ABSTRACT
Social media and the resulting tidal wave of the available data have changed the ways and methods researchers analyze communities at scale. But the full potential for social scientists (and others) is not yet achieved. Despite the popularity of social media analysis in the past decade, few researchers invest in cross-platform analyses. This is a major oversight as a majority of online social media users have multiple social media accounts. Missing are the models and tools necessary to undertake analysis at scale across multiple platforms. Especially promising in support of cross-platform analysis is the mixed-method approach (e.g., qualitative and quantitative methods) in order to better understand how users and society interact online. This special issue “Following User Pathways” addresses methodological, analytical, conceptual, and technological challenges and opportunities of cross-platform analysis in social media ecosystems.
Social media users traverse well-trodden paths across their preferred platforms, even as individual’s breadth and depth may vary significantly. Research works concentrating on theories and applications of cross-platform social media are notably absent from the current discourse. This special issue continues the conversation of the “Following User Pathways” workshop introduced at association for computing machinery (ACM) factors in human–computer interaction in 2015 (Hall et al., Citation2016). In spite of the prevalence and availability of application programming interfaces (APIs) for data extraction, and an almost endless supply of papers and studies that focus on extraction and analysis of specific platforms, cross-platform analyses are lacking in the social media research domain. This is an oversight, in an age where over half of social media users have multiple social media profiles.Footnote1 Chorley and Williams (Citation2017) define social media as the set of applications having three characteristics:
The capability to support user-generated content.
The provision of a means for users to connect with one another.
Support for members to engage with one another.
A deeper discussion on what exactly is “social media” can be found in McCay-Peet and Quan-Haase (2017) and Chorley and Williams (Citation2017), as well as in Chorley and Williams (Citation2017).
This article discusses the merits and challenges of cross-platform analyses, and introduces four new studies in the cross-platform domain (Grange, Citation2018; Jordan, Citation2018; Kazanidis, Pellas, Fotaris, & Tsinakos, Citation2018; Lowenstein & Lev-On, Citation2018). Social media analyses, such as those done in marketing (Li & Duan, Citation2014; Phethean, Tiropanis, & Harris, Citation2015; Schacht, Hall, & Chorley, Citation2015), politics (Caton, Hall, & Weinhardt, Citation2015; Chung & Mustafaraj, Citation2011; Ratkiewicz et al., Citation2011), and bioinformatics (Bloom, Amber, Hu, & Kirsner, Citation2015; Jiang, Citation2017; Narimatsu, Sugawara, & Fukao, Citation2012; Tresp et al., Citation2016), pioneer applications for social media analyses to support better outcomes for individuals, commerce, and society. However, new technologies and permeability of the social media realms and real-life realms demand more accurate, more complete, and suitable research design.
A level of yet-unknown research bias also exists due to literature’s current concentration on single-platform analyses (Ruppert, Citation2013). The current maturity level and the reliability of social media and social network research are lower than its potential due to this oversight. In order to facilitate more realistic analyses, social models, and theories, researchers need to approach social media as a holistic ecosystem: the scientific community must map user pathways to match users’ activities.
Foundational articles comparing the same user or phenomenon on different social media platforms, or path mapping can be found (Davenport, Bergman, Bergman, & Fearrington, Citation2014; Lin & Qiu, Citation2013; Schacht et al., Citation2015), but are still limited in scope. It has, for example, been established that individuals’ sentiment valence and conversation styles differ across platforms (Davenport et al., Citation2014; Lin & Qiu, Citation2013), and that unrelated posts on different social media platforms can predict return on advertising investments (Schacht et al., Citation2015). Yet, because users cannot be tracked across platforms the available tools do not match the research need up to now (Kivelä & Lyytinen, Citation2004). Missing is a better concept of how to plug all these artifacts together for the benefit as opposed to exploitation of users as well as (social) researchers.
While it is apparent that the technical functionalities exist for capturing multiple streams of data, currently few scientific papers or services undertake this in a way that is easily replicable. Few platforms or packages exist which pull social media data from multiple platforms in order to facilitate cross-platform analyses. Nor do many works exist focusing on platforms with smaller market shares or nonstandard combinations (e.g., social media and learning platforms (see Kazanidis et al., Citation2018)). Of particular interest are mapping the pathways to include alternative platforms like enterprise social networks and online social networks which specialize in professional networks like LinkedIn, or aim at particular target groups (e.g., researchers on Mendeley or ResearchGate). Visually based social networks like YouTube, Snapchat, and Pinterest are also of interest considering their growing user bases (Hall et al., Citation2016), but the status of research makes image processing a challenge.
Several issues must be considered. Activities performed on individual platforms often cannot be compared since they are driven by different conceptual frameworks and motivations (e.g., watching a television report and reading a news blurb from social media (see Lowenstein & Lev-On, Citation2018)). For example, differences in motivation for such activities have been neither qualitatively nor theoretically addressed. Likewise unaddressed are user motivations in creating content on different platforms – i.e., what motivation does a user have behind posting a similar, different, or even the same message on different platforms (see Rhiu & Hwan Yun, Citation2018)? Here, research on the linking of conceptual and analytical models is needed.
The technical challenges also differ significantly when receiving a continuous stream of data (i.e., tweets) and paginated results (i.e., Facebook). The latter incites large numbers of API calls, which are not limitless. Restricted word counts present an interesting validation challenge considering the overall small n for posts outside of Facebook. These mismatches impact analyses considering individuals across platforms (Lin & Qiu, Citation2013). Moreover, the method of data curation is not without its ambivalence and consequences for research design. Twitter data curation tends to be forward-facing and proactive, accessing future tweets that fulfill a specific set of attributes starting at a given time point (Lemke, Mazarakis, & Peters, Citation2015) or alternatively through third-party resellers. Facebook is retrospective; given a Facebook entity (e.g., a person or page) researchers access current and historical posts, profiles, likes, etc. Visually driven sites are still different, where Snaps are transient in nature and Instagram posts are considered art by the platform, therefore leaving images unextractable. From the perspective of analyzing cross-platform social data, such subtle differences significantly alter the research design, effort, and planning needed to curate a data set and the implicit biases associated with the method (González-Bailón, Wang, Rivero, Borge-Holthoefer, & Moreno, Citation2014; Ruppert, Citation2013). For cross-platform studies, such differences, however subtle, can fundamentally inhibit researchers from answering their research question(s) if not meticulously observed.
Also, ethical considerations for research have to be taken into account (Gosling & Mason, Citation2015; Markham & Buchanan, Citation2012). A significantly higher volume of data, with a yet-unaddressed level of granularity, is afforded by cross-platform analysis. Users may be unaware of the implications of cross-platform analyses. The privacy of social media users in cross-platform approaches should be of paramount concern.
While blended approaches from physics, neuroscience, computer science, and social science are becoming more prevalent into the field of computational social science (Cioffi-Revilla, Citation2010; Lazer, Brewer, Christakis, Fowler, & King, Citation2009), the question of research methodology is often a poignant discussion point and challenge that cannot be overlooked (Grange, Citation2018; Jordan, Citation2018); computer and social scientists leverage diverse and often nonoverlapping research methodologies. Therefore, cross-platform analyses need to accommodate a vast array of (interdisciplinary) methodological approaches.
Irrespective of methodology, an important feature of cross-platform analysis is the ability to view a community at a variety of resolutions; starting from an individual micro layer and progressively zooming out via ego-centric networks, social groups, and demographic (sub)groups, up to the macro layer: community. This ability is of significant importance for understanding a community as a whole, as well as following the complete user path (Caton et al., Citation2015). Also, including temporal information in analyses of user activities across platforms (e.g., if Twitter is always visited after Facebook) can enhance the understanding of how users navigate the information space, process information, and make use of platforms, and, thus, shed light on why particular platforms are used and for what reasons. Key contribution differences are the observation viewpoint and elicitation of points of reference by analyzing multiple platforms. While the scientific value of single-platform studies is significant, their isolated investigations only give us insights into well-grounded research processes rather than assisting in the construction of a general approach or general theories of observed phenomena.
0.1. Considerations of the special issue
Due to the nature of social media data collection, it is currently not possible to validate “ground truth” in a way that standard social research approaches can. However, broad social models require ground truth for validity. To move close to this goal, researchers must consider cross-platform aspects of user identification, data filtering, user-generated content, granularity, and technical implementations (see section 2). There is also an unknown bias present in the sample associated with the medium. Individuals may or may not represent themselves truthfully, and there are issues of representativeness to consider in the research design (see section 3). Finally, in creating a cross-platform design, researchers must carefully weigh the benefit of the research output against the amount of types of (personally identifying) data required to realize the design (see section 4). In this special issue, the authors address the following conceptual and theoretical boundaries of cross-platform social media research:
1. How can a complete social media path be mapped? What does a complete representation look like? How can we tackle these challenges without being immersive? What technical affordances need to be implemented to develop a single framework?
Few instantiations exist that support cross-platform data extraction (Grange, Citation2018). Even fewer exist that support mixed-method analyses (Kazanidis et al., Citation2018; Lowenstein & Lev-On, Citation2018). It is expected that the observation lenses across platforms and with the differing methods captures differing structural, content, and temporal aspects. As such, the data must be reconciled to support a holistic analysis. Theoretical and empirical contributions addressing challenges and added value are envisioned with these questions.
Grange (Citation2018) introduces an experimental method for the analysis of hybrid social media networks. The article introduces and discusses two case studies around the myTable platform and Facebook as it impacts social interdependence, content, and social heterogeneity. The article derives a series of suggestions for designing and implementing cross-platform social media studies especially considering the management of data with multiple origin points or other combinatorial aspects.
Kazanidis et al. (Citation2018) analyze in a classic experimental design the participation of students with Facebook and Moodle. They compare the learning experience of their students with the Community of Inquiry model. This model gives insight in indicators such as cognitive, teaching, and social presence. Though both platforms seem to provide similar results, Facebook is in terms of social presence still superior.
Lowenstein and Lev-On (Citation2018) approach multitasking from the angle of news consumption and social media platforms, or multi-platform viewing. Using a news consumption diary log app, the authors tracked news consumption across a variety of platforms. They report multi-platform viewing being a reality for the majority of users in their study. Long-form news videos are generally only consumed while second screening. The growing importance of short-form and social news consumption in daily life indicates a growing dominance of device-support news consumption.
Rhiu and Hwan Yun (Citation2018) investigate social media content to infer the product usefulness of smartphones. Mining tweets for user opinion and user experience, the authors categorized users’ experiences around product autonomy, adaptability, multifunctionality, connectivity, and personalization to validate user satisfaction. The authors find that increased productivity is the leading factor in user satisfaction and that device security is a major detractor from user experience.
2. Is the value of a complete path higher than the amount of personal data required to map it with respect to data privacy (i.e., beneficence)? What are the ethical parameters of path mapping to avoid exploitative conduct?
Necessary to note is that ethical data curation follows the Belmont principles and/or the guidelines of the Association of Internet researchers (Markham & Buchanan, Citation2012). The study design and curation must be reasonable, nonexploitative, and balance data extraction with benefit to society (Jordan, Citation2018). These questions address the broad ethical issues in Internet and cross-platform research.
Jordan (Citation2018) asks the readers to reconsider their concepts of reliability and validity in social media research. Strong implications exist about data veracity, representativeness, and reuse in cross-platform and mixed-method analyses. Reviewing the results of a related social network study, Jordan advocates for stronger deployment of qualitative research approaches to mitigate issues reducing veracity, while increasing the value of the research implications.
1. Challenges in mapping pathways
Living in an era with a huge variety of multiple social media networks and platforms, it is self-explanatory that the analysis of user behavior which relies only on a single social media network falls short in terms of a holistic view (Abel, Gao, Houben, & Tao, Citation2011; Lim, Lu, Chen, & Kan, Citation2015). But how can a complete social media path be mapped? What does a complete representation look like? How can we tackle these challenges without being immersive? These questions ask for an answer concerning how to frame and provide a conceptual model of a complete user pathway. Qualitative aspects like motivation and needs go hand in hand with quantitative aspects of the instantiation design (Grange, Citation2018). In this relation, we are also interested to understand what technical affordances need to be implemented to develop a single framework? This question looks for contributions on integrated and automated cross-platform analyses.
To shed light to these pressing questions, we need to express and divide the challenges into five subchallenges:
How can different platform accounts be mapped to an individual (user identification)?
Which data entities and entities in general are necessary for modeling (filtering)?
How can we analyze systematically cross-platform user-generated content (content analysis)?
Which level of data is essential to get meaningful information (granularity)?
What are additional considerations to make cross-platform analysis possible (implementation)?
It is clear that the widespread use of numerous social media accounts by individuals results in a large amount of information being available to researchers interested in society and the world at large. Whether this is seen as an individual security risk (Rose, Citation2011; Pontes et al., Citation2012) or a researcher bonanza depends on individuals’ viewpoint. Releasing the theoretically large-scale information available in these platforms for research depends on the ability to carry out analyses across multiple platforms presenting multiple views of data on different individuals in a coherent fashion. The technical challenges involved in conducting these sorts of automated mixed-method analyses across multiple platforms are numerous and varied. Many social media services operate as distinct “walled gardens” (Berners-Lee, Citation2010), seeking to keep users trapped within their pages and prevent those users’ eyeballs straying to rival services (and so away, one could cynically conclude, from competitors’ revenue generation).
Linkage between social media services (beyond those links included within user-generated content) is often restricted and limited in scope far behind what an unrestricted technology actually allows. As a concrete example of this, see the public fallout between Twitter and Facebook which leads to Instagram images no longer being visible within the Twitter timeline (Rao, Citation2012). Content that could be readily shared between networks allowing easier discoverability and access is hidden behind URLs, thus reducing the user experience and complicating the task of the researcher looking to study the application of social media across multiple networks.
Meanwhile, the initial “wave” of web 2.0 type social media services arrived with full featured and accessible APIs (Felt & Evans, Citation2008), allowing easy, automated access to the data behind their services, and further extensions of these APIs such as OpenSocial allowing platforms to share structure have been proposed. Over time, these APIs have become more restricted, closed off, and limited in their capabilities. Privacy concerns of services such as Facebook have correctly led to an inability to capture large amounts of user data without the informed consent of the user on many services. Newer social media services offer no API at all, meaning automated machine-driven access is a much larger technical challenge involving potential scraping or creating fake accounts (Tyson, Perta, Haddadi, & Seto, Citation2016), approaches which may be against the terms and conditions of the service, and so find themselves on questionable ethical grounds or incurring specific biases. Large or historical data sets cannot be easily gained by either automated API or scraping of the website, and are often only available through third-party resellers such as Gnip, OneAll, or Social Mention.
Any affordance providing the ability to perform simple and machine-driven cross-platform analysis has two potential directions to follow. The first (and most likely) direction must provide an ability to link user profile information across different services (if the user, not the content is the actor of interest in the research). This information could be accessed in different ways depending on the platform, from simple web scraping to automated API access. The second direction, which is less likely due to market forces and current entrenchment of social network providers within the web sphere, is to remove the data from the social media provider and allow individuals to be responsible for the storage and management of their own data. This “data-lake” (Walker & Alrehamy, Citation2015) approach would permit cross-platform research on users by allowing them the agency to provide access to their own data across a range or set of services.
Of course, a third pathway is simpler and on a clearer ethical footing than the automated (and nonuser involved) approaches advocated above. In this situation, users are recruited to a study as in a traditional user survey and are explicitly asked for access to (or the identity of) their account information on social-media platforms. This allows the researcher to conduct research on individual users across multiple platforms with a high level of reliability, at the potential cost of the size of the study. Examples of this approach (such as Chorley, Colombo, Allen, & Whitaker, Citation2012; Chorley, Whitaker, & Allen, Citation2015; Noë et al, Citation2016) show that users are readily willing to give access to their social media data in return for different rewards or for the perceived benefit of aiding research.
2.1. How can different platform accounts mapped to an individual (user identification)?
The challenge of automatically and computationally identifying individual users across multiple social media services is a difficult one. Users may not always use the same public identity across the range of services in which they participate. For those who do, the use of similarity matching algorithms to match duplicate users across multiple platforms has seen some success (e.g., Vosecky, Hong, & Shen, Citation2009), although of course this leaves aside the privacy issues of whether the profile information is even available for this form of analysis. Outside of newer social media services, software exists for linking user profiles between different online forums, relying on the fact that most online forums present a similar schema for user information (Nagpal, Boecking, Miller, & Dubrawski, Citation2016).
For users who do not provide the same identity across multiple platforms, the challenge of identifying and linking these profiles is much harder. Although it may be possible to assess demographic characteristics of social media users (Sloan, Morgan, Burnap, & Williams, Citation2015; Sloan et al., Citation2013), this is limited in scope and accuracy. The idea of therefore being able to use these demographics to link individuals across networks therefore seems a distant possibility. Work has been done looking at the matching of partially or fully anonymized social network data in an attempt to either de-anonymize the data, link users between the two networks (Zhang & Yu, Citation2015), or even predict or recommend links that exist in one network but not in the other (Yan, Sang, Mei, & Xu, Citation2013). Anonymization has been shown to not be effective in protecting privacy in social network data (Backstrom, Dwork, & Kleinberg, Citation2007), as there are several techniques that can identify elements of the social network even in anonymized data (Hay, Miklau, Jensen, Towsley, & Weis, Citation2010) or where only portions of the network are known (Frikken & Golle, Citation2006). Behavioral modeling has been shown to be useful in this area (Zafarani & Liu, Citation2013), providing linkage between users based upon their activity within the social network.
Users themselves often provide the best link between their identities within the varied social networks. Often profiles within one service allow links to other online services—these can be used to connect separate profiles on multiple services together with some level of reliability and trust, allowing disambiguation of users between services. For example, Wang, He, and Zhao (Citation2014) use the social media links available on Foursquare user pages to link to user profiles on both Facebook and Twitter, enabling a comparison of activity between the different social networks. Similarly, existing services such as about.me provide individuals with unique landing pages on which they are encouraged to link their identities on a number of social media services. Such links can provide disambiguation across a range of services and provide weighting to discovering additional users through the social networks linked to.
The overarching question is how to identify individual users and their corresponding multiple social media accounts. Of course, the most obvious and easiest way would be to ask them directly. But for even more obvious reasons, this might not work if the user does not want to share their account or provides inaccurate information. Xu et al. provide a different approach without too much technical effort (Xu, Lu, Compton, & Allen, Citation2014). Usually, today cross-posting is common to share identical social media messages to different social media platforms, e.g., posting a tweet on Twitter and sharing this tweet additionally on Facebook, Tumblr, and Instagram. shows such an example.
Figure 1. Basic situation explained by Xu et al. (Citation2014).
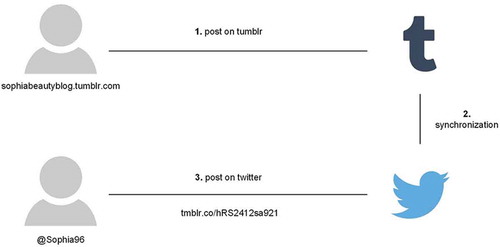
We assume that a hypothetical individual has a Twitter account called @Sophia96 and a Tumblr account called sophiabeautyblog. If there is a post on Tumblr, a software tool shares this message automatically on Twitter and synchronizes the accounts. Moreover, a provided link is transformed to a short URL to comply with Twitter’s terms of service. This link is now searchable via the Twitter search API by looking for the term “tmblr.co.” With this search, it is relatively easy to identify cross-posting of Tumblr posts to Twitter. Similar approaches are possible with other social media networks. If we have now identified two identical social media messages on different social media platforms, then we can look up for the different user account names, which provide a high possibility to identify different accounts of one individual. provides a model of the proposed procedure.
Users of the location-based social network Swarm (previously Foursquare) can choose to share their “checkins” in a given location through their accounts on Twitter or Facebook. Searching for these shared locations on a public network such as Twitter provides a sample of the data from the more closed and private social network, and has provided data sets for multiple analyses (for example Noulas, Scellato, Lambiotte, Pontil, and Mascolo (Citation2012) and Hristova, Williams, and Panzarasa (Citation2016) looking at Foursquare checkins). Silva, Vaz De Melo, O. S., Almeida, M., Salles, and Loureiro (Citation2013), which look at both Foursquare checkins and Instagram posts, use this method to come up with sets of users using either one of the services or both by locating the sharing of the content from each network through Twitter. Of course, there is also the reverse case—where sharing of content from one network to another actually hinders the research being carried out, as in Lee, Ganti, Srivatsa, and Liu (Citation2014) where the aim of identifying tweet location is made trivial where that Tweet contains information from Foursquare or Instagram.
Of course, more sophisticated (or at least more complex) methods are possible. Machine learning methods with training sets make it possible to find matches on different social media networks (Nagpal et al., Citation2016). Human verification might still be necessary because we need to ask ourselves what we are following, analyzing, or modeling: is this really a human being, an advertiser, or maybe only a social bot (Azizian, Rastegari, Ricks, & Hall, Citation2017)? This is a very important differentiation because social bots might use algorithms which determine their complete behavior, which of course is not the case for human beings (Gruzd, Citation2016).
Only a few tools exist that conduct (or enable) research on multiple platforms without requiring some in-depth technical knowledge or the creation of custom code. Tools do exist that aim to simplify and conduct multiple analyses of social media data. COSMOS (Burnap et al., Citation2015) is one such tool, allowing social media data provided either as CSV, RSS, or a collection of Twitter data files. However, as of yet it does not provide access to other social media services. SocialLab (Garaizar & Reips, Citation2014) provides an open-source Facebook clone, which can be useful for user-focused research, but does not provide for capturing of data on existing social media services.
2.2. Which data entities and entities in general are necessary for modeling (filtering)?
Filtering data is always an important step in the quantitative analysis. With respect to cross-platform analysis, we need to verify if individual user accounts are real or bots. To stick with the example, maybe the Tumblr account sophiabeautyblog uses the Twitter account @Sophia96 only for cross-posting. Despite the fact that each account maps to one individual, it is debatable if the Twitter account provides additional insight.
Of course, the provided example expects favorable conditions. If now a user is not engaged in cross-posting, this method falls short. More complex procedures like vector-based comparison algorithms or vector fields matching with fuzzy matching are necessary (Vosecky et al., Citation2009). This allows researchers to expand the level of analysis to, e.g., followers, retweets, likes, and other social media interactions. The content is key and can be analyzed by different text and data mining techniques.
A majority of social media research is focused on a single form of social media, with Twitter by far and away being the most popular (Tufekci, Citation2014). However, studies that cross platform boundaries do exist. In the main, these have relied upon individual participation and surveys in order to identify users, rather than automated approaches that seek to link users across different platforms. Skeels and Grudin (Citation2009) used a survey-based methodology to gather user opinion and usage of different social networks within the corporate environment. However, this work relied solely on self-reporting and did not examine the individual’s social network accounts. Hanson, Haridakis, Cunningham, Sharma, and Ponder (Citation2010) used a survey methodology to ascertain information about individuals’ usage of social networks and video sharing sites in relation to the 2008 US Presidential campaign. A future direction that would allow further research could be the encouragement of researchers carrying out this kind of analysis to collect User IDs or profile addresses. This may allow a more complex automated cross-platform analysis of user activity, content, and behavior, alongside the self-reported survey results.
2.3. How can we analyze systematically cross-platform user-generated content (content analysis)?
After understanding that also the content of the user-generated content needs to be analyzed, it is essential to introduce further methods. Semantic enrichment is one possible way, which has been used already successfully for cross-platform analysis (Abel et al., Citation2011). provides an example for semantic enriched tweets.
Semantic enrichment maximizes the value of tweets given the character limit of Twitter such that it might now display the complete information from the Tumblr posting. Using named-entity recognition allows us to extract meaningful information. This procedure is subdivided into three aspects: tokenizing, tagging, and chunking. gives an example for these three aspects. First, we need to identify the different parts of a sentence. Then each part is tagged and semantically enriched through part of speech, which means that every part gets classified, e.g., as adjective, verb, or noun. Finally, chunking means to assign information with different entities, e.g., organizations.
2.4. Which level of data is essential to get meaningful information (granularity)?
The result of the first three subchallenges is shown in . This is an example for different Twitter and Tumblr postings.
We can observe in this example five posts on Twitter and three posts on Tumblr. We can see that this model matches identical actions and we identify three cross-postings. The broken red line represents the five actions of user 1. Users 2, 3, and 4 retweet at different stages and services the postings of user 1. But this is more a bird’s eye view perspective. If we zoom in a different view is possible, which can be seen in .
Now we can analyze the diffusion of a particular post. In this case, a reblog–retweet network can be used to analyze different user accounts of one individual. An initial posting at Tumblr turns to a cross-posting. Each other user (user 1, user 6, other user, and original poster) can be a part of a reblog–retweet network. For example, user 1 reblogs an article which points to user 6, who also is just retweeting something from other user, until we can finally point to the original poster. If we are interested in communities, then we have to zoom again out. depicts this.
shows the different parts to construct a possible complete user pathway. In these simplified examples, retweeting, explicit mentions, and reblogging are the methods to identify an individual with accounts on different social media services.
2.5. What are additional considerations to make cross-platform analysis possible (implementation)?
Further considerations are necessary to be taken in the context of the implementation of a successful cross-platform analysis. Maybe one of the easier questions to answer is, if someone wants to perform a real-time analysis or a post-analysis. In favor of a real-time analysis is the aspect of being up-to-date. This might be, e.g., interesting for emergency management, where up-to-date data are important (Hughes & Palen, Citation2014), e.g., if it is necessary to verify the credibility of a user. On the other hand, a not time critical analysis can be conducted later, where accuracy might be more of an issue.
Last but not least, privacy and ethical aspects are important as well. These aspects will be discussed in more detail in section 4.
3. Bias in social media
The idea of bias in social media research is not new, but there are a surprisingly small number of studies that actively address bias when compared to studies that analyze social media in some way. When a study does address bias, it is more often than not specific to one platform, which is problematic for two reasons. First, the very nature of social media research is as diverse as the platforms themselves. When solutions to biases in social media research are offered, it is often not clear how they could be applied in a general sense to different platforms. Second, and as discussed in this section, biases can be exacerbated in cross-platform studies; different biases may be in place or coalesce; a specific type of bias may manifest itself in different ways across different platforms; the undertaking of a cross-platform study may itself result in certain types of bias (especially in sampling, samples, data availability, information asymmetry, non-intersecting samples, etc.). As already noted, there is a disparity of attention that platforms receive (Tufekci, Citation2014) with Twitter receiving the bulk of attention in both “normal” studies and those that investigate bias. This too is problematic, given the user base of Twitter—it is neither generally representative (Ruths & Pfeffer, Citation2014) nor does it describe a singular geographic group.
In this section, we briefly outline aspects of bias that single- as well as cross-platform studies need to be aware of. However, more importantly, we call for more studies that directly, reproducibly, and tangibly provide mechanisms to handle: data availability, sampling bias, self-selection bias, self-representation, the bias variance trade-off, effects of homophily and cliques, compounding effects of online social media platforms especially in cross-platform scenarios, and finally content biases. While much of the discussion here reflects on single-platform studies (largely due to the lack of cross-platform studies of bias), many of the challenges discussed will be inflated, compounded, and/or exacerbated in cross-platform settings.
3.1. What is the impact of data (non-)availability in social media studies?
The start of any social media study is the data acquisition phase. It is here that many of the biases discussed below begin to materialize, through the ability and permission to access data. Many platforms place limitations on the amount of data the researchers can access, or how often they can request data over a defined time period. For example, Twitter offers 1% or 10% access to its streaming API. Platforms like Facebook, Instagram, and Reddit, which offer APIs for historical access to data, limit the number of requests that can be made to their APIs over a predefined time period. Platforms like LinkedIn, which do not explicitly offer APIs for data collection in the same manner as other platforms, mean that researchers have to web crawl their platform of choice. This has issues with respect to honoring the terms and conditions of platforms as well as implications for crawler politeness (Koster, Citation1995). Invariably, some studies circumvent methods established to prevent (or hinder) the platform being crawled. In all scenarios, the rate at which researchers can gather data is impeded, and thus more time and/or effort is required to curate a data set of an appropriate size. Unfortunately, many of the platforms do not disclose how their sampling methods work (if in place) or when they change, thus leaving researchers in the dark in reference to the completeness or representativeness of their data.
Aside from representability of platforms and the samples they can provide, Wang et al. (Citation2014) note that different security and privacy policies implemented across platforms also correspond to information asymmetriesFootnote2 hindering researchers from investigating different user contexts and scenarios. The authors also note that these information asymmetries also hinder the ability to remove or even bound error in cross-platform studies. Similarly, data are often incomplete or not provided in their raw form which further limits analyses, and cross-platform or participant group studies (Pedrana et al., Citation2013).
No discussion on bias would be complete without at least mentioning researcher bias in the sense of availability of data. Ruths and Pfeffer (Citation2014) note that there is a huge disparity within the social media research community with the notion of “embedded researchers” who have elevated access to platform data, a principal example here being the Facebook contagion studies (Kramer, Guillory, & Hancock, Citation2014). Such studies are not bad per se (aside from any ethical concerns) as they provide excellent insights into the platforms they study. Yet, the unprecedented access to data often results in redacted components or corrections to the data that are not or cannot be revealed. Consequently, these studies cannot be replicated, and their findings and methods are often not transferable to smaller scale studies. In the context of cross-platform studies, the notion of an “embedded researcher” disintegrates, they are only embedded in one platform, and as such the data they have access to would completely overshadow those of another platform in the sense of having significantly more data for one platform than another.
3.2. Are the populations representative?
Probably the most common bias that researchers face is in reference to demographic samples, typically surrounding age, gender, nationality, or education level. Gayo-Avello (Citation2011) forensically dissected a series of prediction tasks (elections) outlining how these biases impede prediction accuracy. Typically, sampling biases occur because social media studies are convenience samples (Malthouse, Haenlein, Skiera, Wege, & Zhang, Citation2013), be it of Fortune 500 companies (Kim, Kim, & Nam, Citation2014), seeking specific participant properties (Gudelunas, Citation2012) (Ruehl & Ingenhoff, Citation2015), “popular” content (Lerman & Ghosh, Citation2010), crowd workers (Hall & Caton, Citation2017; Oh & Syn, Citation2015), and/or simply due to the technical challenges of extracting data. These samples may in fact often be large, but estimates and conclusions made from a biased sampling procedure have uncertain usefulness (Malthouse et al., Citation2013). Many studies are also affected by a social desirability bias, be it self-representation, self-selection, observer biases, etc. The overarching effect of sampling biases is the generalizability and reproducibility of findings. This is even more the case in cross-platform studies, if users across the platforms involved cannot be interlaced or related in some manner (Oh & Syn, Citation2015). Mejova and Srinivasan (Citation2012) go slightly further and note that the choice of platform can change the results (or their interpretation) of a study.
There have been a number of useful studies that can assist researchers in the assessment of their samples. Duggan and Brenner (Citation2013) provide a demographic overview of social media (Facebook, Twitter, Instagram, Pinterest, and Tumblr) across the USA. Similarly, Bolton et al. (Citation2013) seek to understand the Generation Y and Millennial demographic with respect to their relationship with social media. We do note, however, that these papers, as well as similar papers (e.g., Mislove, Lehmann, Ahn, Onnela, and Rosenquist, Citation2011; Morstatter, Pfeffer, and Liu, Citation2014; Tufekci, Citation2014), focus on a subset of the available demographic and thus provide research insights on the appropriateness of their samples in certain scenarios, but are not all encapsulating. It is also worth noting that there is no consensus on the definition of inclusion criteria of platforms or users in studies (Panek, Nardis, & Konrath, Citation2013).
There have been studies on the effects of different sampling approaches (e.g., De Choudhury et al., Citation2010; González-Bailón et al., Citation2014) with the objective to better understand sample biases and their consequences. De Choudhury et al. (Citation2010) interestingly comment that their own study on sample bias may itself be biased by sample size. Culotta (Citation2014) reports some success in handling sampling biases by reweighting through inferred demographic properties of the sample (to account for class imbalance(s)). Hughes, Rowe, Batey, and Lee (Citation2012) performing a comparative study of Twitter vs. Facebook also note that researchers need to be wary of their sampling methods, noting that the (relatively common) application of snowball sampling results in the overrepresentation of specific demographics. Similarly, the need for participants to actively use and engage with multiple platforms further exacerbates any effects of incurred sampling biases (Correa, Hinsley, & De Zuniga, Citation2010; Ruths & Pfeffer, Citation2014; Wang et al., Citation2014). In general, however, it is hard to determine how generalizable social media findings are, yet authors often claim generalizability from one platform to another (Panek et al., Citation2013).
There are also avenues open to researchers to at least partially mitigate these effects at the design and/or data collection stage. Ruths and Pfeffer (Citation2014) provide a checklist to researchers to ensure that they at least check for potential biases in the data collection or analysis phases. There are also papers that approach (cross-platform or otherwise) such situations through additional data collection. Lee et al. (Citation2014) is one such example, demonstrating partially overcoming the absence of twitter geo-tagged tweets (critical in this case for a location-based study) by inspecting various aspects of the tweet itself and often capturing content from other platforms such as Foursquare and/or Instagram. This permitted the researchers to overcome a sample bias (imbalanced location representation) caused via missing information.
Referred to as a “thorny” problem for social media researchers (Aral, Dellarocas, & Godes, Citation2013), self-selection bias is endemic in social media. Many studies either recruit or attempt to unobtrusively observe users of the social media platforms. However, such is the nature of social media platforms that the set of users that are active and visible on the platform(s) affects the availability of data for researchers to analyze. Correspondingly, elite biases and power-law properties increase the propensity for sampling, self-selection, and majority biases in social media studies.
Aside from the technical hurdles of accessing social media data and curating a sample that abides by some definition of “representative” in the context of the platform(s) in use, there are minimum technological and economic requirements to access and engage with social media (Corley, Cook, Mikler, & Singh, Citation2010)—even more so in studies of niche or minority users (e.g., Baker, Bricout, Moon, Coughlan, and Pater, Citation2013). Despite Facebook’s recent announcement of having reached two billion users worldwide,Footnote3 many of these users belong to specific geographic regions, are generally literate and educated, have access to the Internet, and have a certain minimum socioeconomic status. Similarly, many social media platforms require their users to be of a minimum age, typically, 13. Critically, different platforms are more prevalent in some countries than others, Facebook and Twitter have been blocked in China since 2009, and platforms like Sina Weibo and VK address specific demographic groups further exacerbating potential sampling biases. Also noteworthy is the prevalence of English across social media platforms, followed by Japanese, Portuguese, Spanish, Indonesian, Dutch, and Malay (Baldwin, Cook, Lui, MacKinlay, & Wang, Citation2013).
While we do not advocate specific sampling methods over others, as often researchers may not be in a position to “improve” their samples or sampling methods. It is important that social media researchers are aware that sample biases can materialize in a variety of ways. In cross-platform studies, there may be different sampling biases in place that require more careful consideration than isolated cases within single-platform studies.
3.3. Is there a difference between real life and online data?
Once researchers have curated their data, it can be difficult to penetrate the “real” profile of the users they observe. Correspondingly, any disparity between the real user and the virtual user means that any research the user is “involved” in is biased to some extent (Hall & Caton, Citation2017). As an endeavor to understand the differences in self-promotion across different platforms, van Dijck (Citation2013) juxtaposes Facebook and LinkedIn identifying that different contexts affect how the platforms are used by users to curate their own digital identity—thus indicating that self-representation is not an isolated issue, but specific to the platform or platforms of inquiry.
A common issue, for many studies, is in interpreting self-reported measures that try to mitigate or at least contextualize self-representation. In such cases, any predictor and/or outcome variable may incur a method bias, serving to inflate model parameter estimates (Hughes et al., Citation2012). This is not a new problem; problems such as central tendency and acquiescence bias are well known in the context of self-reporting measures (Krosnick, Citation1999; Schwarz, Hippler, Deutsch, & Strack, Citation1985) and usually categorized as method biases. There are several possibilities to counteract the effects of method bias, which are extensively discussed by Podsakoff, MacKenzie, Lee, and Podsakoff (Citation2003). Specific to social media research, Ruths and Pfeffer (Citation2014) and González-Bailón et al. (Citation2014) outline other issues of methods that can bias studies across one or multiple platforms.
Other resources that are garnering attention are the use of surveys capturing the use of social media coupled with data drawn from respondents’ profiles (e.g., Ellison, Steinfield, and Lampe, Citation2007; Ewig, Citation2011; Steinfield, Ellison, and Lampe, Citation2008). Such approaches are typically used to identify inaccuracies (intentional or otherwise) in self-reported measures. Yet, when used in isolation, they foster an overreliance on passive usage variables representing an oversimplified view of user behavior (Davenport et al., Citation2014). Giglietto et al. (Citation2012) specifically note the advantages of mixed-method study designs where more traditional social science methods work alongside those of computer science, but also call for caution, careful design, and interdisciplinary collaboration.
The effects of self-representation can also manifest themselves in other interesting ways. As noted by Muntinga, Moorman, and Smit (Citation2011) when researchers wish to understand perceptions and/or motivations of social media users (in this case with respect to products and brands), the act of social posturing significantly complicates the research process. They also note the potential for compounding effects in interview or survey scenarios, depending on the level of anonymity (of both researchers and participants) and how prescribed or transparent the objectives of the study are.
Similar biases can occur in the context of public figures, politicians, celebrities, and organizations with professionally managed accounts (Azizian, Rastegari, Ricks, & Hall, Citation2017; Ruths & Pfeffer, Citation2014). Managed accounts have masquerading elements similar to that of self-representation, but which need to be handled differently. Some platforms, where Facebook is a key example, do sometimes list the account admins, as well as who authored a post. However, this is usually largely dependent upon the administration and the associated privacy settings. Thus, researchers may need to exercise care in content-based studies where multiple authors could be present.
3.4. Which structural aspects of bias may be impacting social media research?
Users often follow homophilous responses to their online relationships, ties, or links within social graphs (McPherson, Smith-Lovin, & Cook, Citation2001). However, in the absence of good metadata (or even a sufficient number of observations) pertaining to the “conditions” of these links, the presence of cliques can bias the quantitative models of researchers. Compelling examples are noted by Gilbert and Karahalios (Citation2009) who investigate relationship strength and ties on Facebook, and Cranshaw, Hong, and Sadeh (Citation2012) who study cross-platform (Twitter and Foursquare) checkin behaviors to understand the dynamics of city life. Gilbert and Karahalios (Citation2009) note the presence (and use of) of cliques in the modeling process means that models can result in false positives in prediction settings. Cranshaw et al. (Citation2012) similarly note that “majority” behaviors will crowd out or misrepresent minority behaviors. Consequently, cliques can correspond to echo chamber effects as well as imbalances in the data, i.e., where one category of users dominates all other users, and thus centering the data around one (or few) specific user type(s). The resultant bias is that the presence of such effects may disproportionately overemphasize the commonality of any effects observed within cliques or underemphaszse effects observed (if at all detected) outside cliques. However, it is noteworthy that effects such as these may also be ideal circumstances depending on the research design, and thus not constitute a bias at all.
3.5. Do confounding effects of online mediums exist?
All users have multifaceted online and offline relationships. Aside from the self-representation bias, researchers also need to be cognizant that the context of use for users can confound their models. For cross-platform studies, this creates additional challenges, as researchers are often not aware of exogenous activities outside their lens of view. For example, if two users are connected on Facebook, LinkedIn, and/or follow each other on Twitter, but also interact offline researchers suffer (mostly unknowingly) from context loss. The absence of this context (and multifaceted view of a relationship), as noted by Gilbert and Karahalios (Citation2009), means it is hard to imagine a system that could ever (or should ever) pick a rich view of the phenomena or interactions being observed. Consequently, any conclusions drawn may be polarized. That said, Vosecky et al. (Citation2009, Zhang, Kong, and Philip (Citation2013), Qi, Aggarwal, and Huang (Citation2013, and Nagpal et al. (Citation2016) have all approached addressing the object identification, linking, and entity resolution problems in cross-platform scenarios, which is a significant step toward alerting researchers of potential multifaceted relationships as well as linking them across platforms. Yet, as users also vie for increasingly more “friends” or followers this results in a disparity of relationship types, which in the absence of meaningful metadataFootnote4 create interpretational challenges (Caton et al., Citation2012; Sorensen, Citation2009). That is not to say that researchers have not made progress delineating different relationship types across multiple platforms by looking at the number of interactions (e.g., Pappalardo, Rossetti, and Pedreschi, Citation2012), and more generally also the structure of these interactions (Gilbert & Karahalios, Citation2009). However, the latter required fairly invasive permissions for access profile data.
Social media is often leveraged for information of the now, but when used historically it (as has been discussed) can be difficult to access representative data. Yet, the type information users wish to share on different platforms also needs to be considered (Cranshaw et al., Citation2012; Poell & Borra, Citation2012). As noted by Quan-Haase and Young (Citation2010), users do not completely replace one form of social media with another; different platforms support unique needs that another potentially cannot completely fulfill. Many of the consequences of these effects go unnoticed, but they may have important theoretical implications depending on the question being asked (boyd & Crawford, Citation2011). It is generally understood that social media data should be seen as communicative data, i.e., data produced as a side effect of some communicative act between users. Any interactions captured within the data are not always representative of a “reality” beyond an overarching desire to be a part of something (boyd & Crawford, Citation2011; Caton et al., Citation2012; González-Bailón et al., Citation2014; Rost, Barkhuus, Cramer, & Brown, Citation2013). This is not just isolated to individual users, but also industry uses of social media are also aligned with their industry sector, reach aspirations, and engagement opportunities (Kim et al., Citation2014; Ruehl & Ingenhoff, Citation2015).
3.6. How does content bias impact social media research?
In the era of fake news, echo chambers, and polarized information spaces, social media studies are now becoming aware of biased content (Hobbs et al., Citation2017; Lazer et al., Citation2017). Saez-Trumper, Castillo, and Lalmas (Citation2013) report on the results of a cross-platform study on the permeation of news stories for online news and the social media communities that surround them. They identify a variety of biases aligned with how news agencies or platform users share news. They outline an approach to observe selection (which stories are selected), coverage (how much attention stories receive), and statement (how the story is reported) biases. This has strong practical implications as well, considering the magnitude of the influence campaign created by Russia in the 2016 (and beyond) US elections. Fake news and echo chambers created a scenario that allowed motivated citizens to cross not only platforms, but turn their social media browsing into real-life actions (Balmas, Citation2014; Silverman & Singer-Vine, Citation2016). Another study by Lumezanu, Feamster, and Klein (Citation2012) also investigates the proliferation of propaganda on Twitter, highlighting the importance for researchers to consider content biases in their work. A similar study conducted with Facebook data by Schmidt et al. (Citation2017) goes to further illustrate that social media researchers need to be especially careful when considering content consumption in their models. This sentiment is mirrored in Bessi et al. (Citation2016) who investigate and report on confirmation biases and content polarization within the scope of echo chambers across Facebook and YouTube.
Spam content has been reported in the context of Twitter (Kwak, Lee, Park, & Moon, Citation2010) and (public) Facebook data (Caton et al., Citation2015). Yet, dealing with this kind of content is still nontrivial. It should also be noted that it is very difficult to ascertain whether spam content significantly affects studies, especially if spam content is significant in size. Saez-Trumper et al. (Citation2013) also note that mechanisms of identifying and blacklisting accounts are necessary to not overrepresent specific information. However, Ruths and Pfeffer (Citation2014) note that it is currently impossible to accurately remove or correct for the vast majority of spam accounts from studies. Closely, related to spam is noise in social media content. Yet methods to cleanse or denoise social media data exist (Baldwin et al., Citation2013).
We can also articulate similar concerns in the context of content censorship. Bamman et al. (Citation2012) conducted a cross-platform study (Twitter and Sina Weibo), investigating how censorship influences social media behavior. Censorship here refers to both formal censorship (Twitter is IP blocked) and self-censorship: users deleting comments. They note two interesting biases: APIs limit the number of requests for deleted content thus resulting in a sample bias, and a locale bias (users within the IP block vs. those circumventing it).
Content biases can also arise from the transitivity of content (Grange, Citation2018; Lowenstein & Lev-On, Citation2018). As noted in Smith, Fischer, and Yongjian (Citation2012), social media platforms delete data that are made available to search engines over time. Thus, historical studies that leverage search platforms to sample publically available user-generated content have no way of knowing if or to what extent this practice biases the content collected. The authors note this impedes extrapolation of their results beyond the sample collected, and that scholars should do so with extreme caution. Deletion can also occur due to copyright violations or poster prerogative (Thorson et al., Citation2013), which can also cause significant content biases in cross-platform studies; consider a tweet with a link to a removed YouTube video.
3.7. What is the correct bias-variance trade-off?
There are an increasing number of studies relying on machine and statistical learning methods. However, such approaches have specific requirements for the data used to build or train models (in the context of prediction, forecasting or supervised machine learning scenarios) to model interpretability (in explorative or unsupervised machine learning scenarios). Typically, studies that attempt to predict the results of elections or public opinion as expressed via social media can fall foul of these requirements. In brief, the bias variance trade-off is a problem of trying to minimize the effect of two different sources of error in a model—bias is the error stemming from erroneous assumptions in the learning process (underfitting the data) and variance error stemming from over emphasizing small components of the data (overfitting the data). While there are many methods to minimize the effects of this trade-off (regularization, loss/cost functions, feature selection, boosting, bagging, etc.), the idiosyncrasies of social media data require increased methodological fluency for researchers. Data are often significantly skewed, incomplete, or, as already discussed above, biased in a variety of ways.
Notwithstanding method challenges, social media data are often not appropriately labeled for supervised machine learning methods, as noted by Becker, Naaman, and Gravano (Citation2010). While the community has started to release labeled data sets for social media on platforms such as Kaggle, CrowdFlower, and others, there are still broad questions regarding the transferability and generalizability of any machine learning models trained on data from one platform, and applied to data from another.
4. Ethical questions regarding cross-platform data collection
Pollsters, politicians, and marketers alike have long histories of collating data from myriad sources to increase return on investment (ROI) (Malinen, Citation2015; Phethean et al., Citation2015; Schacht et al., Citation2015). More recently, citizen journalists and scientists are referencing across platforms as well (Starbird, Muzny, & Palen, Citation2012). This goes for e-businesses as well. In the aftermath of the dual Russian election hacking and Cambridge Analytica scandals, one particular revelation shocked and roused the #deleteFacebook movement. Namely, since 2014 certain Android system users have (unwittingly) granted Facebook’s app permission to store phone data, including detailed phone records, such as dates, times, call lengths, call recipients, and phone numbers.Footnote5 The use of these data further supports Facebook’s business model and value proposition, including seamless connectivity with and across platforms and people. However, in the face of Facebook’s position in the 2016 social media influence campaign, this is cross-platform data harvesting is widely recognized as violating an ethical norm. This is because while the definitions of ethical Internet research do not change when more than one platform is considered, the stakes of the analyses raise.
There are ethical boundaries in any analyses of social data (Hall, Citation2018). The domain is broad and currently based on guidelines, thus under-regulated. The use of multiple platforms only increases the potential for ethical issues, and thus research design must be treated carefully. This section points out various points for concern, as cross-platform ethics has at the point of publication not been treated by literature to the extent of our knowledge. The guidelines and causes of concern in research design have been well pointed out for single platforms and methods by studies and commentaries such as found in Eysenbach and Till (Citation2001), Grimmelmann (Citation2015), Schroeder (Citation2014), and Zimmer (Citation2010). Moreno, Goniu, Moreno, and Diekema (Citation2013) note the importance of confidentiality and beneficence in social media research. Particularly, these aspects are under-addressed in literature regarding cross-platform studies. With the presence of more personally identifying information as is available in a cross-platform design, the chance of incidental confidentiality breaches is higher.
According to current research ethics guidelines specific to social media research, informed consent is required if the analysis goes beyond passive review of (publicly) posted items (i.e., is more than observational in nature). Both Twitter and Facebook have set in their terms and conditions of language allowing for the anonymized assessment of data, stating that use of the platforms (i.e., interactions, posts, and other actions) is in fact in agreement with anonymized, public analyses of individuals’ data. In the ethical fallout surrounding the emotional contagion study (Kramer et al., Citation2014), Facebook specifically argued that use is tantamount to informed consent; this is a common position across the major social media platforms. Because the traditional lines between public and private have changed since the advent of social media sites, this stance has been soundly rejected by some leading researchers in the domain (boyd & Crawford, Citation2011). In a cross-platform scenario, it should be inferred that consent for each platform under observation is required: one general blanket accept does not sufficiently inform research participants of the data being gathered.
While standards may be evolving, the current status is that publicly posted data have implicit informed consent attached (Jordan, Citation2018; Zimmer, Citation2010). However, in the case of actively recruited participants or apps that take data outside of normal personal privacy settings, informed consent must continue to be in place as the standards of human subject research demand. Still, due to the nature of the data and its harvesting, the line between observational and interactive research is thin. In their work on qualitative research across social media, Eysenbach and Till (Citation2001, 1105) pose a useful series of questions with regard to the need for informed consent in social media studies:
Intrusiveness—Discuss to what degree the research conducted is intrusive (“passive” analysis of internet postings versus active involvement in the community by participating in communications)
Perceived privacy—Discuss (preferably in consultation with members of the community) the level of perceived privacy of the community (Is it a closed group requiring registration? What is the membership size? What are the group norms?)
Vulnerability—Discuss how vulnerable the community is: for example, a mailing list for victims of sexual abuse or AIDS patients will be a highly vulnerable community
Potential harm—As a result of the above considerations, discuss whether the intrusion of the researcher or publication of results has the potential to harm individuals or the community as a whole
Informed consent—Discuss whether informed consent is required or can be waived (If it is required how will it be obtained?)
Confidentiality—How can the anonymity of participants be protected (if verbatim quotes are given originators can be identified easily using search engines, thus informed consent is always required)
Intellectual property rights—In some cases, participants may not seek anonymity, but publicity, so that use of postings without attribution may not be appropriate.
The questions and statements posed by the authors carefully follow the Belmont principles, thereby tailoring the concepts to the Internet age. The Belmont report outlines basic ethical principles for the protection of human subjects in research projects, specifying that ethical research “maximize[s] possible benefits and minimize[s] possible harms” (National Commission for the Protection of Human Subjects of Biomedical and Behavioral Research, 1979) to all persons and in data collection.
Closely related to issues of informed consent is the relationship between social media research and the Belmont principles, the standard of human subjects research. The suggested guidelines of Eysenbach and Till (Citation2001) are instructive on the appropriateness of the research design. Studies should carefully consider the intrusiveness, individuals’ perceived privacy of the forum and required confidentiality of the individuals, whether the group contains vulnerable individuals or whether the research questions being investigated can otherwise pose potential harms to the group, and any explicit informed consent or intellectual property rights at hand.
Several ethical drawbacks of social media research have been delineated. Social media data are vast, granular, and have lower access barriers than standard social science approaches. In our increasingly digital lifestyle, social media data re-create a play by play of individuals’ lives. This enables analysis at a scale previously unimagined. However, the chances of inadvertent exposure in cross-platform studies are much higher due in part to the much higher granularity of data per individual that is available. The fundamental concept of ethical research, maximizing benefit to all and minimizing harm to each, is indeed even more prominent considering the amount and granularity of available data from cross-platform studies.
5. Conclusion and research directions
Social media and the digital lifestyle are here to stay. While there is a considerable body of work focusing on single attributes, phenomena, and platforms, works which span boundaries are scarce. We ignore the reality and significance of people moving between and across platforms at the detriment of the domain of research. This overview introduces four new studies on cross-platform, mixed-method social media analysis (Grange, Citation2018; Jordan, Citation2018; Kazanidis et al., Citation2018; Lowenstein & Lev-On, Citation2018; Rhiu & Hwan Yun, Citation2018). These articles discuss pathway mapping between platforms and the trade-offs between completeness and excessiveness. The article further discusses the state of the art and challenges associated with the technical construction of a multi-platform analysis, and the potential of multi-modal analysis to address bias in social media research. We conclude with an overview of the ethics of such studies. Scholars should be encouraged to consider further applications such as health studies, marketing, and politics in their research; further addressing of bias in social media data; and a standard of unified ethics in multi-modal analysis. Of particular interest to future scholars is the combination of differing data formats (i.e., text and image) as well as the theoretical underpinnings of platform modality and choice. Immense research and practical value is available for future cross-platform, mixed-method scientists.
Additional information
Notes on contributors
Margeret Hall
Margeret Hall is an Assistant Professor of IT Innovation at the University of Nebraska at Omaha. She received her PhD in Industrial Engineering focusing on Social Computing from the Karlsruhe Institute of Technology. Her research investigates the integration of the digital lifestyle using a combination of quantitative and qualitative approaches.
Athanasios Mazarakis
Athanasios Mazarakis is a postdoctoral researcher for Web Science at Kiel University, Germany. He received his PhD in Economics with a focus in Incentives and Web 2.0 from the Karlsruhe Institute of Technology. In Kiel his research is about Gamification, Open Science, Social Media and Altmetrics.
Martin Chorley
Martin Chorley is a Lecturer in the School of Computer Science & Informatics at Cardiff University. His research interests are focused on computational data journalism, social networks and social media, and the interaction of people and systems.
Simon Caton
Simon Caton is a Lecturer of Data Analytics at the National College of Ireland. He researches the computational aspects of analyzing multiple social media platforms simultaneously, as well as the facilitation of data analytics platforms through parallel and distributed computing methods. He reviews for several international conferences and journals.
Notes
2. In this context, information asymmetry corresponds to a disparity of information available for a given research context and/or question. An example for a cross-platform study is user location. Consider using both Facebook and Twitter. Facebook typically encodes the home town/city, last registered location, or current place of residence, whereas Twitter users seldom provide (accurate) location information, as it is optional profile information. Similarly, where Twitter profile information is public, Facebook profile information is not (with the exception of Facebook ID, username, and name) unless the account is a public one (where celebrities and politicians are notable examples here). As such, despite certain Facebook entities being publically accessible (e.g., pages, locations, public groups, etc.) a smaller portion of the user profile is accessible when a user falls under the lens of a researcher.
3. https://www.facebook.com/zuck/posts/10103831654565331?pnref = story - last accessed Nov. 2017.
4. It is very difficult to distinguish the type of relationship, and many platforms do not represent (or make available) relationship tags or types. Platforms such as Facebook which permit users to identify relationship groupings (e.g., friends, family, acquaintances, colleagues, etc.) require a user to authorize access to this data (and quite rightly too).
5. https://mashable.com/2018/03/25/facebook-android-phone-call-data-gathering/#pS5dhYe.usqC.
References
- Abel, F., Gao, Q., Houben, G.-J., & Tao, K. (2011). Semantic enrichment of Twitter posts for user profile construction on the social web. In G. Antoniou, M. Grobelnik, E. Simperl, B. Parsia, D. Plexousakis, P. De Leenheer, & J. Pan ( Hrsg.), Proceedings of the 8th Extended Semantic Web Conference (pp. 375–389). Berlin, Germany: Springer Berlin/Heidelberg.
- Aral, S., Dellarocas, C., & Godes, D. (2013). Introduction to the special issue—Social media and business transformation: A framework for research. Information Systems Research, 24(1), 3–13. doi:10.1287/isre.1120.0470
- Azizian, S., Rastegari, E., Ricks, B., & Hall, M. (2017). Identifying personal messages: a step towards product/service review and opinion mining. In International Conference on Computational Science and Computational Intelligence.
- Backstrom, L., Dwork, C., & Kleinberg, J. (2007). Wherefore art thou r3579x? Anonymized social networks, hidden patterns, and structural steganography. Proceedings of the 16th International Conference on World Wide Web - WWW ’07, 54(12), 181. doi:http://doi.org/10.1145/1242572.1242598
- Baker, P. M., Bricout, J. C., Moon, N. W., Coughlan, B., & Pater, J. (2013). Communities of participation: A comparison of disability and aging identified groups on Facebook and LinkedIn. Telematics and Informatics, 30(1), 22–34. doi:10.1016/j.tele.2012.03.004
- Baldwin, T., Cook, P., Lui, M., MacKinlay, A., & Wang, L. (2013, October). How noisy social media text, how different social media sources? In IJCNLP (pp. 356–364). Nagoya, Japan.
- Balmas, M. (2014). When fake news becomes real: Combined exposure to multiple news sources and political attitudes of inefficacy, alienation, and cynicism. Communication Research, 41(3), 430–454. doi:10.1177/0093650212453600
- Bamman, D., O'Connor, B., & Smith, N. (2012). Censorship and deletion practices in Chinese social media. First Monday, 17, 3.
- Becker, H., Naaman, M., & Gravano, L., 2010, February. Learning similarity metrics for event identification in social media. In Proceedings of the third ACM international conference on Web search and data mining (pp. 291–300). ACM.
- Berners-Lee, T. (2010). Long live the web. Scientific American, 303(6), 80–85. doi:10.1038/scientificamerican1210-80
- Bessi, A., Zollo, F., Del Vicario, M., Puliga, M., Scala, A., Caldarelli, G., … Preis, T. (2016). Users polarization on Facebook and YouTube. PloS One, 11(8), e0159641. doi:10.1371/journal.pone.0159641
- Bloom, R., Amber, K. T., Hu, S., & Kirsner, R. (2015). Google search trends and skin cancer: Evaluating the US population’s interest in skin cancer and its association with melanoma outcomes. JAMA Dermatology, 151(8), 903–905. doi:10.1001/jamadermatol.2015.12
- Bolton, R. N., Parasuraman, A., Hoefnagels, A., Migchels, N., Kabadayi, S., Gruber, T., … Solnet, D. (2013). Understanding generation Y and their use of social media: A review and research agenda. Journal of Service Management, 24(3), 245–267. doi:10.1108/09564231311326987
- boyd, d., & Crawford, K. (2011, September 21). Six provocations for big data. In A decade in internet time: Symposium on the dynamics of the internet and society (Vol. 21). Oxford: Oxford Internet Institute.
- Burnap, P., Rana, O., Williams, M., Housley, W., Edwards, A., Morgan, J., … Conejero, J. (2015). COSMOS: Towards an integrated and scalable service for analysing social media on demand. International Journal of Parallel, Emergent and Distributed Systems, 30(2), 80–100. http://doi.org/10.1080/17445760.2014.902057
- Caton, S., Dukat, C., Grenz, T., Haas, C., Pfadenhauer, M., & Weinhardt, C., 2012, November. Foundations of trust: Contextualising trust in social clouds. In Cloud and Green Computing (CGC), 2012 Second International Conference on (pp. 424–429). IEEE.
- Caton, S., Hall, M., & Weinhardt, C. (2015). How do politicians use Facebook? An applied social observatory. Big Data & Society, 2(2), 2053951715612822. doi:10.1177/2053951715612822
- Chorley, M. J., Colombo, G. B., Allen, S. M., & Whitaker, R. M. (2012). Better the Tweeter you know: Social signals on Twitter. In 2012 ASE/IEEE international conference on social computing (pp. 277–282). Amsterdam, The Netherlands: ASE/IEEE International Conference on Social Computing. doi:http://doi.org/10.1109/SocialCom-PASSAT.2012.27
- Chorley, M. J., Whitaker, R. M., & Allen, S. M. (2015). Personality and location-based social networks. Computers in Human Behavior, 46, 45–56. doi:10.1016/j.chb.2014.12.038
- Chorley, M. J., & Williams, M. J. (2017). Foursquare. In L. Sloan & A. Quan-Haase (Eds.), The SAGE handbook of social media research methods (pp. 610–626). London, UK: SAGE.
- Chung, J., & Mustafaraj, E. (2011). Can collective sentiment expressed on twitter predict political elections? In Proceedings of the Twenty-Fifth AAAI Conference on Artificial Intelligence (pp. 1770–1771). San Francisco, CA. https://doi.org/10.1007/s00247-002-0848-7
- Cioffi-Revilla, C. (2010). Computational social science. Wiley Interdisciplinary Reviews: Computational Statistics, 2(3), 259–271. doi:https://doi.org/10.1002/wics.95
- Corley, C. D., Cook, D. J., Mikler, A. R., & Singh, K. P. (2010). Text and structural data mining of influenza mentions in web and social media. International Journal of Environmental Research and Public Health, 7(2), 596–615. doi:10.3390/ijerph7020596
- Correa, T., Hinsley, A. W., & De Zuniga, H. G. (2010). Who interacts on the Web?: The intersection of users’ personality and social media use. Computers in Human Behavior, 26(2), 247–253. doi:10.1016/j.chb.2009.09.003
- Cranshaw, J., Hong, J. I., & Sadeh, N. (2012). The livehoods project: utilizing social media to understand the dynamics of a city. Icwsm (pp. 58–65). Dublin.
- Culotta, A., 2014. Reducing sampling bias in social media data for county health inference. In Joint Statistical Meetings Proceedings (pp. 1–12).
- Davenport, S. W., Bergman, S. M., Bergman, J. Z., & Fearrington, M. E. (2014). Twitter versus Facebook: Exploring the role of narcissism in the motives and usage of different social media platforms. Computers in Human Behavior, 32, 212–220. doi:10.1016/j.chb.2013.12.011
- De Choudhury, M., Lin, Y. R., Sundaram, H., Candan, K. S., Xie, L., & Kelliher, A. (2010). How does the data sampling strategy impact the discovery of information diffusion in social media? ICWSM, 10, 34–41.
- Duggan, M., & Brenner, J. (2013). The demographics of social media users, 2012 (Vol. 14). Washington, DC: Pew Research Center’s Internet & American Life Project.
- Ellison, N. B., Steinfield, C., & Lampe, C. (2007). The benefits of Facebook “friends:” Social capital and college students’ use of online social network sites. Journal of Computer‐Mediated Communication, 12(4), 1143–1168. doi:10.1111/jcmc.2007.12.issue-4
- Ewig, C. (2011). Social Media: Theorie und praxis digitaler sozialität/social media: Theory and practice of digital sociality. In Social media: theorie und praxis digitaler sozialität. Frankfurt, Germany: Peter Lang.
- Eysenbach, G., & Till, J. E. (2001). Ethical issues in qualitative research on internet communities. British Medical Journal, 323, 1103–1105. https://doi.org/10.1136/bmj.323.7321.1103
- Felt, A., & Evans, D. (2008). Privacy protection for social networking APIs. Security, (Section 4) 34, Retrieved from http://www.cs.virginia.edu/felt/privacybyproxy.pdf
- Frikken, K. B., & Golle, P. (2006). Private social network analysis: How to assemble pieces of a graph privately. WPES, 89–98. doi:http://doi.org/10.1145/1179601.1179619
- Garaizar, P., & Reips, U.-D. (2014). Build your own social network laboratory with social lab: A tool for research in social media. Behavior Research Methods, 46(2), 430–438. doi:10.3758/s13428-013-0385-3
- Gayo-Avello, D. (2011). Don’t turn social media into another ‘Literary Digest’ poll. Communications of the ACM, 54(10), 121–128. doi:10.1145/2001269
- Giglietto, F., Rossi, L., & Bennato, D. (2012). The open laboratory: limits and possibilities of using Facebook, Twitter, and YouTube as a research data source. Journal of Technology in Human Services, 30(3–4), 145–159.
- Gilbert, E., & Karahalios, K. (2009, April). Predicting tie strength with social media. In Proceedings of the SIGCHI Conference on Human Factors in Computing Systems (pp. 211–220). New York, NY: ACM.
- González-Bailón, S., Wang, N., Rivero, A., Borge-Holthoefer, J., & Moreno, Y. (2014). Assessing the bias in samples of large online networks. Social Networks, 38, 16–27. doi:10.1016/j.socnet.2014.01.004
- Gosling, S. D., & Mason, W. (2015). Internet research in psychology. Annual Review of Psychology, 66(1), 877–902. doi:10.1146/annurev-psych-010814-015321
- Grange, C. (2018). The generativity of social media: Opportunities, challenges, and guidelines for conducting experimental research. International Journal of Human-Computer Interaction. doi:https://doi.org/10.1207/s15327590ijhc2003
- Grimmelmann, J. (2015). The law and ethics of experiments on social media users. Colorado Technology Law Journal, 13(219), 219–272. Retrieved from http://ssrn.com/abstract=2604168
- Gruzd, A. (2016). Who are we modelling: Bots or humans? In Proceedings of the 25th international conference companion on world wide web (pp. 551). New York, NY, USA: International World Wide Web Conferences Steering Committee.
- Gudelunas, D. (2012). There’s an app for that: The uses and gratifications of online social networks for gay men. Sexuality & Culture, 16(4), 347–365. doi:10.1007/s12119-012-9127-4
- Hall, M. (2018). A mixed-methods approach to ethical data extraction in social media studies. London, UK: SAGE Research Methods Case Study.
- Hall, M., & Caton, S. (2017). Am I who I say I am? Unobtrusive self-representation and personality recognition on Facebook. PloS One, 12(9), e0184417. doi:10.1371/journal.pone.0184417
- Hall, M., Mazarakis, A., Peters, I., Chorley, M., Caton, S., Mai, J.-E., & Strohmaier, M. (2016). Following user pathways: Cross platform and mixed methods analysis. Proceedings of the 2016 CHI conference extended abstracts on human factors in computing systems (pp. 3400–3407). San Jose, USA: ACM Press. https://doi.org/http://dx.doi.org/10.1145/2851581.2856500
- Hanson, G., Haridakis, P. M., Cunningham, A. W., Sharma, R., & Ponder, J. D. (2010). The 2008 Presidential campaign: Political cynicism in the age of Facebook, Myspace, and YouTube. Mass Communication and Society, 13(5), 584–607. doi:10.1080/15205436.2010.5134
- Hay, M., Miklau, G., Jensen, D., Towsley, D., & Li, C. (2010). Resisting structural re-identification in anonymized social networks. The VLDB Journal—The International Journal on Very Large Data Bases, 19(6), 797–823.
- Hobbs, W., Friedland, L., Joseph, K., Tsur, O., Wojcik, S., & Lazer, D. (2017). Voters of the Year. 19 Voters Who Were Unintentional Election Poll Sensors on Twitter (ICWSM), 544–547.
- Hristova, D., Williams, M. J., & Panzarasa, P. (2016). Measuring urban social diversity using interconnected geo-social networks. WWW, 21–30. doi:http://doi.org/10.1145/2872427.2883065
- Hughes, A. L., & Palen, L. (2014). Social media and emergency management. In J. E. Trainor & T. Subbio (Eds.), Critical issues in disaster science and management: A dialogue between scientists and emergency managers (pp. 349–392). Washington, DC: FEMA Higher Education Project.
- Hughes, D. J., Rowe, M., Batey, M., & Lee, A. (2012). A tale of two sites: Twitter vs. Facebook and the Personality Predictors of Social Media Usage. Computers in Human Behavior, 28(2), 561–569.
- Jiang, S. (2017). The role of social media use in improving cancer survivors’ emotional well-being: A moderated mediation study. Journal of Cancer Survivorship, 11(3), 386–392. doi:10.1007/s11764-017-0595-2
- Jordan, K. (2018). Validity, reliability and the case for participant-centred research: Reflections on a multi-platform social media study. International Journal of Human Computer Interaction.
- Kazanidis, I., Pellas, N., Fotaris, P., & Tsinakos, A. (2018). Facebook and Moodle integration into instructional media design courses: A comparative analysis of students’ learning experiences using the Community of Inquiry. International Journal of Human-Computer Interaction.
- Kim, D., Kim, J. H., & Nam, Y. (2014). How does industry use social networking sites? An analysis of corporate dialogic uses of Facebook, Twitter, YouTube, and LinkedIn by industry type. Quality & Quantity, 48(5), 2605–2614. doi:10.1007/s11135-013-9910-9
- Kivelä, A., & Lyytinen, O. (2004). Topic map aided publishing – A case study of assembly media archive. In STeP 2004 - The 11th Finnish Artificial Intelligence Conference Proceedings. Vantaa, Finland.
- Koster, M. (1995). Robots in the Web: Threat or treat? OII Spectrum, 2(9), 8–18.
- Kramer, A. D., Guillory, J. E., & Hancock, J. T., 2014. Experimental evidence of massive-scale emotional contagion through social networks. Proceedings of the National Academy of Sciences, 111( 24), pp.8788–8790.
- Krosnick, J. A. (1999). Survey research. Annual Review of Psychology, 50(1), 537–567. doi:10.1146/annurev.psych.50.1.537
- Kwak, H., Lee, C., Park, H., & Moon, S., 2010, April. What is Twitter, a social network or a news media? In Proceedings of the 19th international conference on world wide web (pp. 591–600). ACM.
- Lazer, D., Baum, M., Grinberg, N., Friedland, L., Joseph, K., Hobbs, W., … Watts, D. (2017). Combating fake news: An agenda for research and action drawn from presentations by (May), 1–17. Retrieved from https://shorensteincenter.org/wp-content/uploads/2017/05/Combating-Fake-News-Agenda-for-Research-1.pdf
- Lazer, D., Brewer, D., Christakis, N., Fowler, J., & King, G. (2009). Life in the network: The coming age of computational social. Science, 323(5915), 721–723.https://doi.org/10.1126/science.1167742.Life
- Lee, K., Ganti, R., Srivatsa, M., & Liu, L. (2014). When Twitter meets Foursquare: Tweet location prediction using Foursquare. Proceedings of the 11th International Conference on Mobile and Ubiquitous Systems: Computing, Networking and Services, 198–207. http://doi.org/10.4108/icst.mobiquitous.2014.258092
- Lemke, S., Mazarakis, A., & Peters, I. (2015). Understanding scientific conference tweets. In Proceedings of the 17th General Online Research Conference (GOR 2015) (pp. 52–53). Cologne, Germany: German Society for Online Research (DGOF).
- Lerman, K., & Ghosh, R. (2010). Information contagion: An empirical study of the spread of news on Digg and Twitter social networks. ICWSM, 10, 90–97.
- Li, Z., & Duan, J. A. (2014). Dynamic strategies for successful online crowdfunding. SSRN Electronic Journal (September). doi:https://doi.org/10.2139/ssrn.2506352
- Lim, B. H., Lu, D., Chen, T., & Kan, M. Y. (2015). #mytweet via Instagram: Exploring user behaviour across multiple social networks. In Proceedings of the IEEE/ACM International Conference on Advances in Social Networks Analysis and Mining (pp. 113–120).
- Lin, H., & Qiu, L. (2013). Two sites, two voices: Linguistic differences between Facebook status updates and tweets. Lecture Notes in Computer Science (Including Subseries Lecture Notes in Artificial Intelligence and Lecture Notes in Bioinformatics), 8024(LNCS), 432–440. doi:https://doi.org/10.1007/978-3-642-39137-8-48
- Lowenstein, H., & Lev-On, A. (2018). Complementing or Substituting? News in an era of multiple platforms and second screens. International Journal of Human Computer Interaction.
- Lumezanu, C., Feamster, N., & Klein, H., 2012, May. # bias: Measuring the tweeting behavior of propagandists. In Sixth International AAAI Conference on Weblogs and Social Media.
- Malinen, S. (2015). Understanding user participation in online communities: A systematic literature review of empirical studies. Computers in Human Behavior, 46(June), 228–238. doi:10.1016/j.chb.2015.01.004
- Malthouse, E. C., Haenlein, M., Skiera, B., Wege, E., & Zhang, M. (2013). Managing customer relationships in the social media era: Introducing the social CRM house. Journal of Interactive Marketing, 27(4), 270–280. doi:10.1016/j.intmar.2013.09.008
- Markham, A., & Buchanan, E. (2012). Ethical Decision-Making and Internet Research Recommendations from the AoIR ethics working committee. In Recommendations from the AoIR ethics working committee (Version 2.0). Chicago, IL: Association of Internet Researchers. Retrieved from www.aoir.org
- McCay-Peet, L., & Quan-Haase, A. (2017). What is social media and what questions can social media research help us answer?. In L. Sloan & A. Quan-Haase (Eds.), The SAGE handbook of social media research methods (pp. 13–26). London, UK: SAGE.
- McPherson, M., Smith-Lovin, L., & Cook, J. M. (2001). Birds of a feather: Homophily in social networks. Annual Review of Sociology, 27(1), 415–444. doi:10.1146/annurev.soc.27.1.415
- Mejova, Y., & Srinivasan, P., 2012, June. Political speech in social media streams: YouTube comments and Twitter posts. In Proceedings of the 4th Annual ACM Web Science Conference(pp. 205–208). ACM.
- Mislove, A., Lehmann, S., Ahn, Y. Y., Onnela, J. P., & Rosenquist, J. N. (2011). Understanding the demographics of Twitter users. Barcelona, Spain: ICWSM, 11, p.5th.
- Moreno, M. A., Goniu, N., Moreno, P. S., & Diekema, D. (2013). Ethics of social media research: Common concerns and practical considerations. Cyberpsychology, Behavior, and Social Networking, 16(9), 708–713. doi:10.1089/cyber.2012.0334
- Morstatter, F., Pfeffer, J., & Liu, H., 2014, April. When is it biased?: Assessing the representativeness of Twitter’s streaming API. In Proceedings of the 23rd international conference on world wide web (pp. 555–556). ACM.
- Muntinga, D. G., Moorman, M., & Smit, E. G. (2011). Introducing COBRAs: Exploring motivations for brand-related social media use. International Journal of Advertising, 30(1), 13–46. doi:10.2501/IJA-30-1-013-046
- Nagpal, C., Boecking, B., Miller, K., & Dubrawski, A. (2016). personaLink. In A tool to link users across online forums. Pittsburgh, USA: Robotics Institute
- Narimatsu, H., Sugawara, Y., & Fukao, A. (2012). Cancer patients on twitter: The novel communities on social media. Annals of Oncology. 23, xi109. Retrieved from http://www.embase.com/search/results?subaction=viewrecord&from=export&id=L71792037%5Cnhttp://dx.doi.org/10.1093/annonc/mds572%5Cnhttp://sfx.metabib.ch/sfx_uzh?sid=EMBASE&sid=EMBASE&issn=09237534&id=doi:10.1093%2Fannonc%2Fmds572&atitle=Cancer+patients+on+twitter
- Noë, N., Whitaker, R. M., Chorley, M. J., & Pollet, T. V. (2016). Birds of a feather locate together? Foursquare checkins and personality homophily. Computers in Human Behavior, 58, 343–353. doi:10.1016/j.chb.2016.01.009
- Noulas, A., Scellato, S., Lambiotte, R., Pontil, M., & Mascolo, C. (2012). A tale of many cities: Universal patterns in human urban mobility. PLoS ONE, 7, 5. http://doi.org/10.1371/journal.pone.0037027
- Oh, S., & Syn, S. Y. (2015). Motivations for sharing information and social support in social media: A comparative analysis of Facebook, Twitter, delicious, YouTube, and Flickr. Journal of the Association for Information Science and Technology, 66(10), 2045–2060. doi:10.1002/asi.2015.66.issue-10
- Panek, E. T., Nardis, Y., & Konrath, S. (2013). Mirror or Megaphone?: How relationships between narcissism and social networking site use differ on Facebook and Twitter. Computers in Human Behavior, 29(5), 2004–2012. doi:10.1016/j.chb.2013.04.012
- Pappalardo, L., Rossetti, G., & Pedreschi, D., 2012, August. “How well do we know each other?” Detecting Tie Strength in Multidimensional Social Networks. In Advances in Social Networks Analysis and Mining (ASONAM), 2012 IEEE/ACM International Conference on (pp. 1040–1045). IEEE.
- Pedrana, A., Hellard, M., Gold, J., Ata, N., Chang, S., Howard, S., … Stoove, M. (2013). Queer as F** k: Reaching and engaging gay men in sexual health promotion through social networking sites. Journal of Medical Internet Research, 15, 2. doi:10.2196/jmir.2334
- Phethean, C., Tiropanis, T., & Harris, L. (2015). Assessing the value of social media for organisations : The case for charitable use uses of social media. In WebSci Vol. 15. Oxford, UK: ACM Press. https://doi.org/http://dx.doi.org/10.1145/2786451.2786457
- Podsakoff, P. M., MacKenzie, S. B., Lee, J. Y., & Podsakoff, N. P. (2003). Common method biases in behavioral research: A critical review of the literature and recommended remedies. Journal of Applied Psychology, 88(5), 879. doi:10.1037/0021-9010.88.5.879
- Poell, T., & Borra, E. (2012). Twitter, YouTube, and Flickr as platforms of alternative journalism: The social media account of the 2010 Toronto G20 protests. Journalism, 13(6), 695–713. doi:10.1177/1464884911431533
- Pontes, T., Magno, G., Vasconcelos, M., Gupta, A., Almeida, J., Kumaraguru, P., & Almeida, V. (2012). Beware of what you share: Inferring home location in social networks. Proceedings - 12th IEEE International Conference on Data Mining Workshops, ICDMW 2012, 571–578. http://doi.org/10.1109/ICDMW.2012.106
- Qi, G. J., Aggarwal, C. C., & Huang, T., 2013, April. Link prediction across networks by biased cross-network sampling. In Data Engineering (ICDE), 2013 IEEE 29th International Conference on (pp. 793–804). IEEE.
- Quan-Haase, A., & Young, A. L. (2010). Uses and gratifications of social media: A comparison of Facebook and instant messaging. Bulletin of Science. Technology & Society, 30(5), 350–361.
- Rao, L. (2012) Instagram photos will no longer appear In Twitter Streams At All, TechCrunch (Online) Available at: https://techcrunch.com/2012/12/09/it-appears-that-instagram-photos-arent-showing-up-in-twitter-streams-at-all/
- Ratkiewicz, J., Conover, M. D., Meiss, M., Gonc, B., Flammini, A., & Menczer, F. (2011). Detecting and tracking political abuse in social media. Artificial Intelligence, 297–304. Retrieved from http://www.aaai.org/ocs/index.php/ICWSM/ICWSM11/paper/viewFile/2850/3274
- Rhiu, I., & Hwan Yun, M. (2018). Exploring user experience of smartphones in social media: A mixed-method analysis. International Journal of Human Computer Interaction, 2018.
- Rose, C. (2011). The security implications of ubiquitous social media. International Journal of Management and Information Systems, 15(1), 35.
- Rost, M., Barkhuus, L., Cramer, H., & Brown, B., 2013, February. Representation and communication: Challenges in interpreting large social media datasets. In Proceedings of the 2013 conference on Computer supported cooperative work (pp. 357–362). ACM.
- Ruehl, C. H., & Ingenhoff, D. (2015). Communication management on social networking sites: Stakeholder motives and usage types of corporate Facebook, Twitter and YouTube pages. Journal of Communication Management, 19(3), 288–302. doi:10.1108/JCOM-04-2015-0025
- Ruppert, E. (2013). Rethinking empirical social sciences. Dialogues in Human Geography, 3(3), 268–273. doi:10.1177/2043820613514321
- Ruths, D., & Pfeffer, J. (2014). Social media for large studies of behavior. Science, 346(6213), 1063–1064. doi:10.1126/science.346.6213.1
- Saez-Trumper, D., Castillo, C., & Lalmas, M. (2013, October). Social media news communities: Gatekeeping, coverage, and statement bias. In Proceedings of the 22nd ACM international conference on Conference on information & knowledge management. San Francisco, USA:ACM: 1679–1684.
- Schacht, J., Hall, M., & Chorley, M. (2015). Tweet if you will – The real question is, who do you influence? WebSci, 15, Article 55. doi:https://doi.org/10.1145/2786451.2786923
- Schmidt, A. L., Zollo, F., Del Vicario, M., Bessi, A., Scala, A., Caldarelli, G., … Quattrociocchi, W., 2017. Anatomy of news consumption on Facebook. Proceedings of the National Academy of Sciences, p.201617052.
- Schroeder, R. (2014). Big data and the brave new world of social research. Big Data & Society, 1(2), 2053951714563194. https://doi.org/10.1177/2053951714563194.
- Schwarz, N., Hippler, H. J., Deutsch, B., & Strack, F. (1985). Response scales: Effects of category range on reported behavior and comparative judgments. Public Opinion Quarterly, 49(3), 388–395. doi:10.1086/268936
- Silva, T. H., vaz de Melo, P., O. S., Almeida, J., M., Salles, J., & Loureiro, A. A. F. (2013). A comparison of Foursquare and Instagram to the study of city dynamics and urban social behaviour. Proceedings of the 2nd ACM SIGKDD International Workshop on Urban Computing - UrbComp, ’13, 1–8. doi:http://doi.org/10.1145/2505821.2505836 July 2014
- Silverman, C., & Singer-Vine, J. (2016). Most Americans who see fake news believe it, new survey says. Buzzfeed, 1–14. Retrieved from https://online225.psych.wisc.edu/wp-content/uploads/225-Master/225-UnitPages/Unit-02/Silverman_Singer-Vine_BuzzFeed_2016.pdf%0Ahttps://www.buzzfeed.com/craigsilverman/fake-news-survey?utm_source=API+Need+to+Know+newsletter&utm_campaign=83c0a6de60-EMAIL_CA
- Skeels, M. M., & Grudin, J. (2009). when social networks cross boundaries: a case study of workplace use of Facebook and Linkedin. Proceedings of the ACM 2009 International Conference on Supporting Group Work, 95–103. http://doi.org/10.1145/1531674.1531689
- Sloan, L., Morgan, J., Burnap, P., & Williams, M. (2015). Who tweets? Deriving the demographic characteristics of age, occupation and social class from twitter user meta-data. Plos One, 10(3), e0115545. doi:http://doi.org/10.1371/journal.pone.0115545
- Sloan, L., Morgan, J., Housley, W., Williams, M., Edwards, A., Burnap, P., & Rana, O. (2013). Knowing the Tweeters: Deriving sociologically relevant demographics from Twitter. Sociological Research Online, 18(3), 1–11. doi:10.5153/sro.3001
- Sloan, L., & Quan-Haase, A. (2017). The SAGE handbook of social media research methods. Retrieved from https://uk.sagepub.com/en-gb/eur/the-sage-handbook-of-social-media-research-methods/book245370
- Smith, A. N., Fischer, E., & Yongjian, C. (2012). How does brand-related user-generated content differ across YouTube, Facebook, and Twitter? Journal of Interactive Marketing, 26(2), 102–113. doi:10.1016/j.intmar.2012.01.002
- Sorensen, L., 2009, May. User managed trust in social networking-Comparing Facebook, MySpace and Linkedin. In Wireless Communication, Vehicular Technology, Information Theory and Aerospace & Electronic Systems Technology, 2009. Wireless VITAE 2009. 1st International Conference on (pp. 427–431). IEEE.
- Starbird, K., Muzny, G., & Palen, L. (2012). Learning from the crowd: Collaborative filtering techniques for identifying on-the-ground Twitterers during mass disruptions. Proceedings of 9th International Conference on Information Systems for Crisis Response and Management, ISCRAM, 2011 (April), 1–10.
- Steinfield, C., Ellison, N. B., & Lampe, C. (2008). Social capital, self-esteem, and use of online social network sites: A longitudinal analysis. Journal of Applied Developmental Psychology, 29(6), 434–445. doi:10.1016/j.appdev.2008.07.002
- Thorson, K., Driscoll, K., Ekdale, B., Edgerly, S., Thompson, L. G., Schrock, A., & Wells, C. (2013). YouTube, Twitter and the occupy movement: Connecting content and circulation practices. Information, Communication & Society, 16(3), 421-451.
- Tresp, V., Marc Overhage, J., Bundschus, M., Rabizadeh, S., Fasching, P. A., & Yu, S. (2016). Going digital: A survey on digitalization and large-scale data analytics in healthcare. Proceedings of the IEEE, 104( 11),2180–2206. https://doi.org/10.1109/JPROC.2016.2615052
- Tufekci, Z. (2014). Big questions for social media big data: Representativeness, validity and other methodological pitfalls. ICWSM, 14, 505–514.
- Tyson, G., Perta, V. C., Haddadi, H., & Seto, M. C. (2016). A first look at user activity on tinder. ASONAM, 2016, 1–8. doi:http://doi.org/10.1109/ASONAM.2016.7752275
- van Dijck, J. (2013). ‘You have one identity’: Performing the self on Facebook and LinkedIn. Media, Culture & Society, 35(2), 199–215. doi: 10.1177/0163443712468605
- Vosecky, J., Hong, D., & Shen, V. Y. (2009). User identification across multiple social networks. In Proceedings of the First International Conference on Networked Digital Technologies (pp. 360–365). New York, NY, USA: IEEE. http://doi.org/10.1109/NDT.2009.5272173
- Walker, C., & Alrehamy, H. (2015). Personal data lake with data gravity pull. Proceedings - 2015 IEEE 5th International Conference on Big Data and Cloud Computing, BDCloud 2015, 160–167. http://doi.org/10.1109/BDCloud.2015.62
- Wang, P., He, W., & Zhao, J. (2014). A tale of three social networks: User activity comparisons across Facebook, Twitter, and Foursquare. IEEE Internet Computing, 18(2), 10–15. doi:10.1109/MIC.2013.128
- Xu, J., Lu, T.-C., Compton, R., & Allen, D. (2014). Quantifying cross-platform engagement through large-scale user alignment. In Proceedings of the 2014 ACM Conference on Web Science (pp. 281–282). New York, NY, USA: ACM.
- Yan, M., Sang, J., Mei, T., & Xu, C. (2013). Friend transfer: Cold-start friend recommendation with cross-platform transfer learning of social knowledge. Proceedings - IEEE International Conference on Multimedia and Expo. http://doi.org/10.1109/ICME.2013.6607510
- Zafarani, R., & Liu, H. (2013). Connecting users across social media sites: A behavioral-modeling approach. Proceedings of the 19th ACM SIGKDD International Conference on Knowledge Discovery and Data Mining - KDD ’13, 41. http://doi.org/10.1145/2487575.2487648
- Zhang, J., Kong, X., & Philip, S. Y., 2013, December. Predicting social links for new users across aligned heterogeneous social networks. In Data Mining (ICDM), 2013 IEEE 13th International Conference on (pp. 1289–1294). IEEE.
- Zhang, J., & Yu, P. S. (2015). Multiple anonymized social networks alignment. In 2015 IEEE International Conference on Data Mining (pp. 599–608). IEEE. http://doi.org/10.1109/ICDM.2015.114
- Zimmer, M. (2010). “But the data is already public”: On the ethics of research in Facebook. Ethics and Information Technology, 12(4), 313–325. doi:10.1007/s10676-010-9227-5