ABSTRACT
This study tests the effects of environmental health risk messages on perceived risk, information needs and decisions to seek information, testing a reduced risk information seeking and processing model (R-RISP). Participants (N = 1,823) were randomized to one of three risk conditions (arsenic, bisphenol A [BPA] or volatile organic compounds [VOCs]) and one of the three message conditions (high threat, low threat or no message); participants in the high and low threat message conditions were also randomly assigned to a seeking cue to action condition (with or without seeking cue). Overall, the results support the R-RISP model, demonstrating the importance of current knowledge perceptions and informational subjective norms in information acquisition decisions. In addition, the results also provide initial evidence that environmental health risk messages can prompt information seeking and increase intentions to seek information in the future. Avenues for future research are discussed.
Introduction
We are increasingly faced with health and environmental threats that must be communicated to the public over extended periods of time to audiences with varying levels of preexisting knowledge. To maximize the effectiveness of health communication, particularly for risks still under investigation, a broader understanding of what drives people to engage with messages about these threats is needed, as well as knowledge of what prompts information acquisition in response. However, most studies examining information seeking rely on cross-sectional data drawn from self-reports of past seeking or seeking intention; thus, the factors that motivate individuals to start and suspend actual information acquisition behaviors at the time of an environmental health threat are less clear, including the effects of risk communication messages. Understanding the drivers of actual information seeking is important – particularly over time as information evolves and risk information needs change – as information seeking is a proposed antecedent of health-related attitudes and behaviors (Kontos et al., Citation2007) and is associated with beliefs and behavioral intentions (e.g., Bigsby & Hovick, Citation2018; Myrick, Citation2017; Shim et al., Citation2006), including intentions and decisions to engage in behaviors to reduce environmental health threats (Fitzpatrick-Lewis et al., Citation2010; Trumbo & O’Keefe, Citation2001, Citation2005).
To better understand the mechanisms driving seeking decisions and future seeking intentions, we tested a reduced version of the risk information seeking and processing (RISP) model proposed by Yang et al. (Citation2014). Studies of risk information seeking often revolve around the RISP model (Griffin et al., Citation1999) and planned risk information seeking model (PRISM; Kahlor, Citation2010), which propose that perceived health hazards, feelings of information insufficiency and seeking-related cognitions drive decisions to seek information. These frameworks can also provide insight into the cognitive and affective links between risk message exposure and information seeking and processing (Griffin et al., Citation2013).
In this study, we sought to investigate how risk messages impact seeking decisions. Only recently have researchers examined seeking behaviors in response to message cues and to incorporate, observe and measure the information search process (e.g., Hwang & Jeong, Citation2016; Myrick, Citation2017; So et al., Citation2019). Specifically, we sought to test whether exposure to a high threat (versus a low threat) environmental health message increased the likelihood of actual information seeking and intentions to seek information in the future.
So et al. (Citation2019), in an attempt to bridge divergent literatures in risk message effects and information seeking, showed that many individuals (44%) sought information following exposure to a risk message about a health topic. Their results provided support for predictions made in the RISP model and PRISM regarding key antecedents (i.e., perceived threat, anger, fear and uncertainty discrepancy) of information seeking. Furthermore, they showed information seeking mediated the effects of perceived susceptibility, fear and anxiety on response- and self-efficacy, as well as the effects of perceived susceptibility and fear on risk message acceptance, which suggests that information seeking may indeed help facilitate positive health outcomes (So et al., Citation2019).
Theoretical framework
This study draws on RISP model predictions because the model focuses on information seeking and processing about risk topics specifically, draws from multiple theoretical perspectives including the theory of planned behavior (Ajzen, Citation1991), heuristic-systematic model (Eagly & Chaiken, Citation1993) and theories of risk perception (outlined in Griffin et al., Citation2013), and has been widely used across health contexts. For example, the model has been used to examine environmental risk topics including water quality and contaminated fish consumption (Griffin et al., Citation2002; Kahlor et al., Citation2004), flooding (Griffin et al., Citation2008) and climate change (Kahlor, Citation2007), as well as topics such as clinical trial enrollment (Yang et al., Citation2010a) and H1N1 vaccination (Yang, Citation2012). The RISP model is comprised of seven major factors that predict information seeking and information processing behaviors: (a) individual characteristics, (b) perceived hazard characteristics (i.e., severity and susceptibility), (c) affective responses to hazard, (d) informational subjective norms (i.e., others expectations for our seeking), (e) perceived information insufficiency (i.e., the gap between perceived current and needed knowledge), (f) relevant channel beliefs and (g) perceived information gathering capacity (Griffin et al., Citation1999).
Although the RISP model performs reasonably well across studies, the “behavior of some model components waxes and wanes” (p. 24) based on the type of risk, measurement or other factors (Griffin et al., Citation2013); noted in particular was the less consistent performance of negative affect, information gathering capacity and channel beliefs. As a result, Yang et al. (Citation2014) conducted a meta-analysis to identify “more parsimonious explanations of information seeking and processing” (p. 26). Parsimony is an important aspect of theory development; “when competing theories with the same predictive power exist, the simplest theory or the one with the fewest number of assumptions (i.e., the more parsimonious theory) is preferred as it reduces the risk for error” (May, Citation2018, p. 1183). A more parsimonious RISP model, including the most robust predictors of seeking and processing, may allow for a more efficient use of the model (Braun & Niederdeppe, Citation2012; Yang et al., Citation2014).
Meta-analytic results showed that a reduced RISP (R-RISP) model composed of just two predictors, current knowledge perceptions and informational subjective norms, may be as powerful a tool for predicting information seeking and processing as the full RISP model (Yang et al., Citation2014). Thus, other RISP model variables (i.e., perceived hazard characteristics, affective response, channel beliefs, perceived information gathering capacity and individual characteristics) are not included in the R-RISP model. In response to a call for further testing of the R-RISP model (Yang et al., Citation2014), we test a model incorporating R-RISP predictions () to predict actual and intended information seeking in response to risk communication.
Figure 1. Study conceptual model and hypotheses based on the reduced risk information seeking and processing model (Yang et al., Citation2014) and risk information seeking and processing model (Griffin et al., Citation1999).
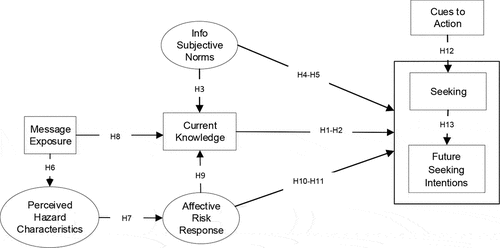
Current knowledge perceptions
Perceived information insufficiency is proposed to drive information seeking behaviors in the RISP model and PRISM. This prediction is rooted in the sufficiency principle (Chaiken et al., Citation1989; Eagly & Chaiken, Citation1993) or idea that people process or seek information at a minimally sufficient level to feel confident in their knowledge about an issue (Griffin et al., Citation2013). However, meta-analyses have revealed individuals’ perceived current knowledge, rather than their information sufficiency threshold, explained a larger proportion of variance in seeking and systematic processing, which may be due to the difficulty in estimating how much information one needs to manage a risk (Yang et al., Citation2014). More knowledgeable individuals may also be better equipped to comprehend and process information gained from seeking (Alba & Hutchinson, Citation1987).
Multiple studies have shown perceived knowledge to be positively related to intended or actual information seeking (e.g., Griffin et al., Citation2008; Yang et al., Citation2010b, Citation2011; Ter Huurne et al., Citation2009); this relationship suggests that perceptions of risk-related knowledge may motivate information acquisition, although some have shown no relationship (e.g., Hwang & Jeong, Citation2016; Kahlor et al., Citation2003). Studies in non-health contexts (e.g., Kerstetter & Cho, Citation2004; Khosrowjerdi & Iranshahi, Citation2011) also provide support for the knowledge and information seeking relationship. Therefore, we predict that perceptions of current knowledge will be positively associated with decisions to seek information (H1), as well future information seeking (H2) intentions.
Informational subjective norms
Within the R-RISP model, subjective norms predict current knowledge and information seeking. Informational subjective norms refer to perceived social expectations about information seeking (Griffin et al., Citation1999). Previous tests of the RISP model have provided support for informational subjective norms as a predictor of information seeking and processing (Griffin et al., Citation2013), which may be due to the fact that seeking norms is not specifically related to a risk context (Yang et al., Citation2014).
Studies testing the PRISM (Kahlor, Citation2010) further show subjective norms predict seeking intention (e.g., Kahlor, Citation2007, Citation2010) and knowledge (Kahlor, Citation2010; Willoughby & Myrick, Citation2016). A meta-analysis of 204 health-related studies also showed social norms have a reliable effect on intended and actual behaviors (Sheeran et al., Citation2016). Therefore, we expect subjective norms to be positively associated with perceptions of current knowledge (H3), as well as decisions to seek information following exposure to risk messages (H4) and intentions to seek information in the future (H5).
Effects of messages to increase perceived hazards
The RISP model includes the perceived hazard characteristics variable to capture individual evaluations of the nature of hazard (Griffin et al., Citation2013). Although perceived hazard is not included in Yang et al.’s (Citation2014) R-RISP model, and may be less influential than knowledge and subjective norms, they note that care must be taken to ensure the perceived relevance of and sensitivity to risks is taken into account when applying the model. Factors associated with increased perceptions of risk in the RISP model are also not well defined beyond the identification of individual characteristics (Griffin et al., Citation1999).
Greater perceived hazard should increase the likelihood of a negative affective response, which is associated with increased perceived information insufficiency and information seeking (Yang et al., Citation2014). According to the precaution adoption process model (PAPM: Weinstein & Sandman, Citation2002), awareness of risks (via messages or other means) motivates decisions to act. The protection action decision model (PADM) expands on this idea, suggesting that exposure to and comprehension of warning messages may increase threat perceptions (Lindell & Perry, Citation2012). Indeed, the extended parallel process model (Witte, Citation1992) and risk perception attitude framework (Rimal, Citation2001) suggest that perceived threat (i.e., perceived susceptibility and severity) acts as a triggering mechanism for message processing and behaviors to manage the threat. Prior work has indeed shown perceived susceptibility and negative affective risk response (i.e., fear and anxiety) positively predict information seeking time (So et al., Citation2019). PRISM studies also show risk perceptions to be associated with negative risk-related affect (Kahlor, Citation2010; Kahlor et al., Citation2018), which is associated with greater seeking intention (Hovick et al., Citation2014; Kahlor, Citation2010).
Based on these perspectives, we expect participants exposed to a high threat message (indicating potentially high susceptibility and severity) or low threat message (not indicating potentially high-risk susceptibility and severity) will report greater perceived hazard than those not exposed to a message (H6a). Furthermore, we expect those exposed to a high threat message will report greater perceived hazard than those exposed to a low threat message (H6b). In turn, we expect perceived hazard to be positively associated with negative affective response (H7). We also anticipate that exposure to a high or low threat message (versus no message) will be associated with increased perceptions of current knowledge regarding environmental health risks (H8). Exposure to risk communication is likely to have an impact on individual knowledge of environmental health risks (Fitzpatrick-Lewis et al., Citation2010; Hwang & Jeong, Citation2016), particularly when individuals perceive they are at risk. Finally, we propose that risk-related negative affect will be positively associated with current knowledge perceptions (H9), information seeking (H10) and future seeking intentions (H11).
Effects of cues to action
In addition to examining the effects of risk messages, we examine the addition of cues to seek information within the message. The “cues to action” concept is rooted in the health belief model (HBM), which proposes that these cues may directly influence perceptions of threat and indirectly influence health behaviors (Rosenstock, Citation1990). In the HBM, a message may even act as a cue to action in and of itself (Janz et al., Citation2002; Rosenstock, Citation1990). Seeking cues are commonly included in health and risk campaigns, including specific calls to seek e.g., “For free help, visit cdc.gov/tips” (Centers for Disease Control and Prevention, Citation2014) and/or the provision of a hashtag or website information. Thus, as illustrated in , we propose that exposure to cues to seek additional information will positively affect seeking behavior beyond mere message exposure (H12).
Seeking as a predictor of future seeking intentions
According to social cognitive theory (SCT; Bandura, Citation2001), past behavior is a powerful predictor of future behavior. Although this relationship was unsupported in the information seeking context (Hovick et al., Citation2014), measurements of past seeking behaviors were broad and included any past seeking recalled by participant (e.g., “I have sought information about my cancer risk in the past”; Hovick et al., Citation2014, p. 519). We addressed these measurement concerns by providing participants the opportunity to seek information and then assessed the effects of seeking on their future intentions to seek. We focus on information seeking, which is the most active and directed form of information acquisition (Bates, Citation2002). Based on SCT, we predict that those who seek information will report greater future seeking intentions (; H13). Examining this relationship will help illuminate whether seeking facilitates or deters future seeking. This information may be useful to health communicators responsible for dissemination of health information, particularly over time. If seekers report greater future seeking intentions than non-seekers, they may be more likely to engage with health messages and stay informed about health risks.
Methods
We conducted a 3 (risk topic: Arsenic, BPA, Volatile Organic Compounds [VOCs]) x 2 (message type: high or low threat) x 2 (seeking cue: present, absent) between-subjects online experiment with no message control conditions for each risk topic. Therefore, there were a total of 15 conditions with 12 messages tested and 3 no message controls.
Participant recruitment and study procedures
We recruited participants from an online panel coordinated by Qualtrics, Inc. between June and August 2018. To be eligible for the study, participants had to live in the U.S., be able to read and speak English and be age 18 to 65. Because the results of in-person lab versus online experiments are comparable (Finley & Penningroth, Citation2015), our study took place online. Participants over age 65 were not recruited because it is estimated that 33% of this population does not use the Internet (Anderson & Perrin, Citation2017) and thus may have felt less comfortable seeking information online. Panel participants earned redeemable points as compensation. Eligible participants were invited to participate via e-mail. Interested participants viewed an online consent statement and were directed to the online survey if they agreed to participate. Approximately 70% of those who began the survey completed it (798 did not); thus, our final sample size (N) was 1,823.
The experiment was conducted online using the Qualtrics survey interface. Participants first answered demographic, health history, and environment and health behavior questions. Next, participants responded to several items not used in this manuscript. Participants were then randomly assigned to one of three risks (BPA, Arsenic or VOCs) and one of three message conditions (high threat, low threat or no message). Participants in high and low threat message conditions also were randomly assigned to receive a seeking cue (or not).
All participants then responded to R-RISP model variables and were given an opportunity to seek information online by responding to the question: “Would you like to seek more information about [risk topic]?” Participants who selected “yes” were taken to a sample search page (created by the researchers) with clickable, live links. The search page linked to real websites hosted by credible health and news organizations that contained additional information on their topic. Participants who selected “no” to seeking were asked why they choose not to seek. Over half of participants (54%) choose to seek information during the study. Those who did not want to seek reported they did not have enough time to seek right now (46% of non-seekers), were already informed about the risk (23%), were not concerned about the risk (17%), did not feel like the risk or information was relevant to them (7%), or felt seeking was a waste of time (3%). Afterward, participants were asked about their intentions to seek information in the future.
Stimuli
We investigated differences across three common household environmental risks known to be associated with increased cancer risk: VOCs (gases used in household products that are released into the air from some solids and liquids; United States Environmental Protection Agency, Citation2017), bisphenol A (BPA; an industrial chemical used to make plastics and resins; Centers for Disease Control and Prevention, Citation2017b) and arsenic (a chemical from natural and human sources found in some foods; Centers for Disease Control and Prevention, Citation2017a).
The low threat message presented basic information about the risk and stated that most current levels of exposure were safe. The high threat message, in contrast, included severity and susceptibility information and used more negative emotion (e.g., dangerous, damage, kill), body and health words (e.g., eat, blood, cancer) and personal pronouns (i.e., “you”), which were intended to increase perceived hazard as compared to the low threat message.
Information for the 12 messages came from a variety of sources including the Centers for Disease Control and Prevention (Citation2017a, Citation2017b, Citation2017c), the U.S. Food and Drug Administration (Citation2017), National Institute of Environmental Health Sciences (Citation2017), the United States Environmental Protection Agency (Citation2017), The Dartmouth Toxic Metals Superfund Research Program (Citation2017), American Cancer Society (Citation2017), American Lung Association (Citation2017), Mayo Clinic (Bauer, Citation2017), and WebMD (Citation2017). These same sources also were included as links in the search pages for the information seeking opportunity. All 12 messages were of similar length; the largest difference between the longest and shortest message was 29 words. For an example of a high and low threat message, see .Footnote1
Measures
Means (M), standard deviations (SD), and correlations (r) between model variables are presented in . Items were assessed on a 1 (strongly disagree) to 5 (strongly agree) scale unless noted.Footnote2
Table 1. Descriptive Statistics and Pearson Correlations for Main Model Variables (N = 1,823).
Perceived hazard characteristics
Perceived hazard characteristics were measured using three items modified from prior research (Hovick et al., Citation2014) and one original item. Two items captured perceived severity (e.g., “If [risk topic] were to negatively impact your health, how serious would it be?”) and two items captured perceived susceptibility (e.g., “How likely is [risk topic] to negatively impact your health in your lifetime?”). For all four items, Cronbach’s α = .77.
Affective response
Hazard-related affective response (i.e., worried, scared, overwhelmed, concerned) was measured using four items based on Kahlor (Citation2010). Individuals were asked about the extent to which they felt these emotions when thinking about the risks associated with their topic (VOCs, BPA or Arsenic) on a scale of 1 (none of this feeling) to 5 (a lot of this feeling; Cronbach’s α = .88).
Current knowledge perceptions
As in previous studies (Griffin et al., Citation2002), participants were asked to assess their perceived knowledge of the risk. We used a scale of 1–10 (versus the typical 1–100 scale) to allow for better alignment with other item responses, anchored with 1 = “knowing nothing” and 10 = “knowing everything you could possibly know.”
Informational subjective norms
Subjective norms were assessed (based on Kahlor, Citation2010; Park & Smith, Citation2007) using three items (e.g., most people whose opinions I value would approve of my seeking information about [risk topic]). Assessments of informational subjective norms are predominately (e.g., Kahlor, Citation2010) or completely (e.g., Yang, Citation2012) comprised of injunctive norms items; thus, our measure reflects that practice (Cronbach’s α = .89).
Information seeking
As previously described, participants were given an opportunity to seek information during the study. In this analysis, we used responses to the question, “would you like to seek more information about [risk topic]?” as the measure of seeking (1 = “yes,” 0 = “no”). If a participant selected “yes,” they were taken to the sample search page (as previously described). Participants could search as long as they wanted before completing the survey. If a participant selected “no,” we assessed why and directed them to complete the survey. Our original intention was to capture time seeking and number of sites accessed, but we were unable to match individual seeking analytic data with survey data using IP address; thus, the binary seeking variable is used here.
Information seeking intentions
Future seeking intention was the main outcome variable. It was assessed with three items from Kahlor (Citation2010), including “I plan to seek more information about my risk of [risk topic] in the near future” (Cronbach’s α = .94).
Data analysis
Analyses were conducted in SPSS version 24.0 and Mplus version 8 statistical software packages. As in several previous RISP studies (e.g., Yang et al., Citation2011; Yang, Citation2012), structural equation modeling (SEM) was used. First, confirmatory factor analyses (CFAs) were conducted to confirm the dimensionality of each scale. Second, the full structural model was estimated in Mplus using maximum likelihood estimation with robust standard errors (MLR estimator; Muthén & Muthén, Citation2015). Perceived susceptibility, perceived severity, affective response, informational subjective norms and seeking intention were entered into the model as continuous latent variables, along with seeking cue (1 = yes, 0 = no), and message type (dummy coded with the control [no message] as the comparison condition). Based on Bollen and Long (Citation1993) and Holbert and Stephenson (Holbert & Stephenson, Citation2008), model fit indices included the Chi-Square Test of Model Fit (χ2), Root Mean Square Error of Approximation (RSMEA; cutoff values ≤0.08), Comparative Fit Index (CFI; with cutoff values ≥0.90) and the Standardized Root Mean Square Residual (SRMR cutoff values ≤0.09).
Results
Participant demographics
Of participants, over 80% (n = 1,475) were female and most were non-Hispanic White (n = 1,306; 71.6%). The mean age of participants was 38.75 years (SD = 10.13) and the majority lived in urban areas (n = 993, 54.5%). Over 60% of participants had attended at least some college (n = 1,249), but 38.6% (n = 688) had household incomes of 35,000 USD per year or less. Chronic disease was relatively common, with 41.2% (n = 992) reporting that they or someone in their household lived with asthma, heart disease, COPD, or cancer of any type. Additionally, 60% (n = 1,094) had children or were pregnant, making them potentially more vulnerable to environmental health risks (e.g., arsenic; American Cancer Society, Citation2017). However, perceptions of current knowledge of risk topics, even following exposure to risk messages, were low (arsenic M = 4.39, SD = 2.62; BPA M = 4.62, SD = 2.73; VOC M = 3.68, SD = 3.00; range = 1–10).
Confirmatory factor analysis
We tested three measurement models to confirm the dimensionality of latent variables (i.e., perceived hazard, affective response, informational subjective norms and seeking intention) for each risk topic. All three models demonstrated acceptable fit. For arsenic, χ2(71, n = 603) = 321.70, p < .001, RMSEA = .08 (90% CI = .07 to .09), CFI = .93, SRMR = .06. For BPA, χ2(71, n = 621) = 280.18, p < .001, RMSEA = .07 (90% CI = .06 to .08), CFI = .94, SRMR = .05. For VOC, χ2(71, n = 596) = 277.39, p < .001, RMSEA = .07 (90% CI = .06 to .08), CFI = .94, SRMR = .05. Standardized factor loadings across models ranged from .43 to .94, providing further construct validity.
The acceptable model fit across the three measurement models demonstrated measurement invariance, or evidence the scales assess “the same underlying construct” (Bialosiewicz et al., Citation2013, p. 3) across risk topics. Therefore, rather than testing a separate SEM for each risk, one SEM was tested that also controlled for risk topic (dummy coded with BPA as the comparison condition). BPA served as the comparison because it has received considerable media coverage (Kiss, Citation2013; Mello, Citation2015).
Main model test
The proposed model fits the data moderately well, χ2(161) = 1402.53, p < .001, RMSEA = 0.07 (90% CI = .06 to .07), CFI = 0.90, SRMR = 0.12.Footnote3 Standardized parameter estimates for model paths are presented in . Looking first at risk topic differences, perceived hazard was significantly lower, but affective risk response was significantly higher, among those exposed to arsenic versus BPA messages.Footnote4 Additionally, those exposed to BPA messages reported significantly greater current knowledge perceptions than those exposed to VOC messages but were less likely to seek information. No other risk topic differences were detected.
Table 2. Standardized Results and Significance Levels for Structural Model Paths (N = 1,823).
Overall, our results support the proposed model; all but one hypothesis was supported and one received partial support (see ). Although participants in the high threat condition reported greater perceived hazard than those in the control conditions, as expected, no differences were detected between the low threat and control conditions (H6a partially supported). Independent samples t-tests further showed that perceived risk was significantly lower in the low threat (M = 3.55, SD = 0.85) than in the high threat (M = 3.77, SD = 0.88) message condition, t(1363) = −4.69, p < .001, 95% CI = −0.31 to −0.13 (H6b supported). Results also showed exposure to a seeking cue did not increase actual seeking (H12 not supported). The model explained a moderate amount of variance in seeking behavior (R2 = 0.15, SE = 0.01, p < .001) and larger amount in seeking intention (R2 = 0.41, SE = 0.02, p < .001).
Table 3. Overview of Study Predictions and Outcomes.
Discussion
We conducted an online experiment to examine whether exposure to environmental risk messages, along with R-RISP model factors, would impact information seeking behaviors and intentions. Specifically, we compared high threat risk messages, low threat risk messages and no message control conditions to explore these relationships. Our results demonstrate it is possible to prompt information seeking by exposing individuals to high or low threat environmental risk messages. Our results also show that R-RISP model factors are central in the information seeking decision-making process; perceived hazard, affective risk response, perceived norms and current knowledge perceptions predicted actual seeking and future intentions to seek.
Seeking as a response to messages
Our study is among only a handful to experimentally test information behaviors in response to risk message exposure (Hwang & Jeong, Citation2016; So et al., Citation2019). As predicted, exposure to a high threat message (but not a low threat message) was positively associated with perceptions of hazard, which were associated with greater negative affect. Furthermore, similar to the driving mechanism outlined in the EPPM (Witte, Citation1992), exposure to high threat risk messages increased hazard perceptions and negative affective responses to risks, which ultimately increased information behaviors and intentions.
We also tested the potential for high and low threat messages to influence current knowledge perceptions, and trigger information acquisition behaviors in turn. Our results showed both risk message types increased assessments of current knowledge perceptions, which increased information seeking behavior and future intentions to seek. This finding is likely the result of simple exposure and is not surprising, given that we designed both messages to provide information about the risks. Thus, people who read a message had an opportunity for learning via the information provided in the message; people in the control condition did not. This result compliments prior research that found any exposure to risk information increased knowledge (e.g., Fitzpatrick-Lewis et al., Citation2010). Importantly, our results suggest high or low-risk environmental health messages could drive information seeking.
Practically, these findings suggest environmental health messages need not be threatening to have an effect on information seeking behaviors and intentions. Within the literature on health message effects, scholars have debated the need for and ethics of using threat, risk and/or negative affect as a way to encourage behavior change (e.g., Hastings et al., Citation2004; Ruiter et al., Citation2014; Witte & Allen, Citation2000). In the case of the present study, participants were provided links to credible information about these topics. However, in less controlled settings, providing risk information (even low threat information) without additional guidance on where to seek could result in individuals accessing low credibility sources and misinformation that may affect individual beliefs, attitudes and behaviors (Southwell & Thorson, Citation2015). Thus, it may be important for health communicators to link to credible information when encouraging information acquisition, as well as build media literacy interventions for the general public to help them better detect misinformation (Lee, Citation2018).
Interestingly, the inclusion of a seeking cue (i.e., seek more information today) did not increase the likelihood of information seeking in this study. We varied the presence of this type of cue to action to mimic a common strategy, rooted in the HBM (Rosenstock, Citation1990). Although the goal of health campaigns is often to change behavior, traditional (e.g., billboards, television spots) and online (e.g., social media) messages are constrained by time or space within each medium. Because campaign messages may not be able to provide all of the information individuals need to successfully change a behavior, campaigners often include cues to seek or provide a secondary place (e.g., telephone number, website) where the public can get additional information on the topic. Although exposure to hazard information appears to influence information acquisition behaviors, our results suggest a seeking cue is unlikely to add an additional trigger. However, we tested only one type of seeking cue across conditions that did not reference a source of information from which to seek. Further studies should investigate how the type, length and framing of seeking cues impacts seeking decision-making.
Support for the R-RISP model
Our results largely support R-RISP model predictions. Individuals who perceived greater subjective norms were more likely to seek information and had greater future seeking intentions. Thus, our perceptions of others’ behaviors and expectations continue to be powerful predictors of seeking. Health communicators who desire to influence information seeking should focus on shaping perceived norms. Messages communicating that close others, or people who also care about environmental health risks, expect us to seek information to stay informed, may be particularly helpful for encouraging information seeking.
Current knowledge perceptions were also associated with actual and intended seeking. Those who perceived themselves as having more knowledge were more likely to seek and reported greater future seeking intentions. Those who sought information also reported greater seeking intentions than those who did not. Our findings support studies showing a relationship between knowledge and seeking intentions (e.g., Griffin et al., Citation2008; Yang et al., Citation2010b; Ter Huurne et al., Citation2009) and seem to suggest that increasing knowledge perceptions, which may increase awareness of knowledge gaps, may increase the likelihood of subsequent seeking.
Given the ease at which information can be accessed online, scholars should explore how and why seeking influences future information acquisition behaviors, including the conditions under which knowledge (perceived or actual) increases or decreases information needs. The positive link between seeking and future seeking intentions here suggests that health messages designed to promote information acquisition, particularly in limited information or high uncertainty environments, may have more impact on those who have previously engaged in information seeking and perceive themselves to be knowledgeable. Health communicators must therefore make efforts to reach out to those with limited information access, as well as those who may be less likely to seek.
Limitations
Due to the fact that we sampled using an online panel, we may have had a selection bias toward individuals who are skilled at seeking information online. Future research should explore other channels utilized in information acquisition (e.g., family and friends, medical professionals). Additionally, we did not quantify the extent to which individuals sought information. Future research should continue to examine information seeking in real-time, which may require more lab-based studies.
Conclusions
Overall, our findings provide additional support for theories of information seeking, including a reduced RISP model. Our results show it is possible to trigger health information seeking by exposing individuals to high or low threat messages. Message exposure increased perceptions of current knowledge, which increased seeking and future seeking intentions. Exposure to a high threat message (versus a low threat or no message) also increased seeking behavior and future seeking intentions by increasing perceived hazard. Our results also provide further evidence of the important role subjective norms and perceived knowledge play in information acquisition.
Notes
1. All 12 messages are available at the second author’s personal website or the following Open Science Foundation (OSF) link: https://osf.io/c5bu3/?view_only=36e5ff4a093d4da082c1a5029ff60a51
2. A complete list of measures is available at the second author’s personal website or the following OSF link: https://osf.io/c5bu3/?view_only=36e5ff4a093d4da082c1a5029ff60a51
3. For a good model fit, the χ2 test should not be statistically significant; however, χ2 is sensitive to sample size and models with a large number of cases (> 400) often have statistically significant values, which is why we also considered the SRMR (Kenny, Citation2015; Schermelleh-Engel et al., Citation2003).
4. Coefficients for dummy coded variables are interpreted the same way they would in linear regression. For example, in , those in the arsenic condition reported.10 points lower on the perceived hazard scale than those in the BPA condition.
References
- Ajzen, I. (1991). The theory of planned behaviour. Organizational Behavior and Human Decision Processes, 50(2), 179–211. https://doi.org/10.1016/0749-5978(91)90020-T
- Alba, J. W., & Hutchinson, J. W. (1987). Dimensions of consumer expertise. Journal of Consumer Research, 13(4), 411–454. https://doi.org/10.1086/209080
- American Cancer Society. (2017). Arsenic and cancer risk. https://www.cancer.org/cancer/cancer-causes/arsenic.html.
- American Lung Association. (2017). Volatile organic compounds. https://www.lung.org/our-initiatives/healthy-air/indoor/indoor-air-pollutants/volatile-organic-compounds.html.
- Anderson, M., & Perrin, A. (2017). Technology use among seniors. Pew Research Center. https://www.pewresearch.org/internet/2017/05/17/technology-use-among-seniors/
- Bandura, A. (2001). Social cognitive theory: An agentic perspective. Annual Review of Psychology, 52(1), 1–26. https://doi.org/10.1146/annurev.psych.52.1.1
- Bates, M. J. (2002). Toward an integrated model of information seeking and searching. The New Review of Information Behaviour Research, 3(1), 1–15.
- Bauer, B. A. (2017). What is bpa and what are the concerns about bpa? Mayo Clinic. https://www.mayoclinic.org/healthy-lifestyle/nutrition-and-healthy-eating/expert-answers/bpa/faq-20058331
- Bialosiewicz, S., Murphy, K., & Berry, T. (2013). An introduction to measurement invariance testing: Resource packet for participants [Paper presentation]. The American Evaluation Association, Washington DC. http://comm.eval.org/HigherLogic/System/DownloadDocumentFile.ashx?DocumentFileKey=63758fed-a490-43f2-8862-2de0217a08b8.
- Bigsby, E., & Hovick, S. R. (2018). Understanding associations between information seeking and scanning and health risk behaviors: An early test of the structural influence model. Health Communication, 33(3), 315–325. https://doi.org/10.1080/10410236.2016.1266575
- Bollen, K. A., & Long, J. S. (1993). Testing structural equation model (Vol. 154). Sage.
- Braun, J., & Niederdeppe, J. (2012). Disruption and identity maintenance in risk information seeking and processing. Communication Theory, 22(2), 138–162. https://doi.org/10.1111/j.1468-2885.2012.01402.x
- Centers for Disease Control and Prevention. (2017a). National biomonitoring program: Arsenic factsheet. https://www.cdc.gov/biomonitoring/Arsenic_FactSheet.html.
- Centers for Disease Control and Prevention. (2017b). National biomonitoring program: Bispenol a (bpa) factsheet. https://www.cdc.gov/biomonitoring/BisphenolA_FactSheet.html.
- Centers for Disease Control and Prevention. (2017c). Toxic substances portal – Arsenic: Toxfaqs for arsenic. https://www.atsdr.cdc.gov/toxfaqs/tf.asp?id=19 &tid=3.
- Centers for Disease Control and Prevention. (2014, June 24). CDC: Tips from former smokers - Amanda B.'s tip ad [Video]. YouTube. https://www.youtube.com/watch?v=qi_JwXTXLA0&feature=youtu.be
- Chaiken, S., Liberman, A., & Eagly, A. H. (1989). Heuristic and systematic information processing within and beyond the persuasion context. In J. S. Uleman & J. A. Bargh (Eds.), Unintended thought. Guilford Press.
- Eagly, A. H., & Chaiken, S. (1993). The psychology of attitudes: Harcourt Brace Jovanovich College Publishers.
- Finley, A. J., & Penningroth, S. L. (2015). Online versus in-lab: pros and cons of an online prospective memory experiment. In A. M. Columbus (Ed.), Advances in Psychology Research, (Vol. 113, pp. 135-162). Nova Science Publishers, Inc.
- Fitzpatrick-Lewis, D., Yost, J., Ciliska, D., & Krishnaratne, S. (2010). Communication about environmental health risks: A systematic review. Environmental Health, 9(1), 67. https://doi.org/10.1186/1476-069X-9-67
- Griffin, R. J., Dunwoody, S., & Neuwirth, K. (1999). Proposed model of the relationship of risk information seeking and processing to the development of preventive behaviors. Environmental Research, 80(2), S230–245. https://doi.org/10.1006/enrs.1998.3940
- Griffin, R. J., Dunwoody, S., & Yang, Z. J. (2013). Linking risk messages to information seeking and processing. Annals of the International Communication Association, 36(1), 323–362. https://doi.org/10.1080/23808985.2013.11679138
- Griffin, R. J., Neuwirth, K., Giese, J., & Dunwoody, S. (2002). Linking the heuristic-systematic model and depth of processing. Communication Research, 29(6), 705–732. https://doi.org/10.1177/009365002237833
- Griffin, R. J., Yang, Z. J., Ter Huurne, E., Boerner, F., Ortiz, S., & Dunwoody, S. (2008). After the flood: Anger, attribution, and the seeking of information. Science Communication, 29(3), 285–315. https://doi.org/10.1177/1075547007312309
- Hastings, G., Stead, M., & Webb, J. (2004). Fear appeals in social marketing: Strategic and ethical reasons for concern. Psychology & Marketing, 21(11), 961–986. https://doi.org/10.1002/mar.20043
- Holbert, R. L., & Stephenson, M. T. (2008). Commentary on the uses and misuses of structural equation modeling in communication research. In A. F. Hayes, M. D. Slater, & L. B. Snyder (Eds.), The Sage sourcebook of advanced data analysis methods for communication research (pp. 185–218). Sage.
- Hovick, S. R., Kahlor, L., & Liang, M. C. (2014). Cancer knowledge and information seeking through prism: The planned risk information seeking model. Journal of Health Communication, 19(4), 511–527. https://doi.org/10.1080/10810730.2013.821556
- Hwang, Y., & Jeong, S.-H. (2016). Information insufficiency and information seeking: An experiment. Science Communication, 38(6), 679–698. https://doi.org/10.1177/1075547016673200
- Janz, N. K., Champion, V. L., & Strecher, V. J. (2002). The health belief model. In K. Glanz, B. K. Rimer, & F. M. Lewis (Eds.), Health behavior and health education: Theory, research, and practice (pp. 45–66). Jossey-Bass.
- Kahlor, L. (2007). An augmented risk information seeking model: The case of global warming. Media Psychology, 10(3), 414–435. https://doi.org/10.1080/15213260701532971
- Kahlor, L. (2010). Prism: A planned risk information seeking model. Health Communication, 25(4), 345–356. https://doi.org/10.1080/10410231003775172
- Kahlor, L., Dunwoody, S., & Griffin, R. J. (2004). Predicting knowledge complexity in the wake of an environmental risk. Science Communication, 26(1), 5–30. https://doi.org/10.1177/1075547004267231
- Kahlor, L., Dunwoody, S., Griffin, R. J., Neuwirth, K., & Giese, J. (2003). Studying heuristic-systematic processing of risk communication. Risk Analysis, 23(2), 355–368. https://doi.org/10.1111/1539-6924.00314
- Kahlor, L., Yang, Z. J., & Liang, M. (2018). Risky politics: Applying the planned risk information seeking model to the 2016 U.S. Presidential election. Mass Communication and Society, 21(6), 697–719. https://doi.org/10.1080/15205436.2018.1498900
- Kenny, D. A. (2015). Measuring model fit. http://davidakenny.net/cm/fit.htm
- Kerstetter, D., & Cho, M.-H. (2004). Prior knowledge, credibility and information search. Annals of Tourism Research, 31(4), 961–985. https://doi.org/10.1016/j.annals.2004.04.002
- Khosrowjerdi, M., & Iranshahi, M. (2011). Prior knowledge and information-seeking behavior of PhD and MA students. Library & Information Science Research, 33(4), 331–335. https://doi.org/10.1016/j.lisr.2010.04.008
- Kiss, S. (2013). Legislation by agenda-setting: Assessing the media’s role in the regulation of bisphenol a in the United States. Mass Communication and Society, 16(5), 687–712. https://doi.org/10.1080/15205436.2013.768345
- Kontos, E. Z., Bennett, G. G., & Viswanath, K. (2007). Barriers and facilitators to home computer and internet use among urban novice computer users of low socioeconomic position. Journal of Medical Internet Research, 9(4), e31–e31. https://doi.org/10.2196/jmir.9.4.e31
- Lee, N. M. (2018). Fake news, phishing, and fraud: A call for research on digital media literacy education beyond the classroom. Communication Education, 67(4), 460–466. https://doi.org/10.1080/03634523.2018.1503313
- Lindell, M. K., & Perry, R. W. (2012). The protective action decision model: Theoretical modifications and additional evidence. Risk Analysis, 32(4), 616–632. https://doi.org/10.1111/j.1539-6924.2011.01647.x
- May, A. (2018). Parsimony. In M. A. Allen (Ed.), The Sage encyclopedia of communication research methods (pp. 1181–1184). Sage.
- Mello, S. (2015). Media coverage of toxic risks: A content analysis of pediatric environmental health information available to new and expecting mothers. Health Communication, 30(12), 1245–1255. https://doi.org/10.1080/10410236.2014.930398
- Muthén, L., & Muthén, B. (2015). Mplus user’s guide: Seventh edition. Muthén & Muthén.
- Myrick, J. G. (2017). The role of emotions and social cognitive variables in online health information seeking processes and effects. Computers in Human Behavior, 68, 422–433. https://doi.org/10.1016/j.chb.2016.11.071
- National Institute of Environmental Health Sciences. (2017). Bisphenol a (bpa). https://www.niehs.nih.gov/health/topics/agents/sya-bpa/index.cfm
- Park, H. S., & Smith, S. W. (2007). Distinctiveness and influence of subjective norms, personal descriptive and injunctive norms, and societal descriptive norms on behavioral intent: A case of two behaviors critical to organ donation. Human Communication Research, 33(2), 194–218. https://doi.org/10.1111/j.1468-2958.2007.00296.x
- Rimal, R. N. (2001). Perceived risk and self‐efficacy as motivators: Understanding individuals’ long‐term use of health information. Journal of Communication, 51(4), 633–654. https://doi.org/10.1111/j.1460-2466.2001.tb02900.x
- Rosenstock, I. M. (1990). The health belief model: Explaining health behavior through expectancies. In K. F. Glanz, M. Lewis, & B. Rimer (Eds.), Health behavior and health education (Vol. 2, pp. 328–335). Jossey-Bass Publishers.
- Ruiter, R. A., Kessels, L. T., Peters, G. J. Y., & Kok, G. (2014). Sixty years of fear appeal research: Current state of the evidence. International Journal of Psychology, 49(2), 63–70. https://doi.org/10.1002/ijop.12042
- Schermelleh-Engel, K., Moosbrugger, H., & Müller, H. (2003). Evaluating the fit of structural equation models: Tests of significance and descriptive goodness-of-fit measures. Methods of Psychological Research Online, 8(2), 23–74.
- Sheeran, P., Maki, A., Montanaro, E., Avishai-Yitshak, A., Bryan, A., Klein, W. M. P., Miles, E. & Rothman, A. J. (2016). The impact of changing attitudes, norms, and self-efficacy on health-related intentions and behavior: A meta-analysis. Health Psychology, 35(11), 1178–1188. https://doi.org/10.1037/hea0000387
- Shim, M., Kelly, B., & Hornik, R. (2006). Cancer information scanning and seeking behavior is associated with knowledge, lifestyle choices, and screening. Journal of Health Communication, 11(Suppl 1), 157–172. https://doi.org/10.1080/10810730600637475
- So, J., Kuang, K., & Cho, H. (2019). Information seeking upon exposure to risk messages: Predictors, outcomes, and mediating roles of health information seeking. Communication Research, 46(5), 663–687. https://doi.org/10.1177/0093650216679536
- Southwell, B. G., & Thorson, E. A. (2015). The prevalence, consequence, and remedy of misinformation in mass media systems. Journal of Communication, 65(4), 589–595. https://doi.org/10.1111/jcom.12168
- Ter Huurne, E. F., Griffin, R. J., & Gutteling, J. M. (2009). Risk information seeking among US and Dutch residents: An application of the model of risk information seeking and processing. Science Communication, 31(2), 215–237. https://doi.org/10.1177/1075547009332653
- The Dartmouth Toxic Metals Superfund Research Program. (2017). Welcome to arsenic and you. Trustees of Dartmouth College. http://www.dartmouth.edu/~arsenicandyou/
- Trumbo, C. W., & O’Keefe, G. J. (2001). Intention to conserve water: Environmental values, planned behavior, and information effects. A comparison of three communities sharing a watershed. Society & Natural Resources, 14(10), 889–899. https://doi.org/10.1080/089419201753242797
- Trumbo, C. W., & O’Keefe, G. J. (2005). Intention to conserve water: Environmental values, reasoned action, and information effects across time. Society & Natural Resources, 18(6), 573–585. https://doi.org/10.1080/08941920590948002
- U.S. Food and Drug Administration. (2017). Arsenic in food and dietary supplements. https://www.fda.gov/food/metals/arsenic-food-and-dietary-supplements.
- United States Environmental Protection Agency. (2017). Volatile organic compounds’ impact on indoor air quality. https://www.epa.gov/indoor-air-quality-iaq/volatile-organic-compounds-impact-indoor-air-quality.
- WebMD. (2017). The facts about bisphenol a. https://www.webmd.com/children/bpa#1.
- Weinstein, N. D., & Sandman, P. M. (2002). The precaution adoption process model and its application. In R. J. DiClemente, R. A. Crosby, & M. C. Kegler (Eds.), Emerging theories in health promotion practice and research (pp. 16–39). Jossey-Bass.
- Willoughby, J. F., & Myrick, J. G. (2016). Does context matter? Examining PRISM as a guiding framework for context-specific health risk information seeking among young adults. Journal of Health Communication, 21(6), 696–704. https://doi.org/10.1080/10810730.2016.1153764
- Witte, K. (1992). Putting the fear back into fear appeals: The extended parallel process model. Communication Monographs, 59(4), 401–421. https://doi.org/10.1080/03637759209376276
- Witte, K., & Allen, M. (2000). A meta-analysis of fear appeals: Implications for effective public health campaigns. Health Education & Behavior, 27(5), 591–615. https://doi.org/10.1177/109019810002700506
- Yang, Z. J. (2012). Too scared or too capable? Why do college students stay away from the H1N1 vaccine? Risk Analysis, 32(10), 1703–1716. https://doi.org/10.1111/j.1539-6924.2012.01799.x
- Yang, Z. J., Aloe, A. M., & Feeley, T. H. (2014). Risk information seeking and processing model: A meta-analysis. Journal of Communication, 64(1), 20–41. https://doi.org/10.1111/jcom.12071
- Yang, Z. J., McComas, K., Gay, G., Leonard, J. P., Dannenberg, A. J., & Dillon, H. (2010a). From information processing to behavioral intentions: Exploring cancer patients’ motivations for clinical trial enrollment. Patient Education and Counseling, 79(2), 231–238. https://doi.org/10.1016/j.pec.2009.08.010
- Yang, Z. J., McComas, K., Gay, G., Leonard, J. P., Dannenberg, A. J., & Dillon, H. (2010b). Motivation for health information seeking and processing about clinical trial enrollment. Health Communication, 25(5), 423–436. https://doi.org/10.1080/10410236.2010.483338
- Yang, Z. J., McComas, K. A., Gay, G., Leonard, J. P., Dannenberg, A. J., & Dillon, H. (2011). Information seeking related to clinical trial enrollment. Communication Research, 38(6), 856–882. https://doi.org/10.1177/0093650210380411