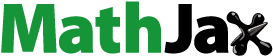
Abstract
This study verified how consumers determine their willingness to provide personal information for the Internet of Things (IoT) services and the role of procedural fairness of information disclosure on that behavior using the privacy calculus theory. We used survey data collected from 2,292 consumers with experience in using IoT services and performed path and parallel mediation analyses. The result indicates that procedural fairness is an important variable for explaining consumers’ decision-making about providing their personal information, despite the skepticism surrounding it within the IoT environment. Moreover, the role of trust in one’s service provider has increased in importance compared to that of privacy concerns regarding consumers’ personal information-disclosing behavior under everyday technology environments. Our findings highlight the implications that empirically verify the importance of building trust in service providers in a new technology environment such as IoT, and what role fair information statements may play in forming that trust.
1. Introduction
The contemporary market environment surrounding personal information and personalized services is rapidly changing. The exponential increase in the number of personalized services using consumers’ usage behavior data has resulted in numerous conveniences for consumers but also exposed them to greater privacy risks (Karwatzki et al., Citation2017; Li & Unger, Citation2012). Internet of Things (IoT), an innovative technology that connects and enables interaction among various devices, has intensified the risk of exposure to privacy because it is becoming increasingly difficult to recognize what devices do with our personal information that they collect, share, and process using unseen embedded sensors and networks (Federal Trade Commission, Citation2015). Under these circumstances, the existing models on information disclosure behavior, such as notice and choice, and the long-supported principle of personal information processing, are under threat.
For example, prior studies on the willingness to provide personal information (WPPI) have focused on the decision-making process through which consumers decide about providing their personal information in return for the service being provided. Previous studies have considered various variables as antecedents that determine the consumers’ WPPI, and the most important variable among them has been consumers’ privacy concern. However, privacy concerns are no longer sufficient to explain consumers’ information disclosure behavior in the recent technological environment (Kim et al., Citation2019; Nguyen et al., Citation2008; Spiekermann et al., Citation2001; Xu & Wang, Citation2022). Therefore, renowned scholars like Dinev and Hart (Citation2006a), who proposed the extended privacy calculus theory, and Norberg et al. (Citation2007), who proposed the privacy paradox theory, have begun emphasizing the role of trust in consumer’s information disclosure behavior. This tendency has continued in recent studies on IoT services (Koohang et al., Citation2022; Pal et al., Citation2021; Schomakers et al., Citation2022; Xu & Wang, Citation2022).
Meanwhile, as the collection and use of personal information becomes routinized, a debate has ensued about the usefulness of the principle called notice and choice, which is “the foundational principle underlying the regulation of privacy issues” (Rothchild, Citation2017, p. 559). The Federal Trade Commission of the United States mentioned that “notice and choice is challenging under the IoT environment because of the ubiquity of data collection and the practical obstacles to providing information without a user interface” (Federal Trade Commission [FTC], Citation2015, p. 5). Sloan and Warner (Citation2014) insisted that skepticism about privacy notices has increased because consumers’ self-determination of their personal information has continued to change and become an unrealistic ideal notion. Researchers have warned that consumer consent could just be a type of responsibility evasion for service providers since, after the procurement of initial consent, the information is automatically collected and stored in ways consumers cannot recognize (Cranor, Citation2012; Rothchild, Citation2017).
However, notice and choice has historically served as an essential requirement to secure procedural fairness in utilizing consumers’ personal information (Culnan & Armstrong, Citation1999). The European Union’s General Data Protection Regulation (GDPR), which produces new leading privacy policies for the emerging data economy, also emphasizes providing procedural fairness and self-determination while seeking personal information (Utz et al., Citation2019). Furthermore, recent studies have confirmed that fair information practices and consumers’ perceived privacy control are effective in alleviating consumers’ privacy concerns and increasing their WPPI (Cichy et al., Citation2021; Koohang et al., Citation2022; Pal et al., Citation2021; Princi & Krämer, Citation2020; Zhu et al., Citation2021).
Against this background, we intended to empirically verify the impact of privacy concerns and trust on consumers’ WPPI, and the importance of procedural fairness of information disclosure in the everyday technological environment, such as that of IoT. Through this study, we hope to inform the IoT service providers of the factors they should consider to encourage consumers’ information disclosure intention for IoT services, and the role of procedural fairness in that process.
1.1. Theoretical background and literature review
1.1.1. Privacy calculus
In the process of receiving personalized service, the personal information provided by the consumer has non-monetary value; however, the consumer presents it to the service provider to obtain a personalized service that has monetary value (Milne & Gordon, Citation1993).
Consumers and service providers make a social contract, and consumers weigh the risks and benefits of providing personal information in return for receiving personalized services (Phelps et al., Citation2000). The notion of privacy calculus was first mentioned by Laufer and Wolfe (Citation1977), and the model was adopted in the context of transactions by Culnan and Armstrong (Citation1999). This theory was then used in numerous subsequent studies (e.g., Dienlin & Metzger, Citation2016; Kehr et al., Citation2015; Keith et al., Citation2013; Kim et al., Citation2019; Schomakers et al., Citation2022; Sun et al., Citation2015; Wilson & Valacich, Citation2012). The privacy calculus theory is considered to be one of the most convincing theories that explains how consumers make decisions to disclose their personal information.
However, the increasing use of the internet has been threatening the reputation of privacy calculus. In the everyday technology environment, it has become virtually impossible for consumers to refuse to provide their personal information. Consumers are in a situation where they have no choice but to provide personal information despite their concern about the risk to their privacy. Under these circumstances, consumers can no longer use the privacy concern as a critical antecedent reason to explain their personal information disclosure behavior. Therefore, researchers have been pouring out a plethora of claims to explain the contradictory privacy-related behaviors of consumers regarding privacy. Of these, particularly noteworthy ones have been the “extended privacy calculus theory” of Dinev and Hart (Citation2006a) and the “privacy paradox theory” of Norberg et al. (Citation2007).
Dinev and Hart (Citation2006a) applied the privacy calculus theory of Culnan and Armstrong (Citation1999) to internet transactions and tried to explain consumers’ contradictory behavior regarding the disclosure of personal information observed in the online environment. For this, the variable that the researchers emphasized was internet trust. Specifically, Dinev and Hart (Citation2006a) replaced perceived risk and benefit presented by Culnan and Armstrong (Citation1999) with privacy concern and internet trust as antecedent variables for WPPI. Norberg et al. (Citation2007) also emphasized the role of trust but tried to explain that role in actual information disclosure behavior, and not in the intention to disclose like Dinev and Hart (Citation2006a). Specifically, researchers believe that it is trust that determines actual disclosure behavior, although perceived risk still has a role as an antecedent of disclosure intention.
These two studies revealed antecedent variables for different dependent variables, namely intention and behavior. Moreover, they explained the contradictory behavior of consumers’ personal information disclosure decision and suggested that future researchers pay attention to the role of trust. The effect of this call was reflected in follow-up studies in which trust was examined as an important antecedent determinant of information disclosure intention and behavior. This trend was more prominent in new technology environments, such as social networking services (SNS) (Lo & Riemenschneider, Citation2010; Wang et al., Citation2016), IoT (Kim et al., Citation2019; Schomakers et al., Citation2022) and health information environments (Bansal et al., Citation2010; Citation2016; Chen et al., Citation2017). Those services cannot be used unless the consumer agrees to information collection and use.
Therefore, the privacy calculus theory initially focused on the balance between the benefits and risks of information disclosure. However, increasing prevalence of the collection and use of personal information has shifted its focus to the psychological conflict between privacy concerns and trust in a service provider that arises in the consumer’s mind. However, even amid the continuously changing variables used in the privacy calculus theory, it has persisted and been verified in various contexts as a theoretical basis for explaining information disclosure intention and behavior (Smith et al., Citation2011).
1.1.2. Procedural fairness
Procedural fairness of information disclosure decision refers to consumers’ ability to control overall decisions about their personal information (Greenberg & Folger, Citation1983) and the level of awareness or knowledge about information processing (Folger & Bies, Citation1989). It refers to consumers’ freedom to make informed choices based on adequately detailed and intelligible privacy statements, and a freely selectable system environment provided by the service provider.
Culnan and Armstrong (Citation1999), who applied the privacy calculus theory to the transaction field, emphasized procedural fairness as an important antecedent variable determining information disclosure intention. They found that when consumers were provided with fair information practice, privacy concern was no longer a significant variable in determining whether consumers would provide their personal information. Smith et al. (Citation2011) proposed the APCO model (Antecedents-Privacy Concerns-Outcomes) and defined privacy notice as an antecedent variable that increases trust. They insisted that the privacy concern will be mitigated when service providers earn consumers’ trust through procedural fairness.
In the real world, “notice and choice” is a general principle determining the procedural fairness of personal information collection and utilization. Privacy notice has been key in implementing that principle (Culnan & Armstrong, Citation1999). However, under everyday technology environments such as IoT, decisions about personal data usage are increasingly automated, and the principle of notice and choice has ensued (FTC, Citation2015). Solove (Citation2012) pointed out that the patriarchal privacy law and policies based on the notice and choice principle result in a consent dilemma, hindering consumers’ self-management of personal information. Similarly, Sloan and Warner (Citation2014) asserted “notice skepticism,” the notion that a standardized notice of privacy policy is meaningless, and Rothchild (Citation2017) warned that consent-based privacy policy could be used as a way for service providers to avoid legal responsibility.
As such, procedural fairness is a very important concept that determines an individual’s decision-making regarding personal information and the direction of privacy law and policy. Meanwhile, despite its theoretical importance, the effect of a privacy notice has not been sufficiently verified in previous studies (Smith et al., Citation2011) because of disagreements over the definition and virtualization of the good practice of privacy notice. However, since the initiation of research on procedural fairness, it has been repeatedly stated that it refers to the level of fairness perceived by consumers (Culnan & Bies, Citation2003). In other words, the level of procedural fairness can be measured empirically using the level of consumers’ privacy awareness, which is consumers’ level of awareness of the contents of the privacy statement. Following previous studies, we chose consumers’ awareness of the privacy statement, referred to as privacy awareness, as a measurement variable to estimate the level of procedural fairness.
2. Privacy calculus and procedural fairness in IoT environment
Studies on the suitability of the privacy calculus theory and the necessity of procedural fairness are continually featured from a new perspective in the new technological environment of IoT. First, even in the IoT environment, the privacy calculus theory is widely used as a powerful theory to explain the intention of consumers to provide personal information. However, the studies on this theory take two perspectives. First, some studies compare the benefits and risks of providing personal information for IoT services (Cichy et al., Citation2021; Kim et al., Citation2019; Kim & Choi, Citation2022; Pal et al., Citation2021). The other are studies that consider trust as another important variable explaining the behavior of providing personal information for IoT services (Koohang et al., Citation2022; Pal et al., 2021; Schomakers et al., Citation2022; Xu & Wang, Citation2022).
Studies on procedural fairness in the IoT environment are also divided into two perspectives: one are studies claiming that efforts to secure informed consent will become meaningless due to an automated personal information collection environment (Rothchild, Citation2017; Sloan & Warner, Citation2014). Meanwhile, some studies claim that even under the new technological environment, effective privacy notices (Zhu et al., Citation2021), privacy knowledge (Koohang et al., Citation2022), and privacy control (Pal et al., Citation2021; Princi & Krämer, Citation2020) will help form the intention to use IoT services and provide personal information ().
Table 1. Related research in an IoT environment.
2.1. Research design and hypotheses
The IoT service is a kind of basic technology that will rapidly change our lives in the future, although its level of influence is still in its infancy. In IoT service, which aims to provide automated services through device-to-device connection, personal information provided by consumers functions as a key resource for service development. Therefore, for the stable growth of the IoT technology market, a fair and legal way to collect more personal information is most important. If this is accurate, when and under what circumstances do consumers decide to provide more personal information about IoT services? Under the automated personal information collection environment such as IoT, will procedural fairness, or a fair information statement—which was considered a prerequisite for legitimate data transactions—become meaningless?
We designed this study to address the questions raised after reviewing previous studies. We posed three research questions and five hypotheses to empirically verify the relationship between consumer’s privacy calculus and procedural fairness under an IoT environment. The contents of our research questions, our hypotheses, and the earlier studies that we reviewed to develop our research model are presented below.
Research Question 1. How do the two construct variables of the privacy calculus theory, privacy concern and trust for one’s service provider, function under the everyday technology environment such as IoT? (Hypothesis 1, 2; Path analysis)
Research Question 2. How does the privacy awareness that is shaped by fair information statements presented by service providers affect the consumer’s privacy calculation behavior? (Hypothesis 3, 4, 5; Path analysis)
Research Question 3. If the mediating effect of privacy concern and trust for one’s service provider were statistically significant, how does the mediating effect of those two variables differ? (Parallel mediation analysis)
2.1.1. Privacy concern and willingness to provide personal information
Privacy concern means worry, fear, or anxiety that service providers may collect, provide, and use consumers’ personal information in an unfair way (McKnight et al., Citation2011). Privacy concern has attracted attention for a long time as the most important antecedent variable that determines consumers’ WPPI. However, the explanatory power of the variable is weakening under the technological environment where the collection and use of personal information is routine. As if reflecting these research trends, the empirical results of earlier studies on privacy concerns and WPPI have been contradictory. In certain cases, a statistically significant negative relationship has been confirmed between the two variables (Al-Jabri et al., Citation2019; Bansal et al., Citation2010; Citation2016; Baruh et al., Citation2017; Dinev & Hart, Citation2006a; Fernandes & Pereira, Citation2021; Kim & Choi, Citation2022; Lo & Riemenschneider, Citation2010; Princi & Krämer, Citation2020; Schomakers et al., Citation2022). However, while using everyday services such as making a credit card payment (Nguyen et al., Citation2008), transacting in e-commerce portals (Spiekermann et al., Citation2001; Xu & Wang, Citation2022), or using IoT (Kim et al., Citation2019), that routinely involves the collection and use of personal information, the relationship between the two variables has not been statistically significant. In this study, we judged that privacy concern is still an important variable that determines WPPI. For this reason and based on previous studies, we assumed that there would be a negative relationship between privacy concern and WPPI.
Hypothesis 1.
Privacy concerns are negatively related to the WPPI in IoT services.
2.1.2. Trust and willingness to provide personal information
Trust refers to the confidence that consumers have with their personal information being handled competently, reliably, and safely after submission to IoT service providers (Dinev & Hart, Citation2006a). The importance of trust began to draw attention as a new addition in the extended privacy calculus model that applied the privacy calculus theory to e-commerce transactions. Since then, trust has attracted attention as a new explanatory variable—a better predictor of consumers’ information disclosure behavior in an environment where the collection and use of personal information are routine. In previous studies, trust was the new antecedent relied upon for accurately explaining consumer information disclosure intention and behavior under the new technological environment, such as SNS (Lo & Riemenschneider, Citation2010; Wang et al., Citation2016), smart technologies including IoT (Kim et al., Citation2019; Schomakers et al., Citation2022), and health information (Bansal et al., Citation2010; Citation2016; Chen et al., Citation2017). Therefore, we selected consumers’ trust in the service provider as another explanatory variable for consumers’ WPPI to their IoT service. We also assumed there would be a positive relationship between consumers’ trust and consumers’ WPPI.
Hypothesis 2.
Trust in one’s service provider is positively related to the WPPI in IoT services.
2.1.3. Privacy awareness and other constructs
Privacy awareness refers to consumers’ general knowledge of privacy issues and policies (Borena et al., Citation2015; Brecht et al., Citation2012; Dinev & Hart, Citation2006b; Ermakova et al., Citation2014) or their understanding of the privacy statement presented by the service provider (Li et al., Citation2011; Malhotra et al., Citation2004; Xu & Gupta, Citation2009; Yun et al., Citation2014). Consumers’ privacy awareness is also measured in terms of their level of subjective awareness (Dinev & Hart, Citation2006b; Hong et al., Citation2016; Kuo & Talley, Citation2014; Malhotra et al., Citation2004; Morrison, Citation2013) and objective awareness based on their responses to true-or-false questions (Ermakova et al., Citation2014).
We defined privacy awareness as the IoT service consumer’s subjective awareness of the privacy statement provided while the consumer used the service to focus on their experience of using the IoT technology.
In this research, the level of consumer privacy awareness is regarded as an outcome variable that shows the extent of intelligibility and effective presentation of the privacy statement by the service provider (Langheinrich, Citation2001; Utz et al., Citation2019).
We relied on the results of previous studies to assume a negative relationship between privacy awareness and privacy concern (Andrade et al., Citation2002; Maseeh et al., Citation2021; Mousavizadeh et al., Citation2016; Nam et al., Citation2006), a positive relationship between privacy awareness and trust (Chen et al., Citation2017; Liu et al., Citation2005; Smith et al., Citation2011; Wang et al., Citation2004; Wu et al., Citation2012), and a positive relationship between privacy awareness and WPPI (Cichy et al., Citation2021; Meinert et al., Citation2006; Wang et al., Citation2004).
Hypothesis 3.
Consumer’s privacy awareness is negatively related to privacy concerns.
Hypothesis 4.
Consumer’s privacy awareness is positively related to WPPI to the IoT service.
Hypothesis 5.
Consumer’s privacy awareness is positively related to trust in the service provider.
In sum, to explain how consumers determine their intention to provide personal information for IoT services, we select consumer’s privacy concern, trust, and privacy awareness as antecedents of WPPI based on earlier reviewed studies. The construct variables are defined in , and their causal relationships are depicted in .
Table 2. Construct definitions.
2.1.4. Materials and methods
To verify the hypotheses included in our research model, we used data collected by the Korea Consumer Agency, which is the national agency of South Korea that formulates and improves the government’s consumer policies, supports their implementation, and plans new policies to resolve data privacy problems in the IoT environment.
The countrywide survey targeted adult consumers between the ages of 20 and 70 with experience in using IoT services, such as smart health bands, smart medical instruments, smart home services, internet protocol (IP) cameras, and vehicle telematics. The subjects were recruited from the online panel of Embrain, a Korean online research company. Since the purpose of the survey was to recruit users with experience in using IoT services, convenience sampling was chosen as the sampling method.
Written informed consent was obtained from the study participants with the statements about who, for why this study was conducted, and how the results of the survey would be used and protected. After that, the respondents were asked to respond to all the items in the questionnaire based on their experience with their most frequently or most recently used IoT device or service. After filtering out incomplete and unreasonable responses, 2,292 valid responses were selected. The percentages of male and female respondents were 60.5% and 39.5%, respectively. Of the respondents, 22.3% were in their 20s, 24.8% were in their 30s, 21.6% were in their 40s, 19.5% were in their 50s, and 11.9% were in their 60s ().
Table 3. Distribution of the sample (n = 2,292).
The measures for the five variables used in our research model were developed based on previous research on privacy calculus behavior in the IoT environment. First, modifying the research of Kowatsch and Maass (Citation2012), WPPI was measured using five items representing various levels of consumers’ intention to provide different types of information. These five items were personally identifiable information, appliance identifiable information, video information, location information, and health information that could be collected when consumers use IoT products. Second, the five items for measuring privacy concerns were developed by applying the theory put forward by Smith et al. (Citation1996). They suggested four dimensions of privacy concerns: collection, errors, unauthorized access, and the secondary use of information.
Following this, we developed three items to measure the level of consumers’ privacy concerns regarding unknown collection, unauthorized access and use, and secondary use—basically, addressing consumers’ concern of using or accessing the data for purposes other than the stated purpose. We also added a new item to indicate the level of technical difficulty in destroying the provided information in consideration of the new issues surrounding the post-consent rights of consumers.
Third, five items were used to measure consumers’ trust in the IoT service provider to whom they submitted their personal information. Based on the perspectives of Bansal et al. (Citation2010) and Bansal et al. (Citation2016), we wanted to measure the level of consumers’ trust in IoT service providers based on their user experience. However, we adapted the items to suit the IoT environment, because the items in previous research were targeted at the digital and online environments. Therefore, we developed items to measure consumers’ confidence that IoT service providers would do their best to protect the consumers’ rights as subjects of the collected information in terms of information collection, security, and damage relief.
Finally, five items were used for measuring consumers’ privacy awareness. Following Li et al.’s (Citation2011) research, we defined privacy awareness as consumers’ awareness of the content of the privacy statement. To measure the consumers’ levels of awareness of the main content of privacy notice, we developed five items in the following dimensions—collecting, storing, processing, the time needed to process the collected data, and scope of third-party provider (i.e., the extent of access permitted to third parties). A five-point semantic differential scale was used for measuring WPPI, and a five-point Likert scale was used for the other variables. The questionnaire is presented in Appendix A ().
We performed a path analysis for hypothesis testing of our structural model and a parallel mediation analysis to verify the mediating effects of privacy concern and trust for one’s service provider in the relationship between privacy awareness and WPPI. We used IBM SPSS Amos 26 and process macro package 4.0 for performing a path analysis and a parallel mediation analysis for each. Before that, we examined the normality of the constructs by checking their descriptive and distributional statistics (). As a result, we confirmed that none of the measures severely violated the requirements for a normal distribution (West et al., Citation1995). We also performed a confirmatory factor analysis and estimated the pairwise correlation coefficients of the latent variables to examine the validity of our construct model. As shown in and , we confirmed that the model satisfied the requirements of convergent and discriminant validity (Steenkamp & Baumgartner, Citation1998).
Table 4. Descriptive and distributional statistics results.
Table 5. Confirmatory factor analysis results.
Table 6. Pairwise correlation results.
3. Results
3.1. Path analysis results
shows the path analysis results obtained using our research model. The indices such as RMSEA, NNFI, and CFI shows that our research model satisfies the goodness of fit criteria (RMSEA values below .5 [Rigdon, Citation1996], and NFI and CFI values greater than .9 [Bentler, Citation1990; Tucker & Lewis, Citation1973]). We also confirmed that all the hypotheses among the four construct variables were significant. Specifically, privacy concerns and trust, the two fundamental variables of the extended privacy calculus model, showed different results. Trust in the IoT service provider positively affected (β = .32, p < 0.001), but privacy concern negatively affected (β = −.12, p < 0.001) consumers’ WPPI. Privacy awareness, a new variable we added to consider the procedural fairness of providing personal information, significantly affected all three variables in the privacy calculus model. Specifically, privacy awareness negatively affected privacy concerns (β = −.11, p < 0.001), and positively affected trust for service provider (β = .52, p < 0.001) and WPPI (β = .20, p < 0.001).
Table 7. AMOS results: path analysis
3.2. Parallel mediation analysis results
The research model of this study corresponds to a “multiple parallel mediator model” in which two mediating effects exist in parallel form. Previously, we used the AMOS program to conduct a path analysis. However, since AMOS cannot verify or compare the statistical significance of each parameter, we also tested the statistical significance of the indirect effect and the difference between the indirect effect of each mediator using Hayes’ (Citation2015) PROCESS macro.
The results showed that the direct path between all construct variables was statistically significant, similar to the path analysis results (, ). The explanatory power of the model was .19. The total effect of privacy awareness on WPPI was .36, and the direct effect was .22 (). From the result of verifying the statistical significance of the indirect effect, the total indirect effect was statistically significant. This indicates that privacy concern and trust for one’s service provider collectively and partially mediate the relationship between privacy awareness and WPPI (). The partial mediating effect of privacy concern and trust for one’s service provider was .01 and .14, respectively, and the mediating effect of trust was significantly larger than that of privacy concern ().
Figure 2. Parallel-multiple mediating effect of privacy concern and trust in one’s service provider in the relationship between privacy awareness and willingness to provide personal information
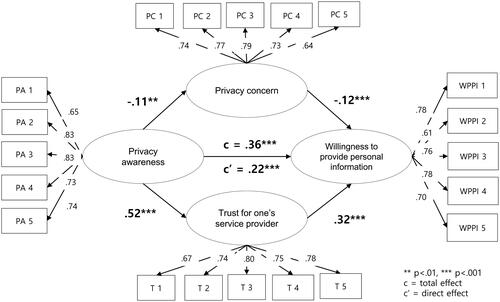
Table 8. PROCESS Macro result: direct effects between privacy awareness, privacy concern, trust in one’s service provider, and willingness to provide personal information
Table 9. PROCESS Macro result: total effect and direct effect of privacy awareness on willingness to provide personal information
Table 10. PROCESS Macro result: Indirect effects of privacy awareness on willingness to provide personal information
Table 11. PROCESS Macro result: Difference test between indirect effects
4. Discussion
This research aimed to verify how consumers determine their intention to provide personal information to IoT services and the role of procedural fairness in that consumer behavior. Based on previous studies that used privacy calculus and procedural fairness, we selected consumers’ privacy concerns, trust in the service provider, and privacy awareness as antecedents to WPPI. We hypothesized a causal relationship between privacy concern, trust in IoT service providers, and WPPI (Hypotheses 1 and 2), and assumed consumer privacy awareness and privacy concern (Hypothesis 3), trust in IoT service providers (Hypothesis 4), and WPPI for future service use (Hypothesis 5).
The results of the path analysis confirmed Hypotheses 1 and 2, by showing that consumers’ WPPI for future use of IoT services is determined by both privacy concerns (Hypothesis 1, β = −.12, p < 0.001) and trust in one’s service provider (Hypothesis 2, β = .32, p < 0.001). Specifically, there was a negative relationship between privacy concern and WPPI and a positive relationship between trust in one’s service provider and WPPI.
These results were identical to the empirical results of the extended privacy calculus theory of Dinev and Hart (Citation2006a), who developed the privacy calculus theory in the context of the e-commerce environment. Meanwhile, trust in the IoT service provider proved to have greater explanatory power than privacy concerns for WPPI. In previous studies, the influence of privacy concerns on an individual’s decision-making regarding providing personal information was found to be insignificant or weak (Nguyen et al., Citation2008; Spiekermann et al., Citation2001; Wang et al., Citation2016). This trend was more pronounced in a technology environment where the collection and use of personal information are routine, such as IoT (Kim et al., Citation2019). The results of our study show the same trend. Even under the IoT environment, privacy concern is still a significant variable that determines the WPPI, but its influence is weaker than that of the trust in one’s service provider.
Furthermore, the results from path analysis confirmed that consumers’ privacy awareness significantly affects all variables in our construct model. Consumer’s privacy awareness decreases privacy concern (Hypotheses 3, β = −.11, p < 0.001), and increases WPPI (Hypothesis 4, β =.20, p < 0.001) and trust in one’s service provider (Hypothesis 5, β =.52, p < 0.001). In terms of the power of effect, the standard path coefficients showed that the positive effect of privacy awareness on trust in one’s service provider was the most powerful. This indicates that the consumers’ perception of having formed sufficient privacy awareness through a fair privacy statement, lowers their privacy concerns, deepens their trust in the service provider, and raises their WPPI for using IoT services. Some researchers have expressed concern that the emphasis on procedural fairness would be meaningless in the IoT environment, if the personal information is automatically collected and freely utilized after the consumer’s initial consent (Calo, Citation2013; FTC, Citation2015; Rothchild, Citation2017). However, this study’s results provide evidence that even in a futuristic technology environment, such as that of IoT, the service provider’s efforts to secure procedural fairness through a clear and comprehensible privacy statement, would have a positive effect on consumers’ psychological stability and result in the service providers securing more potential service users.
Meanwhile, as all the hypotheses in the research model were significant, we confirmed that privacy concern and trust in one’s service provider partially mediate the relationship between privacy awareness and WPPI (). In the parallel mediation analysis conducted to compare the power of the mediating effect of each mediator, the mediating effect of trust in one’s service provider was significantly greater than that of privacy concern. These results correspond to those of the path analysis and confirm that the relative influence of trust on WPPI was greater in terms of indirect effects as well as direct effects. In short, to inspire consumers’ future usage intentions, IoT service providers must strive to gain consumers’ trust during the process of using their services, by providing clear and comprehensible privacy statements.
Within the IoT environment, privacy concerns and procedural fairness are still important variables that explain consumers’ decision-making about providing their personal information, despite their explanatory power being threatened by the recent developments in the technology environment. However, as with previous studies, we confirmed that the role of trust in one’s service provider has become increasingly more important than that of privacy concerns, as reflected in consumers’ personal information-disclosing behavior under everyday technology environments, such as that of the IoT.
The results also justify our continuing to strive to secure procedural fairness through fair information statements, and why IoT service providers should use fair information statements in their effort to gain the consumers’ trust. Although a “notice skepticism” that securing procedural fairness through fair information statements is no longer meaningless in an environment in which the collection and use of personal information are routine has been raised, this research empirically verified that procedural fairness functions as a significant antecedent that directly influences consumers to continue using new technology services, such as IoT, with confidence. It means both service providers and policymakers need to secure procedural fairness in the information disclosure process under new technology environments, such as those of the IoT, to enable stable growth for the markets for such technologies.
5. Conclusion
This research makes two contributions to the existing literature. First, we applied the privacy calculus theory to a new field of technology called IoT and empirically verified the effectiveness of privacy concerns and trust in one’s service provider, the two representative and controversial variables of previous studies. We confirmed that privacy concern was a significant antecedent in determining information disclosure intention, even in the IoT environment in which collection and use of personal information are indispensable. However, trust in one’s service provider functioned as the more important variable, which was also confirmed in recent research. This result suggests understanding consumers’ information-disclosing behavior in a new data environment, such as that of the IoT, necessitates paying more attention to relational service provider aspects, such as trust.
Second, we provided empirical evidence for the substantive, positive function of procedural fairness and privacy notice in consumers’ information-disclosing decisions. In prior research, the importance of the privacy notice was limited to the conceptual level, and empirical research was insufficient due to difficulties defining and virtualizing the term. In this research, we used consumers’ privacy awareness as a measurement variable of procedural fairness. As a result, we were able to confirm that when sufficient privacy awareness is created by providing a privacy notice, users show a lower level of privacy concern and a higher level of trust in one’s service provider. Consequently, this induces consumers to disclose their personal information more willingly. These results confirm that the policy direction of the EU’s GDPR, which reemphasized “notice and choice” as a prerequisite for legal use of personal information, is correct despite the rationale of the recently raised “notice skepticism.”
However, this study has limitations in two respects. First, it is necessary to use consumers’ objective awareness—not subjective awareness—to indicate the fairness of a privacy notice. In this study, we accepted the level of consumers’ privacy awareness reported by the consumers themselves to assess the fairness of the privacy notice. In previous research, the fairness of the privacy notice has remained a conceptual discourse or been treated as a nominal variable. Hence, even though there are limitations, in this empirical research, we selected consumers’ subjective privacy awareness as our moderate variable to verify the positive effect of the privacy notice. However, it is necessary to exercise caution while interpreting subjective evaluation because of the possibility of its being affected by individual dispositional attributes, such as self-efficacy. Therefore, in follow-up research, we suggest using the objective awareness of consumers as a proxy variable by preparing a virtualized privacy notice to measure procedural fairness, along with true-or-false questions to measure the respondents’ level of awareness regarding its content.
Second, it is necessary to extend the research on consumers’ decision-making about providing personal information related to IoT service providers to include general consumers with less user experience. In this study, to measure the constructs in the privacy calculus model based on real experiences, we included in our sample those with sufficient user experience with IoT services. However, the sample was not large enough because IoT is a new technology, and there are many individuals who have not yet used IoT-related services. Nevertheless, over time, the number of consumers with long-term experience of using IoT will increase. This will enable future researchers to collect an adequate sample of subjects with long-term experience of using IoT services and consider more effectively, the potential consumers’ privacy awareness and the effectiveness of fair privacy notice. The findings from such research will provide a reliable benchmark with which to compare and verify the effect of the privacy notice on the personal information-related decision-making of consumers with no experience of using IoT services.
Acknowledgements
This research was performed to apply the data collected from the research titled “A Study on Consumer Rights to Control Personal Data in the IoT Era (ISBN 979-11-5649-345-7)” which is conducted by the Korea Consumer Agency.
Disclosure statement
No potential conflict of interest was reported by the author(s).
Data availability statement
All data generated or analyzed during this study is included in this published report titled ‘A Study on Consumer Rights to Control Personal Data in the IoT Era (ISBN 979-11-5649-345-7)’ published by Korea Consumer Agency.
Additional information
Notes on contributors
Jeeyeon Sah
Jeeyeon Sah is a policy research fellow at the Korea Consumer Agency. She earned her PhD in consumer science at Seoul National University. Her research is focused on consumer policy, consumer safety, and, more recently, data safety issues within new technology environments.
Sangmin Jun
Sangmin Jun is an Associate Professor in the Department of Consumer Science at Chungbuk National University in Chungju, Republic of Korea. She completed her PhD in consumer science at Seoul National University. Her current research focuses on consumer policy, consumer information, and data issues.
References
- Al-Jabri, I. M., Eid, M. I., & Abed, A. (2019). The willingness to disclose personal information: Trade-off between privacy concerns and benefits. Information & Computer Security, 28(2), 161–181. https://doi.org/10.1108/ICS-01-2018-0012
- Andrade, E. B., Kaltcheva, V., & Weitz, B. (2002). Self-disclosure on the web: The impact of privacy policy, reward, and company reputation. In S. M. Broniarczyk & K. Nakamoto (Eds.), ACR North American advances (pp. 350–353). Association for Consumer Research.
- Bansal, G., Zahedi, F. & Gefen, D. (2010). The impact of personal dispositions on information sensitivity, privacy concern and trust in disclosing health information online. Decision Support Systems, 49(2), 138–150. https://doi.org/10.1016/j.dss.2010.01.010
- Bansal, G., Zahedi, F. M., & Gefen, D. (2016). Do context and personality matter? Trust and privacy concerns in disclosing private information online. Information & Management, 53(1), 1–21. https://doi.org/10.1016/j.im.2015.08.001
- Baruh, L., Secinti, E., & Cemalcilar, Z. (2017). Online privacy concerns and privacy management: A meta-analytical review. Journal of Communication, 67(1), 26–53. https://doi.org/10.1111/jcom.12276
- Bentler, P. M. (1990). Comparative fit indexes in structural models. Psychological Bulletin, 107(2), 238–246. https://doi.org/10.1037/0033-2909.107.2.238
- Borena, B., Bélanger, F., Egigu, D. (2015). Information privacy protection practices in Africa: A review through the lens of critical social theory. In 2015 48th Hawaii International Conference on System Sciences (pp. 3490–3497). Ieee. https://doi.org/10.1109/HICSS.2015.420
- Brecht, F., Fabian, B., Kunz, S., Müller, S. (2012). Communication anonymizers: Personality, internet privacy literacy and their influence on technology acceptance. ECIS 2012 Proceedings.
- Calo, R. (2013). Against notice skepticism in privacy (and elsewhere). Notre Dame Law Review, 87(3), 1027–1072.
- Chen, L., Zarifis, A., Kroenung, J. (2017). The role of trust in personal information disclosure on health-related websites. In Proceedings of the European Conference on Information Systems (ECIS) (No. 1, pp. 771–786). AISeL.
- Cichy, P., Salge, T. O., & Kohli, R. (2021). Privacy concerns and data sharing in the internet of things: Mixed methods evidence from connected cars. MIS Quarterly, 45(4), 1863–1892. https://doi.org/10.25300/MISQ/2021/14165
- Cranor, L. F. (2012). Necessary but not sufficient: Standardized mechanisms for privacy notice and choice. Journal on Telecommunications and High Technology Law, 10, 273.
- Culnan, M. J., & Armstrong, P. K. (1999). Information privacy concerns, procedural fairness, and impersonal trust: An empirical investigation. Organization Science, 10(1), 104–115. https://doi.org/10.1287/orsc.10.1.104
- Culnan, M. J., & Bies, R. J. (2003). Consumer privacy: Balancing economic and justice considerations. Journal of Social Issues, 59(2), 323–342. https://doi.org/10.1111/1540-4560.00067
- Dienlin, T., & Metzger, M. J. (2016). An extended privacy calculus model for SNSs: Analyzing self-disclosure and self-withdrawal in a representative US sample. Journal of Computer-Mediated Communication, 21(5), 368–383. https://doi.org/10.1111/jcc4.12163
- Dinev, T., & Hart, P. (2006a). An extended privacy calculus model for e-commerce transactions. Information Systems Research, 17(1), 61–80. https://doi.org/10.1287/isre.1060.0080
- Dinev, T., & Hart, P. (2006b). Internet privacy concerns and social awareness as determinants of intention to transact. International Journal of Electronic Commerce, 10(2), 7–29. https://doi.org/10.2753/JEC1086-4415100201
- Ermakova, T., Fabian, B., Zarnekow, R. (2014). Acceptance of health clouds-a privacy calculus perspective. In Proceedings of the European Conference on Information Systems (ECIS). https://aisel.aisnet.org/ecis2014/proceedings/track09/11/.
- Federal Trade Commission. (2015). Internet of things: Privacy & security in a connected world. FTC Staff Report.
- Fernandes, T., & Pereira, N. (2021). Revisiting the privacy calculus: Why are consumers (really) willing to disclose personal data online? Telematics and Informatics, 65, 101717. https://doi.org/10.1016/j.tele.2021.101717
- Folger, R., & Bies, R. J. (1989). Managerial responsibilities and procedural justice. Employee Responsibilities and Rights Journal, 2(2), 79–90. https://doi.org/10.1007/BF01384939
- Fornell, C., & Larcker, D. F. (1981). Structural equation models with unobservable variables and measurement error: Algebra and statistics. Journal of Marketing Research, 18(3), 382–388. https://doi.org/10.1177/002224378101800313
- Greenberg, J., & Folger, R. (1983). Procedural justice, participation, and the fair process effect in groups and organizations. In P. B. Paulus (Ed.), Basic group processes (pp. 235–256). Springer. https://doi.org/10.1007/978-1-4612-5578-9_10
- Hayes, A. F. (2015). An index and test of linear moderated mediation. Multivariate Behavioral Research, 50(1), 1–22. https://doi.org/10.1080/00273171.2014.962683
- Hong, S., Malik, M. L., & Lee, M. K. (2016). Testing configural, metric, scalar, and latent mean invariance across genders in sociotropy and autonomy using a non-Western sample. Educational and Psychological Measurement, 63(4), 636–654. https://doi.org/10.1177/0013164403251332
- Johnson, S. D., Blythe, J. M., Manning, M., & Wong, G. T. (2020). The impact of IoT security labelling on consumer product choice and willingness to pay. PloS one, 15(1), e0227800. https://doi.org/10.1371/journal.pone.0227800
- Karwatzki, S., Dytynko, O., Trenz, M., & Veit, D. (2017). Beyond the personalization-privacy paradox: Privacy valuation, transparency features, and service personalization. Journal of Management Information Systems, 34(2), 369–400. https://doi.org/10.1080/07421222.2017.1334467
- Kehr, F., Wentzel, D., Kowatsch, T., & Fleisch, E. (2015). Rethinking privacy decisions: Pre-existing attitudes, pre-existing emotional states, and a situational privacy calculus. In Twenty-Third European Conference on Information Systems. https://www.alexandria.unisg.ch/publications/224696
- Keith, M. J., Thompson, S. C., Hale, J., Lowry, P. B., & Greer, C. (2013). Information disclosure on mobile devices: Re-examining privacy calculus with actual user behavior. International Journal of Human-Computer Studies, 71(12), 1163–1173. https://doi.org/10.1016/j.ijhcs.2013.08.016
- Kim, D., Park, K., Park, Y., & Ahn, J. H. (2019). Willingness to provide personal information: Perspective of privacy calculus in IoT services. Computers in Human Behavior, 92, 273–281. https://doi.org/10.1016/j.chb.2018.11.022
- Kim, M., & Choi, B. R. (2022). The impact of privacy control on users’ intention to use smart home internet of things (IoT) services. Asia Marketing Journal, 24(1), 4. https://doi.org/10.53728/2765-6500.1586
- Koohang, A., Sargent, C. S., Nord, J. H., & Paliszkiewicz, J. (2022). Internet of Things (IoT): From awareness to continued use. International Journal of Information Management, 62, 102442. https://doi.org/10.1016/j.ijinfomgt.2021.102442
- Kowatsch, T., & Maass, W. (2012, September). Critical privacy factors of internet of things services: An empirical investigation with domain experts. In Mediterranean Conference on Information Systems (pp. 200–211). Springer.
- Kuo, K. M., & Talley, P. C. (2014). An empirical investigation of the privacy concerns of social network site users in Taiwan. International Journal of Scientific Knowledge, 5(2), 1–19.
- Langheinrich, M. (2001, September). Privacy by design – Principles of privacy-aware ubiquitous systems. In International Conference on Ubiquitous Computing (pp. 273–291). Springer. https://doi.org/10.1007/3-540-45427-6_23
- Laufer, R. S., & Wolfe, M. (1977). Privacy as a concept and a social issue: A multidimensional developmental theory. Journal of Social Issues, 33(3), 22–42. https://doi.org/10.1111/j.1540-4560.1977.tb01880.x
- Li, H., Sarathy, R., & Xu, H. (2011). The role of affect and cognition on online consumers’ decision to disclose personal information to unfamiliar online vendors. Decision Support Systems, 51(3), 434–445. https://doi.org/10.1016/j.dss.2011.01.017
- Li, T., & Unger, T. (2012). Willing to pay for quality personalization? Trade-off between quality and privacy. European Journal of Information Systems, 21(6), 621–642. https://doi.org/10.1057/ejis.2012.13
- Liu, C., Marchewka, J. T., Lu, J., & Yu, C. S. (2005). Beyond concern – A privacy-trust-behavioral intention model of electronic commerce. Information & Management, 42(2), 289–304. https://doi.org/10.1016/j.im.2004.01.003
- Lo, J., Riemenschneider, C. (2010). An examination of privacy concerns and trust entities in determining willingness to disclose personal information on a social networking site. In AMCIS 2010 Proceedings.
- Malhotra, N. K., Kim, S. S., & Agarwal, J. (2004). Internet users’ information privacy concerns (IUIPC): The construct, the scale, and a causal model. Information Systems Research, 15(4), 336–355. https://doi.org/10.1287/isre.1040.0032
- Maseeh, H. I., Jebarajakirthy, C., Pentecost, R., Arli, D., Weaven, S., & Ashaduzzaman, M. (2021). Privacy concerns in e‐commerce: A multilevel meta‐analysis. Psychology & Marketing, 38(10), 1779–1798. https://doi.org/10.1002/mar.21493
- Mcknight, D. H., Lankton, N., Tripp, J. (2011, January). Social networking information disclosure and continuance intention: A disconnect. In 2011 44th Hawaii International Conference on System Sciences (pp. 1–10). IEEE. https://doi.org/10.1109/Hicss.2011.379
- Meinert, D. B., Peterson, D. K., Criswell, J. R., & Crossland, M. D. (2006). Privacy policy statements and consumer willingness to provide personal information. Journal of Electronic Commerce in Organizations (JECO), 4(1), 1–17. https://doi.org/10.4018/jeco.2006010101
- Milne, G. R., & Gordon, M. E. (1993). Direct mail privacy-efficiency trade-offs within an implied social contract framework. Journal of Public Policy & Marketing, 12(2), 206–215. https://doi.org/10.1177/074391569101200206
- Morrison, C., Marschark, M., Sarchet, T., Convertino, C. M., Borgna, G., & Dirmyer, R. (2013). Deaf students’ metacognitive awareness during language comprehension. European Journal of Special Needs Education, 28(1), 78–90. https://doi.org/10.1080/08856257.2012.749610
- Mousavizadeh, M., Kim, D. J., & Chen, R. (2016). Effects of assurance mechanisms and consumer concerns on online purchase decisions: An empirical study. Decision Support Systems, 92, 79–90. https://doi.org/10.1016/j.dss.2016.09.011
- Nam, C., Song, C., Park, E. L., & Ik, C. (2006). Consumers’ privacy concerns and willingness to provide marketing-related personal information online. In S. M. Broniarczyk & K. Nakamoto (Eds.), ACR North American Advances (pp. 212–217). Association for Consumer Research.
- Nguyen, D. H., Kobsa, A., Hayes, G. R. (2008, September). An empirical investigation of concerns of everyday tracking and recording technologies. In Proceedings of the 10th International Conference on Ubiquitous Computing (pp. 182–191). https://doi.org/10.1145/1409635.1409661
- Norberg, P. A., Horne, D. R., & Horne, D. A. (2007). The privacy paradox: Personal information disclosure intentions versus behaviors. Journal of Consumer Affairs, 41(1), 100–126. https://doi.org/10.1111/j.1745-6606.2006.00070.x
- Pal, D., Funilkul, S., & Zhang, X. (2021). Should I disclose my personal data? Perspectives from Internet of Things services. IEEE Access. 9, 4141–4157. https://doi.org/10.1109/ACCESS.2020.3048163
- Phelps, J., Nowak, G., & Ferrell, E. (2000). Privacy concerns and consumer willingness to provide personal information. Journal of Public Policy & Marketing, 19(1), 27–41. https://doi.org/10.1509/jppm.19.1.27.16941
- Princi, E., & Krämer, N. C. (2020). Out of control–privacy calculus and the effect of perceived control and moral considerations on the usage of IoT healthcare devices. Frontiers in Psychology, 11, 582054. https://doi.org/10.3389/fpsyg.2020.582054
- Rigdon, E. E. (1996). CFI versus RMSEA: A comparison of two fit indexes for structural equation modeling. Structural Equation Modeling, 3(4), 369–379. https://doi.org/10.1080/10705519609540052
- Rothchild, J. A. (2017). Against notice and choice: The manifest failure of the proceduralist paradigm to protect privacy online (or anywhere else). Cleveland State Law Revie, 66, 559.
- Schomakers, E. M., Lidynia, C., & Ziefle, M. (2022). The role of privacy in the acceptance of smart technologies: Applying the privacy calculus to technology acceptance. International Journal of Human–Computer Interaction, 38(13), 1276–1289. https://doi.org/10.1080/10447318.2021.1994211
- Sloan, R. H., & Warner, R. (2014). Beyond notice and choice: Privacy, norms, and consent. Journal of High Technology Law, 14, 370. https://doi.org/10.2307/249477
- Smith, H. J., Dinev, T., & Xu, H. (2011). Information privacy research: An interdisciplinary review. MIS Quarterly, 35(4), 989–1015. https://doi.org/10.2307/41409970
- Smith, H. J., Milberg, S. J., & Burke, S. J. (1996). Information privacy: Measuring individuals’ concerns about organizational practices. MIS Quarterly, 20(2), 167–196. https://doi.org/10.2307/249477
- Solove, D. J. (2012). Introduction: Privacy self-management and the consent dilemma. Harvard Law Review, 126, 1880.
- Spiekermann, S., Grossklags, J., Berendt, B. (2001, October). E-privacy in 2nd generation E-commerce: Privacy preferences versus actual behavior. In Proceedings of the 3rd ACM Conference on Electronic Commerce (pp. 38–47). https://doi.org/10.1145/501158.501163
- Steenkamp, J. B. E., & Baumgartner, H. (1998). Assessing measurement invariance in cross-national consumer research. Journal of Consumer Research, 25(1), 78–107. https://doi.org/10.1086/209528
- Sun, Y., Wang, N., Shen, X. L., & Zhang, J. X. (2015). Location information disclosure in location-based social network services: Privacy calculus, benefit structure, and gender differences. Computers in Human Behavior, 52, 278–292. https://doi.org/10.1016/j.chb.2015.06.006
- Tucker, L. R., & Lewis, C. (1973). A reliability coefficient for maximum likelihood factor analysis. Psychometrika, 38(1), 1–10. https://doi.org/10.1007/BF02291170
- Utz, C., Degeling, M., Fahl, S., Schaub, F., Holz, T. (2019). November). (Un)informed consent: Studying GDPR consent notices in the field. In Proceedings of the 2019 ACM SIGSAC Conference on Computer and Communications Security (pp. 973–990). https://doi.org/10.1145/3319535.3354212
- Wang, S., Beatty, S. E., & Foxx, W. (2004). Signaling the trustworthiness of small online retailers. Journal of Interactive Marketing, 18(1), 53–69. https://doi.org/10.1002/dir.10071
- Wang, Y., Min, Q., & Han, S. (2016). Understanding the effects of trust and risk on individual behavior toward social media platforms: A meta-analysis of the empirical evidence. Computers in Human Behavior, 56, 34–44. https://doi.org/10.1016/j.chb.2015.11.011
- West, S. G., Finch, J. F., & Curran, P. J. (1995). Structural equation models with nonnormal variables: Problems and remedies. In R. H. Hoyle (Ed.), Structural equation modeling: Concepts, issues, and applications (pp. 56–75). Sage Publications, Inc.
- Wilson, D., Valacich, J. S. (2012). Unpacking the privacy paradox: Irrational decision-making within the privacy calculus. In 33rd International Conference on Information Systems (pp. 1–11).
- Wu, K. W., Huang, S. Y., Yen, D. C., & Popova, I. (2012). The effect of online privacy policy on consumer privacy concern and trust. Computers in Human Behavior, 28(3), 889–897. https://doi.org/10.1016/j.chb.2011.12.008
- Xu, H., & Gupta, S. (2009). The effects of privacy concerns and personal innovativeness on potential and experienced customers’ adoption of location-based services. Electronic Markets, 19(2–3), 137–149. https://doi.org/10.1007/s12525-009-0012-4
- Xu, Y., Wang, D. (2022, November). Understanding the willingness to share building data by a social study based on privacy calculus theory. In Proceedings of the 9th ACM International Conference on Systems for Energy-Efficient Buildings, Cities, and Transportation (pp. 59–68). https://doi.org/10.1145/3563357.3564059
- Yun, H., Lee, G., & Kim, D. J. (2014). A meta-analytic review of empirical research on online information privacy concerns: Antecedents, outcomes, and moderators. In 35th International Conference on Information Systems: Building a Better World through Information Systems, ICIS 2014. Association for Information Systems.
- Zhu, M., Wu, C., Huang, S., Zheng, K., Young, S. D., Yan, X., & Yuan, Q. (2021). Privacy paradox in mHealth applications: An integrated elaboration likelihood model incorporating privacy calculus and privacy fatigue. Telematics and Informatics, 61, 101601. https://doi.org/10.1016/j.tele.2021.101601
Appendix A.
Instruments
Table A1. Questionnaire items and scales of the construct variables.