Abstract
With the emergent focus on human-centered artificial intelligence (HCAI), research is required to understand the humanistic aspects of AI design, identify the mechanisms through which user concerns may be alleviated, thereby positively influencing AI adoption. To fill this void, we introduce “Situation Awareness” (SA) as a conceptual framework for considering human-AI interaction (HAII). We argue that SA is an appropriate and valuable theoretical lens through which to decompose and view HAII as hierarchical layers that allow for closer inquiry and discovery. Furthermore, we illustrate why the SA perspective is particularly relevant to the current need to understand HCAI by identifying three tensions inherent in AI design and explaining how an SA-oriented approach may help alleviate these tensions. We posit that users’ enactment of SA will mitigate some negative impacts of AI systems on user experience, improve human agency during AI system use, and promote more efficient and effective in-situ decision-making.
1. Introduction
Decades of applied experience and scholarly insights speak to the central role of the user’s experience with their technology interactions in the latter’s initial acceptance, ongoing and optimal use. Emerging in the 1970s within the domain of software psychology (Shneiderman, Citation1980), early-stage human-computer interaction (HCI) efforts aimed to consider the characteristics of human beings so as to inform the design and development of interactive systems (Carroll, Citation1997). Interactive systems, and information technology (IT) more broadly, have become exponentially more powerful over time, culminating in the inception of Artificial Intelligence (AI). Following Kolbjørnsrud et al. (Citation2017), we define AI broadly as technologies that enable computers to perceive the world, analyze and understand the information collected, make informed decisions or recommend action, and learn from experience (p. 78). Nearly five decades later, with a rich corpus of HCI literature on the one hand, and rapid advancements in AI on the other, a new scholarly perspective and interest have recently emerged in ‘human-centered AI’ (HCAI). While a generally accepted definition is still lacking, given the embryonic state of this domain, HCAI is considered to encompass the underlying process of designing AI—be it a system, tool, or agent—as well as the developed artifact that aims to empower people and in turn enhance, augment, and amplify human performance (Shneiderman, Citation2021). This presents a radical shift from the previously central role of considering human characteristics to merely inform the design of the IT artifact so as to elicit utilitarian system gains to the contemporary perspective of understanding the implications of AI design on humans broadly and beyond the context of system use.
Nonetheless, the boundary condition of the contextual use of AI may serve as a springboard for producing HCAI scholarship during the domain’s infancy and ambitious agenda. Enriching the understanding of AI’s effects on humans in-situ can have significant short- and longer-term implications on both the human users of this technology as well as on the organizations utilizing AI to support their missions. This becomes particularly important given users’ relative mistrust (Gillath et al., Citation2021) and reluctance to use AI in many scenarios (Alsheiabni et al., Citation2019). Thus, research is needed to explore mechanisms through which user concerns may be effectively alleviated and AI be more optimally used.
Our goal is to help narrow the research gap between knowledge and application with regard to AI design. With this goal in mind, the work presented here has three aims: firstly, to introduce the concept of ‘Situation Awareness’ (SA) as a conceptual framework for understanding human-AI interaction (HAII). We argue that SA, a concept first introduced in the context of aviation, is an appropriate and useful theoretical lens as it can be used to decompose HAII into hierarchical layers that allow for closer inquiry and discovery. Second, we identify from prior research and elaborate on three prominent tensions inherent in HAII, and lastly, we leverage SA in proposing mechanisms to alleviate these HCAII tensions. In doing so, we enhance contemporary understanding of challenges related to the design of AI when viewed from a human user perspective and provide a lens through which future research can further refine both theoretical conceptualizations and applied practices of AI uses and effects.
The rest of the paper is organized as follows: we first briefly overview SA and its related concepts to demonstrate how SA might decompose HAII into hierarchical layers. Then, we articulate each side of the tensions inherent in HAII that we have identified from the literature. We further propose mechanisms from both the agent and human sides, through which the SA perspective might help to alleviate the tension. We conclude by highlighting the usefulness of the SA perspective in promoting HCAI research as a field.
2. Background: Situation awareness
Endsley (Citation1995, p. 1) defined SA as “the perception of the elements in the environment within a volume of time and space, the comprehension of their meaning, and the projection of their status in the near future”. When SA was defined initially, it was taken to refer solely to human situational awareness, given that human operators were also the primary decision-makers. However, SA has since become an essential factor to consider when designing operator interfaces, automation concepts, and training programs (Endsley, Citation2000). In essence, situational awareness is the mechanism that drives the reduction in information uncertainty within complex political, social, and industrial organizations.
2.1. Endsley’s three-tier model of situation awareness
Endsley’s three-tier model of SA was conceived to demonstrate that true situation awareness involves more than simply accessing the varied available sources of information. Instead, SA requires a synthesis of available information to form a model of understanding of the “situation” at hand and subsequently make projections of the future states of the elements represented in the situation related to the system operator’s goals. shows the original conceptualization of SA Endsley (Citation1995).
Figure 1. Model of situational awareness, adapted from Endsley (Citation1995).
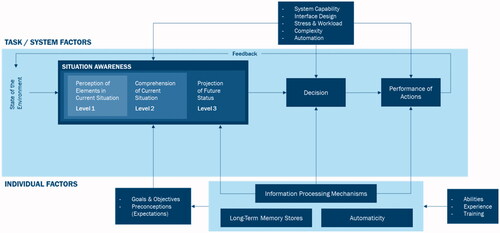
Endsley states that as a matter of consistent terminology, it is necessary to distinguish situation awareness as a state of knowledge from the processes required to achieve and maintain that state, which is referred to as situation assessment. Moreover, the SA model does not encompass the complete knowledge corpus of an individual but instead places the focus solely upon the state of SA within the operation of a specific dynamic system. In other words, the state of SA reflects the extent of a user’s perception, comprehension, and projection of elements related to the context that they aim to understand. Furthermore, the SA model does not explicitly include any decision-making process within its bounds nor take into any performance metric. This apparent lack of coverage is based upon an appraisal of human realities wherein even good decision-makers are prone to poor decisions if they have developed incorrect or incomplete SA. Conversely, even a decision-maker with a perfect state of SA may still make poor decisions based upon incorrect training or process knowledge, and both may present poor performance due to an inability to perform necessary actions. Thus, decision-making and performance are influenced not only by the state of SA held by the system operator (user), but also by a number of individual and task factors, such as a user’s information-processing mechanisms, task complexity, workload, automation, and interface design amongst others. Hence, the three tiers of the Endsley SA model—perception, comprehension, and projection—details the human factors associated with a dynamic system’s current and future state of information.
2.1.1. Level 1 SA—perception of elements in the environment
According to the model, the first tier is concerned with the initial steps required to attain a state of SA and begin creating a mental model, such as perceiving the status, attributes, and dynamics of elements in the environment. Using an aircraft pilot as an example, these elements would concern the status of the aircraft, the status of the mechanics of the aircraft, and externalities such as wind speed and meteorological conditions, among others. The system communicates these elements to the human operator through the vehicular interface. For example, the instrumentation can display relevant characteristics specific to the operating environment (e.g., speed, location, height) to allow the operator to perceive the environment. Thus, the initial stage in developing a state of SA requires becoming aware of multiple activities and their attributes within both the system and the environment so that the user could begin constructing a mental model of the ‘world’, the situational context being observed and operating in.
2.1.2. Level 2 SA—comprehension of the current situation
This tier concerns synthesizing the attributes and dynamics of the identified elements within tier 1, emphasizing the operator assigning significance to those elements to achieve system goals or complete tasks. The aim is for the decision-maker to generate a gestalt consisting of patterns and knowledge gained from tier 1 elements and processes to form a holistic picture of the now through the comprehension of events and the significance of active objects, which provide signalling for those events. For example, in the case of a power plant operator, this gestalt refers to the need to integrate disparate information sources related to the system function consisting of individual system variables and how these sources form a picture of optimal system function. Once this sense of current SA is in operation, any difference in the variables that form the gestalt will “stick out” as deviations from the current norm, allowing for a faster need to engage in a decision-making response, most often in the form to either accept or override the system’s operations.
2.1.3. Level 3 SA—projection of future status
The final tier of the SA model is concerned with the ability to project future actions of the elements within the operating environment. This projection is achieved by combining the knowledge of the elements’ status and comprehension of the situation from tiers 1 and 2 to project future system or operating environment changes. For example, an automobile driver needs to detect possible future collisions in order to act accordingly and eliminate the likelihood of such collisions. In this case, the driving action is largely autonomic in nature, requiring minimal mental effort on behalf of the driver, allowing them to assess the dynamics of the driving environment. However, automaticity in driving develops only after learning the driving skills and the operation appraisal of vehicular elements such as level of fuel, engine temperature, oil (Masters et al., Citation2008). This is an implicit learning process, a concept that was defined by Frensch (Citation1998) as the “non-intentional automatic acquisition of knowledge about structural relations between objects and events” (p.76).
This example further exemplifies the need to consider additional elements such as time and space to develop a sense of SA. It can be seen that in the case of vehicular activity, knowledge is temporal in nature, developed over time, and not exclusively related to the current operation of the vehicle, highlighting the importance of training to yield a degree of automaticity. Similarly, SA can be highly spatial and directly related to the operating environment. In the case of vehicular activity, this can be land or sky and the external elements that may dynamically interact with the operator or vehicle to force changes in SA.
Thus, it can be said that Endsley’s model of SA is cartesian in nature, in that within the model, SA as a state involves creating a conscious, stable, detailed internal representation of a situation requiring the completion of distinct linear processes that lead to decision-action before the internal representation can be updated to include to novel stimuli. Gaining a clear understanding of SA for a given task or operating environment rests on precise requirements analysis to enumerate and unambiguously identify the elements required by the operator for the perception and understanding of either. Without such analysis informing design thinking, the development of SA by operators will happen at a reduced rate and may lead to errors further downstream as the elements required for a stable sense of SA may be missing affecting decision making.
2.2. Distributed SA: Shared SA vs. compatible SA
Endsley’s (Citation1995) situation awareness model attempts to model SA from the standpoint of a human operator completing tasks within a complex system or dynamic environment. Some propositions for SA within teams were presented. However, this is more of a light treatment than a deep investigation into how SA changes in response to exposure to large complex dynamic systems involving multiple players and agents such as those existing pervasively in modern industrial domains. The theoretical basis, while broad in regard to the antecedents of human SA, barely touches upon the impact of automation and the effect of intelligent agents upon SA. This stands to reason as the original SA model is a product of its time. As such, it is widely recognized and utilized to form the basis of many current interface design and decision support agent concepts. However, technology has advanced significantly since the original conceptualization of SA to a point now that SA has become more of a distributed asset and one that requires updating to reflect those advances. To address this specific limitation of the original SA model when applied to modern complex systems that feature new hierarchies of non-human active agents, Stanton and colleagues (Stanton et al., Citation2006) proposed the Distributed Situation Awareness (DSA) model.
The DSA approach utilizes distributed cognition, in which humans offload internal representation and computation to the environment where possible in an attempt to limit the cognitive resources necessary to complete a task. This approach entails a fusion of socio-technical and systems theory to consider both human and technological agents, in addition to the ways in which they interact within complex systems. In 1995, Hutchins (Citation1995a, Citation1995b) described how socio-technical systems work in practice. Hutchins proposed that these types of systems have cognitive properties that are not reducible to the properties of individuals. He further described socio-technical systems as a division of labor in which work is divided up between agents. As an illustration, he uses the cockpit of an aircraft with its integrated displays, human interfaces, and procedural mechanisms for various functions related to flight to make the point that no human agent can actively remember and act upon all available information pertaining to the aircraft. Thus, some of the aircraft’s operation information is necessarily hidden from the pilot but available to procedural agents, which then signals the pilot to build an internal SA model. To drive the point, Hutchins describes aircraft landing procedures in which technological artifacts largely control the micro-operation of the flaps/slats due to the need to calculate aircraft weight and angular vectors to compensate for the associated lift and drag coefficients. Macro-operational control, i.e., the pulling of the flaps, is then controlled by the human pilot who exists in a state of oblivious but “perfect” task-related SA informed by positive agent signaling.
A fitting metaphor to gain further insight into how socio-technical systems operate is to imagine a network where nodes are activated and deactivated as time passes in response to changes in the task, environment, and interactions (social and technological). Within the network as a whole, it does not matter who owns the information, either humans or technological agents, just that the correct information is activated and passed to the right agent at the right time per the needs of the task. Therefore, the foundation of socio-technical systems theory is based upon a premise of “shared memory” or, more appropriately, “transactional memory,” which involves people’s reliance upon other people and technology to remember them (Sparrow et al., Citation2011). Thus, it is of little consequence if the individual human agents do not know everything, provided that all of the required information is embedded and dynamically updated within the system, enabling the system to function effectively. In this regard, the system can maintain a form of compensatory homeostasis, where agents operating within the system compensate for each other through shared transactional memory, which acts as a foundation for a shared and distributed SA that is compatible across agent types acting within the system.
The systems thinking paradigm provides the necessary theoretical foundations and tools to effectively model the dynamics inherent in complex non-linear socio-technical systems, as shown in . The model of DSA has gained traction within the ergonomics community as a valuable tool to apply when involved in systems design where situational awareness is a priority.
Figure 2. The Distributed Situation Awareness model, adapted from Salmon et al. (Citation2009).
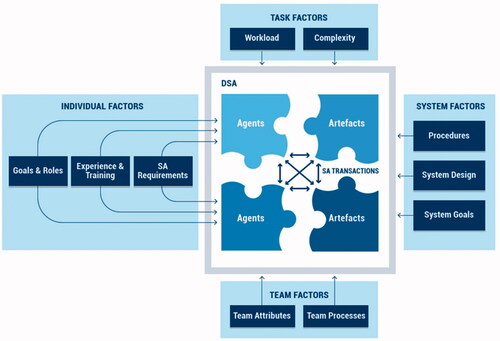
The DSA model (Stanton et al., Citation2006) consists of four sets of factors: individual, task, system, and team factors, each affecting the development of DSA. The individual and task factors are derived from the accepted SA canon. The systems factors deviate from canon in that system design is added as an external effector, while the addition of team factors insert socio-technical systems thinking to allow the modelling of team dynamics. Arguably, the main contribution of the model lies in the transactional nature of SA between artifacts (technological devices) and agents (each with individual factors) within a complex system. The model illustrates that gaining a state of SA for respective agents is not simply dependent on satisfying the elements of the individual factors for an agent. Instead, a complex system has a “baked in” requirement to distribute task, system, and team information as transactions between agents and artifacts to support the SA development of each agent within the system.
Stanton and colleagues (2006) utilized these fundamental concepts of DSA to devise a set of tenets that form the basis of the theory of DSA (Stanton et al., Citation2006). These propositions are as follows:
Human and non-human agents hold SA. Both technological artifacts and human operators have some level of SA so that they are holders of contextually relevant information. This view is particularly true as technologies are now able to sense their environment and become more animate.
Different agents have different views of the same scene. This tenet draws on schema theory, suggesting the role of experience, memory, training, and perspective. Accordingly, technological artifacts and human operators’ SA may give a different understanding of the situation.
Whether or not one agent’s SA overlaps with that of another depends on their respective goals. Hence, different agents could ‘perceive’ a world that is represented by different aspects of SA individually.
Transactions between agents—in the form of communication exchanges and other interactions—may be verbal and non-verbal, customs and practice. Artifacts and human operators transact through sounds, signs, symbols, and other aspects relating to their state.
SA holds loosely coupled systems together. It is argued that without this coupling, the performance of the system may collapse. Dynamic changes in system coupling may lead to associated changes in DSA.
One agent may compensate for degradation in SA in another agent, which represents an aspect of the emergent behavior associated with complex systems.
By considering the nature of DSA mentioned above, designing multiagent systems with DSA in mind builds resilience through redundancy (e.g., overlapping or shared SA among actors). This redundancy allows any failure of agent or artifact within a system to be compensated for in the short term, helping to maintain a balanced system-level SA through transactional memory, reducing information uncertainty and increasing individual SA until the compensation becomes apparent for failure correction.
Furthermore, from the standpoint of systems and interface design, taking a transactional approach to include both the directional and transactional nature of information flow through a system may aid in developing a form of situational memory silo. This silo would serve as a depot of information informing interfaces for signaling at a critical time or process execution. Furthermore, such a memory store also serves the purpose of providing decision transparency as all agents within the system dip into a shared memory store to perform actions or make decisions, allowing for a backtrace from a decision inflexion point to the data source for that decision. In sum, the DSA model indicates that agents (human or technological) have individual factor requirements influenced by system, task, and team factors. Using a transactional memory paradigm to store and forward information through interfaces dynamically would improve individual and organizational SA during system operations and potentially act as a system activity log to stimulate further improvements in system design.
2.3. Intelligent agents and relevance of SA
The DSA model makes no firm distinction between a human agent and an intelligent agent operating within a complex system. Agents are active elements within a system, performing tasks to fulfill the task requirements. When doing so, agents create transactional information for other agents to utilize while holding SA to perform functions within the systems architecture. The contemporary research and development of intelligent agents have made it possible to construct technological artifacts similar to how humans function. Still, and even with the nearly exponential growth in AI architectures and techniques, the holy grail of generalized and human-level artificial intelligence remains a distant future aspiration (McCarthy, Citation2007). However, with this said, current AI modelled for specific functions, typically referred to as intelligent agents, are fast becoming integral to large heterogenous technological systems. There is currently no universally accepted agreement for a standard definition of what constitutes an intelligent agent. Padgham and Winikoff (Citation2005) proposed a definition of intelligent agents based on a series of properties, including:
Situated—Exists in an environment
Autonomous—Is independent, not controlled externally
Reactive—Responds in a timely manner to changes in its environment
Proactive—Persistently pursues goals
Flexible—Has multiple ways of achieving goals
Robust—Recovers from failure
Social—Interacts with other agents
Given this definition and based on the fact that most models of intelligent agents are currently designed with most if not all of these properties in mind (Schleiffer, Citation2005), it is not hard to see why the DSA model was proposed to take into account the various forms of agents that operate within a system. An intelligent agent with all of these properties has the exact SA requirements and antecedents as a human agent within a given system. Such an agent would create a model of its ‘reality’, i.e., its operating environment, through sensors and data streams, and through reasoning or learning, build a sufficiently detailed working environment mapping to project future actions. However, this is not to say that such an agent possesses “agency” in the human sense but possesses sufficient autonomy to complete tasks and update the system to affect the SA of other agents within that system through actions or decisions taken to perform its functions. Therefore, the need for transactional memory within large complex systems dictates that an intelligent agent should be designed with inter-agent communication and moderate transparency in mind to ensure the system’s robustness.
As has been discussed previously, the concept of SA and DSA has been widely studied, especially in the context of the human-automation team operating environments. The concept of SA applies to any complex scenario in which humans have multifaceted informational needs to achieve the tasks they are performing or are expected to perform. In this regard, models of SA can provide structure for information provided that may lead to greater levels of transparency on the part of the AI agent and the engendering of trust and confidence in the AI agent on the part of a human. Endsley’s (Citation1995) three-tier model has direct relevance for the designers of complex systems due to its relative simplicity and its division into three decomposable levels. These three levels allow for the easy enumeration of SA requirements for different scenarios and provide the opportunity to evaluate SA held by each agent within a complex system. Endsley’s SA model and Stanton et al.’s DSA model could be used to define a framework for agent transparency that is primarily concerned with what information should be displayed on interfaces to explain agent behavior (Chen et al., Citation2014).
According to Endsley (Citation1995), SA represents the continuous diagnosis of the state of a dynamic world. Thus, there exists a “ground truth” against which and agents (both human and AI) SA can be measured using the situation awareness global assessment technique (SAGAT) test—a test that attempts to measure the discrepancies between a human user’s SA and this “ground truth” state of the world. SA is relevant in the context of human-agent interaction and teaming as it provides a method to define both human and AI informational needs to provide transparency and engender trust in a system for the human counterpart. However, there is a hard divide between human SA, intelligent agent SA, and what the human user needs as a subset of AI SA to understand an intelligent agent’s behavior within a system. In this regard, both the Endsley and DSA model of SA can describe the type of information that systems should provide to explain agent behaviors.
It is currently a question of policy, philosophy, and design as to whether full autonomy—to include human-level agency—should ever be programmed into autonomous intelligent systems. However, it appears that regardless of prevailing cultural mores, autonomy is manifesting increasingly in these types of agents within various industrial domains.
3. Developing SA in human-AI interaction: Alleviating three tensions
From the preceding presentation, it becomes apparent why SA is relevant to HCAI. In essence, the very mission of HCAI, which is to enhance, augment, and amplify human performance (Shneiderman, Citation2021), would be severely impaired if the user’s SA is either lacking or deviating from the operational reality of a given human-AI interaction context. Therefore, identifying such situations and developing mechanisms through which the development of user SA can be supported becomes of paramount importance. To this end, we engaged in a review of the HCAI literature to date, distilling retrieved concepts and findings. The research team analyzed the literature and took notes when interesting inconsistent results were identified (i.e., positive and negative impacts on users’ SA). Iterative analysis of theoretical reasons resulted in the revelation of three prominent tensions inherent in human-AI interaction that indeed may adversely impact a user’s SA, namely (i) automation and human agency, (ii) system uncertainty and user confidence, and (iii) system’s objective complexity and a user’s perceived complexity. These three tensions are presented next, along with our systematic literature search and analysis process. For each tension, we leverage SA in proposing mechanisms to alleviate them, contributing to optimized human-AI interaction and the design of HCAI.
3.1. Literature search and screening
This project is part of a large-scale systematic literature project on human-AI collaboration, which adopted systematic and rigorous approaches in literature search, screening, and coding procedure, following the guidance of conducting a standalone systematic literature review (Okoli, Citation2015). To systematically identify the literature related to SA and HCAI, we utilized Web of Science (WoS), EBSCO, IEEE Xplore, and ACM library as our primary databases. They provide excellent coverage of peer-reviewed scientific articles across different domains. The search was performed using keywords including “situation* awareness,” “distribu* awareness,” “team SA,” “shared SA,” “compatible SA,” and “collective SA” for articles published between 2006 and 2021 and yielded 6,897 articles. Since the objective of the review is to understand the role of SA in the context of human-AI interaction, a vast number of these articles had limited value, and thus we initiated a systematic screening and coding procedure to select the relevant articles based on the following criteria:
The study should involve some form of AI (as defined in the Introduction section).
The study should involve human-AI interaction (regardless of the level of user control versus agent autonomy or agent automation).
The study should investigate nomological relationships of SA or Distributed SA (DSA) (i.e., antecedents, processes, and outcomes of SA or DSA).
System design research articles should include empirical user tests.
Conference papers should be at least three pages long for a minimum expected value and quality control.
Only the most recent one is included for multiple publications based on the same dataset.
Overall, this process gave us 133 articles that investigate antecedents or outcomes of SA in human-AI interactions. Then, we coded each article based on (1) research context, (2) type of AI (e.g., chatbot, medical system, auto-driving systems), (3) type of interaction (e.g., task support, decision making), (4) the role of SA in human-AI interactions (e.g., as an antecedent, mediator, moderator), (5) outcomes of human-AI interaction, (6) impacts of SA, (7) how SA manifests, and (8) how SA is measured. The coding process also provided opportunities for inductive sense-making, which yielded the three tensions that we explain in the following sections. We also delineate why SA might be useful for addressing these tensions.
3.2. Tension 1: Automation and human agency
AI systems are often characterized by a high level of automation, which significantly influences how humans interact with these systems and make decisions (Prangnell & Wright, Citation2015). To give a general sense of what automation means in its contemporary context, we offer a distillation of various dictionary definitions of the term: automation is a technique, method, or system of operating a controlling process by automatic means, such as by algorithm or digital device, therefore reducing or replacing human intervention. It is critical to note that automation has different levels and can be applied to a brand range of tasks. For example, M. R. Endsley and Kaber (Citation1999) proposed that four types of tasks can be automated, including monitoring the information, generating options, selecting the options to employ, and carrying out the actions. The classic 10-level model of automation (see ) developed by Sheridan and Verplank (Citation1978) views computer automation as ranging from a fully human-controlled to a fully autonomous system, which can be used as the basis for understanding the extent to which the human versus the AI agent has control over the decisions and actions involved. Contemporary AI studies may differentiate automated systems and autonomous systems, arguing that an automated system can only perform pre-defined tasks and produce deterministic results, whereas an autonomous system can perform tasks independently and generate non-deterministic results (de Visser et al., Citation2018; Xu, Citation2021). In this essay, we follow Sheridan and Verplank (Citation1978) and view autonomous as the most advanced level of automation. Accordingly, we view contemporary AI agents as possessing varying levels of automation that require or allow different levels of human control.
Table 1. Sheridan and Verplank (Citation1978) ten levels of automation.
Whereas many people believe that automation is beneficial, the extant literature on AI has identified various automation-related issues and pitfalls. For example, automation may bring unexpected errors and degrade users’ knowledge and skills, ultimately harming task performance (Miller et al., Citation2014; Onnasch et al., Citation2014; Smith & Baumann, Citation2020). Moreover, increasingly automated systems become more difficult for users to understand what the system is doing and its rationale, resulting in mistrust, increased mental workload, perceived complexity, and system resistance (Hjalmdahl et al., Citation2017; Miller et al., Citation2014; Skraaning & Jamieson, Citation2021; Sonoda & Wada, Citation2017; Yamani et al., Citation2020).
While various theoretical mechanisms can contribute to the countervailing effects of automation, we found that the tension between automation and human agency (i.e., an increase in automation may harm human agency) is particularly critical (Berberian et al., Citation2012; Engen et al., Citation2016; Sundar, Citation2020). For example, in the transportation and logistics sector, ensuring products are taken from one place and delivered to another at a speed that meets the demands of the “just-in-time” inventory management methods requires tremendous automation in domains such as delivery vehicle loading and procurement forecasting. Such automation can mean greater flexibility and precision, leading to improved profit margins. However, the tension would arise when the intelligent system makes decisions based upon data that are opaque to the human counterpart and at timescales that the human counterpart cannot comprehend or process, leading to a mismatch in shared “reality” between humans and AI. There is a potential loss of agency and, hence, the human’s inability to reach consonant decision-action cycles. To place the human in a supervisorial role in this context without some form of dynamic information provisioning would, in most cases, denude the benefit gained from emplacing the intelligent agent within the logistics chain.
Three theoretical perspectives may explain how the tension between automation and human agency arises—the automation paradox, the automation-control tradeoff, and automation-induced complacency. First, the automation paradox, frequently mentioned in human-AI interaction research (De Bruyn et al., Citation2020; Janssen et al., Citation2019), refers to the phenomenon that as the system becomes increasingly automated, human operators’ contribution becomes increasingly crucial (Bainbridge, Citation1983). It challenges the conventional view that as the system increases its automation level, the need for human input ceases. This is because, when the simpler and routine work has been automated, the tasks left over for humans tend to be more complex, obliging humans to know more. Human operators not only need to know how the automated systems work but also how and why the system may fail to work. Otherwise, automation bias may happen when humans are deskilled and lose vigilance (Bond et al., Citation2018). This means humans are often expected to become domain experts to stay in the loop and collaborate closely with automated systems when augmentation happens. For example, managers need to evaluate, revise, and select the appropriate logistics outputs to solve specific inventory planning problems when using the logistics AI system, and thus a good command of domain knowledge is needed. However, humans may not have the required level of knowledge or mental model to collaborate with the machine deliberately and effectively, and hence lose their agency and may fail in the task.
Second, the automation-control tradeoff has also long been an area of discussion. Tracing back to Sheridan and Verplank (Citation1978) ten-level model, it has an inherent tradeoff that as the level of automation increases, the room for human participation—and hence, control—decreases, and ultimately, an autonomous system can ignore humans completely without even informing humans about the system decisions. In this scenario, humans lose their agency, which is generally considered undesirable since humans may feel out of control and thus be reluctant to trust the system, hindering decision-making and task performance (Berberian et al., Citation2012; Engen et al., Citation2016). This phenomenon is also called the “out-of-loop” problem, where humans lose their control of the system both operationally and cognitively as automation increasingly takes over the operations—humans become handicapped in their ability to understand the situation and system intentions (Endsley & Kiris, Citation1995; Le Goff et al., Citation2018 ).
Third, related to the lack of control problem, the literature also discussed automation-induced complacency—the potential for humans to over-trust automated systems and thus get trapped in self-satisfaction, lack of vigilance, and lower agency (Merritt et al., Citation2019; Parasuraman et al., Citation1993). Automation-induced complacency is a multifaceted issue relating to both attitudinal and behavioral aspects of human-AI interaction. For example, Singh et al. (Citation1993) found that such complacency can be attributed to users’ general attitudes towards automation, confidence in the system, perception of the automation reliability, trust in automation, and perception of automation safety. When human-in-the-loop is required, automation-induced complacency may hinder humans’ role as a supervisor or a collaborator of the system because when humans are over-reliant on an automated system, they allocate less attention to it, thus reducing their supervisory effort and performance (Parasuraman & Manzey, Citation2010). Previous research has reported that automation-induced complacency leads to lower error detection and higher task disengagement (Bowden et al., Citation2021; Ferraro & Mouloua, Citation2021).
As the discussion of automation and beyond is still highly relevant in the field of HCAI (Schmidt, Citation2020; Shneiderman, Citation2020), the issues brought on by automation can be a major roadblock to the development of the field. On the one hand, human-in-the-loop is needed to accomplish the augmented tasks, and thus humans need to be empowered to work with the highly automated system. On the other hand, automation may harm human agency in performing these tasks, and thus, humans lack empowerment. Consequently, when, where, and how to automate processes has become an issue of ever-increasing importance in HCAI. Despite the number of studies on this topic, the question of how best to implement automation is still the subject of much theoretical research (Colombo et al., Citation2019; Ng et al., Citation2021; Villaren et al., Citation2010).
We expect a lack of information to be a major cause of the tension—either due to a lack of transparency and explainability in the automated systems, or humans’ cognitive deficiency in processing the information available such as lack of attention due to over-reliance. From an AI agent’s perspective, if the information held by the agent can be made well visible and comprehensible by human users, a high level of system transparency and explainability can be achieved. This can alleviate the tensions between automation and lack of human agency. In the logistics systems example, if the system can provide managers with dynamic transactional information, managers will be better empowered (i.e., increased sense of human agency) to handle exigent circumstances by tracking the transaction history of the system’s decision-action cycles. From a user’s perspective, if users’ expertise and domain knowledge can be well communicated with AI agents where necessary—such as being used as the system input or supervisory mechanisms—humans can develop their sense of agency through deliberate engagement, thereby alleviating the tension with user cognitive deficiencies brought on by automation-induced such as lack of attention and complacency (Glikson & Woolley, Citation2020; Guzman & Lewis, Citation2020; J. T. Hancock et al., Citation2020).
3.2.1. The role of SA in alleviating the tension
Numerous studies have proposed various approaches to enhance AI’s transparency, explainability, and human comprehensibility (Miller & Parasuraman, Citation2007; Mohseni et al., Citation2021; Schoonderwoerd et al., Citation2021 ). However, a holistic framework to organize the various elements that have been proposed is currently lacking. To this end, we propose that SA is a useful lens to understand how to empower users through enhanced visibility of the systems’ underlying processes (Level 1 SA—perception), which allows users to understand the current status (Level 2 SA—comprehension), and prime users about future steps (Level 3 SA—projection). Specifically, the SA transparency (SAT) model (Chen & Barnes, Citation2015; Chen et al., Citation2018 ) is of particular interest. SAT provides an overarching framework to organize the various elements that can improve agent transparency, which we believe can subsequently improve users’ comprehensibility of the AI system, user SA, and task performance.
In line with the three-tier SA model, SAT posits that an autonomous system should convey three levels of information when interacting with humans to achieve transparency. The first level is the agent’s goals and actions, which is the agent’s current status and plans, including the agent’s goal selection, intention execution, progress, current performance, and perception of the environment. This level of information supports human users’ level 1 SA (i.e., perception) since it helps users perceive various environmental elements captured by the autonomous system. The second level of information that should be conveyed is the agent’s reasoning process, such as the various conditions and constraints that afford the agent’s plan and actions in level 1. This level of information answers the “why” question to users, which helps users comprehend the status and agent’s current behaviors, thus improving AI explainability and supporting users’ level 2 SA (i.e., comprehension). Finally, the agent should provide users with information regarding its future projection, such as predicted outcomes, the likelihood of success or failure, and the associated uncertainties. This level of information supports level 3 SA (i.e., projection) in users since it helps users understand what might happen if they continue following the intelligent agent’s plan. Furthermore, users are more likely to build trust in the agents if the course of action and the associated uncertainty can be well explained (Hoff & Bashir, Citation2015; B. Liu, Citation2021).
In the logistics system example, the design of the interface can be ecologically valid and task-driven by leveraging the SA transparency model. For example, providing past transactional information and current decision-action cycles packaged in human-readable format can support level 1 SA. The information used by human agents to make just-in-time decisions should be updated in an appropriate timescale (i.e., fitting the pace of the task) and passed back to transaction history, supporting level 2 SA. In addition, forecasted decision-action cycles can be posited with the current and past transactional information presented as a chain to be followed by the human as needed to reduce epistemic uncertainty and increase their sense of agency in cases of exigent circumstance, supporting level 3 SA.
In summary, we expect the usefulness of the SA framework to be reflected in its flexibility to handle both AI-centric and human-centric designs by simultaneously improving system transparency and human comprehensibility of the system. Since both AI agents and humans have their own SA, juggling the information and knowledge between the three levels during human-AI interactions harmonizes the tensions between automation and human agency. When users are directed to the most relevant information—be it current status, reasoning process, or future projection—users are empowered and less likely to experience cognitive deficiencies. That being said, automation and control are no longer mutually exclusive as long as there is a sufficient level of automation transparency to allow users to exert their agency in supervising the system and be accountable for their decision-making.
3.3. Tension 2: System uncertainty and user confidence
Besides automation, uncertainty is another inherent characteristic of AI. From a modeling perspective, probability theory—the mathematical foundation for most AI systems—plays a significant role in quantifying uncertainty (Zadeh, Citation1986). Moreover, data—a core ingredient of AI—is uncertain in various aspects, such as how well it can capture reality and quality issues (Vial et al., Citation2021). Various other forms of uncertainty in AI have been discussed, such as the problem scope, the performance of data-driven modeling, partial information, imprecise representation, and conflicting information (Kanal & Lemmer, Citation2014; Kläs & Vollmer, Citation2018; Wu & Shang, Citation2020). For complex socio-technical systems like AI, presenting the information efficiently to help users understand the uncertainty in the situation and build confidence in the system is a major challenge, which consists of our second tension—between system uncertainty and user confidence. That is, when AI information uncertainty is inadequately represented by the system to the user, user confidence suffers, exacerbating decision-making uncertainty and in turn having detrimental impacts on user satisfaction and in situ decision-making.
Taking a workforce management system as an example, shift planning can consist of various sub-tasks like demand prediction, resource planning, worker assignment, team development, and same-day micro-planning. There are various types of uncertainty inherent in these sub-tasks due to—for example, a lack of explicit knowledge regarding human relations and their social constraints—so human verification is often needed to intervene in system decisions (e.g., who should do the night shift and for how long). If the system cannot present its uncertainty properly, users may be less convinced by the system’s decision (i.e., lack of user confidence), especially when conflicts exist (e.g., the automated worker assignment violates the worker’s social constraints).
Information uncertainty in AI encompasses various aspects, such as ambiguity, low probability, and high complexity (Bonneau et al., Citation2014; Dequech, Citation2011), which influences AI performance and inhibits users’ comprehension and projections of the situation. Although machines have various technical mechanisms to gather, perceive and integrate the information to generate system knowledge, end-users rely on interface knowledge (i.e., visualized knowledge on the interface) to understand the situation (Endsley & Garland, Citation2000). Besides the uncertainty captured by the system knowledge, when the machine converts system knowledge to interface knowledge, the discrepancies may create new uncertainties in AI explainability and human interpretation, inhibiting user trust and confidence in AI. Accordingly, managing users’ uncertainty perception to help them establish confidence in the system when facing high system uncertainty becomes an important challenge that needs to be addressed.
3.3.1. The role of SA in alleviating the tension
Previous studies show that presenting uncertainty-related information, often using decision aids like thresholds, decision paths, and potential conflict resolutions, can help users understand the situation and improve task performance (Bhaskara et al., Citation2020; Kunze et al., Citation2019; Rajabiyazdi & Jamieson, Citation2020; Schaefer et al., Citation2017). Various types of uncertainty representation strategies have been investigated, such as displaying detailed process information, explaining automation modes and reasoning, and showing numeric uncertainty directly (Kunze et al., Citation2019; Skraaning & Jamieson, Citation2021; Stowers et al., Citation2020). Despite the tremendous effort that has been made in the field, the implementation of uncertainty representation strategies is relatively piecemeal, and the extent to which reducing information uncertainty in AI can help improve user confidence in the system and decision-making is largely unknown.
We expect the SA lens to guide the design of uncertainty representation in AI and explain how such design may harmonize the tension between the inadequacy in AI agents’ interface knowledge and users’ confidence in AI and themselves. The three levels of SA provide a holistic view to formalize the domain representation of the complex ecosystem where AI and humans interact. A formalization is needed because the information and knowledge held by the system should be communicated in a structured way that is compatible with users’ cognitive and perceptual properties (Engin & Vetschera, Citation2017; Paulius & Sun, Citation2019). The uncertainty-related information can be organized into system perception, system reasoning, and system projection, which corresponds to users’ three levels of SA. Hierarchies may exist depending on the work process and work domain so that multiple layers of SA allow users to juggle among decisions at different levels of granularity and develop in-situ coping strategies to manage the uncertainty.
In the workforce management system example, the shift planning interface can try to clearly indicate non-modeled dimensions, such as worker relations and dynamics, expertise coupling, and employee personal circumstances not captured by the system (e.g., employee’s psychological well-being and disposition; social constraints). These non-modeled dimensions impose uncertainty in the system (e.g., incomplete information about the worker), and presenting them will help reduce managers’ perceived uncertainty about the system decisions. Now, managers have the option to take into account these tactics knowledge if needed. While supporting level 1 and level 2 SA regarding workers’ past and current situations, the system uncertainty information help managers gain better awareness, thereby enhancing user confidence, which is helpful for future planning tasks (i.e., further supporting level 3 SA).
This SA-oriented design is in line with the widely adopted ecological interface design approaches, which suggest that the complex work domain should be represented using a hierarchical structure with clear means-end relationships that are goal-oriented (Vicente & Rasmussen, Citation1992). From an SA perspective, transparency in uncertainty (e.g., present unexpected events and constraints) provides users with guidance on action-oriented uncertainty management. Users perceive the elements related to uncertainty in the work domain, comprehend why the unanticipated events or outcomes might happen by interpreting the confidence assessment of the output and reliability information provided by the system, and finally, they can project the coping strategy and the associated outcomes (Endsley, Citation2016).
Moreover, the SA lens provides a psychological foundation for uncertainty management. During human-AI interaction, user SA development is not a passive process by simply receiving displayed information. They need to be actively engaged—for example, by controlling the display mode, performing drill-down or aggregation to locate the relevant information, and determining which information to attend to. This process is called situation assessment, an underlying process for users to obtain SA (Endsley & Garland, Citation2000). For example, if the uncertainty-related workforce management information can be organized around users’ goals (e.g., planning night shifts) and support both top-down and bottom-up work processes (e.g., daily micro-planning versus monthly planning), users can easily absorb the information and perform the confidence assessment of the system output and information reliability. We expect an SA-oriented interface knowledge design that fits the users’ cognitive needs can help users manage information uncertainty and establish confidence because it helps users move from level 1 SA (i.e., perceive the uncertainty elements in the situation) to level 2 and level 3 SA (i.e., understand the uncertainty management strategy and the projected outcomes). During this process, the system can guide users to pay attention to the most relevant information (e.g., specific social constraints on certain workers). Users can also actively locate this information from the whole system to yield action-oriented uncertainty management strategies (e.g., pre-plan the resource to compensate for a future medical leave that may break a team workflow).
In summary, the SA lens can provide useful design guidelines because it can present the work process and domains in the form of an abstraction hierarchyFootnote1 (i.e., based on level 1 to level 3 SA) to serve as a goal model that clarifies the constraints and cues related to uncertainty, which improve the interpretability of the information uncertainty in AI. Furthermore, information uncertainty may arise from various sources—some relate to the state of the environment (e.g., missing information, less reliable data source, noisy data, outdated data). In contrast, others arise during human-agent interactions due to users’ limited understanding, skills, and abilities to predict (Kruger et al., Citation2008). The SA lens provides a psychological foundation to understand how users interpret various cues and develop their perception, comprehension, and projection of the situation, further informing decision-making and establishing confidence.
3.4. Tension 3: System’s objective complexity and user’s perceived complexity
As mentioned earlier, automation adds complexity to the system so that users may feel challenged to understand the system’s view of reality or the decisions it made. AI is inherently complex, and the extant literature has investigated various elements that may contribute to different types of complexity. For example, factors such as system components, features, objects, the interdependence of these components, degree of interactions, system dynamics like the pace of status change, and predictability of the dynamics contribute to system-level complexity (Endsley, Citation2016; Tegarden et al., Citation1995; Windt et al., Citation2008). An over-complex system may result from feature creep, which increases users’ mental workload and harms task performance since the entire system becomes difficult to understand (Page, Citation2009). However, a complex system does not necessarily mean that it is complex to use. For example, a self-driving car has complex automobile mechanics (e.g., more sensors, information fusion mechanisms, and diagnostic components), but the operation can be much less complex than a traditional car.
Nevertheless, even if a highly-automated system is easy to use, users may still see complexities in the AI tasks and information flow (Bonchev & Rouvray, Citation2003; Sivadasan et al., Citation2006), which gives rise to operational or task-level complexity in AI. For instance, a highly automated route planning system in a self-driving vehicle may have a high level of task complexity due to the multiple decision paths and the interdependence among these paths. If the task augmented by AI requires various interdependent components to proceed, users may experience a high level of complexity, which hinders task efficiency and performance (Fan et al., Citation2020; Guo et al., Citation2019). Specifically, users may face equifinality (i.e., multiple paths may lead to the same outcome) and multifinality (i.e., multiple outcomes can be developed from a specific factor), leading to information overload and high cognitive load. If a driver who experiences information and cognitive overload needs to handle emergency issues during route planning, poor decisions may be made due to such task complexity.
Moreover, like the uncertainty coming from interface knowledge, the information presented on the interface may bring additional complexity, also called presentation complexity or apparent complexity (Endsley, Citation2016). In the self-driving car example, presentation complexity can easily be escalated if all environmental information influencing speeding and routing is presented to the driver. Although the deep structure of a system can be complex, the representation of such complexity needs to be well-organized to help users reduce perceived complexity. Whereas an oversimplified presentation may reduce the system’s transparency, a complicated presentation costs users a significant amount of cognitive resources to process the information, which often contributes to errors and failures (Jiang & Benbasat, Citation2007; Woods, Citation2018). A tradeoff is needed to determine to what extent system and operational level complexity is presented to users and in what way.
3.4.1. The role of SA in alleviating the tension
Empirical evidence has shown that presenting environment complexity information to users can enhance user understanding of the situation; however, when the presented information is too dynamic, the understandability decreases (Habib et al., Citation2018; Kang et al., Citation2017). Task complexity also influences users’ understandability in a curvilinear way such that a tipping point exists for users to maintain task performance (Cummings & Guerlain, Citation2007; Mansikka et al., Citation2019). In general, a system design that can be aware and adaptable to users’ mental models and goals is beneficial (D’Aniello et al., Citation2019; Ilbeygi & Kangavari, Citation2018; Park et al., Citation2020). However, defining in-situ goals and building a good mental model is more difficult for users when the AI system has high system complexity, operational complexity, and apparent complexity because more components and interdependencies are involved.
Various techniques have been discussed to improve complexity representation. For example, explicitly mapping the system function, reducing information density on display, and simplifying the user’s actions to fulfill the task goals have been proposed in the literature (Liu & Li, Citation2012; Windt et al., Citation2008). Although these design principles may help reduce perceived complexity from a technical perspective, to what extent are they consistent with users’ in-situ task goals and mental models is largely unknown. Users may perceive the system as more complex (or simpler) than it really is since the information embedded in the system and displayed by the interface may be comprehended by the user in different ways depending on their mental models, experience with the system, and task goals (Agrawal et al., Citation2017; Habib et al., Citation2018; Li & Wieringa, Citation2000; Marquez & Cummings, Citation2008). Moreover, in many situations, novice users may not have a mature mental model, and thus designing a system to fit existing mental models becomes impossible (Nadkarni & Gupta, Citation2007; Te’eni, Citation1989).
To this end, we expect the SA lens to be useful in alleviating the tension between the system’s objective complexity and users’ perceived complexity in two ways: first, SA provides a holistic framework to guide the design for complexity reduction; and second, SA provides a psychological foundation, explaining how users’ development of SA allows them to navigate the focus of attention and different levels of construal, contributing to the reduction of perceived complexity and improved perceived control (Hancock et al., Citation2011; Kim & Duhachek, Citation2020). Specifically, SA provides a guiding framework to enhance the design of the system for complexity reduction. The three levels of SA allow multiple abstraction levels (e.g., detailed environmental elements vs. in-situ reasonings vs. context-free actions rules) to represent the complexity, covering system-wide technical complexity, process-wide operational complexity, and interface-wide informational complexity. For example, the black-box prediction models in a self-driving vehicle may bring complexity at the system and process levels so that drivers may fail to understand the rationale behind AI decisions like speeding and lane changing. The representation of such complexity contributes to the explainability of AI, which can be local or global and model-specific or model agnostic (Rai, Citation2020). It ultimately enhances users’ perception, comprehension, and projection of the situation through presenting system perception, comprehension, and projection in a structured way. Hence, regardless of the type of objective complexity in the AI system, it is recommended that how well a user could understand the system rationale in a given context should be taken into consideration during the system design (Miller, Citation2019). The complex environment information perceived by the self-driving vehicle should be presented to the driver in a way relevant to the task (e.g., lane following vs. routing need different environment information) and the driver’s mental model (e.g., novice driver vs. expert driver need different information). We expect that the SA lens provides a framework to guide such design by mapping the various design elements onto the three levels of agent SA, which can guide users to understand and absorb different types of complexity, consequently reducing perceived complexity and improving perceived control for in-situ decision-making.
Furthermore, the development of SA in users provides psychological justification regarding how users may earn a sense of control in a highly automated yet complex AI. When users juggle multiple levels of perceived complexity, they are navigating ones’ attention to comprehend the situation so that they can be on the right track to accomplish the task goal. This is in line with the temporal construal level theory (Liberman & Trope, Citation1998), which explains the importance of aligning different abstraction levels of mental representation with one’s goals to improve prediction and action (Trope & Liberman, Citation2010). A more abstract level of mental representation of the event is subject to multiple interpretations since details are omitted; however, the process of abstraction also creates hierarchies for information interpretation and actions since goals can further be developed into subordinate and superordinate goals. Thus, people with different construal levels may respond to the same stimuli differently (Freitas et al., Citation2009; Lee, Citation2019; Trope et al., Citation2007). Think about a scenario where the driver’s primary task in operating a self-driving vehicle is lane following, which is highly automated (i.e., a less complex situation for the driver). An element change in the environment (e.g., previously unknown road construction) may create an emergent situation that requires route re-planning—a secondary semi-automated task emerges with a higher level of complexity for both the system and driver. A notification may pop up, and the system may provide multiple alternate routes for drivers to select. If the information provided to support the route selection decision does not match the driver’s construal level (e.g., too abstract or too specific), the secondary task may disrupt the primary task, causing a negative driving experience and even dangers.
The hierarchies created by SA (i.e., perception—comprehension—projection) allow users to actively leverage different system complexity to achieve an appropriate level of task goal abstraction and course of action. In the case of a vehicle complying with lane following, the system’s complexity is high. However, the user need not be exposed to the system’s underlying modeling of the ‘world’. Instead, they merely need to be informed that the vehicle is functioning within set boundaries (e.g., the lanes). For route planning, which also has inherent objective system complexity, user perceived complexity varies between the phases of a driving journey (e.g., routine drive vs. unfamiliar routes vs. emergencies). In case of emergent information (e.g., road accidents; traffic jams) during lane following, when the primary task is now operating the vehicle to adapt to the new situation, minimizing perceived complexity is paramount. The SA lens would afford an optimal in-situ design for recommending alternate routes in a manner that fits both the primary task by reducing the cognitive demands of the secondary task and the secondary task by displaying the minimum viable set of information required in decision-making by the driver. If the users can actively adjust their complexity interpretation to match their in-situ goals and mental models, they will experience a lower level of perceived complexity, enhancing decision-making and task performance.
In summary, an SA-oriented design can aid users’ SA development by directing user attention to relevant information, which should be organized around users’ goals and mental models. The development of SA will help users reduce the system’s perceived complexity so that they are in a better position to exert human control and make better in-situ decisions.
4. Reflection and conclusion
Inspired by AI’s augmentation and empowerment potential for humans, the recent call for HCAI represents a clash and deviation from the traditional approach to building AI systems, which focused more on automation and replacing human roles. The HCAI community advocates empowering humans and preserving human agency in AI design. In many contemporary working environments, intelligent agents and human actors bring about complex socio-technical systems that require both human-in-the-loop and agent-in-the-loop to accomplish the task effectively. Various tensions emerge during human-AI interactions, imposing user concerns and harming task performance. Despite the flourishing development of the field, research is needed to understand the mechanisms through which these user concerns may be effectively alleviated and AI be more optimally designed and used.
This essay aims to fill in the gap by introducing the concept of ‘Situation Awareness’ as a conceptual framework for considering human-AI interaction. The merit of the SA lens lies in its ability to consider both system design and user perceptions and behaviors concurrently. Hence, It can provide both psychological foundations of human behaviors when interacting with AI and help describe AI effects while prescribing human-centered design approaches that mitigate the inherent tensions. We contribute to the field of HCAI research by introducing a new lens that can better position the role of humans in human-AI collaboration, which goes beyond the tool view of AI systems (i.e., humans simply use AI as a tool).
After reviewing the recent HCAI literature, we further identify three fundamental tensions inherent in human-AI interactions. Furthermore, we posit the SA perspective is a useful framework for alleviating the tensions by enhancing the design of agent SA and the development of user SA. summarizes our key arguments regarding how the SA perspective might address the tension between (1) automation and human agency, (2) system uncertainty and user confidence, and (3) system’s objective complexity and users’ perceived complexity. The two approaches and the concrete examples we discussed provide practical implications for how to implement the SA lens in designing specific interface elements for better AI transparency and explainability, making the information presentation efficient and trustworthy. They also have practical implications for educating and empowering users by facilitating active leverage of the three levels of SA to organize the complexity and uncertainty at the right construal level based on the task relevance.
Table 2. Summary of the perspective and role of Situational Awareness (SA) in alleviating the three tensions.
We expect addressing these three tensions is a key step in promoting HCAI since they directly speak to user experience during human-AI interaction and, consequently, the effectiveness of AI-enabled human augmentation and empowerment. First, improving human agency becomes essential for affording user control, which is the individual’s perceived self-efficacy and autonomy in using the AI in a particular context. Increasing users’ sense of control is an important boundary condition for improving technological acceptance and task performance (Le et al., Citation2022; Zafari & Koeszegi, Citation2021). Second, establishing confidence in users by better presenting relevant information, plans, and projections will enhance the perceived usefulness of the system through better decision support (van der Waa et al., Citation2020). Finally, reducing users’ perceived complexity in the system and the associated tasks can enhance the system’s perceived ease of use and learnability (Keil et al., Citation1995; Ziefle, Citation2002). These three tensions we have identified are logical extensions from well-established theories in information systems that speak to the role of the technology (e.g., usefulness and ease of use), as well as the effects of user characteristics (e.g., user control and empowerment) in influencing AI acceptance and task performance.
The Technology Acceptance Model (Davis et al., Citation1989) popularized the former effects and has since been both extensively validated and extended by various further investigations such as UTAUT (Venkatesh et al., Citation2003) and UTAUT2 (Venkatesh et al., Citation2012). The constructs of ease of use and usefulness and their variants have persisted in their relevance in the contemporary AI landscape with nuanced manifestations (e.g., new designs and features) and expectations from users. Their mission-critical role has been exemplified through research in the domain of usability, a subset of user experience research that focuses on promoting effectiveness, efficiency, and satisfaction in a specified context of technology use.
Regarding the latter effects, user control and empowerment have been explored through the decades from varied perspectives ranging from Psychology to Information Systems (Kim & Gupta, Citation2014; Psoinos et al., Citation2000; Vatanasombut et al., Citation2008). User empowerment was defined as an integrative motivational concept formed by four cognitive task assessments—user autonomy, computer self-efficacy, intrinsic motivation, and perceived usefulness—reflecting a user’s orientation to their technology-facilitated work (Doll et al., Citation2003). The link between user empowerment and effective IT use for problem-solving and decision support is well-documented in the literature (Holsapple & Sena, Citation2005; Miah et al., Citation2019; Sehgal & Stewart, Citation2004). Hence, it should come as no surprise that our literature review revealed tensions related to human agency and technology usability. While the tradeoff between empowerment gains (e.g., as a result of improved user control) and process loss (e.g., due to extra human effort and coordination) is an ongoing and unresolved topic (Mills & Ungson, Citation2003; Schneider et al., Citation2018), we expect the SA perspective to be useful in enhancing user empowerment by facilitating all four dimensions. For example, enhancing human agency should improve user autonomy and self-efficacy while facilitating user confidence and reducing perceived complexity would enhance users’ intrinsic motivation and perceived usefulness.
The debate of whether AI would replace humans or play an augmentation (and even amplification role) is still ongoing. We take the stance that HCAI is an appropriate term because it places the human truly at the core of the AI’s consideration: on the one hand, it considers the potential of AI in automating, enhancing, augmenting, and amplifying human performance; on the other, it considers the design of AI such that the user’s experience with it is optimized and likely to yield acceptance and ongoing use with utilization rates. Whereas the HCAI view is accompanied by various emerging tensions between human users and intelligent agents, the adverse effects can be mitigated if system usability is improved, and in the meantime, users can develop a proper mental model to understand the system, process, and the situation. The usefulness of the SA perspective is evident here as it creates a structured and shared space to allow both human users and intelligent agents to meet their informational requirements and reach decisional empowerment. Human users and intelligent agents are not viewed as competing entities under the SA paradigm, and collaboration among the two parties is possible for creating a hybrid human-machine learning system (Lyytinen et al., Citation2021).
Disclosure statement
No potential conflict of interest was reported by the author(s).
Additional information
Funding
Notes on contributors
Jinglu Jiang
Jinglu Jiang is an assistant professor of MIS at Binghamton University, School of Management. Her research focuses on emerging technologies, human-AI interactions, and information diffusion on digital platforms. She has published in various journals, including MIS Quarterly, MIT Sloan Management Review, Information Systems Frontiers, and International Journal of Human-Computer Studies.
Alexander J. Karran
Alexander J. Karran is an AI research associate at Tech3lab HEC Montréal. He holds a Ph.D. in Psychology from Liverpool John Moores University United Kingdom. His research orbits around physiologically adaptive systems using algorithmic and machine learning approaches applied in the automotive, aerospace and A.I. decision support domains.
Constantinos K. Coursaris
Constantinos K. Coursaris is Associate Professor of Information Technology, Director of M.Sc. User Experience, and Co-Director of Tech3Lab at HEC Montréal. His research has been financially supported in excess of $4 million and has been published in more than 100 articles in peer-reviewed journals and conference proceedings.
Pierre-Majorique Léger
Pierre-Majorique Léger is the senior chairholder of the NSERC Industrial Research Chair in UX and he is a Full professor of IT at HEC Montreal, co-director of Tech3lab and director of ERPsim Lab. He is the author of over 100 scientific articles, he holds 10 invention patents.
Joerg Beringer
Joerg Beringer is Chief Design Officer at Blue Yonder. He is leading the UX organization and experience strategy of Blue Yonder’s cloud platform and solutions. Joerg collaborates with the academic community to innovate on new experience paradigms for enterprise software (e.g., end-user development, UX of intelligent systems, and situational workspaces).
Notes
1 Although informational hierarchy may exist, we do not claim that the three levels of SA are hierarchical.
References
- Agrawal, R., Wright, T. J., Samuel, S., Zilberstein, S., & Fisher, D. L. (2017). Effects of a change in environment on the minimum time to situation awareness in transfer of control scenarios. Transportation Research Record: Journal of the Transportation Research Board, 2663(1), 126–133. https://doi.org/10.3141/2663-16
- Alsheiabni, S., Cheung, Y., & Messom, C. (2019, August 15–17). Factors inhibiting the adoption of artificial intelligence at organizational-level: A preliminary investigation [Paper presentation]. Americas Conference on Information Systems, Cancun, Mexico.
- Bainbridge, L. (1983). Ironies of automation (Analysis, design and evaluation of man–machine systems (pp. 129–135). Elsevier.
- Berberian, B., Sarrazin, J.-C., Le Blaye, P., & Haggard, P. (2012). Automation technology and sense of control: A window on human agency. Plos One, 7(3), e34075. https://doi.org/10.1371/journal.pone.0034075
- Bhaskara, A., Skinner, M., & Loft, S. (2020). Agent transparency: A review of current theory and evidence. IEEE Transactions on Human-Machine Systems, 50(3), 215–224. https://doi.org/10.1109/THMS.2020.2965529
- Bonchev, D., & Rouvray, D. H. (2003). Complexity: Introduction and fundamentals (Vol. 7). CRC Press.
- Bond, R. R., Novotny, T., Andrsova, I., Koc, L., Sisakova, M., Finlay, D., Guldenring, D., McLaughlin, J., Peace, A., McGilligan, V., Leslie, S. E. P. J., Wang, H., & Malik, M. (2018). Automation bias in medicine: The influence of automated diagnoses on interpreter accuracy and uncertainty when reading electrocardiograms. Journal of Electrocardiology, 51(6), S6–S11. https://doi.org/10.1016/j.jelectrocard.2018.08.007
- Bonneau, G.-P., Hege, H.-C., Johnson, C. R., Oliveira, M. M., Potter, K., Rheingans, P., & Schultz, T. (2014). Overview and state-of-the-art of uncertainty visualization (Scientific Visualization) (pp. 3–27). Springer.
- Bowden, V. K., Griffiths, N., Strickland, L., & Loft, S. (2021). Detecting a single automation failure: The impact of expected (but not experienced) automation reliability. Human Factors: The Journal of the Human Factors and Ergonomics Society, 001872082110371. https://doi.org/10.1177/00187208211037188
- Carroll, J. M. (1997). Human-computer interaction: Psychology as a science of design. Annual Review of Psychology, 48(1), 61–83. https://doi.org/10.1146/annurev.psych.48.1.61
- Chen, J., & Barnes, M. J. (2015, October 9–12). Agent transparency for human-agent teaming effectiveness [Paper presentation]. 2015 IEEE International Conference on Systems, Man, and Cybernetics, Hong Kong, China.
- Chen, J., Lakhmani, S. G., Stowers, K., Selkowitz, A. R., Wright, J. L., & Barnes, M. (2018). Situation awareness-based agent transparency and human-autonomy teaming effectiveness. Theoretical Issues in Ergonomics Science, 19(3), 259–282. https://doi.org/10.1080/1463922X.2017.1315750
- Chen, J. Y., Procci, K., Boyce, M., Wright, J., Garcia, A., & Barnes, M. (2014). Situation awareness-based agent transparency. US. Army Research Laboratory.
- Colombo, E., Mercorio, F., & Mezzanzanica, M. (2019). AI meets labor market: Exploring the link between automation and skills. Information Economics and Policy, 47, 27–37. https://doi.org/10.1016/j.infoecopol.2019.05.003
- Cummings, M. L., & Guerlain, S. (2007). Developing operator capacity estimates for supervisory control of autonomous vehicles. Human Factors: The Journal of the Human Factors and Ergonomics Society, 49(1), 1–15. http://proxy.binghamton.edu/login?url=https://search.ebscohost.com/login.aspx?direct=true&db=a9h&AN=24622895&site=ehost-live https://doi.org/10.1518/001872007779598109
- D’Aniello, G., Loia, V., & Orciuoli, F. (2019). An adaptive system based on situation awareness for goal-driven management in container terminals. IEEE Intelligent Transportation Systems Magazine, 11(4), 126–136. https://doi.org/10.1109/MITS.2019.2939137
- Davis, F. D., Bagozzi, R. P., & Warshaw, P. R. (1989). User acceptance of computer technology: A comparison of two theoretical models. Management Science, 35(8), 982–1003. https://doi.org/10.1287/mnsc.35.8.982
- De Bruyn, A., Viswanathan, V., Beh, Y. S., Brock, J. K. U., & Von Wangenheim, F. (2020). Artificial intelligence and marketing: Pitfalls and opportunities. Journal of Interactive Marketing, 51(1), 91–105. https://doi.org/10.1016/j.intmar.2020.04.007
- de Visser, E. J., Pak, R., & Shaw, T. H. (2018). From “automation’ to “autonomy’: The importance of trust repair in human-machine interaction. Ergonomics, 61(10), 1409–1427. https://doi.org/10.1080/00140139.2018.1457725
- Dequech, D. (2011). Uncertainty: A typology and refinements of existing concepts. Journal of Economic Issues, 45(3), 621–640. https://doi.org/10.2753/JEI0021-3624450306
- Doll, W. J., Deng, X., & Metts, G. A. (2003). User empowerment in computer-mediated work [Paper presentation]. Proceedings of ISOneWorld Conference , Las Vegas, NV, USA.
- Endsley, M. (1995). Toward a theory of situation awareness in dynamic systems. Human Factors: The Journal of the Human Factors and Ergonomics Society, 37(1), 32–64. https://doi.org/10.1518/001872095779049543
- Endsley, M. R. (2000). Theoretical underpinnings of situation awareness: A critical review. In M. R. Endsley & D. J. Garland (Eds.), Situation awareness analysis and measurement. Lawrence Erlbaum.
- Endsley, M. R. (2016). Designing for situation awareness: An approach to user-centered design. CRC press.
- Endsley, M. R., & Garland, D. J. (2000). Situation awareness analysis and measurement. CRC Press.
- Endsley, M. R., & Kaber, D. B. (1999). Level of automation effects on performance, situation awareness and workload in a dynamic control task. Ergonomics, 42(3), 462–492. https://doi.org/10.1080/001401399185595
- Endsley, M. R., & Kiris, E. O. (1995). The out-of-the-loop performance problem and level of control in automation. Human Factors: The Journal of the Human Factors and Ergonomics Society, 37(2), 381–394. https://doi.org/10.1518/001872095779064555
- Engen, V., Pickering, J. B., & Walland, P. (2016). Machine agency in human-machine networks; impacts and trust implications [Paper presentation]. International Conference on Human-Computer Interaction, Toronto, Canada.
- Engin, A., & Vetschera, R. (2017). Information representation in decision making: The impact of cognitive style and depletion effects. Decision Support Systems, 103, 94–103. https://doi.org/10.1016/j.dss.2017.09.007
- Fan, W., Liu, J., Zhu, S., & Pardalos, P. M. (2020). Investigating the impacting factors for the healthcare professionals to adopt artificial intelligence-based medical diagnosis support system (AIMDSS). Annals of Operations Research, 294(1–2), 567–592. https://doi.org/10.1007/s10479-018-2818-y
- Ferraro, J. C., & Mouloua, M. (2021). Effects of automation reliability on error detection and attention to auditory stimuli in a multi-tasking environment. Applied Ergonomics, 91, 103303. https://doi.org/10.1016/j.apergo.2020.103303
- Freitas, A. L., Clark, S. L., Kim, J. Y., & Levy, S. R. (2009). Action-construal levels and perceived conflict among ongoing goals: Implications for positive affect. Journal of Research in Personality, 43(5), 938–941. https://doi.org/10.1016/j.jrp.2009.05.006
- Frensch, P. A. (1998). One concept, multiple meanings: On how to define the concept of implicit learning. In Handbook of implicit learning (pp. 47–104). Sage.
- Gillath, O., Ai, T., Branicky, M. S., Keshmiri, S., Davison, R. B., & Spaulding, R. (2021). Attachment and trust in artificial intelligence. Computers in Human Behavior, 115, 106607. https://doi.org/10.1016/j.chb.2020.106607
- Glikson, E., & Woolley, A. W. (2020). Human trust in artificial intelligence: review of empirical research. Academy of Management Annals, 14(2), 627–660. https://doi.org/10.5465/annals.2018.0057
- Guo, J., Tao, D., & Yang, C. (2019). The effects of continuous conversation and task complexity on usability of an AI-based conversational agent in smart home environments [Paper presentation]. International Conference on Man-Machine-Environment System Engineering, Shanghai, China.
- Guzman, A. L., & Lewis, S. C. (2020). Artificial intelligence and communication: A Human–Machine Communication research agenda. New Media & Society, 22(1), 70–86. https://doi.org/10.1177/1461444819858691
- Habib, L., Pacaux-Lemoine, M., & Millot, P. (2018, October 7–10). Human-robots team cooperation in crisis management mission [Paper presentation]. 2018 IEEE International Conference on Systems, Man, and Cybernetics (SMC). https://doi.org/10.1109/SMC.2018.00545
- Hancock, J. T., Naaman, M., & Levy, K. (2020). AI-mediated communication: Definition, research agenda, and ethical considerations. Journal of Computer-Mediated Communication, 25(1), 89–100. https://doi.org/10.1093/jcmc/zmz022
- Hancock, P. A., Billings, D. R., Schaefer, K. E., Chen, J. Y. C., de Visser, E. J., & Parasuraman, R. (2011). A meta-analysis of factors affecting trust in human-robot interaction. Human Factors, 53(5), 517–527. https://doi.org/10.1177/0018720811417254
- Hjalmdahl, M., Krupenia, S., & Thorslund, B. (2017). Driver behaviour and driver experience of partial and fully automated truck platooning - a simulator study. European Transport Research Review, 9(1), 8. https://doi.org/10.1007/s12544-017-0222-3
- Hoff, K. A., & Bashir, M. (2015). Trust in automation: Integrating empirical evidence on factors that influence trust. Human Factors, 57(3), 407–434. https://doi.org/10.1177/0018720814547570
- Holsapple, C. W., & Sena, M. P. (2005). ERP plans and decision-support benefits. Decision Support Systems, 38(4), 575–590. https://doi.org/10.1016/j.dss.2003.07.001
- Hutchins, E. (1995a). Cognition in the wild. MIT press.
- Hutchins, E. (1995b). How a cockpit remembers its speeds. Cognitive Science, 19(3), 265–288. https://doi.org/10.1207/s15516709cog1903_1
- Ilbeygi, M., & Kangavari, M. R. (2018). Comprehensive architecture for intelligent adaptive interface in the field of single-human multiple-robot interaction. ETRI Journal, 40(4), 483–498. https://doi.org/10.4218/etrij.2017-0294
- Janssen, C. P., Iqbal, S. T., Kun, A. L., & Donker, S. F. (2019). Interrupted by my car? Implications of interruption and interleaving research for automated vehicles. International Journal of Human-Computer Studies, 130, 221–233. https://doi.org/10.1016/j.ijhcs.2019.07.004
- Jiang, Z., & Benbasat, I. (2007). The effects of presentation formats and task complexity on online consumers’ product understanding. MIS Quarterly, 31(3), 475–500. https://doi.org/10.2307/25148804
- Kanal, L. N., & Lemmer, J. F. (2014). Uncertainty in artificial intelligence. Elsevier.
- Kang, S., Kim, A. R., & Seong, P. H. (2017). Empirical verification for application of Bayesian inference in situation awareness evaluations. Annals of Nuclear Energy, 102, 106–115. https://doi.org/10.1016/j.anucene.2016.12.008
- Keil, M., Beranek, P. M., & Konsynski, B. R. (1995). Usefulness and ease of use: Field study evidence regarding task considerations. Decision Support Systems, 13(1), 75–91. https://doi.org/10.1016/0167-9236(94)E0032-M
- Kim, H.-W., & Gupta, S. (2014). A user empowerment approach to information systems infusion. IEEE Transactions on Engineering Management, 61(4), 656–668. https://doi.org/10.1109/TEM.2014.2354693
- Kim, T. W., & Duhachek, A. (2020). Artificial Intelligence and persuasion: A construal-level account. Psychological Science, 31(4), 363–380. https://doi.org/10.1177/0956797620904985
- Kläs, M., & Vollmer, A. M. (2018). Uncertainty in machine learning applications: A practice-driven classification of uncertainty [Paper presentation]. International Conference on Computer Safety, Reliability, and Security, Västerås, Sweden.
- Kolbjørnsrud, V., Amico, R., & Thomas, R. J. (2017). Partnering with AI: How organizations can win over skeptical managers. Strategy & Leadership, 45(1), 37–43. https://doi.org/10.1108/SL-12-2016-0085
- Kruger, K., Schade, U., & Ziegler, J. (2008, June 30–July 3). Uncertainty in the fusion of information from multiple diverse sources for situation awareness [Paper presentation]. 2008 11th International Conference on Information Fusion, Cologne, Germany.
- Kunze, A., Summerskill, S. J., Marshall, R., & Filtness, A. J. (2019). Automation transparency: implications of uncertainty communication for human-automation interaction and interfaces. Ergonomics, 62(3), 345–360. https://doi.org/10.1080/00140139.2018.1547842
- Le Goff, K., Rey, A., Haggard, P., Oullier, O., & Berberian, B. (2018). Agency modulates interactions with automation technologies. Ergonomics, 61(9), 1282–1297. https://doi.org/10.1080/00140139.2018.1468493
- Le, N. T., Rao Hill, S., & Troshani, I. (2022). Perceived control and perceived risk in self-service technology recovery. Journal of Computer Information Systems, 62(1), 164–173. https://doi.org/10.1080/08874417.2020.1756533
- Lee, S. J. (2019). The role of construal level in message effects research: A review and future directions. Communication Theory, 29(3), 231–338. https://doi.org/10.1093/ct/qty030
- Li, K., & Wieringa, P. A. (2000). Understanding perceived complexity in human supervisory control. Cognition. Technology & Work, 2(2), 75–88. https://doi.org/10.1007/s101110050029
- Liberman, N., & Trope, Y. (1998). The role of feasibility and desirability considerations in near and distant future decisions: A test of temporal construal theory. Journal of Personality and Social Psychology, 75(1), 5–18. https://doi.org/10.1037/0022-3514.75.1.5
- Liu, B. (2021). In AI we trust? Effects of agency locus and transparency on uncertainty reduction in human–AI interaction. Journal of Computer-Mediated Communication, 26(6), 384–402. https://doi.org/10.1093/jcmc/zmab013
- Liu, P., & Li, Z. (2012). Task complexity: A review and conceptualization framework. International Journal of Industrial Ergonomics, 42(6), 553–568. https://doi.org/10.1016/j.ergon.2012.09.001
- Lyytinen, K., Nickerson, J. V., & King, J. L. (2021). Metahuman systems = humans + machines that learn. Journal of Information Technology, 36(4), 427–445. https://doi.org/10.1177/0268396220915917
- Mansikka, H., Virtanen, K., & Harris, D. (2019). Dissociation between mental workload, performance, and task awareness in pilots of high performance aircraft. IEEE Transactions on Human-Machine Systems, 49(1), 1–9. https://doi.org/10.1109/THMS.2018.2874186
- Marquez, J. J., & Cummings, M. L. (2008). Design and evaluation of path planning decision support for planetary surface exploration. Journal of Aerospace Computing Information and Communication, 5(3), 57–71. https://doi.org/10.2514/1.26248
- Masters, R., Poolton, J. M., Maxwell, J. P., & Raab, M. (2008). Implicit motor learning and complex decision making in time-constrained environments. Journal of Motor Behavior, 40(1), 71–79. https://doi.org/10.3200/JMBR.40.1.71-80
- McCarthy, J. (2007). From here to human-level AI. Artificial Intelligence, 171(18), 1174–1182. https://doi.org/10.1016/j.artint.2007.10.009
- Merritt, S. M., Ako-Brew, A., Bryant, W. J., Staley, A., McKenna, M., Leone, A., & Shirase, L. (2019). Automation-induced complacency potential: Development and validation of a new scale. Frontiers in Psychology, 10, 225. https://doi.org/10.3389/fpsyg.2019.00225
- Miah, S. J., Gammack, J. G., & McKay, J. (2019). A Metadesign theory for Tailorable decision support. Journal of the Association for Information Systems, 20(5), 4. https://doi.org/10.17705/1jais.00544
- Miller, C. A., & Parasuraman, R. (2007). Designing for flexible interaction between humans and automation: Delegation interfaces for supervisory control. Human Factors, 49(1), 57–75. https://doi.org/10.1518/001872007779598037
- Miller, D., Sun, A., & Ju, W. (2014, October 5–8). Situation awareness with different levels of automation [Paper presentation]. 2014 IEEE International Conference on Systems, Man, and Cybernetics (SMC), San Diego, CA.
- Miller, T. (2019). Explanation in artificial intelligence: Insights from the social sciences. Artificial Intelligence, 267, 1–38. https://doi.org/10.1016/j.artint.2018.07.007
- Mills, P. K., & Ungson, G. R. (2003). Reassessing the limits of structural empowerment: Organizational constitution and trust as controls. Academy of Management Review, 28(1), 143–153. https://doi.org/10.5465/amr.2003.8925254
- Mohseni, S., Zarei, N., & Ragan, E. D. (2021). A multidisciplinary survey and framework for design and evaluation of explainable AI systems. ACM Transactions on Interactive Intelligent Systems, 11(3–4), 1–45. https://doi.org/10.1145/3387166
- Nadkarni, S., & Gupta, R. (2007). A task-based model of perceived website complexity. MIS Quarterly, 31(3), 501–524. https://doi.org/10.2307/25148805
- Ng, K. K., Chen, C.-H., Lee, C. K., Jiao, J. R., & Yang, Z.-X. (2021). A systematic literature review on intelligent automation: Aligning concepts from theory, practice, and future perspectives. Advanced Engineering Informatics, 47, 101246. https://doi.org/10.1016/j.aei.2021.101246
- Okoli, C. (2015). A guide to conducting a standalone systematic literature review. Communications of the Association for Information Systems, 37(1), 43. https://doi.org/10.17705/1CAIS.03743
- Onnasch, L., Wickens, C. D., Li, H., & Manzey, D. (2014). Human performance consequences of stages and levels of automation: An integrated meta-analysis. Human Factors, 56(3), 476–488. https://doi.org/10.1177/0018720813501549
- Padgham, L., & Winikoff, M. (2005). Developing intelligent agent systems: A practical guide (Vol. 13). John Wiley & Sons.
- Page, T. (2009). Feature creep and usability in consumer electronic product design. International Journal of Product Development, 9(4), 406–428. https://doi.org/10.1504/IJPD.2009.027474
- Parasuraman, R., & Manzey, D. H. (2010). Complacency and bias in human use of automation: An attentional integration. Human Factors, 52(3), 381–410. https://doi.org/10.1177/0018720810376055
- Parasuraman, R., Molloy, R., & Singh, I. L. (1993). Performance consequences of automation-induced’complacency. The International Journal of Aviation Psychology, 3(1), 1–23. https://doi.org/10.1207/s15327108ijap0301_1
- Park, D., Yoon, W. C., & Lee, U. (2020). Cognitive states matter: Design guidelines for driving situation awareness in smart vehicles. Sensors (14248220), 20(10), 2978–2978. https://doi.org/10.3390/s20102978
- Paulius, D., & Sun, Y. (2019). A survey of knowledge representation in service robotics. Robotics and Autonomous Systems, 118, 13–30. https://doi.org/10.1016/j.robot.2019.03.005
- Prangnell, N., & Wright, D. (2015). The robots are coming (No. 2304459804577). Deloitte report. https://www2.deloitte.com/uk/en/pages/finance/articles/robots-coming-global-business-services.html
- Psoinos, A., Kern, T., & Smithson, S. (2000). An exploratory study of information systems in support of employee empowerment. Journal of Information Technology, 15(3), 211–230. https://doi.org/10.1177/026839620001500304
- Rai, A. (2020). Explainable AI: From black box to glass box. Journal of the Academy of Marketing Science, 48(1), 137–141. https://doi.org/10.1007/s11747-019-00710-5
- Rajabiyazdi, F., & Jamieson, G. A. (2020, October 11–14). A review of transparency (seeing-into) models [Paper presentation]. 2020 IEEE International Conference on Systems, Man, and Cybernetics (SMC), Toronto, Ontario, Canada. https://doi.org/10.1109/SMC42975.2020.9282970
- Salmon, P. M., Stanton, N. A., Walker, G. H., Jenkins, D., Ladva, D., Rafferty, L., & Young, M. (2009). Measuring Situation Awareness in complex systems: Comparison of measures study. International Journal of Industrial Ergonomics, 39, 490–500. https://doi.org/10.1016/j.ergon.2008.10.010
- Schaefer, K. E., Straub, E. R., Chen, J. Y., Putney, J., & Evans, A. W. (2017). Communicating intent to develop shared situation awareness and engender trust in human-agent teams. Cognitive Systems Research, 46, 26–39. https://doi.org/10.1016/j.cogsys.2017.02.002
- Schleiffer, R. (2005). An intelligent agent model. European Journal of Operational Research, 166(3), 666–693. https://doi.org/10.1016/j.ejor.2004.03.039
- Schmidt, A. (2020). Interactive human centered artificial intelligence: A definition and research challenges [Paper presentation]. Proceedings of the International Conference on Advanced Visual Interfaces, Island of Ischia, Italy. https://doi.org/10.1145/3399715.3400873
- Schneider, H., Eiband, M., Ullrich, D., & Butz, A. (2018). Empowerment in HCI-A survey and framework [Paper presentation]. Proceedings of the 2018 CHI Conference on Human Factors in Computing Systems.
- Schoonderwoerd, T. A. J., Jorritsma, W., Neerincx, M. A., & van den Bosch, K. (2021). Human-centered XAI: Developing design patterns for explanations of clinical decision support systems. International Journal of Human-Computer Studies, 154, 102684. https://doi.org/10.1016/j.ijhcs.2021.102684
- Sehgal, R., & Stewart, G. (2004). Exploring the relationship between user empowerment and enterprise system success measures. AMCIS 2004 Proceedings, 15, 91–98. https://aisel.aisnet.org/amcis2004/15
- Sheridan, T. B., & Verplank, W. L. (1978). Human and computer control of undersea teleoperators. https://apps.dtic.mil/sti/citations/ADA057655
- Shneiderman, B. (1980). Software psychology: Human factors in computer and information systems. Winthrop Inc.
- Shneiderman, B. (2020). Human-centered artificial intelligence: Reliable, safe & trustworthy. International Journal of Human-Computer Interaction, 36(6), 495–504. https://doi.org/10.1080/10447318.2020.1741118
- Shneiderman, B. (2021). Human-centered AI: A new synthesis [Paper presentation].IFIP Conference on Human-Computer Interaction, Bari, Italy.
- Singh, I. L., Molloy, R., & Parasuraman, R. (1993). Automation-induced “complacency”: Development of the complacency-potential rating scale. The International Journal of Aviation Psychology, 3(2), 111–122. https://doi.org/10.1207/s15327108ijap0302_2
- Sivadasan, S., Efstathiou, J., Calinescu, A., & Huatuco, L. H. (2006). Advances on measuring the operational complexity of supplier–customer systems. European Journal of Operational Research, 171(1), 208–226. https://doi.org/10.1016/j.ejor.2004.08.032
- Skraaning, G., & Jamieson, G. A. (2021). Human performance benefits of the automation transparency design principle: Validation and variation. Human Factors, 63(3), 379–401. https://doi.org/10.1177/0018720819887252
- Smith, P. J., & Baumann, E. (2020, October 11–15). Human-automation teaming: Unintended consequences of automation on user performance [Paper presentation]. 2020 AIAA/IEEE 39th Digital Avionics Systems Conference (DASC), San Antonio, TX.
- Sonoda, K., & Wada, T. (2017). Displaying system situation awareness increases driver trust in automated driving. IEEE Transactions on Intelligent Vehicles, 2(3), 185–193. https://doi.org/10.1109/TIV.2017.2749178
- Sparrow, B., Liu, J., & Wegner, D. M. (2011). Google effects on memory: Cognitive consequences of having information at our fingertips. Science (New York, N.Y.), 333(6043), 776–778.
- Stanton, N. A., Stewart, R., Harris, D., Houghton, R. J., Baber, C., McMaster, R., Salmon, P., Hoyle, G., Walker, G., Young, M. S., Linsell, M., Dymott, R., & Green, D. (2006). Distributed situation awareness in dynamic systems: Theoretical development and application of an ergonomics methodology. Ergonomics, 49(12–13), 1288–1311. https://doi.org/10.1080/00140130600612762
- Stowers, K., Kasdaglis, N., Rupp, M. A., Newton, O. B., Chen, J. Y. C., & Barnes, M. (2020). The IMPACT of agent transparency on human performance. IEEE Transactions on Human-Machine Systems, 50(3), 245–253. https://doi.org/10.1109/THMS.2020.2978041
- Sundar, S. S. (2020). Rise of machine agency: A framework for studying the psychology of human–AI interaction (HAII). Journal of Computer-Mediated Communication, 25(1), 74–88. https://doi.org/10.1093/jcmc/zmz026
- Te’eni, D. (1989). Determinants and consequences of perceived complexity in human‐computer interaction. Decision Sciences, 20(1), 166–181. https://doi.org/10.1111/j.1540-5915.1989.tb01405.x
- Tegarden, D. P., Sheetz, S. D., & Monarchi, D. E. (1995). A software complexity model of object-oriented systems. Decision Support Systems, 13(3–4), 241–262. https://doi.org/10.1016/0167-9236(93)E0045-F
- Trope, Y., & Liberman, N. (2010). Construal-level theory of psychological distance. Psychological Review, 117(2), 440–463. https://doi.org/10.1037/a0018963
- Trope, Y., Liberman, N., & Wakslak, C. (2007). Construal levels and psychological distance: Effects on representation, prediction, evaluation, and behavior. Journal of Consumer Psychology: The Official Journal of the Society for Consumer Psychology, 17(2), 83–95. https://doi.org/10.1016/S1057-7408(07)70013-X
- van der Waa, J., Schoonderwoerd, T., van Diggelen, J., & Neerincx, M. (2020). Interpretable confidence measures for decision support systems. International Journal of Human-Computer Studies, 144, 102493. https://doi.org/10.1016/j.ijhcs.2020.102493
- Vatanasombut, B., Igbaria, M., Stylianou, A. C., & Rodgers, W. (2008). Information systems continuance intention of web-based applications customers: The case of online banking. Information & Management, 45(7), 419–428. https://doi.org/10.1016/j.im.2008.03.005
- Venkatesh, V., Morris, M. G., Davis, G. B., & Davis, F. D. (2003). User acceptance of information technology: Toward a unified view. MIS Quarterly, 27(3), 425–478. [Database] https://doi.org/10.2307/30036540
- Venkatesh, V., Thong, J. Y., & Xu, X. (2012). Consumer acceptance and use of information technology: extending the unified theory of acceptance and use of technology. MIS Quarterly, 36(1), 157–178. https://doi.org/10.2307/41410412
- Vial, G., Jiang, J., Giannelia, T., & Cameron, A.-F. (2021). The data problem stalling AI. MIT Sloan Management Review, 62(2), 47–53.
- Vicente, K. J., & Rasmussen, J. (1992). Ecological interface design: Theoretical foundations. IEEE Transactions on Systems, Man, and Cybernetics, 22(4), 589–606. https://doi.org/10.1109/21.156574
- Villaren, T., Madier, C., Legras, F., Leal, A., Kovacs, B., & Coppin, G. (2010). Towards a method for context-dependent allocation of functions [Paper presentation]. Proceedings of the 2nd Conference on Human Operating Unmanned Systems (HUMOUS’10), Toulouse, France.
- Windt, K., Philipp, T., & Böse, F. (2008). Complexity cube for the characterization of complex production systems. International Journal of Computer Integrated Manufacturing, 21(2), 195–200. https://doi.org/10.1080/09511920701607725
- Woods, D. D. (2018). Decomposing automation: Apparent simplicity, real complexity. In Automation and human performance: Theory and applications (pp. 3–17). CRC Press.
- Wu, J., & Shang, S. (2020). Managing uncertainty in AI-enabled decision making and achieving sustainability. Sustainability, 12(21), 8758. https://doi.org/10.3390/su12218758
- Xu, W. (2021). From automation to autonomy and autonomous vehicles: Challenges and opportunities for human-computer interaction. Interactions, 28(1), 48–53. https://doi.org/10.1145/3434580
- Yamani, Y., Long, S. K., & Itoh, M. (2020). Human-automation trust to technologies for naive users amidst and following the COVID-19 pandemic. Human Factors, 62(7), 1087–1094. https://doi.org/10.1177/0018720820948981
- Zadeh, L. A. (1986). Is probability theory sufficient for dealing with uncertainty in AI: A negative view. In Machine intelligence and pattern recognition (Vol. 4, pp. 103–116). Elsevier.
- Zafari, S., & Koeszegi, S. T. (2021). Attitudes toward attributed agency: Role of perceived control. International Journal of Social Robotics, 13, 2071–2080. https://doi.org/10.1007/s12369-020-00672-7
- Ziefle, M. (2002). The influence of user expertise and phone complexity on performance, ease of use and learnability of different mobile phones. Behaviour & Information Technology, 21(5), 303–311. https://doi.org/10.1080/0144929021000048538