ABSTRACT
Internet searches for health-related purposes are common, with search engines like Google being the most popular starting point. However, results on the popularity of health information-seeking behaviors are based on self-report data, often criticized for suffering from incomplete recall, overreporting, and low reliability. Therefore, the current study builds on user-centric tracking of Internet use to reveal how individuals actually behave online. We conducted a secondary analysis of passively recorded Internet use logs to examine the prevalence of health-related search engine use, the types of health information searched for, and the sources visited after the searches. The analysis revealed two key findings. 1) We largely support earlier survey-based findings on the prevalence of online health information seeking with search engines and the relatively minor differences in information-seeking behaviors between socio-demographic groups. 2) We provide a more granular picture of the process of HISB using search engines by identifying different selection patterns depending on the scope of the searches.
Introduction
Searching the Internet for health information is a common practice. For example, more than 72% of Germans searched online for health-related purposes in 2019, the year of our study (Link & Baumann, Citation2020). Since then, the share of online health information seekers has been steadily increasing; however, the COVID-19 pandemic has not led to the expected boom in digitalization in Germany (Link, Czerwinski, et al., Citation2022). If we look at how most individuals get health-related online information, search engines are the primary access point (Brkic et al., Citation2021; Hassan & Masoud, Citation2021; Macias et al., Citation2018; Ofran et al., Citation2012) and an essential asset in locating health information (Cartright et al., Citation2011). By offering a rapid, easily accessible, low-cost opportunity to get individually tailored information on health conditions and preventive measures (Choudhury et al., Citation2014; Hassan & Masoud, Citation2021; Macias et al., Citation2018), search engines aid users in making informed health decisions (Ayers & Kronenfeld, Citation2007), improving their self-care skills, and coping with uncertainties (Lambert & Loiselle, Citation2007).
Understanding how individuals acquire online health information is a scientifically and practically relevant endeavor for various reasons: First, insights into online health information-seeking behaviors (HISB) are foundational to assessing the extent to which the Internet fulfills its ascribed functions as a means for health-related coping, attitude formation, and decision-making (Choudhury et al., Citation2014; Powell et al., Citation2011; Quinn et al., Citation2017) and in reducing gaps in health knowledge (Bach & Wenz, Citation2020). Second, search queries are patient-generated information on public attention (Phillips et al., Citation2018; Qin & Peng, Citation2016). Since they mirror health-related needs, interests, and concerns (Agarwal et al., Citation2016; Choudhury et al., Citation2014), they can be used to tailor health communication efforts (Ofran et al., Citation2012). Third, in the tradition of infodemiology, search engine queries yield population health indicators (Eysenbach, Citation2009; Hochberg et al., Citation2020; Lee et al., Citation2023) and are used for discovering and addressing adverse events and behaviors (Agarwal et al., Citation2016). For example, influenza and dengue fever have been tracked by the frequency of search queries for their symptoms (e.g., Chan et al., Citation2011; Ginsberg et al., Citation2009).
While an accurate description of health-related search engine use and its users is necessary for such endeavors, the process from information needs to search behavior and source selection is seldom examined in more depth. Although information needs, types of health information sought, and sources selected are vital components of HISB (Zare-Farashbandi & Lalazaryan, Citation2014), they are seldom examined in a theory-driven manner. The widely used information-seeking models, such as the Comprehensive Model of Information Seeking (Johnson & Meischke, Citation1993) or the Risk Information Seeking and Processing Model and its iterations (Griffin et al., Citation1999; Kahlor, Citation2010; Yang et al., Citation2014), focus only on the antecedents of seeking intentions or behaviors instead of the process of HISB (see also Ou & Ho, Citation2022; X. Wang et al., Citation2021).
In addition, most empirical research on online HISB relies on survey self-reports. False recall and self-presentation can result in unreliable and inaccurate self-reports of media and Internet use (Prior, Citation2009; Scharkow, Citation2016, Citation2019). Direct and unobtrusive observation of online HISB promises to overcome such limitations. By collecting every query searched for and every link followed, user-centric tracking of Internet use reveals how individuals behave online (Christner et al., Citation2022; Freelon, Citation2014; Wieland et al., Citation2018), promising more extensive and fine-grained information on individuals’ online HISBs than survey data. In contrast to fields like political communication (e.g., Dvir-Gvirsman et al., Citation2016; Stier et al., Citation2020), health communication still needs to integrate this new data source. The few studies that investigated online HISB with user-centric tracking data were limited by small numbers of participants (e.g., Quinn et al., Citation2017), narrow measurements of HISB (Bach & Wenz, Citation2020), or a narrow topical focus (e.g., on vaccine-related information, Guess et al., Citation2020). Aggregate Google Trend data were more commonly used, for example, for public health monitoring and to investigate search engine use for specific topics such as breast cancer, pregnancy and childbirth, influenza, COVID-19, or vaccines (e.g., Asseo et al., Citation2020; Brkic et al., Citation2021; Cervellin et al., Citation2017; Ginsberg et al., Citation2009; Jun et al., Citation2018; see Mavragani & Ochoa, Citation2019, for an overview). However, these studies have limited informative value regarding individual online HISBs of typical health information seekers because the aggregate trends hide individuals and are driven mainly by extreme users.
Against this background, we aim to deepen the understanding of who searches for health information online and shed light on what individuals actually do. Using user-centric tracking data over three months in 2019 from 994 German Internet users, we study individuals’ health-related search engine use, how common sear ches are, what individuals search for, and which sources they select. We examine the prevalence and frequency of individuals’ health-related searches, the types of health information sought, and the sources visited after the searches.
The role of search engines for online HISB
HISBs are purposeful activities to obtain health information (Lambert & Loiselle, Citation2007; Niederdeppe et al., Citation2007; Zimmerman & Shaw, Citation2020). It is helpful to distinguish interest- and problem-driven HISB. Interest-driven HISB is based on a general interest in health-related topics and preventive efforts. In contrast, problem-driven HISB is triggered by a specific health-related burden (van der Rijt, Citation2000) or health-related uncertainties (Brashers, Citation2001). The Internet is one of the most popular channels for health information seeking (Link, Baumann, & Klimmt, Citation2021, Link, Baumann, & Linn Citation2021; X. Wang et al., Citation2021; Zimmerman & Shaw, Citation2020). Survey data suggest that at least 3 out of 4 individuals acquire information for health-related purposes online in Western countries (Bachl, Citation2016; Finney Rutten et al., Citation2019; Link, Baumann, & Klimmt, Citation2021, Link, Baumann, & Linn, Citation2021). The Internet enables users to find, acquire, and discuss information on various health issues offered by various sources (e.g., Cline & Haynes, Citation2001; Hartoonian et al., Citation2014; Kreps, Citation2017).
According to survey research, search engines are the most common tool for finding and navigating health-related information online (Brkic et al., Citation2021; Hassan & Masoud, Citation2021; Jadhav et al., Citation2014; Macias et al., Citation2018). As such, their use requires scholarly attention in its own right. Moreover, examining search engine use provides a dynamic and holistic view of online HISB. The data identifies how information needs are transformed into actual seeking behaviors, covering the magnitude of sources individuals can choose from (Chi et al., Citation2020; Pang et al., Citation2014). Bach and Wenz (Citation2020) investigated online HISB with user-centric tracking data of Internet use over four months. They found that only 46.1% of German search engine users searched for health-related information at least once. However, their identification of health-related searches relied on a restrictive operationalization, primarily including medical terms related to illness and diseases, which might explain the relatively low prevalence estimate compared to survey results. Our study, in contrast, builds on the WHO’s (Citation1946) integrative understanding of health as “a state of complete physical, mental, and social well-being and not merely the absence of disease and infirmity” when detecting health-related searches. Given this definition, we ask the following research question:
RQ1:
How common is search engine use for health-related information seeking?
The prevalence of health-related search engine use is one important matter. How the phenomenon is distributed across different strata is another. Although primarily based on surveys, previous research has identified relatively nuanced yet consistent differences. Women, younger individuals, and individuals with higher socio-economic status were more likely to engage in online HISB (Alvarez-Galvez et al., Citation2020; Bach & Wenz, Citation2020; Bachl, Citation2016; Baumann et al., Citation2017; Jung, Citation2015; Link, Baumann, Linn, et al., Citation2021; Link, Baumann, et al., Citation2022; X. Wang et al., Citation2021; Zimmerman & Shaw, Citation2020). In this light, we derived the following hypotheses:
H1a-c:
Search engine use for health-related information seeking is more frequent (a) among women than men, (b) individuals of younger age compared to higher age, and (c) individuals possessing higher levels of education compared to lower levels of education.
The process of online health information seeking
HISB is a multi-stage process. Based on the model for the selection of online health information (Sbaffi & Zhao, Citation2020; Zhang, Citation2013; Zhang et al., Citation2017), the process can be separated into four components: establishing an information need; identifying and accessing information sources; examining and evaluating information; and interpreting information. The current study will focus on information needs and source selection, considering that different information needs lead to differences in which types of information are sought and which kinds of sources are selected (Galarce et al., Citation2011; Zhang, Citation2013).
Information needs arise when individuals realize that their existing knowledge is inadequate to satisfy their goals (Case, Citation2002) or they feel uncertain as a situation is perceived as ambiguous, complex, and unpredictable (Brashers, Citation2001). Online HISB based on problem- or interest-driven information needs often starts with visiting a search engine and translating the information need into a search query (Jadhav et al., Citation2014). The information needs that lead to using a search engine can be related to a wide variety of questions – from information on diseases, symptoms, medical procedures, and treatments to questions regarding public health, health systems, unhealthy and healthy lifestyles, prevention, and well-being (Cao et al., Citation2016; Goldner, Citation2006). Survey research indicates that the Internet is used for HISB regarding various issues (Link, Baumann, Linn, et al., Citation2021; Powell et al., Citation2011; Zschorlich et al., Citation2015). For example, Link and colleagues (Citation2021) found that the most frequently searched-for issues were as diverse as symptoms and causes of diseases on the one hand and questions about a healthy lifestyle, fitness, and wellness on the other hand.
Few studies specifically examined health-related queries to search engines beyond specific diseases. Cartright et al. (Citation2011) found that queries referring to symptoms of diseases were the most common. In contrast, Palotti et al. (Citation2016) indicated that search queries foremost revolved around diseases rather than symptoms. However, other research found symptoms, causes, treatments, and drugs to be the most common categories of search queries (Jadhav et al., Citation2014). Again, the sparse literature using behavioral data is limited by its narrow focus on diseases. We aim to examine the types of information searched for against a broader understanding of health and concerning differences based on the socio-demographic characteristics of the information seekers:
RQ2a:
How common is it to seek different types of health-related information with search engines?
RQ2b:
How does search engine use for seeking different types of health-related information differ by gender, age, and education?
After submitting a query to a search engine, the next step is to select and visit one (or more) search results from various sources to satisfy the information needs (Jadhav et al., Citation2014). Although source selection is a defining component of HISB (e.g., Johnson & Meischke, Citation1993), source-selection behaviors are seldom deconstructed by identifying which need is followed by which source-visiting behaviors (Chi et al., Citation2020). The current study covers all online sources selected by the participants to provide a holistic view of online HISB (Chi et al., Citation2020). Online sources for health-related information are highly diverse and can be classified in different ways (Fox & Duggan, Citation2013; Link, Baumann, Linn, et al., Citation2021; Zhang et al., Citation2017). One differentiation is between sources of health content and sources of health community (Gitlow, Citation2000; Rossmann & Karnowski, Citation2014). Sources of health content vary in domain focus (i.e., specialized websites for health information or general sources that cover health information, among other kinds). They are provided by different actors and entities, such as media publishers, NGOs, governmental authorities, insurance companies, and health professionals. Sources of health community include online social networks, health communities, and question-and-answer services.
Survey research into online HISB indicates that, in general, health websites, online encyclopedias, online pharmacies, and websites of health professionals are the most popular types of sources (Link, Baumann, Linn, et al., Citation2021; see also Beck et al., Citation2014; Ratcliff et al., Citation2021; L. Wang et al., Citation2012). Bach & Wenz, (Citation2020) give the first insights into source selection using behavioral data. Most respondents used websites covering health and fitness, exercise and weight loss, alternative medicine, psychology, vitamins and food supplements, and women’s health. However, these results must be considered with the reservation that they rested on automatic domain classifications by the commercial provider Webshrinker. Above and beyond restrictions in research transparency due to the company’s proprietary methodology and the risk of classifications incompatible with scholarly definitions, the domain-level approach has the inherent drawback that we may miss out on essential parts of health-related Internet content. For example, a visit to https://en.wikipedia.org/wiki/headache would be classified as education despite the visit’s apparent health-related nature. We aim to provide a more comprehensive picture of the sources accessed after health-related searches. In addition, we aim to identify the determinants of source selection considering the characteristics of the individuals and their search queries:
RQ3a:
Which kind of sources are accessed after using a search engine?
RQ3b:
How are individual characteristics (gender, age, education) and types of information sought related to the kind of sources accessed after a search?
Methods
We conducted a secondary analysis of the German sample (n = 994) of a comparative study on news use conducted in 2019 (Stier et al., Citation2022). The data integrate participants’ passively collected individual web-browsing histories with the same participants’ survey responses. They consist of a total of 16 million website visits collected over three months. The secondary analysis’s main empirical contribution was identifying, classifying, and entangling health-related searches and website visits to investigate online HISB comprehensively. All steps are described in the subsequent sections. Additional details, including R code, replication data, and materials from the content analyses, are available from the OSF.Footnote1
Participant sample and tracking of internet use
The data was collected by the survey company Netquest (an affiliate of GfK) in compliance with EU General Data Protection Regulation. Panelists in the Netquest online access panel are incentivized to participate in online surveys and install tracking software that hooks into their browsers and logs their website visits, including the complete Uniform Resource Locator (URL), date, time, and duration. The Oxford Internet Institute’s Departmental Research Ethics Committee at the University of Oxford approved the data collection. Participants were informed about the nature of the data collection and asked for their consent to participate in surveys and Web tracking.
Data from 994 participants for whom tracking data had been collected on at least four days were used to answer RQ1, H1 and RQ2a/b. Participants selected themselves into the online access panel and subsequently into the web tracking. Despite the non-probabilistic recruitment, the sample is diverse in terms of age, gender, and education. The average age was 47 (SD = 14; 18 to 35 years: 23%, 36 to 55: 47%, 56 to 84: 30%). 48% of the participants identified as male, 52% as female.Footnote2 We distinguished between lower (28%; up to Mittlere Reife, ≈ GED), middle (38%, Mittlere Reife and vocational training or limited university entrance certificate), and higher (34%, full university entrance certificate or university degree) education. The sample distributions reasonably matched the German population in 2019: 49% were male, and 51% were female. The average age of the German population was 44,5 years. 32,8% have a low, 30% a middle, and 33,5% a high level of education (destatis.de). The analyses of source visits (RQ3a/b) are based only on data from 618 participants who searched at least once for health-related information and for whose searches at least one relevant follow-up visit could be identified. The subsample does not differ meaningfully in terms of age (M = 47 years, SD = 14; 18 to 35: 24%, 36 to 55: 47%, 56 to 84: 29%), education (lower: 27%, middle: 39%, higher: 35%), and gender (54% women, 46% men).
Identification of health-related internet searches
Column A in summarizes the multi-step process used for identifying health-related search queries from the collected URLs. The aim was to identify HISB using search engines that referred to health as understood by the WHO’s definition. We started by identifying all requests to the eight most popular search engines during the study period in Germany. The URL of a search result contains the search query, which was extracted and decoded. We constructed an extensive dictionary consisting of eleven health-related categories and one category for negative terms (i.e., terms often used with a health-related term but indicating that the search query was unrelated to human health). The dictionary was based on three sources: health-related glossaries,Footnote3 domain knowledge of the authors, and exploring the current data set. The dictionary was optimized for recall; that is, we tried to identify as many health-related search queries as possible. In the final step, two authors and a trained student assistant manually selected the truly health-related search queries. Intercoder reliability was sufficient in a test before the selection task (Krippendorff’s α = .82, n = 553 search queries).
The procedure yielded 15,532 health-related search queries, which were matched with the participant data to derive measures of an individual’s online HISB. As primary outcomes, dichotomous variables indicated whether an individual had searched for health-related information at all, both in general and in each of the 11 categories during the three-month study period. In addition, the frequency of search engine use was captured by the number of days an individual has searched for health-related information, again both in general and in each of the categories. The reported findings in the main text focus on the primary outcomes. The results for the frequency measure are provided as supplementing materials. Table A1 in the Online Appendix shows the sample statistics for the individual-level measures.
Identification and categorization of subsequently visited sources
The identified health-related searches were the starting point for studying source selection (, Column B). First, the subsequently visited URL was extracted and paired with the search query for each health-related search. As this simple rule resulted in many visits substantially unrelated to the previous query, we further scrutinized the search visit pairs with three checks: 1) We matched the search terms with the URL of the visit to probe whether at least one of the search terms with a length of at least four characters was contained in the URL. If so, we concluded that the visit was related to the search. 2) Two authors coded the remaining domains as being likely not a result of a health-related search (e.g., banking, e-mail), likely the result of a health-related search (popular websites focusing on health issues or with a health-related label), or as impossible to judge solely based on the domain. The first group of pairs was removed, the second group was included in the source categorization, and the third group was subjected to a final check. 3) Two authors and three trained student assistants manually checked all remaining search visit pairs. Intercoder reliability was sufficient in a test before the selection task (Krippendorff’s α = .78, n = 300 search visit pairs).
In the final step, all sources associated with a health-related search were sorted into one of 15 categories. The categorization was adapted from survey measurements (Link, Baumann, Linn, et al., Citation2021) and designed to reflect the diversity of online sources while reducing complexity to enable quantitative description. It captured different facets of the actors responsible for the sources and the importance of health-related content for the source (see ). Again, intercoder reliability was sufficient (Krippendorff’s α = .66, n = 161 sources).
Table 1. Categorization and frequency of selected online sources.
Statistical analysis
We used Bayesian estimation with (mildly) regularizing priors for all statistical analyses. This approach has two main advantages over frequentist estimation for our analysis. First, the results of both approaches are generally similar if there is sufficient information in the data, but the Bayesian estimator is more robust to the influence of smaller irregularities (McElreath, Citation2020). Second, the approach enables the reliable estimation of complex models with locally sparse data even when Maximum Likelihood estimation fails, as is the case for our last analysis (RQ3b).
The results for RQ1, H1, and RQ2a/b are from (generalized) linear models with mildly regularizing priors. The outcomes were regressed on age categories, education categories, and gender. All interactions between the regressors were included in the models. The reported estimates are average adjusted predictions from these models, i.e., predictions were averaged over the sample distribution of the other regressors. The estimates are adjusted for differences in the distributions of the other variables within the comparison groups. The results for RQ3a are based on generalized linear models estimating the proportions of health information seekers using each source. The results for RQ3b are based on regularized generalized linear mixed-effects models. The outcomes, dichotomous indicators of whether a visited source was in a given category, were regressed on dichotomous indicators of the search query categories and characteristics of the individual participants (i.e., age, gender, and education groups). We report log(odds) coefficients to quantify the partial association of a search or individual characteristics with the probability of selecting a source from a category. All inferential quantities reported in the results section are posterior means with 95% posterior intervals. The Online Appendix reports all data analysis software.
Results
Health-related searches in general (RQ1, H1)
The first research question addresses the prevalence of health-related search engine use (RQ1), whereas H1 postulates that online HISBs differ by gender, age, and education (H1a-c). Overall, we estimate that about two-thirds of the population (P = .68, 95% CI: [.65, .71]) have searched for health-related information during the study period at least once. On average, these individuals searched for health-related information on 6.3 (95% CI: [5.7, 6.9]) out of the 94 recorded days (RQ1a). The differences between sociodemographic groups were mostly nuanced (H1). The share of online health seekers was relatively lowest in the oldest age group (56 to 84 years) and in the group with lower education. The differences in search frequency were similarly minor. Men searched somewhat less often than women. Among the age groups, the 36- to 55-year-olds were most active, and the oldest age group was least active (see Online Appendix, first panel of Figure A2). Therefore, the findings support that search engine use for health-related information seeking was more pronounced in females (H1a), individuals of younger age (H1b), and higher education (H1c).
Health-related searches by types of information sought (RQ2a/b)
The second research question addresses the various types of health-related information searched via search engines overall (RQ2a) and for the socio-demographic groups (RQ2b). Half the population (P = .50, 95% CI: [.47, .53]) searched for health professionals, medical specialties, or health organizations. About one-third of the population used search terms related to diseases, pathogens, or symptoms (P = .38, 95% CI: [.35, .41]) and the anatomy of the body (P = .32, 95% CI: [.30, .35]). Specific medical topics, such as vaccination, contraception, or diagnostics, were searched for by relatively few people. Searches beyond the issues of illness and medical needs were also identified, as about one-fifth of the population used search engines to find information on nutrition, exercise, or self-care (P = .22, 95% CI: [.20, .25]). The frequency of searching for the different types of information showed a similar rank order (see Online Appendix, Figure A1).
To answer RQ2b, presents the prevalence of health-related searches by type of information sought and individual characteristics. The fine-grained breakdown makes some meaningful differences visible. Men were less likely to search for all specific categories, with the notable exception of recreational drugs, where their share (P = .16, 95% CI: [.13, .20]) was double that of women (P = .08, 95% CI: [.06, .11]). Differences between the educational tiers were overall minor and of inconsistent direction. Individuals with lower education were less likely to search for information about nutrition, exercise, and self-care, as well as drugs and pharmaceutical ingredients, and more likely to search for diagnostics. Larger proportions of those with a medium and high level of education were interested in nutrition, exercise, and self-care, as well as in disease, pathogens, and symptoms. Searches for health professionals, medical specialties, and health organizations, as well as drugs and pharmaceutical ingredients, were most common among those with intermediate levels of education. Individuals with higher education were more likely to search for information about the anatomy of the body, therapies, and vaccines.
Figure 2. Prevalence of health-related internet searches by individual characteristics.
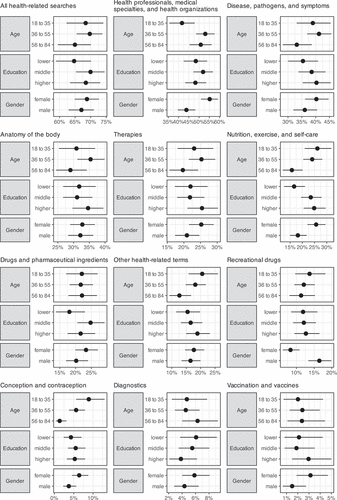
Figure 3. Prevalence of health-related internet searches by type of information.
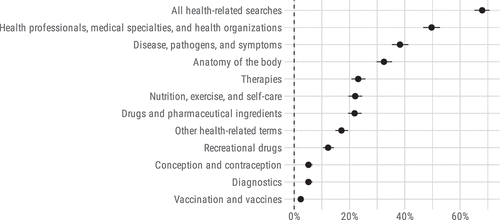
We also identified some age-related patterns. The youngest age group, 18-to-35-year-olds, were less likely to search for health professionals and more likely to use search terms related to conception and contraception as well as nutrition, exercise, and self-care. The overall lower likelihood for health-related searches in the oldest age group, 56-to-84-year-olds, was particularly pronounced in the categories of diseases, pathogens, and symptoms, as well as therapies. They were also less interested in nutrition, exercise, and self-care, as well as conception and contraception. In contrast, they were more likely to search for information about diagnostics. The respondents aged between 36 to 55 years – characterized by the overall highest likelihood for health-related searches – were most likely to search for information about disease, pathogens, and symptoms, the anatomy of the body, therapies, as well as health professionals, medical specialties, and health organizations.
Source selection after health-related searches (RQ3a/b)
The third research question asked about source selection after submitting a query to a search engine. shows the shares of online health information seekers (i.e., individuals with at least one health-related search) who selected a source type at least once after a search (RQ3a).
Figure 4. Sources selected after health-related searches.
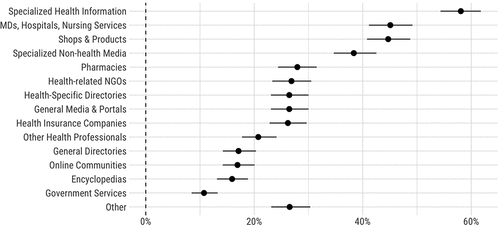
Specialized health websites were the most popular sources (P = .58, 95% CI: [.54, .62]). Websites of medical doctors, hospitals, and nursing services were also commonly selected. About a quarter to a fifth of health information seekers selected websites from other health-related actors, such as pharmacies, health-related NGOs, health insurance companies, and other health professionals (e.g., physiotherapists, health coaches), and used health-specific directories. Online shops and product presentations were the most common not-health-specific source categories. Content from special-interest, non-health media outlets and portals as well as from general-interest media outlets and portals, was also regularly selected from the search results. Online communities, including general-interest and topic-specific community platforms, were chosen only by about 17%. Finally, it is noteworthy that while relatively few individuals selected sources from the category general-interest encyclopedias compared to other categories, wikipedia.org was the second-most-popular single domain.
RQ3b asks how individual characteristics and the types of information sought were related to the kind of sources accessed after a search. Because of the large scope of the models, the presentation focuses on the seven most common types of information sought () and the individual characteristics (). The Online Appendix (Figure A3) provides a complete overview of the results.
Figure 5. Source selection by type of information sought.
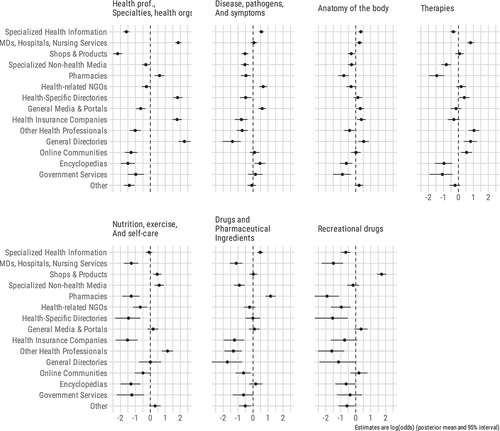
Figure 6. Source selection by individual characteristics.
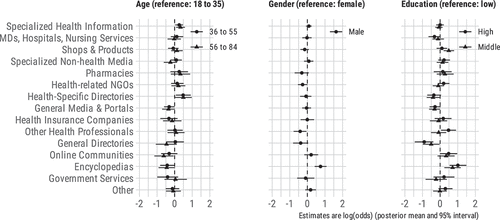
Focusing on the relationship between the types of information sought and the selected sources, searches for health professionals, medical specialties, and health organizations were most likely followed by visits to the websites of actors of the healthcare system (e.g., health professionals), health-specific directories (e.g., jameda.de) and general directories (e.g., Google Maps). A similarly distinctive pattern emerged for searches for drugs and pharmaceutical ingredients, most likely leading to visits to (online) pharmacies or specialized health information websites (see ).
Searches for diseases, pathogens, or symptoms were more likely followed by the selection of expert sources such as specialized health information websites and websites of health-related NGOs, as well as general-interest media outlets and Wikipedia (encyclopedias).
Searches related to therapies led mainly to visiting those who provided the therapies: medical doctors, hospitals, and nursing services; other health professionals; and information provided about these institutions and professionals in general directories. Notably, online communities were also among the more likely follow-up visits.
Queries about nutrition, exercise, and self-care were more likely followed by visiting online shops, product information websites, and special-interest, not health-related media outlets. Information from other health professionals (e.g., fitness coaches or nutritionists) was also more frequently selected from the search results.
Searching for recreational drugs primarily led to visits to shops and product information. Search terms that referred to the anatomy of the human body were not strongly related to the selection of sources in specific categories but led to a somewhat more likely selection of general directories, health insurance companies, and general media and portals.
When accounting for the search interests, the sociodemographic characteristics of age, gender, and education had relatively weak relationships with individuals’ source selection (see ). Older individuals were less likely to visit general directories and online communities than younger individuals. Younger individuals were less likely to use health-specific directories and websites of pharmacies but more likely to turn to encyclopedias than both older age groups. Lower education was associated with a higher probability of selecting general directories, health-specific directories, and general media and portals and a lower probability of selecting encyclopedias. Individuals with a higher level of education are further characterized by a higher probability of turning to other health professionals than individuals with a low level of education. Men, compared to women, were more likely to use encyclopedias but less likely to turn to other health professionals and general directories.
Discussion
We analyzed user-centric Internet tracking data to provide an accurate and detailed characterization of health-related search engine use and its users. Our study revealed two key findings. First, the analysis of passively measured behavioral data showed similar patterns as earlier studies based on survey self-reports, instilling confidence in the prior literature on online HISB. Second, the new data source enabled a more granular picture of the process of HISB with search engines, opening new opportunities for future theorizing.
Towards a more robust picture of health-related search engines use
In contrast to research on general Internet use (Scharkow, Citation2016) or political news use (Prior, Citation2009) which identified discrepancies between self-reports and tracking data, the patterns found in the passive observations of health-related search engine use showed pronounced commonalities with the patterns identified in previous studies using survey self-reports to examine online HISB. This strengthened confidence in the top-level findings from earlier research, which is crucial for the entire field of health communication using survey data to describe and understand online HISB. From a methodological perspective, the higher concordance between self-reports and behavioral data aligns with evidence that more specific forms of media use suffer less from reporting errors than more general media use patterns (Scharkow, Citation2016). In addition, the emotional importance of health-related issues (Brashers, Citation2001) might also improve recall. Two similarities between our findings and research based on survey data are particularly noteworthy.
First, the share of online health information seekers, the frequency of online HISB, and the most common sources resembled current findings of survey data (e.g., Finney Rutten et al., Citation2019; Link, Baumann, Linn, et al., Citation2021; Ratcliff et al., Citation2021). Over two in three Internet users searched for health information and services using general-purpose search engines, navigating them to websites for specialized health information and websites of health professionals and other health actors (Beck et al., Citation2014; Link, Baumann, Linn, et al., Citation2021; Ratcliff et al., Citation2021; L. Wang et al., Citation2012). However, we found a significantly higher share of online seekers than in an earlier tracking study from Germany (Bach & Wenz, Citation2020). The differences highlighted the need to consider health information’s various facets beyond illness and disease. Based on our findings, search engines can thus be regarded as an established tool for HISB (Brkic et al., Citation2021; Hassan & Masoud, Citation2021; Jadhav et al., Citation2014; Macias et al., Citation2018). Meanwhile, its usage frequency indicates that it is not an everyday behavior but triggered by interest- or problem-oriented needs (Galarce et al., Citation2011; Zhang, Citation2013).
Second, there were only minor socio-demographic differences in the prevalence of search engine use (H1a-c), the different types of health-related information (RQ2b), and source selection (RQ3b). In line with prior research, we found that women and individuals with higher education are more likely to engage in online HISB for various interest-driven as well as problem-driven purposes (Bach & Wenz, Citation2020; Baumann et al., Citation2017; Link, Baumann, Linn, et al., Citation2021; Link, Baumann, et al., Citation2022; Zimmerman & Shaw, Citation2020). With age in focus, we can further differentiate extant research. Instead of pointing to a higher prevalence of online HISB at younger ages (Link, Baumann, Linn, et al., Citation2021), our study showed that search engine use for health purposes was most prevalent among individuals aged between 36 to 55. This is an age group in which health complaints tend to increase. Problem-oriented searches, e.g., for consultations and therapies, add to the interest-oriented searches already common at a younger age, like searching for nutrition, exercise, and self-care. In addition, with a view to media socialization, it can be assumed that, on average, Internet use is more routine in this age group than among those older than 56. Media socialization might also explain the minor differences in source selection, particularly a lower openness to and experience with online communities and encyclopedias shown in the older age group. Older individuals also seemed less interested in using Internet searches for self-diagnosis and self-treatment, as indicated by their lower likelihood of searches about disease, pathogens, symptoms, and therapies.
In sum, the relatively nuanced differences related to participants’ age, gender, and education strengthen the evidence about the limited influence of socio-demographic factors on online HISB, suggesting that socio-psychological and situational factors are more crucial than individual characteristics (Kahlor, Citation2007, Citation2010). In addition, the minor differences speak against worries about digital divides regarding the use of health information within the population of (routine) Internet users. However, as more and more people get accustomed to searching for health information online, the divide between individuals who have and who have not integrated the Internet into their lives must be feared to grow even wider (Bachl, Citation2016; Viswanath & Kreuter, Citation2007).
Towards a granular picture of HISB processes with search engines
Above and beyond supporting the top-level findings from survey-based research, the integration of user-centric Internet tracking data offered novel insights into the process of online HISB. Our findings are more granular than ever possible with survey-based methods because they are built on categorizing all original search queries and source visits instead of only asking about a few categories (Pang et al., Citation2014). Moreover, the findings are process-oriented, in contrast to the static snapshots of survey research. We were able to link search queries and subsequently visited sources within search processes and combine them with person-level characteristics. Overall, we got a clearer picture of the actual HISB, which in turn allows us to make inferences about likely information needs and how they were fulfilled using online health information (Chi et al., Citation2020).
The fine-grained recordings of the search queries allowed more precise descriptions of the health-related functions of search engines, which enriches the prior literature on health-related information needs, sought health issues and their fulfillment using online HISB (e.g., Cao et al., Citation2016; Link, Baumann, Linn, et al., Citation2021; Palotti et al., Citation2016). The most common category included search queries about health professionals, medical specialties, and health organizations. The finding indicates search engines’ essential role in navigating the modern healthcare system because they support action planning and health provision. The common inquiries about diseases, pathogens, and symptoms suggest that people also turn to search engines to increase their understanding of health concerns. On a more general level, the findings suggest that health-related searches are triggered by both information (e.g., understanding disease, pathogens, or symptoms) and instrumental needs (e.g., navigating the healthcare system). In addition, more searches were problem- rather than interest-driven. They referred to specific health-related burdens or uncertainties, including searches for health professionals and search queries related to disease, pathogens, or symptoms. Interest-driven HISB, such as queries related to information about nutrition, exercise, or self-care (Brashers, Citation2001; van der Rijt, Citation2000), was less common.
This distinction between patterns of HISB related to various needs and sought issues should be considered when modeling the process of online HISB. Regarding the process from information needs to search queries and source selection behaviors, our findings revealed two general patterns. First, some search-selection processes showed a close correspondence between the type of information searched for and the selected sources. The pattern is characterized by a narrow scope and selection from a rather small set of information sources. This pattern was most notable when the queries looked for instrumental support or very specific informational support, for example, queries on health professionals, drugs and pharmaceutical ingredients, and recreational drugs. Particular sources, such as health professionals’ and pharmacies’ websites or online shops, offer these types of support. For example, particular needs like recommendations for a nearby dentist, searches for drugs, or the range of therapies offered by a physiotherapist indicate specific instrumental and information needs, which online information and services can quickly fulfill.
Second, medical and disease-related informational support needs, as inferred from the search queries, led to selecting a broader, more diverse set of sources. For example, individuals turned most likely to general media, health insurance companies, and general directories when their searches referred to the anatomy of the human body. Searches for diseases, pathogens, and symptoms were associated with a higher probability of selecting specialized health information, health-related NGO websites, general media, and encyclopedias. In general, expert sources were among the most likely selected, suggesting a preference for high-quality information. The attempt to evaluate information quality is reflected in the preference for sources of health information over sources of health community (Gitlow, Citation2000; Rossmann & Karnowski, Citation2014).
In summary, two selection patterns emerged. The first pattern is characterized by a narrow scope and close correspondence of searches and sources. Search engines are used as instrumental support tools for navigating the healthcare system. The second pattern is characterized by a broader scope and greater source type variety, corresponding to various medical and disease-related information needs. Of course, these prototypical descriptions are only a first attempt at understanding the individual and situational processes of online HISB in detail. They call for developing theories that focus on the process of online HISB that explain how individual differences and situational needs impact search processes and their outcomes. Such theories must build on established health communication models explaining information-seeking intentions (Griffin et al., Citation1999; Kahlor, Citation2010). They must be supplemented with a dynamic perspective on the mutual influences of seeking behaviors and fulfilled and newly emerging needs during a search process and its health-related outcomes. Generic models from the information science literature (e.g., Kuhlthau, Citation1991; Zhang, Citation2013) can provide some guidance, but they need reflection from a dedicated health communication perspective.
Limitations and tasks for further research
The study has several limitations that provide starting points for further research. First, we must assume that participants in an opt-in web tracking panel are usually experienced Internet users who might be more inclined to health-related online behaviors than the average user. Second, several blind spots of tracking data, such as omissions and measurement errors when respondents turn off the tracking, tracking only some devices, or technical or user errors, must be considered (Scharkow, Citation2016). Third, we were able to analyze individuals’ online behaviors but not their motives. Our descriptive and explorative approach, which was necessary because of the limitations of the secondary analysis, had to neglect the theoretically-derived motivational factors of information behaviors subsumed, for example, in models such as the Planned Risk Information Seeking Model (Kahlor, Citation2010; Link, Baumann, & Klimmt, Citation2021; X. Wang et al., Citation2021). Fourth, we focused on types of sources but did not analyze the actual content of each visit. Work on research infrastructures is needed to remedy the first and the second limitations. The communication research community desperately needs high-quality respondent panels in which user-centered Internet use tracking is conducted across devices and with better coverage while preserving the respondents’ privacy. To address the third and fourth shortcomings, future research should, among others, expand the survey section with dedicated health-related questions and consider the content of the visited pages. Fifth, the data were collected before the COVID-19 pandemic, which raises the question whether the results still hold today. Even though COVID-19 may have displaced many other health topics and contributed to an increase in online use, studies from Germany before, during and after the pandemic suggest no sustained boost in digitalization (Link, Czerwinski, et al., Citation2022). We, therefore, assume that the general patterns of search engine use identified in this study persist as society enters a post-pandemic state. Of course, this assumption needs to be tested in future studies.
Conclusion
The similarities of the findings based on user-centric Internet tracking data and prior survey research support the viability of existing findings. Furthermore, we provided a granular picture of the search processes showing either specific needs for instrumental support or a broader search for information related to health challenges. These findings also show which health information needs could be addressed by online health communication interventions, and they suggest appropriate sources for health campaigns. In addition, the function of search engines to prepare for and supplement consultations became apparent. They serve a crucial navigation function within the healthcare system, highlighting the need for search engine optimization of expert sources such as health professionals.
HealthSearches_Appendix.pdf
Download PDF (78.5 KB)Acknowledgements
We thank Sophie Mann, Sabine Sonnemann and Mona Wehming for supporting the identification and categorization of the visited URLs. Sebastian Stier acknowledges the Volkswagen Foundation, which has funded the data collection of the original study (grant number 94 758).
Disclosure statement
No potential conflict of interest was reported by the author(s).
Supplementary material
Supplemental data for this article can be accessed online at https://doi.org/10.1080/10410236.2024.2309810
Correction Statement
This article was originally published with errors, which have now been corrected in the online version. Please see Correction (http://dx.doi.org/10.1080/10410236.2024.2318084)
Additional information
Funding
Notes
2. One person who identified as non-binary had to be excluded from the quantitative analysis. While this was necessary because of the statistical approach, it also highlights the lack of research effort and knowledge on non-binary and genderqueer groups in health communication. Research including and addressing these groups is needed for broader inclusivity.
3. From netdoktor.de, gesundheitsinformationen.de, and de.wikipedia.org.
References
- Agarwal, V., Zhang, L., Zhu, J., Fang, S., Cheng, T., Hong, C., & Shah, N. (2016). Impact of predicting health care utilization via web search behavior: A data-driven analysis. Journal of Medical Internet Research, 18(9), e251. https://doi.org/10.2196/jmir.6240
- Alvarez-Galvez, J., Salinas-Perez, J. A., Montagni, I., & Salvador-Carulla, L. (2020). The persistence of digital divides in the use of health information: A comparative study in 28 European countries. International Journal of Public Health, 65(3), 325–333. https://doi.org/10.1007/s00038-020-01363-w
- Asseo, K., Fierro, F., Slavutsky, Y., Frasnelli, J., & Niv, M. Y. (2020). Tracking COVID-19 using taste and smell loss Google searches is not a reliable strategy. Scientific Reports, 10(1), 20527. https://doi.org/10.1038/s41598-020-77316-3
- Ayers, S. L., & Kronenfeld, J. J. (2007). Chronic illness and health-seeking information on the Internet. Health: An Interdisciplinary Journal for the Social Study of Health, Illness and Medicine, 11(3), 327–347. https://doi.org/10.1177/1363459307077547
- Bach, R. L., & Wenz, A. (2020). Studying health-related internet and mobile device use using web logs and smartphone records. PloS One, 15(6), e0234663. https://doi.org/10.1371/journal.pone.0234663
- Bachl, M. (2016). Online health information seeking in Europe: Do digital divides persist? Studies in Communication and Media, 5(4), 427–453. https://doi.org/10.5771/2192-4007-2016-4-427
- Baumann, E., Czerwinski, F., & Reifegerste, D. (2017). Gender-specific determinants and patterns of online health information seeking: Results from a representative German health survey. Journal of Medical Internet Research, 19(4), e92. https://doi.org/10.2196/jmir.6668
- Beck, F., Richard, J.-B., Nguyen-Thanh, V., Montagni, I., Parizot, I., & Renahy, E. (2014). Use of the internet as a health information resource among French young adults: Results from a nationally representative survey. Journal of Medical Internet Research, 16(5), e128. https://doi.org/10.2196/jmir.2934
- Brashers, D. E. (2001). Communication and uncertainty management. Journal of Communication, 51(3), 477–497. https://doi.org/10.1111/j.1460-2466.2001.tb02892.x
- Brkic, F. F., Besser, G., Schally, M., Schmid, E. M., Parzefall, T., Riss, D., & Liu, D. T. (2021). Biannual differences in interest peaks for web inquiries into ear pain and ear drops: Infodemiology study. Journal of Medical Internet Research, 23(6), e28328. https://doi.org/10.2196/28328
- Cao, W., Zhang, X., Xu, K., & Wang, Y. (2016). Modeling online health information-seeking behavior in China: The roles of source characteristics, reward assessment, and internet self-efficacy. Health Communication, 31(9), 1105–1114. https://doi.org/10.1080/10410236.2015.1045236
- Cartright, M.-A., White, R. W., & Horvitz, E. (2011). Intentions and attention in exploratory health search. In W.-Y. Ma, J.-Y. Nie, R. Baeza-Yates, T.-S. Chua & W. B. Croft (Eds.), Proceedings of the 34th international ACM SIGIR conference on research and development in information retrieval (pp. 65–74). ACM. https://doi.org/10.1145/2009916.2009929
- Case, D. O. (2002). Looking for information: A survey of research on information seeking, needs, and behavior. Elsevier/Academic Press.
- Cervellin, G., Comelli, I., & Lippi, G. (2017). Is Google trends a reliable tool for digital epidemiology? Insights from different clinical settings. Journal of Epidemiology and Global Health, 7(3), 185–189. https://doi.org/10.1016/j.jegh.2017.06.001
- Chan, E. H., Sahai, V., Conrad, C., Brownstein, J. S., & Aksoy, S. (2011). Using web search query data to monitor dengue epidemics: A new model for neglected tropical disease surveillance. PloS Neglected Tropical Diseases, 5(5), e1206. https://doi.org/10.1371/journal.pntd.0001206
- Chi, Y., He, D., & Jeng, W. (2020). Laypeople’s source selection in online health information-seeking process. Journal of the Association for Information Science and Technology, 71(12), 1484–1499. https://doi.org/10.1002/asi.24343
- Choudhury, M., de Morris, M. R., & White, R. W. (2014). Seeking and sharing health information online. Proceedings of the SIGCHI Conference on Human Factors in Computing Systems, 1365–1376. https://doi.org/10.1145/2556288.2557214
- Christner, C., Urman, A., Adam, S., & Maier, M. (2022). Automated tracking approaches for studying online media use: A critical review and recommendations. Communication Methods and Measures, 16(2), 79–95. https://doi.org/10.1080/19312458.2021.1907841
- Cline, R. J., & Haynes, K. M. (2001). Consumer health information seeking on the Internet: The state of the art. Health Education Research, 16(6), 671–692. https://doi.org/10.1093/her/16.6.671
- Dvir-Gvirsman, S., Tsfati, Y., & Menchen-Trevino, E. (2016). The extent and nature of ideological selective exposure online: Combining survey responses with actual web log data from the 2013 Israeli elections. New Media & Society, 18(5), 857–877. https://doi.org/10.1177/1461444814549041
- Eysenbach, G. (2009). Infodemiology and infoveillance: Framework for an emerging set of public health informatics methods to analyze search, communication and publication behavior on the Internet. Journal of Medical Internet Research, 11(1), e11. https://doi.org/10.2196/jmir.1157
- Finney Rutten, L. J., Blake, K. D., Greenberg-Worisek, A. J., Allen, S. V., Moser, R. P., & Hesse, B. W. (2019). Online health information seeking among US adults: Measuring progress toward a healthy people 2020 objective. Public Health Reports, 134(6), 617–625. https://doi.org/10.1177/0033354919874074
- Fox, S., & Duggan, M. (2013). Health online 2013. Pew Research Center. http://www.pewinternet.org/files/old-media/Files/Reports/PIP_HealthOnline.pdfhttp://www.pewinternet.org/files/old-media/Files/Reports/PIP_HealthOnline.pdf
- Freelon, D. (2014). On the interpretation of digital trace data in communication and social computing research. Journal of Broadcasting & Electronic Media, 58(1), 59–75. https://doi.org/10.1080/08838151.2013.875018
- Galarce, E. M., Ramanadhan, S., & Viswanath, K. (2011). Health information seeking. In T. L. Thompson, R. Parrott & J. F. Nussbaum (Eds.), The Routledge handbook of health communication (2nd ed., pp. 167–180). Routledge.
- Ginsberg, J., Mohebbi, M. H., Patel, R. S., Brammer, L., Smolinski, M. S., & Brilliant, L. (2009). Detecting influenza epidemics using search engine query data. Nature, 457(7232), 1012–1014. https://doi.org/10.1038/nature07634
- Gitlow, S. (2000). The online community as a healthcare resource. In D. B. Nash, M. P. Manfredi, B. Bozarth & S. Howell (Eds.), Connecting with the new healthcare consumer: Defining your strategy (pp. 113–133). Mc-Graw-Hill.
- Goldner, M. (2006). How health status impacts the types of information consumers seek online. Information, Communication & Society, 9(6), 693–713. https://doi.org/10.1080/13691180601063982
- Griffin, R. J., Dunwoody, S., & Neuwirth, K. (1999). Proposed model of the relationship of risk information seeking and processing to the development of preventive behaviors. Environmental Research, 80(2 Pt 2), S230–S245. https://doi.org/10.1006/enrs.1998.3940
- Guess, A. M., Nyhan, B., O’Keeffe, Z., & Reifler, J. (2020). The sources and correlates of exposure to vaccine-related (mis)information online. Vaccine: X, 38(49), 7799–7805. https://doi.org/10.1016/j.vaccine.2020.10.018
- Hartoonian, N., Ormseth, S. R., Hanson, E. R., Bantum, E. O., & Owen, J. E. (2014). Information-seeking in cancer survivors: Application of the comprehensive model of information seeking to HINTS 2007 data. Journal of Health Communication, 19(11), 1308–1325. https://doi.org/10.1080/10810730.2013.872730
- Hassan, S., & Masoud, O. (2021). Online health information seeking and health literacy among non-medical college students: Gender differences. Journal of Public Health, 29(6), 1267–1273. https://doi.org/10.1007/s10389-020-01243-w
- Hochberg, I., Allon, R., & Yom-Tov, E. (2020). Assessment of the frequency of online searches for symptoms before diagnosis: Analysis of archival data. Journal of Medical Internet Research, 22(3), e15065. https://doi.org/10.2196/15065
- Jadhav, A., Andrews, D., Fiksdal, A., Kumbamu, A., McCormick, J. B., Misitano, A., Nelsen, L., Ryu, E., Sheth, A., Wu, S., & Pathak, J. (2014). Comparative analysis of online health queries originating from personal computers and smart devices on a consumer health information portal. Journal of Medical Internet Research, 16(7), e160. https://doi.org/10.2196/jmir.3186
- Johnson, J. D., & Meischke, H. (1993). A comprehensive model of cancer-related information seeking applied to magazines. Human Communication Research, 19(3), 343–367. https://doi.org/10.1111/j.1468-2958.1993.tb00305.x
- Jun, S.-P., Yoo, H. S., & Choi, S. (2018). Ten years of research change using Google trends: From the perspective of big data utilizations and applications. Technological Forecasting & Social Change, 130, 69–87. https://doi.org/10.1016/j.techfore.2017.11.009
- Jung, M. (2015). Identifying social characteristics of health-related information seeker: A gender-specific approach for cancer survivors. Asian Pacific Journal of Cancer Prevention: APJCP, 16(5), 1865–1871. https://doi.org/10.7314/APJCP.2015.16.5.1865
- Kahlor, L. (2010). PRISM: A planned risk information seeking model. Health Communication, 25(4), 345–356. https://doi.org/10.1080/10410231003775172
- Kahlor, L. A. (2007). An augmented risk information seeking model: The case of global warming. Media psychology, 10(3), 414–435. https://doi.org/10.1080/15213260701532971
- Kreps, G. L. (2017). Online information and communication systems to enhance health outcomes through communication convergence. Human Communication Research, 43(4), 518–530. https://doi.org/10.1111/hcre.12117
- Kuhlthau, C. C. (1991). Inside the search process: Information seeking from the user’s perspective. Journal of the American Society for Information Science and Technology, 42(5), 361–371. https://doi.org/10.1002/(SICI)1097-4571(199106)42:5<361:AID-ASI6>3.0.CO;2-%23
- Lambert, S. D., & Loiselle, C. G. (2007). Health information seeking behavior. Qualitative Health Research, 17(8), 1006–1019. https://doi.org/10.1177/1049732307305199
- Lee, E. W. J., Bekalu, M. A., McCloud, R. F., & Viswanath, K. (2023). Toward an extended infodemiology framework: Leveraging social media data and web search queries as digital pulse on cancer communication. Health Communication, 38(2), 335–348. https://doi.org/10.1080/10410236.2021.1951957
- Link, E., & Baumann, E. (2020). Nutzung von Gesundheitsinformationen im Internet: personenbezogene und motivationale Einflussfaktoren. [Use of health information on the internet: personal and motivational influencing factors]. Bundesgesundheitsblatt - Gesundheitsforschung - Gesundheitsschutz, 63(6), 681–689. https://doi.org/10.1007/s00103-020-03144-5
- Link, E., Baumann, E., & Klimmt, C. (2021). Explaining online information seeking behaviors in people with different health statuses: German representative cross-sectional survey. Journal of Medical Internet Research, 23(12), e25963. https://doi.org/10.2196/25963
- Link, E., Baumann, E., Kreps, G. L., Czerwinski, F., Rosset, M., & Suhr, R. (2022). Expanding the Health Information National Trends Survey Research Program internationally to examine global health communication trends: Comparing health information seeking behaviors in the U.S. and Germany. Journal of Health Communication, 27(8), 545–554. https://doi.org/10.1080/10810730.2022.2134522
- Link, E., Baumann, E., Linn, A., Fahr, A., Schulz, P., & Abuzahra, M. E. (2021). Influencing factors of online health information seeking in selected European countries. European Journal of Health Communication, 2(1), 29–55. https://doi.org/10.47368/ejhc.2021.002
- Link, E., Czerwinski, F., Calhoun, K., Grimm, M., Großmann, U., & Baumann, E. (2022). Hat sich das Informationsverhalten zu Gesundheitsthemen in der Corona-Pandemie verändert? Teilergebnisse der Studie “HINTS Germany”. [Has information behavior on health topics changed during the COVID-19 pandemic? Partial results of the “HINTS Germany” study]. Trendmonitor: Der Monitor für aktuelles Gesundheitswissen, 6. https://www.stiftung-gesundheitswissen.de/sites/default/files/pdf/20022_03_24_tm_trends_informationsverhalten_vf_0.pdf
- Macias, W., Lee, M., & Cunningham, N. (2018). Inside the mind of the online health information searcher using think-aloud protocol. Health Communication, 33(12), 1482–1493. https://doi.org/10.1080/10410236.2017.1372040
- Mavragani, A., & Ochoa, G. (2019). Google trends in infodemiology and infoveillance: Methodology framework. JMIR Public Health and Surveillance, 5(2), e13439. https://doi.org/10.2196/13439
- McElreath, R. (2020). Statistical rethinking: A Bayesian course with examples in R and Stan (2nd ed.). Taylor and Francis, CRC Press.
- Niederdeppe, J., Hornik, R. C., Kelly, B. J., Frosch, D. L., Romantan, A., Stevens, R. S., Barg, F. K., Weiner, J. L., & Schwartz, J. S. (2007). Examining the dimensions of cancer-related information seeking and scanning behavior. Health Communication, 22(2), 153–167. https://doi.org/10.1080/10410230701454189
- Ofran, Y., Paltiel, O., Pelleg, D., Rowe, J. M., Yom-Tov, E., & Holme, P. (2012). Patterns of information-seeking for cancer on the internet: An analysis of real world data. PloS One, 7(9), e45921. https://doi.org/10.1371/journal.pone.0045921
- Ou, M., & Ho, S. S. (2022). A meta-analysis of factors related to health iInformation seeking: An integration from six theoretical frameworks. Communication Research, 49(4), 567–593. https://doi.org/10.1177/00936502211043024
- Palotti, J., Hanbury, A., Müller, H., & Kahn, C. E. (2016). How users search and what they search for in the medical domain. Information Retrieval Journal, 19(1–2), 189–224. https://doi.org/10.1007/s10791-015-9269-8
- Pang, P., Chang, S., Pearce, J., & Verspoor, K. (2014). Online health information behaviour: Understanding different search approaches. PACIS 2014 Processings. https://aisel.aisnet.org/pacis2014/229
- Phillips, C. A., Barz Leahy, A., Li, Y., Schapira, M. M., Bailey, L. C., & Merchant, R. M. (2018). Relationship between state-level google online search volume and cancer incidence in the United States: Retrospective study. Journal of Medical Internet Research, 20(1), e6. https://doi.org/10.2196/jmir.8870
- Powell, J., Inglis, N., Ronnie, J., & Large, S. (2011). The characteristics and motivations of online health information seekers: Cross-sectional survey and qualitative interview study. Journal of Medical Internet Research, 13(1), e20. https://doi.org/10.2196/jmir.1600
- Prior, M. (2009). The immensely inflated news audience: Assessing bias in self-reported news exposure. Public Opinion Quarterly, 73(1), 130–143. https://doi.org/10.1093/poq/nfp002
- Qin, J., & Peng, T.-Q. (2016). Googling environmental issues. Internet Research, 26(1), 57–73. https://doi.org/10.1108/IntR-04-2014-0104
- Quinn, S., Bond, R., & Nugent, C. (2017). Quantifying health literacy and eHealth literacy using existing instruments and browser-based software for tracking online health information seeking behavior. Computers in Human Behavior, 69, 256–267. https://doi.org/10.1016/j.chb.2016.12.032
- Ratcliff, C. L., Krakow, M., Greenberg-Worisek, A., & Hesse, B. W. (2021). Digital health engagement in the US population: Insights from the 2018 health information national trends survey. American Journal of Public Health, 111(7), 1348–1351. https://doi.org/10.2105/AJPH.2021.306282
- Rossmann, C., & Karnowski, V. (2014). eHealth & mHealth: Gesundheitskommunikation online und mobil. In K. Hurrelmann & E. Baumann (Eds.), Handbuch Gesundheitskommunikation (pp. 271–285). Verlag Hans Huber.
- Sbaffi, L., & Zhao, C. (2020). Modeling the online health information seeking process: Information channel selection among university students. Journal of the Association for Information Science and Technology, 71(2), 196–207. https://doi.org/10.1002/asi.24230
- Scharkow, M. (2016). The accuracy of self-reported Internet use—A validation study using client log data. Communication Methods and Measures, 10(1), 13–27. https://doi.org/10.1080/19312458.2015.1118446
- Scharkow, M. (2019). The reliability and temporal stability of self-reported media exposure: A meta-analysis. Communication Methods and Measures, 13(3), 198–211. https://doi.org/10.1080/19312458.2019.1594742
- Stier, S., Breuer, J., Siegers, P., & Thorson, K. (2020). Integrating survey data and digital trace data: Key issues in developing an emerging field. Social Science Computer Review, 38(5), 503–516. https://doi.org/10.1177/0894439319843669
- Stier, S., Mangold, F., Scharkow, M., & Breuer, J. (2022). Post post-broadcast democracy? News exposure in the age of online intermediaries. American Political Science Review, 116(2), 768–774. https://doi.org/10.1017/S0003055421001222
- van der Rijt, G. A. J. (2000). Health complaints and the search for health information. Communications, 25(2), 143–160. https://doi.org/10.1515/comm.2000.25.2.143
- Viswanath, K., & Kreuter, M. W. (2007). Health disparities, communication inequalities, and eHealth. American Journal of Preventive Medicine, 32(5), S131–S133.
- Wang, L., Wang, J., Wang, M., Li, Y., Liang, Y., & Xu, D. (2012). Using internet search engines to obtain medical information: A comparative study. Journal of Medical Internet Research, 14(3), e74. https://doi.org/10.2196/jmir.1943
- Wang, X., Shi, J., & Kong, H. (2021). Online health information seeking: A review and meta-analysis. Health Communication, 36(10), 1163–1175. https://doi.org/10.1080/10410236.2020.1748829
- WHO. (1946). Constitution of the World Health Organization. https://www.who.int/about/governance/constitution
- Wieland, M., Der Au, A.-M., Keller, C., Brunk, S., Bettermann, T., Hagen, L. M., & Schlegel, T. (2018). Online behavior tracking in social sciences: Quality criteria and technical implementation. In C. Stützer, M. Welker & M. Egger (Eds.), Computational social science in the age of big data: Concepts, methodologies, tools, and applications (pp. 131–160). Herbert von Halem.
- Yang, Z. J., Aloe, A. M., & Feeley, T. H. (2014). Risk information seeking and processing model: A meta-analysis. Journal of Communication, 64(1), 20–41. https://doi.org/10.1111/jcom.12071
- Zare-Farashbandi, F., & Lalazaryan, A. (2014). A review of models and theories of health information seeking behavior. International Journal of Health System and Disaster Management, 2(4), 193. https://doi.org/10.4103/2347-9019.144371
- Zhang, Y. (2013). Consumer health information searching process in real life settings. Proceedings of the American Society for Information Science and Technology, 49(1), 1–10. https://doi.org/10.1002/meet.14504901046
- Zhang, Y., Sun, Y., & Kim, Y. (2017). The influence of individual differences on consumer’s selection of online sources for health information. Computers in Human Behavior, 67, 303–312. https://doi.org/10.1016/j.chb.2016.11.008
- Zimmerman, M. S., & Shaw, G. (2020). Health information seeking behaviour: A concept analysis. Health Information and Libraries Journal, 37(3), 173–191. https://doi.org/10.1111/hir.12287
- Zschorlich, B., Gechter, D., Janßen, I. M., Swinehart, T., Wiegard, B., & Koch, K. (2015). Gesundheitsinformationen im Internet: Wer sucht was, wann und wie? [Health information on the Internet: Who is searching for what, when and how?]. Zeitschrift für Evidenz, Fortbildung und Qualität im Gesundheitswesen, 109(2), 144–152. https://doi.org/10.1016/j.zefq.2015.03.003