techniques. J Am Water Resour Assoc. 48:745–761.
- Ringnér M. 2008. What is principal component analysis? Nature Biotechnol. 26:303–304.
- Roelke DL, Brooks BW, Grover JP, Gable GM, Schwierzke-Wade L, Hewitt NC. 2012. Anticipated human population and climate change effects on algal blooms of a toxic haptophyte in the south-central USA. Can J Fish Aquat Sci. 69:1389–1404.
- Sahoo GB, Schladow SG, Reuter JE, Coats R. 2011. Effects of climate change on thermal properties of lakes and reservoirs, and possible implications. Stoch Env Res Risk Assess. 25:445–456.
- Sen PK. 1968. Estimates of the regression coefficient based on Kendall's tau. J Am Stat Assoc. 63:1379–1389.
- Shrestha RR, Dibike YB, Prowse TD. 2012. Modelling of climate-induced hydrologic changes in the Lake Winnipeg watershed. J Great Lakes Res. 38(Suppl. 3):83–94.
- Singh KP, Malik A, Mohan D, Sinha S. 2004. Multivariate statistical techniques for the evaluation of spatial and temporal variations in water quality of Gompti River (India)–a case study. Water Res. 38:3980–3992.
- Southard GM, Fries LT, Barkoh A. 2010. Prymnesium parvum: the Texas experience. J Am Water Resour Assoc. 46:14–23.
- Storey JD. 2002. A direct approach to false dicovery rates. J Roy Stat Soc B: 64: 479–498.
- Storey JD. 2003. The positive false discovery rate: a bayesian interpretation and the q-value. Ann Stat. 31:2013–2035.
- Storey JD, Tibshirani R. 2003. Statistical significance for genomewide studies. P Nat Acad Sci-USA: 100:9440–9445.
- Tadonleke RD, Lazzarotto J, Anneville O, Druart JC. 2009. Phytoplankton productivity increased in Lake Geneva despite phosphorus loading reduction. J Plankt Res. 31:1179–1194.
- [TCEQ] Texas Commission on Environmental Quality. 2011a. 2010 Texas integrated report for Clean Water Act Sections 305(b) and 303(d): executive Summary. Austin (TX): Texas Commission on Environmental Quality; [cited 9 May 2014]. Available from: http://www.tceq.texas.gov/assets/public/compliance/monops/water/10twqi/2010_exec_summ.pdf
- [TCEQ] Texas Commission on Environmental Quality. 2011b. Trophic classification of Texas reservoirs: 2010 Texas water quality inventory and 303(d) list. Austin (TX): Texas Commission on Environmental Quality; [cited 9 May 2014]. Available from: http://www.tceq.texas.gov/assets/public/compliance/monops/water/10twqi/2010_reservoir_narrative.pdf
- [TCEQ] Texas Commission on Environmental Quality. 2013. Status report: implementing TMDLs to reduce phosphorus. Improving water quality in the North Bosque River. Austin (TX): Texas Commission on Environmental Quality; [cited 9 May 2014]. Available from:. http://www.tceq.texas.gov/assets/public/waterquality/tmdl/06bosque/06-BosqueStatus2013-06-13.pdf
- [TWDB] Texas Water Development Board. 2012. 2012 State water plan. Austin (TX): Texas Water Development Board. 299 p. [cited 9 May 2014]. Available from: https://www.twdb.state.tx.us/publications/state_water_plan/2012/2012_SWP.pdf
- Tibby J, Tiller D. 2007. Climate-water quality relationships in three Western Victorian (Australia) lakes 1984–2000. Hydrobiologia. 591:219–234.
- [USCB] US Census Bureau. 2013a. Intercensal estimates of the total resident population of states: 2000–2010; [cited 9 May 2014]. Available from: http://www.census.gov/popest/data/intercensal/state/state2010.html
- [USCB] US Census Bureau. 2013b. Population of counties by decennial census: 1900 to 1990; [cited 9 May 2014]. Available from: http://www.census.gov/population/cencounts/tx190090.txt
- [USEPA] US Environmental Protection Agency. 1977. Report on Possum Kingdom Reservoir, Palo Pinto, Stephens, and Young counties, Texas. Washington (DC): EPA Region VI, National Eutrophication Survey. Working Paper Series 655.
- [USEPA] US Environmental Protection Agency. 2000. Ambient water quality criteria recommendations: lakes and reservoirs in Nutrient Ecoregion IX. Washington (DC): EPA 822-B-00-011; [cited 9 May 2014]. Available from: http://www2.epa.gov/sites/production/files/documents/lakes9.pdf
- [USEPA] US Environmental Protection Agency. 2001. Ambient water quality criteria recommendations: lakes and reservoirs in Nutrient Ecoregion V. Washington (DC): EPA 822-B-01-010; [cited 9 May 2014]. Available from: http://www2.epa.gov/sites/production/files/documents/lakes5.pdf
- [USGS] US Geological Survey. 2013. Current conditions for Texas: streamflow; [cited 13 August 2013]. Available from: http://waterdata.usgs.gov/tx/nwis/current/?type=flow
- VanLandeghem MM, Farooqi M, Farquhar B, Patiño R. 2013. Impacts of golden alga Prymnesium parvum on fish populations in reservoirs of the Upper Colorado River and Brazos River basins, Texas. T Am Fish Soc. 142:581–595.
- VanLandeghem MM, Farooqi M, Southard G, Patiño R. 2015a. Associations between water physicochemistry and Prymnesium parvum presence, abundance, and toxicity in west Texas reservoirs. J Am Water Res Assoc. 51:471–486.
- VanLandeghem MM, Farooqi M, Southard G, Patiño R. 2015b. Spatiotemporal associations of reservoir nutrient characteristics and the invasive, harmful alga Prymnesium parvum in west Texas. J Am Water Resour Assoc. 51:487–501.
- VanLandeghem MM, Meyer MD, Cox SB, Sharma B, Patiño R. 2012. Spatial and temporal patterns of surface water quality and ichthyotoxicity in urban and rural river basins in Texas. Water Res. 46:6638–6651.
- Wagner KJ. 2010. Loading of phosphorus and nitrogen to Lake Waco, Texas. Lake Reserv Manage. 26:123–146.
- Wetzel RG. 2001. Limnology: lake and river ecosystems. 3rd ed. San Diego (CA): Academic Press.
- Winters KE. 2013. A historical perspective on precipitation, drought severity, and streamflow in Texas during 1951–56 and 2011. US Geological Survey Scientific Investigations Report 2013–5113. 34 p.
- Wishart DJ, editor. 2011. Encyclopedia of the Great Plains. University of Nebraska-Lincoln; [cited 27 March 2015]. Available from: http://plainshumanities.unl.edu/encyclopedia/
- Wurbs RA, Karama AS, Saleh I, Ganze CK. 1993. Natural salt pollution and water supply reliability in the Brazos River Basin. College Station (TX): Texas A&M University, Texas Water Resources Institute. Technical Report No. 160; [cited 9 May 2014]. Available from: https://www.twdb.state.tx.us/publications/reports/contracted_reports/doc/92483332a.pdf
- Zhang QA, Xu CY, Chen YD. 2010. Variability of water levels and impacts of streamflow changes and human activity within the Pearl River Delta, China. Hydrol Sci J. 55:512–525.