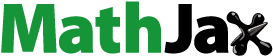
ABSTRACT
Aquaculture products are presently becoming a crucial part of consumers’ diets. However, asymmetric information regarding farmed fish exposes consumers to ambiguity and often makes them confused. Therefore, this study aims to ascertain the levels of ambiguity tolerance (AT) and confusion avoidance (CA) related to farmed fish and to test if consumers’ AT and CA influence their purchase intent (PI) of such fish. The impact of demographics on consumers’ CA and AT is also explored. The data were obtained through a survey conducted on a randomly selected sample of 1053 households who either purchase and have purchased fish. The collected data were analyzed employing explorative factor analysis, structural equation modeling, and a simple regression model. The study demonstrates that AT had a positive and significant impact on the PI of farmed fish, and also reduced CA. However, the current high level of CA does not influence the PI of farmed fish.
Introduction
Fish is widely accepted as an essential part of a balanced and healthy diet because of its low fat content and high quality protein, such as minerals and vitamins (Nesheim and Yaktine Citation2007). Due to its nutritional value, per capita fish consumption has increased globally (FAO Citation2018). This increasing demand leads to a relatively high production of 171 million tonnes of fish, of which 88% was consumed directly as food in 2016 (FAO Citation2018). Therefore, the fisheries sector plays an essential role in regional food security, and its responsible supply chain contributes to reducing poverty (FAO Citation2000). Currently, as a result of population growth, overfishing, pollution, and ocean acidification, natural fish stocks are decreasing significantly (Gordon et al. Citation2018). To meet the demand for fish products, aquaculture, or farmed fish, has been proliferating in the last decade (Little, Newton, and Beveridge Citation2016). The contribution of aquaculture to total global fish production was 46.8% in 2016, up from 25.7% in 2000 (FAO Citation2018). With the rapid growth in aquaculture and its rising demand, consumers are receiving inconsistent and mixed messages about the contribution and adverse impacts of farm-raised fish on the environment and biomass (Schlag and Ystgaard Citation2013). As a result, consumers are torn between the appeal of cheap and convenient food and concern about intensive fish farming and the denaturalization of foodstuffs (Gaskell Citation2010). For instance, they hold a positive view of farmed fish in general, but this becomes negative when environmental issues arise (Gentry et al. Citation2017).
The inconsistent information related to consumers’ beliefs might lead to them facing ambiguity (Grewal, Gotlieb, and Marmorstein Citation1994; Maheswaran and Meyers-Levy Citation1990). The extent to which consumers will tolerate this level of ambiguity depends on their openness (Bardi, Guerra, and Ramdeny Citation2009). Tolerance of ambiguity is concerned with the degree to which people are able to restrain their need for a perfect, unobstructed view of the environment (Feather Citation1969). Individuals with a low tolerance may prematurely close their information processing activities and be rigidly resistant to new information. Consequently, ambiguity tolerance might be a valued functional variable for research in many fields of study, since it plays a moderating role between situational information and consumers’ buying behavior (McLain, Kefallonitis, and Armani Citation2015). In the marketing literature, ambiguity tolerance has been found to exert significant influences over a wide variety of consumer behaviors, such as willingness to pay (Hazen et al. Citation2012), brand preference (Richardson, Jain, and Dick Citation1996), and satisfaction (Wittenberg and Norcross Citation2001). Therefore, practitioners should know whether consumers face any ambiguity in their farmed fish experience, and how this perceived value affects their choices, as consumers’ ambiguity tolerance is significantly associated with their confusion avoidance behaviors.
Like other traditional agriculture farming, farmed fish may have some adverse effects on the environment (Amberg and Hall Citation2008). Interestingly, these various effects have yet to be fully assessed, and are a fascinating issue in the food contamination field, creating confusion for consumers (Watterson et al. Citation2008). In addition, product similarity, information overload, and ambiguous information all create consumer confusion, affecting their decision-making adversely (Turnbull, Leek, and Ying Citation2000). From the massive amount of information available, they tend to just process a small fraction of it (Hauser, Wernerfelt, and Wernerfelt Citation1990), eventually choosing the default option, deferring their decision, or simply deciding not to make a choice (Iyengar and Lepper Citation2000; Shafir, Simonson, and Tversky Citation2000). As confusion leads to consumer dissatisfaction, lower repeat sales, more returned products, and reduced customer loyalty (Mitchell and Papavassiliou Citation1999), marketers need to have a clear idea of the causes of any confusion. They also need to be concerned about helping consumers clarify products and making decisions on choices (Mitchell and Papavassiliou Citation1999).
As part of a nutritious diet, total fish consumption was estimated to grow substantially by 30% between 2010 and 2030 (World Bank Citation2013). Consumers are heterogeneous in their choice of all the fish attributes (Addeah, Quagrainie, and Chenyambuga Citation2016). This demand and choice heterogeneity for fishery products, together with the technical and commercial opportunities, make aquaculture a critical subject for fish farmers, policymakers, and marketers. Farmed fish should be traded with food safety labels, including environmental and biological sustainability indicators (Hoque Citation2020), and with appropriate communication, such fish could be suitable candidates for functional food (Ramalho Ribeiro et al. Citation2019). However, aquaculture is still a new and unfamiliar industry for many people, and detailed empirical research on consumers’ perceived discrepancy between wild and farm fish is lacking (Schlag and Ystgaard Citation2013). In current agriculture practices, farmed fish are considered to be at an early development stage of domestication and breeding, but a rapid response to genomic selection for growth has been accounted for in many domestic species (Olesen et al. Citation2003). In the market, farmed fish are affected by lower price, fear of counterfeit products, and insufficient information on sustainable farming practices (Altintzoglou et al. Citation2010; Claret et al. Citation2014; Vanhonacker et al. Citation2011). For example, Claret et al. (Citation2014) found that taste was one of the sensory barriers to accepting farmed fish. Earlier studies also found that fish consumers often considered farmed fish to be less healthy than wild fish and of a lower quality (for example, Verbeke et al. Citation2007). A recent study conducted in France showed that consumers perceive wild fish to be best in terms of safety and health, with farmed fish best for environmental sustainability and fish welfare (Kyrre Rickertsen et al. Citation2017). They ranked salmon from advanced countries top, and farmed fish originating from developing countries lowest (Kyrre Rickertsen et al. Citation2017). Regarding its health value, farmed fish contain less contamination, such as mercury, cobalt, copper, and cadmium, than wild fish (Claret et al. Citation2014). Wild fish were preferred in the informed condition and farmed fish in the blind condition, suggesting the development of effective information strategies addressed to the public (Claret et al. Citation2016). To gain consumers’ loyalty and trust, it is farmers’ challenge to eliminate the gap between farmed fish producers and consumer knowledge through public communication, linking the sensory attributes of farm-raised fish and environmental attributes effectively (Gaviglio and Demartini Citation2009).
In additional, fish farming has given rise to a range of potentially controversial issues (e.g., different figures for the feed conversion ratio (FCR) to produce farmed salmon), which may negatively influence public perceptions (Schlag and Ystgaard Citation2013). The high growth of fish farming is constrained by issues of excess water consumption; limited availability of space; the high cost of feed (Naylor et al. Citation2000); water unavailability; the risks of adverse environmental impacts (Duarte et al. Citation2009); and social and organizational risks (Schlag and Ystgaard Citation2013), which show the unsustainable characteristic of the production process. These controversial issues mean that consumers have imbalanced and limited information regarding farmed fish products, and that imbalanced information has been communicated to the public in attempting to influence their choices (O’Rourke and Ringer Citation2016). Though consumers’ confusion does not influence the formation of their attitudes toward farmed fish (Hoque and Alam Citation2020), consumers often face confusion in learning about farmed fish, which results in difficulties for them while purchasing indicate attitude-behavior gap.
Therefore, aquaculture needs to carefully consider these controversial issues and negative perceptions and then communicate facts to stakeholders regarding alternatives to wild fish. To do this, it is vital to understand the impact of ambiguity tolerance and confusion avoidance on purchase intention. Stanley Budner (Citation1962) argued that tolerance of ambiguity is a personality variable that results in individual differences, while Lachance, Ladouceur, and Dugas (Citation1999) proposed the use of ambiguity tolerance in a variety of applied fields in the social sciences. However, how and to what extent consumers tolerate ambiguity and avoid confusion, in face of situations of controversy, confusion, and ambiguity, remain unresearched in the field of aquaculture, thus motivating this study. Furthermore, studies regarding demand estimates and consumers’ purchasing behavior in relation to fish and seafood products in emerging economies are rare (Carlucci et al. Citation2015).
To fill the literature gap, the objective of this study is to investigate how the perceived value of ambiguity tolerance and confusion avoidance affect consumers’ aquaculture product purchase intention; in this case, farmed fish. In addition, the relationship between ambiguity tolerance and confusion avoidance is examined. While considering the above objectives, the effect of sociodemographic factors on consumers’ confusion avoidance and ambiguity tolerance is also observed. The study is based on data collected through a questionnaire survey of 1053 households in the two major cities (Dhaka and Chittagong) of the emerging economy of Bangladesh. The respondents were chosen randomly, and the collected data were analyzed using explorative factor analysis (EFA), structural equation modeling (SEM), and a simple regression model.
The structure of the study is as follows. A discussion of the data and methods follows the literature review, hypothesis development, and conceptual model. Subsequently, the results are discussed and concluding remarks made, including the limitations of the study and opportunities for future research.
Theory and hypothesis development
To meet the rising demand for fish, in Asia aquaculture has produced an additional 23 million tonnes of fish, representing 58% of world fishery production (Hongskul Citation1999). During the last two decades, Asia has contributed around 89% of world aquaculture production, and it is projected that it will remain the leader in world aquaculture until 2030 (FAO Citation2018). Aquaculture has expanded steadily in South Asia in recent years, contributing 40% of total fishery production in the region. Bangladesh, an emerging Asian economy, is one of the world’s top-ranked countries in aquaculture production. This economy has received increased development of aquaculture rapidly where most people prefer to eat fish. In this region, Bangladesh is the second-largest producer of aquaculture products and the first in terms of per capita consumption (Funge-Simon et al. Citation2012).
Although there is substantial product differentiation in aquaculture in the Bangladeshi economy, the market has no use for product information (e.g., prescribed quality certification or labels) for consumers. Therefore, Bangladesh’s inability or unwillingness to adopt fish food labels (e.g., eco-labeling) leads to a weakening of the market’s competitive strength and of consumer trust. Additionally, with regard to local consumers’ growing concerns about safety issues, Bangladesh’s fish supply is becoming questionable, with consumers facing ambiguous situations and confusion in their decision-making after a series of fish scandals. In the domestic value chain, fish traders frequently add formalin to fish as a preservative (Hoque et al. Citation2016). Moreover, producers and fish vendors unethically use formaldehyde to protect fish and seafood from microbial spoilage, a practice which takes place in different wet markets (Rahman et al. Citation2012). Therefore, the growth in aquaculture is associated with consumer confusion regarding safety issues, which leads to ambiguity.
Ambiguity tolerance
Ambiguity may be defined as a perception which is a function of the data regarding a focal stimulus. Ambiguity tolerance (AT) is a person’s deliberate, stable propensity to respond to anticipated ambiguity with more or less magnitude (McLain, Kefallonitis, and Armani Citation2015), and is “the tendency to perceive ambiguous situations as desirable” (Stanley Budner Citation1962, 29). Mclain (Citation1993) advocates the contextual denotation of ambiguity, explaining the construct as “a range, from rejection to attraction, of reactions to stimuli perceived as unfamiliar, complex, dynamically uncertain, or subject to multiple conflicting interpretations” (p. 184). In the cognitive psychology literature, AT is concerned with the extent to which people can control their need for a perfect and unobstructed view of the purchase environment; Feather Citation1969). Although the application of AT began 65 years ago, contemporary scholarly discourse is finding it to be a very appealing tool for research and inquiry into new areas of knowledge and information.
In recent work, researchers have realigned their attention toward analyzing how AT affects the view of situations and decision-making (McLain Citation2009; Van Hook Citation2002; Yurtsever Citation2001, Citation2008). The AT concept has been frequently used in research on management (e.g.,Yurtsever Citation2001); clinical psychology (e.g., Bentall and Swarbrick Citation2003); education (e.g., DeRoma, Martin, and Kessler Citation2003); organizational psychology (e.g., Lysonski Citation1990); entrepreneurship (e.g., Dollinger, Saxton, and Golden Citation1995); and medicine (e.g., Steginga and Occhipinti Citation2004). In more recent work, Eloi, Lopez-Valeiras, and Gonzalez-Sanchez (Citation2020) found that consumer tolerance of ambiguity played a positive role in the purchase of organic wine. Based on these discussions, this study assumes a positive effect of consumer AT on their farmed fish purchase intention. Therefore, the following hypothesis is posited:
H1: Consumers’ ambiguity tolerance (AT) positively affects their farmed fish purchase intention.
Consumers experience a phase of ambiguity if they intend to interpret a choice environment and make a more careful purchase decision. When the limits of error and uncertainty in the data surpass their ability to tolerate error and uncertainty, ambiguity confusion occurs. When comparing two or more complex products, consumers face confusion resulting from ambiguous information, which could result in choice deferral, since they attempt to adapt to what is viewed as a non-comparable substitute (Dhar Citation1997). However, consumers confused by ambiguity are not interested in involving in contrary word-of-mouth if they are unable to fully understand a product themselves. Individuals with strong AT tendencies do not feel constrained to acquire new information or come to conclusive results.
Consequently, AT does not imply resolving uncertainty, but reflects the motive of acceptance by suggesting the propensity to learn passively, so that individuals may pursue opportunities in making wise decisions (Litman Citation2005). One of the attributes familiar to most ambiguous circumstances is that more information will minimize ambiguity (McLain, Kefallonitis, and Armani Citation2015). In general, for those with low AT, there is an averse response to ambiguous circumstances because the absence of information makes it hard to evaluate risk and effectively settle on a choice. These situations are seen as a risk and root of uneasiness. Responses to the perceived risk are in the form of stress, avoidance, deferral, suppression, or refusal (Furnham and Ribchester Citation1995; Mac Donald Citation1970; Mclain Citation1993; Stanley Budner Citation1962). Considering the above findings, we assume that ambiguity-tolerant consumers will avoid confusion, so the following hypothesis is formulated:
H2: Consumers’ ambiguity tolerance (AT) affects their confusion avoidance (CA) positively.
Previous research has suggested that a product’s success is related partly to how companies manage ambiguity (Frishammar, Florén, and Wincent Citation2009). Hazen et al. (Citation2012) found that the ambiguity inherent in the remanufacturing process facilitates consumers’ perceptions of more mediocre quality and reduces their willingness to pay for items. Choice overload and ambiguity have been found to reduce consumers’ choice confidence (Wang and Shukla Citation2013). In analyzing the information received by consumers, Claret et al. (Citation2016) found that sensory perception seemed to be based not only on the intrinsic cues of fish, but also on the information provided to consumers and cognitive processing.
Confusion avoidance
“Confusion is a conscious state of mind that can occur either in the pre or the post-purchase situation and has not only a cognitive dimension but also a behavioural one” (Mitchell et al. Citation2004, 3). Confusion can be perceived as a cognitive state, in which, as Keltner and Shiota (Citation2003) point out, one can be overloaded with information and uncertain about what to do or how to act. As a negative consequence, consumer confusion can create dissatisfaction and not lead to satisfaction. A review of the literature (e.g., Mitchell, Walsh, and Yamin Citation2005; Walsh and Mitchell Citation2010) reveals that the concept of consumer confusion can be traced back to three different areas: (a) brand similarity, (b) information overload, and (c) information ambiguity. Proneness to similarity confusion results in consumers potentially altering their choice because of the perceived physical similarity of products. The personality trait that persuades consumers to withdraw from a confused or tricky situation is confusion avoidance.
In contrast, Walsh, Hennig-Thurau, and Mitchell (Citation2007) define overload confusion proneness as consumers’ difficulty in product decision-making when they encounter high levels of options and information which they cannot process. Such proneness is also defined as consumers’ tolerance for processing unclear, misleading, or ambiguous, product-related information (Turnbull, Leek, and Ying Citation2000), which causes problems in understanding for consumers. Economic value makes consumers conscious and desirous of the best value for money. Therefore, they are less likely to experience similarity overload or ambiguity confusion. Walsh, Hennig-Thurau, and Mitchell (Citation2007) hypothetically surmise that proneness to ambiguity confusion might result in decision postponement. However, they show no explicit evidence to prove such a hypothesis, but merely imply consumers’ proneness to ambiguity confusion. On the other hand, Alarabi and Grönblad (Citation2012) emphasize that ambiguity confusion does not have a (statistically) significant effect on decision postponement and brand loyalty. Their study shows that consumers’ risk avoidance increases brand trust and credibility (Lee, Workman, and Jung Citation2016). Due to information asymmetry (e.g., product similarity, ambiguous information, or information overload), many consumers are currently facing confusion when purchasing farmed fish. Based on the findings of previous studies, it is hypothesized that the avoidance of confusion positively influences farmed fish purchase intention. Therefore, the following hypothesis is proposed:
H3: Consumers’ confusion avoidance positively affects their farmed fish purchase intention.
Balanced information on health benefits and health risks has been reported to result in firm intentions regarding seafood consumption frequency, but concurrently result in more negative attitudes toward seafood consumption (Verbeke et al. Citation2007). Hence, it can be seen that although increased information can create confusion, consumers with balanced information will be able to avoid this.
Farmed fish purchase intention
Different, interrelated factors influence consumers’ preferences for food. In recent years, the buying behavior of consumers in relation to fish and seafood products has drawn the attention of researchers globally because of the political and economic factors that are related to nutrition and diet, food safety, sustainability, and the fish industry (Carlucci et al. Citation2015). Personal traits and environmental factors have proven to be fundamental elements which shape the beliefs, attitudes, intentions, and behaviors of human beings (Fishbein and Ajzen Citation1975). Individual characteristics have also been found to impact directly or indirectly via intermediate constructs, such as attitude, and the intention to consume seafood (Myrland et al. Citation2000; Olsen Citation2003; Trondsen et al. Citation2003). Thong and Solgaard (Citation2017) found that most socio-demographic factors act as significant predictors for seafood consumption.
While consumers confront stimulus overload, their nervous systems are overwhelmed. They start to shut out certain aspect to conform their attention (Schneider, Gruman, and Coutts Citation2012). Similarly, consumers delay making right decisions enduringly while they face information ambiguity. In this case, brand loyalty drives them to make fewer comparisons. Therefore, we can say that consumers are attracted to less ambiguity or fewer stimuli. In the view of Walsh et al. (Citation2007), confusion reduction strategies are in the main concerned with clarifying a choice, reducing ambiguity, and increasing understanding relating to a purchase decision. The density of information received by consumers/individuals is critical in raising confusion that leads to conflicts and, lastly, aggravates ambiguity. To eliminate such ambiguity, consumers prefer to search for more information, compare alternatives, and evaluate the gathered information to make their purchase goals clearer. Dissonance mechanisms postulate that consumers will opt out of the bundle of benefits or postpone purchase. Consequently, they try to compensate any forgone benefits by acquiring products from categories with which they are more comfortable and aware. This result, though exploratory in nature, indicates that most consumers prefer wild sea fish. Therefore, well-organized information strategies focused on the public should be devised to support and expand farmed sea fish consumption, which will reduce the adverse impact of unsustainable fishing methods on natural fish resources.
Based on the theory and the conceptual model, it is necessary to discuss the direct, indirect, and total effects among the latent variables in “causal effect modeling” (Schreiber et al. Citation2006). An indirect effect suggests the influence of an independent variable (IV) on a dependent variable (DV) through a mediating variable (Baron and Kenny Citation1986). In food products, informed liking might mediate the association between cues and purchase intention (Hoque, Xie, and Nazneen Citation2018; Mueller and Szolnoki Citation2010). As confusion avoidance is an intrinsic cue, the literature leads us to believe that consumers’ confusion avoidance with regard to farmed fish can mediate the association between their tolerance of ambiguity and their purchase intention. Therefore, based on H1, H2 and H3, it is posited that:
H4: Confusion avoidance can mediate the relationship between ambiguity tolerance and farmed fish purchase intention.
The study’s main objective is to explore the influences of consumers’ ambiguity tolerance and confusion avoidance on farmed fish purchase intention in a significant emerging economy aquaculture market. To achieve the objective, the study develops a conceptual framework using structural equation modeling (). Evidence shows that consumers’ risk perception in terms of food strongly influences their purchase behavior (Klerck and Sweeney Citation2007). Although health risk is one of the critical barriers to fish consumption (Mancuso, Baldi, and Gasco Citation2016), many consumers are not aware of the risks and benefits of aquaculture products (Schlag and Ystgaard Citation2013). Conversely, regular fish consumption is a healthy habit that provides health benefits and reduces the risk of cardiovascular disease (Verbeke and Vackier Citation2005). As consumers are less conscious of the risk of seafood, and the health benefits of fish consumption are relatively high, this study does not assume that consumers’ risk perception can significantly influence their purchase intention. Therefore, the model excludes the risk perceptions of consumers in their farmed fish purchase intention.
The aquaculture market in Bangladesh
Seafood and fish are essential sources of quality protein and are cheaper than other animal protein sources that can help to form an efficient market structure. In Bangladesh, the farmed fish market grew by 25 times in the last three decades (Hernandez et al. Citation2018). The carp species alone contributes 33.57% to total annual production in 2014 (freshwater and marine water) (DoF Citation2018). About 85% of fish produced in ponds are carp, either exotic carp species such as silver carp or common carp, or indigenous carp species such as rui and mrigal (Roos et al. Citation2007). In Bangladesh, aquaculture production increased by 6% to 8% per annum from 1991 to 2002 (Ahmed Citation2003). Currently, Bangladesh’s total fish production is 41.34 lakh MT, of which aquaculture contributes 56.44% (DoF Citation2018). This increased production has helped Bangladesh gain fifth position in world aquaculture production (DoF Citation2018; FAOSTAT Citation2016), with inland pond culture contributing around 86% of total production. Among inland pond culture, it can be seen that carp polyculture is more productive, profitable, and capital intensive than other fish production methods (FAO Citation2013). Among all the species found, indigenous freshwater carp and exotic carp contribute around 22% and 10% of total production (Azim and Wahab Citation2002). Other freshwater fish include catfish, snakeheads, and small indigenous species.
Evidence shows that 73% of rural households are engaged in some form of aquaculture across the country (Mazid Citation1999); 1.2 million people are directly employed, and 12 million more are indirectly engaged in fishery-related activities (Dey et al. Citation2008). Das et al. (Citation2018) found that over 71% of total sampled farmed fish producers had either a primary or secondary education level. Their study also showed that farmers’ education level affected the utilization of ponds and fish production (Khan Citation1986). Poor households consider fish to be a luxury good, while it is a staple for more affluent households. Therefore, with an increase in population and per capita income in Bangladesh, fish production will increase (Dey, Alam, and Bose Citation2010).
Earlier studies found some barriers to the expansion of aquaculture in Bangladesh. These included lack of technical knowledge; joint ownership of ponds; costly fish feed and other necessary inputs; inadequate knowledge about economics; insufficient quality of fingerlings; and limited access to credit options (Ahmed and Rab Citation1992; Gupta, Ahmed, and Bimbao Citation1992; Gupta et al. Citation1999; Ahmed and Rab Citation1994; Gupta and Rab Citation1994; Lewis Citation1997; Chowdhury and Maharjan Citation2001; Thompson, Sultana, and Nuruzzaman Md Citation2005; ADB Citation2005). In a recent study, Das et al. (Citation2018) found that 80% of farmers do not receive any scientific aquaculture training, learning about fish farming from their ancestors or neighbors in an unscientific way. Another problem of Bangladesh’s aquaculture is that even though pond size and depth affect the efficiency of fish production positively (Khan Citation1986), most aquaculture ponds in Bangladesh are small (e.g., 1–4 Decimal), with only 17.27% of ponds relatively large. Besides environmental pollution, exploitation of resources also hinders the expansion of aquaculture.
Currently, freshwater aquaculture has increased total fish production, and it is expected that further aquaculture development might help to meet the rising demand for fish consumption. Therefore, the Ministry of Fisheries and Livestock is working to bring underutilized ponds under aquaculture. In this initiative, an additional 106,846 ha of land will soon be brought into pond aquaculture (Karim et al. Citation2006). DoF-BARC (Citation2001) also projected that perennial ponds’ future productivity would be 4 t/ha and that of seasonal ponds to be 2 t/ha. Despite the challenges faced, the productivity of aquaculture has been growing in Bangladesh.
Data and methods
Participants and procedures
To test the proposed hypotheses, the study was conducted in Dhaka and Chittagong, Bangladesh. This study area was chosen because they are the two most advanced cities in the country, Dhaka being the capital city, and Chittagong a port and commercial city. Both Dhaka and Chittagong have high strategic importance in the Bangladeshi economy. Another reason for selecting these cities is that their per capita seafood consumption is the highest in the country (DoF Citation2018). Consequently, they are most influenced by the consciousness of food information in advanced countries. Different methodological tools were applied to test the hypotheses. To collect the primary data from the study area, a structured questionnaire (see Appendix A) was distributed to the respondents by enumerators in affiliation with the researcher. The carp species was considered for its high acceptance and economic value in the Bangladesh economy in order to explore the perceived value of farmed fish purchase intention.
Random sampling procedures were used to select the representative sample. The number of respondents from Dhaka and Chittagong was 569 and 484 respectively, totaling 1053 overall. The study approached 1077 respondents, but 24 were omitted as they provided incomplete or partial information. A pretest survey was conducted on 20 people in Dhaka and 15 in Chittagong before initiating the field survey in order to eliminate scientific and measurement problems. The survey was conducted from January 5 to April 30, 2019. The interview took an average 15 minutes per interviewee. The motivation for the study was explained in a cover letter, and the respondents were asked a set of questions. For strong inter-correlations, a sample size of 150 observations should be sufficient for reliable exploratory factor analysis (EFA) (Guadagnoli and Velicer Citation1988), while for confirmatory factor analysis (CFA), a minimum sample size of 100 is recommended (Bollen and Bollen Citation1989). However, Sekaran (Citation2003) prefers a sample size of 30 to 500 to be appropriate. In general, 10% of the total population, or more than 1000, is an adequate sample size. Therefore, these requirements for analysis were met in the study. EFA was used to find the optimum number of dimensions and interrelations, and to form a pattern matrix. To measure the cause and effect relationship among constructs, SEM was used, while for the factor analysis and path model analysis, SPSS and AMOS graphics version 26.00 was employed. The normality and multicollinearity of the variables was also checked. Finally, two regression models were employed to assess the influences of demographics and socio-economic variables on CA and AT, using the STATA computer programme, version 16.00.
Questionnaire and measurements
The questionnaire, developed based on the literature, was in three parts. In the first part, respondents were requested to read about the concept of “consumer ambiguity” and “consumer confusion” in texts, with visual information. They were then asked to rate their feelings on a seven-point Likert scale, from “strongly disagree” (1) to “strongly agree” (7) for the ambiguity tolerance, consumer confusion and purchase intention constructs for farmed fish. The third part of the questionnaire covered the respondents’ demographic information, including age, gender, and fish consumption patterns. The ambiguity tolerance scale was adapted from the study by McLain (Citation2009). The four most relevant questions, relating to tolerance of ambiguous situations, enjoyment in tackling problems, preference for novelty over familiarity, and preference for ambiguous situations, was asked to investigate the feelings of ambiguity tolerance. The participants were asked to rate their feelings based on a series of statements such as “I enjoy tackling problems that are complex enough to be ambiguous.” Second, the confusion avoidance scale was adopted from the study by Schweizer, Kotouc, and Wagner (Citation2006). In order to develop the construct, six questions were asked, through which confusion avoidance was measured based on criteria such as variety, novelty, complexity, conflict, comfort, and reliability. Finally, purchase intention was measured by five statements adapted from Steadman and Rutter (Citation2004), namely “I intend to buy/plan to buy/buy/want to buy/am likely to buy farmed carp next time I buy fish ().
Table 1. Descriptive statistics of consumers’ ambiguity tolerance (AT), confusion avoidance (CA) and purchase intention (PI).
To justify the factorability of the data, the Kaiser−Meyer−Olkin (KMO) test and Bartlett’s test of sphericity (BTS) were performed (Pallant Citation2007). The value of the KMO in the first test ranged from 0 to 1; this should be at least 0.60, with a BTS significant at p <.05 (Tabachnick and Fidell Citation2001). The results were found to be significant (). Cronbach’s alpha was calculated for each construct to test the data reliability and internal consistency (). To gauge the discriminant validity, composite reliability was tested, and correlations between the variables were calculated (). The results show that no multicollinearity problem existed in our dataset. The fitness of the structural model was also tested (), and finally, to investigate the cause-and-effect relationship between the factors, SEM was employed.
Table 2. Kaiser–Meyer–Olkin (KMO) and Bartlett’s test of sphericity.
Table 3. Measurement model.
Table 4. Descriptive statistics and correlations among the latent constructs.
Table 5. Goodness of fit indices.
Measurement model
As a prerequisite of factor analysis, Kaiser-Meyer-Olkin (KMO) and Bartlett’s test of sphericity were conducted, as suggested by Pallant (Citation2007). The KMO test a value of 0.773, and in Bartlett’s test of sphericity, a significant p-value was attained (<0.01). Hence, our test met the criterion, and Bartlett’s test of sphericity also confirmed a high likelihood of successful data factorability (Pallant Citation2007).
Excellent model appropriateness was indicated by the measurement model, with values of χ2 = 3979.149, df = 45, p-value = 0.000, RMSEA = 0.017, GFI = 0.994, AGFI = 0.985, CFI = 0.972 and χ2/df = 1.302. We can assert that the measurement components were consistent with their underlying latent construct, since the model fit indices met the satisfactory threshold level recommended by Hair et al. (Citation2010). The test of normality representing the dataset is well-modeled, with normal distribution.
To test the convergent validity of the proposed constructs and to validate the factor loadings, EFA was run after achieving a satisfactory result (Hair Joseph et al. Citation1998). A total of 11 items were deduced, which had standardized regression weights (λ) ranging from 0.688 to 0.871. These weights (λ) were well above the recommended threshold level of 0.50, suggesting that they were significant in the corresponding latent constructs (Hair Joseph et al. Citation1998). To test for internal consistency, Cronbach’s alpha was calculated. Hair et al. (Citation2010) suggest 0.60, thus, the minimum cutoff value for Cronbach’s alpha is achieved by the three components, although a value higher than 0.70 is recommended. Furthermore, composite reliability (ρ) attained values well above the recommended level of 0.70, and the average variance extracted (AVE) value for each construct was well above 0.50, which indicates convergent validity for each construct (Hair et al. Citation2010).
Robustness check
The AVE of each construct was compared with the corresponding correlations between the respective constructs to test for discriminant validity, as recommended by Fornell and Larcker (Citation1981). In , it can be seen that all the average variance extracted values were more significant than their corresponding squared correlation (r2), which implies that each construct was unique, and that no multicollinearity problem existed in the dataset. Hence, discriminant validity was also confirmed.
Haque et al. (Citation2015) recommend analyzing convergent validity, discriminant validity and face validity to confirm the construct validity. Convergent and discriminant validity were confirmed in section 4.3 and , whereas face validity was confirmed by the individual perception of whether a particular construct measured the intended measure. The theoretical review discussed in this section and the information presented in the affirm the face validity. Therefore, it can be concluded that the observed data and our hypothesized model have a relatively good fit. Besides, the overall measurement model satisfies the validity tests.
Assessment of fitness for the structural model
The hypotheses presented earlier were tested to measure the impact of confusion avoidance and ambiguity tolerance on purchase intention. Several goodness-of-fit test statistics were employed to measure the fitness of the model (Brown Citation2006). The values attained for all the indices from absolute fit measure, incremental fit measure and parsimonious fit measure met the threshold values required for sufficient evidence of model fit that indicates construct validity (Haque et al. Citation2015). In the structural model, the values of χ2, RMSEA, and GFI satisfied the recommended level, suggesting an excellent absolute fit index. Incremental and parsimonious fit were also confirmed, since the values for AGFI, CFI, and Normed χ2 were well above the recommended model (). Hence, it was found that the data fitted well with the proposed constructs.
Results and discussion
Demographic profile of the respondents
The survey was conducted among respondents who frequently shop for their households. The descriptive statistics show that most were male (69.2%), and most belonged to the 20 to 49 year old age group (69.60%). 62.40% shopped by themselves for their family. On average, respondents had 14 years of education, which ensured, to some extent, that they fully understood the questions. It was found that the mean income of the respondents was BDT 48,500. The analysis also revealed that 747 of them (70.9%) earned less than BDT 50,000, while 306 (29.1%) had an income of more than BDT 50,000. 53.3% reported having children in the family, while 46.7% did not have children. Most of the respondents (60.8%) preferred the wet market to buy fish. 54.1% reported that they consumed fish more than once a week, while 13.4% consumed fish daily. The majority, 85.7%, opted not to be in an environmental club. presents the demographic information in full.
Table 6. Demographic profile of the respondents.
Respondents’ scores for different constructs
The scores for the ambiguity tolerance, confusion avoidance and purchase intention constructs are presented in . The respondents were sub-clustered into high, medium and low criteria, based on their preference scores for each construct. Those who ticked 6 to 7 on the seven-point Likert scale were identified as “high” scorers; if 3 to 5 they were “medium” scorers; and if 1 to 2 low scorers. The table shows that the consumers had a high tolerance for ambiguity and that their confusion avoidance rate was also high. However, regarding the intention to purchase farmed fish, mixed results were obtained, with consumers having almost equal low and high purchase intentions.
Table 7. Respondents’ frequency and levels of their given scores for different constructs.
Results and discussion of the hypothesis testing
The primary aim of the study was to investigate the effect of ambiguity tolerance and confusion avoidance on farmed fish purchase intention. It also attempts to explore the impact of ambiguity tolerance on confusion avoidance. Therefore, to test the hypothesized relationship, several hypotheses were developed and tested in light of previous research. shows the results in support of acceptance and rejection of the hypotheses and the SEM. The table indicates that four hypotheses were tested, two of which were found to be statistically significant. In H1, ambiguity tolerance was significant in a trend and positively influenced purchase intention (β = 0.06, S.E = 0.034, C.R = 1.726, and P <.10). Earlier works on ambiguity found that it only affected purchase intention negatively (Hazen et al. Citation2012) and reduced consumers’ confidence choice (Wang and Shukla Citation2013). In contrast, Mitchell, Walsh, and Yamin (Citation2005) found that ambiguity tolerance could moderately influence marketing information on consumer confusion and choice behavior. The literature also revealed that customer support and web content reduced product ambiguity, increasing consumers’ AT (Fang Citation2019). AT is the essential trait that facilitates creative work (Zenasni, Besançon, and Lubart Citation2008), encouraging the production of a large number of ideas (Runco Citation2014), and being associated with esthetic preferences (Furnham and Avison Citation1997).
Table 8. Results of structural equation modeling: standardized path estimates.
Regarding H2, ambiguity tolerance had a strong association with confusion avoidance (β = 0.12, S.E = 0.028, C.R = 4.130 and P <.01). Walsh, Hennig-Thurau, and Mitchell (Citation2007) argue that confusion could arise from information ambiguity and from consumers’ intolerance for processing unclear, misleading, or conflicting product-related information or advertisements. Therefore, it can be affirmed that ambiguity-tolerant customers are more likely to avoid confusion.
Hypothesis H3 was rejected (β = 0.002; S.E = 0.037; C.R = 0.063; P >.10), implying that CA has no significant association with purchase intention. This complies with Walsh and Mitchell et al.’s (Citation2010) findings, that the absence of confusion does not motivate consumers to purchase, nor leads to satisfaction. Additionally, Wang and Shukla (Citation2013) and Mitchell and Papavassiliou (Citation1999) found that overload confusion affected decision postponement, while Trivikram (Citation2017) advocates certain initiatives to avoid consumers’ confusion about a particular product, such as explaining product features, ingredients, benefits, product familiarity, product uniqueness, and promising to deliver better services. This study added increased confusion-avoiding helps to grow consumers’ attitudes toward that product. This study also attempts to explore the mediating effect of CA, AT and PI. For the study model, three tests, Sobel, Aroian and Goodman, were employed, and the results show that the test statistics are minimal in all three tests (β = 0.05) and that the p-values are greater than 0.05. These results do not provide evidence to support H4.
Furthermore, the study attempts to explore the relationships between demographics and socioeconomic variables on purchase intention. From the results, it can be seen that income and age have a strong negative relationship with purchase intention. In contrast, the presence of children in the family is positively associated with consumers’ purchase intention. The literature also suggests that consumers’ choice of fish products is influenced by demographic variables, such as age, gender and children. The research study confirms a significant relationship between AT and CA, and AT and PI. The SEM also demonstrates that the number of family members, education, age, gender, and profession do not affect farmed fish purchase intention.
Regression results
Using multivariate regression analysis, the variation of the independent variables in relation to the dependent variable was observed synchronically. A multiple regression model was deployed, as shown in EquationEquation (1)(1)
(1) . The main objective of the regression analysis was to assess the effects of the sociodemographic variables on consumers’ perceived value of CA and AT. Therefore, two independent regression models (models 1 and 2) were employed, and the results are shown in .
where, = dependent variable,
= independent variable,
parameter, and
= error. The resulting empirical specification for EquationEquation (1)
(1)
(1) is
EquationEquation (2)(2)
(2) is the CA (ca) equation, while EquationEquation (3)
(3)
(3) is the AT (at) equation. In both equations, each score is predicted by consumers’ age (age), income (inc), presence of children in the family (pcl), number of family members (nfm), education in years (edu), if they do the food shopping for the family (fsf), and membership of an environmental club (mec).
Before running the models, using the “avplots” command in STATA, we observed all the added variable plots to reveal any problematic observations in the models. The results demonstrate that all the variables in the models were useful. To check the multicollinearity of the predictors, the VIF and tolerance (TOL) techniques were used. The results show that the VIF score is minimal (less than 10), and the TOL score is greater than 0.10, meaning that our results are free from the multicollinearity problem. Although model 2 deviates slightly from normality, it is good in model 1. In both models, the test of “hatsq” and the Ramsey RESET test are not significant, indicating that “linktest” and “ovtest” failed to reject the assumption that the models were specified correctly. Therefore, we have no specification error.
The regression results () indicate that age has a negative and significant influence on consumers’ perceived values of ambiguity tolerance. AT is particularly useful when people solve problems in a group (Runco Citation2014). Therefore, consumers who are members of a volunteer environmental club are more likely to be ambiguity tolerant. In contrast, members of an environmental club are less likely to avoid CA. This is because their inability to differentiate between unreliable (e.g., greenwash) and credible sources of information indicates that they express frustration and blame policymakers’ confusion (Cornish and Moraes Citation2015). On the contrary, a longer education and responsibility for food shopping for the family could help consumers distinguish between fake and real information sources, thereby leading to increased CA. A high level of education also supports increased consumer AT (Fang Citation2019), and the presence of children in the family also helps adults to increase their CA. Therefore, such presence is significantly correlated with farmed fish PI. However, the presence of children in the family does not influence consumers’ AT substantially. Finally, even though its influence is low, level of income reduces CA significantly. In general, income helps to increase consumers’ purchasing power. However, when they receive an ever-increasing volume of decision-relevant information in their purchasing environment, confusion became more of a problem (Mitchell and Papavassiliou Citation1999).
Table 9. Results of the two regression models: the effects of demographics on confusion avoidance and ambiguity tolerance.
Conclusion and implications
As earlier studies have not focused on the impact of ambiguity tolerance and confusion avoidance, this study has examined how consumers’ farmed fish purchase intention is affected by these two factors. Second, consumers’ fish consumption patterns and the association between sociodemographics and farmed fish purchase intention are examined. This contribution also determines the effect of sociodemographics on confusion avoidance and ambiguity tolerance. To achieve the aims, a questionnaire survey was conducted to collect relevant data and to consider peoples’ level of agreement with the best option regarding their purchase intentions. Consequently, explorative factor analysis, structural equation modeling and simple regression analysis were performed to analyze the data. The paper’s main theoretical contribution is that it conceptualizes and develops the modeling of a set of perceived values of consumers’ ambiguity tolerance and confusion avoidance, and examines the influences of these two factors on their farmed fish purchase intention in an emerging country, namely Bangladesh.
The findings of the study lead to the drawing of various conclusions. First, consumers have a high level of ambiguity tolerance that positively and significantly affects their farmed fish buying intention. The study also found that ambiguity-tolerant consumers can avoid confusion, whereas it cannot be asserted that confusion avoidance has a positive association with the intention to purchase fish from aquaculture. Even confusion avoidance cannot mediate the relationship between ambiguity tolerance and consumers’ farmed fish purchase intent. Second, income and membership of a volunteer environmental club help reduce, while length of education, presence of children in the family, and frequent fish shopping for the family help decrease confusion avoidance. Greater age leads to a decline in consumers’ ambiguity tolerance, whereas environmental club membership encourages an increase in it. Third, consumers’ purchase intent is negatively correlated with their age and income, indicating that older and high-income people are less likely to buy farmed fish.
The study outcomes have important implications for academicians, marketers and policymakers who wish to promote farmed fish cultivation and consumption. One factor that may have affected the results is that as consumers develop their levels of ambiguity tolerance, the need to control ambiguous information regarding the production process and consumption effect of farmed fish increases, thus resulting in higher tolerance of ambiguity. Evidence shows that risk information, adaption to change, and solving the problems associated with farmed fish production and consumption will increase consumers’ tolerance of ambiguity to such fish. This ambiguity tolerance also allows consumers to engage in creative work, produce new ideas, and develop esthetic preferences. Greater use of aquatic technology by fish farmers in disease prevention, fishmeal replacement, aquaculture sustainability, and established blockchains in aquaculture could be the worthy creative goals. However, communication of the sustainability of aquaculture is challenging, and the growth of the market requires intensive action, including the overall value chain, with the retail sector being considered as the main actor (Zander et al. Citation2018). Such creative work could help consumers to form a positive attitude to farmed fish, and subsequently these positive attitudes will enhance their intention to purchase the fish.
Another aspect of ambiguity that has been explored in this study is that ambiguity tolerance affects confusion avoidance positively. The findings reveal that consumers who are able to decide between farmed fish with less or incorrect information are most likely to avoid confusion caused by aquaculture information overload. Information explaining the features, familiarity and uniqueness of farmed fish, and the provision of excellent service by the producers and marketers of the fish, would help to boost the level of confusion avoidance. However, this general or overload information does not help to influence consumers’ buying intention directly, as those who are willing to avoid confusion will avoid knowing more, as they are not enthusiastic about processing more information regarding farmed fish and forming a negative attitude toward its purchase. Therefore, to convince consumers, more appealing information showing the creativity and potential of aquaculture, and the cultural connotations of fish consumption, is required. Consequently, an effective marketing strategy focusing on innovation and communication will positively affect farmed fish. As most of the sampled consumers had a high level of ambiguity tolerance, farmers’ innovations might help increase purchase intention. In addition, customer support and web content regarding farmed fish could help increase ambiguity tolerance for consumers who have a low or medium level.
However, the following limitations should be considered for further research. First, although our research sample was not so small but the strata was not defined strategically. For example, the northern part of the country has excluded capital rationing. However, studies could be conducted in a larger area to generalize the results. A cross-cultural sample could also be employed to assess the variability of the results. Second, this study focused on the quantitative aspect; combining this with a qualitative approach would help better achieve the objective. Finally, other factors may be associated with ambiguity tolerance and confusion avoidance, such as preferences for sensory attributes, consumers’ product knowledge, and behavioral action analysis that can provide a more straightforward concept regarding farmed fish purchase intention.
Acknowledgments
The authors would like to thank the anonymous reviewers for their thoughtful and detailed comments on the development of the papers’ quality.
Disclosure statement
No potential conflict of interest was reported by the authors.
References
- ADB. 2005. An evaluation of small-scale freshwater rural aquaculture development for poverty reduction. Metro Manila, Philippines: Operations Evaluation Department, Asian Development Bank. Asian Development Bank Publications.
- Addeah, D. F., K. K. Quagrainie, and S. Chenyambuga. 2016. Consumer preferences for farmed tilapia in Tanzania: A choice experiment analysis. Journal of Applied Aquaculture 28 (3):131–43. doi:https://doi.org/10.1080/10454438.2016.116996.
- Ahmed, M., and M. A. Rab. 1992. Feasibility of adopting aquaculture to increase resource productivity in existing Bangladesh farming systems. NAGA, WorldFish Center Quarterly 15 (4):21–22.
- Ahmed, M., and M. A. Rab. 1994. Sustainable aquaculture in small water bodies: Experiences from Bangladesh. Wuxi, China: Integrated Fish Farming, CRC Press.
- Ahmed, M. N. U. 2003. Fisheries in the economy of Bangladesh and development potential. In: Fish week compendium, 11–15. Dhaka, Bangladesh: Department of Fisheries, Ministry of Fisheries and Livestock.
- Alarabi, S., and S. Grönblad. 2012. The effects of consumer confusion on decision postponement and brand loyalty in a low involvement product category, 53. Sweden: Department of Business Studies, Uppsala University.
- Altintzoglou, T., W. Verbeke, F. Vanhonacker, and J. Luten. 2010. The image of fish from aquaculture among Europeans: Impact of exposure to balanced information. Journal of Aquatic Food Product Technology 19 (2):103–19. doi:https://doi.org/10.1080/10498850.2010.492093.
- Amberg, S. M., and T. E. Hall. 2008. Communicating risks and benefits of aquaculture: A content analysis of US newsprint representations of farmed salmon. Journal of the World Aquaculture Society 39:143–57. doi:https://doi.org/10.1111/j.1749-7345.2008.00160.x.
- Awang, Z. H. 2012. A handbook on SEM: Structural equation modeling. 4th ed. ed. Kualalumpur: Centre For Graduate Studies, University Teknologi MARA Kelantan.
- Azim, M. E., and M. A. Wahab. 2002. Status of aquaculture and fisheries in Bangladesh. World Aquaculture 67:37–40.
- Babin, B. J., W. R. Darden, and M. Griffin. 1994. Work and/or fun: Measuring hedonic and utilitarian shopping value. Journal of Consumer Research 20 (4):644–56. doi:https://doi.org/10.1086/209376.
- Bardi, A., V. M. Guerra, and G. S. D. Ramdeny. 2009. Openness and ambiguity intolerance: Their differential relations to well-being in the context of an academic life transition. Personality and Individual Differences 47 (3):219–23. doi:https://doi.org/10.1016/j.paid.2009.03.003.
- Baron, R. M., and D. A. Kenny. 1986. The moderator–mediator variable distinction in social psychological research: Conceptual, strategic, and statistical considerations. Journal of Personality and Social Psychology 51 (6):1173–82. doi:https://doi.org/10.1037/0022-3514.51.6.1173.
- Bentall, R. P., and R. Swarbrick. 2003. The best laid schemas of paranoid patients: Autonomy, sociotropy and need for closure. Psychology and Psychotherapy: Theory, Research and Practice 76 (2):163–71. doi:https://doi.org/10.1348/147608303765951195.
- Bollen, K. A., and K. A. Bollen. 1989. Introduction. In Structural equations with latent variables, ed. K. A. Bollen. New York, NY: Willey. doi:https://doi.org/10.1002/9781118619179.ch1.
- Brown, T. A. 2006. Confirmatory factor analysis for applied research. New York, NY: The Guilford Press.
- Carlucci, D., G. Nocella, B. De Devitiis, R. Viscecchia, F. Bimbo, and G. Nardone. 2015. Consumer purchasing behaviour towards fish and seafood products. Patterns and insights from a sample of international studies. Appetite 84:212–27. doi:https://doi.org/10.1016/j.appet.2014.10.008.
- Chowdhury, M. H., and K. L. Maharjan. 2001. Pond fish production through people’s participation in rural Bangladesh. Journal of Development and Cooperation 7 (2):11–28.
- Claret, A., L. Guerrero, I. Gartzia, M. Garcia-Quiroga, and R. Ginés. 2016. Does information affect consumer liking of farmed and wild fish? Aquaculture 454:157–62. doi:https://doi.org/10.1016/j.aquaculture.2015.12.024.
- Claret, A., L. Guerrero, R. Ginés, A. Grau, M. D. Hernández, E. Aguirre, J. B. Peleteiro, C. Fernández-Pato, and C. Rodríguez-Rodríguez. 2014. Consumer beliefs regarding farmed versus wild fish. Appetite 79:25–31. doi:https://doi.org/10.1016/j.appet.2014.03.031.
- Cornish, L., and C. Moraes. 2015. The impact of consumer confusion on nutrition literacy and subsequent dietary behavior. Psychology & Marketing 32:558–74. doi:https://doi.org/10.1002/mar.20800.
- Das, M., M. Islam, T. Akter, A. Kawser, and M. Mondal. 2018. Present status, problems and prospect of fish farming at Gazipur Sadar upazila in Bangladesh. Progressive Agriculture 29 (1):53–63. doi:https://doi.org/10.3329/pa.v29i1.37480.
- DeRoma, V. M., K. M. Martin, and M. L. Kessler. 2003. The relationship between tolerance for ambiguity and need for course structure. Journal of Instructional Psychology 30 (2):104–09.
- Dey, M., R. Briones, Y. Garcia, A. Nissapa, U. Rodriguez, R. Talukder, A. Senaratne, I. Omar, S. Koeshendrajana, N. Khiem, et al. 2008. Strategies and options for increasing and sustaining fisheries and aquaculture production to benefit poorer households in Asia, Vol. 1823, 180. Penang, Malaysia: The World Fish Center.
- Dey, M. M., M. F. Alam, and M. L. Bose. 2010. Demand for aquaculture development: Perspectives from Bangladesh for improved planning. Reviews in Aquaculture 2 (1):16–32. doi:https://doi.org/10.1111/j.1753-5131.2010.01020.x.
- Dhar, R. 1997. Consumer Preference for a No‐Choice Option. Journal of Consumer Research 24 (2):215–31. doi:https://doi.org/10.1086/209506.
- DoF. 2018. Yearbook of fisheries statistics of Bangladesh, 2017-18. Fisheries Resources Survey System (FRSS), Department of Fisheries, vol. 35, 129. Bangladesh: Ministry of Fisheries.
- DoF-BARC. 2001. Fisheries sector review and ten-year (2002-2012) production projection. Dhaka: Department of Fisheries, Ministry of Fisheries and Livestock.
- Dollinger, M. J., T. Saxton, and P. A. Golden. 1995. Intolerance of ambiguity and the decision to form an alliance. Psychological Reports 77 (3_suppl):1197–98. doi:https://doi.org/10.2466/pr0.1995.77.3f.1197.
- Duarte, C. M., M. Holmer, Y. Olsen, D. Soto, N. Marbà, J. Guiu, K. Black, and I. Karakassis. 2009. Will the oceans help feed humanity? BioScience 59 (11):967–76. doi:https://doi.org/10.1525/bio.2009.59.11.8.
- Eloi, J., E. Lopez-Valeiras, and M. B. Gonzalez-Sanchez. 2020. The role of attitudes and tolerance of ambiguity in explaining consumers’ willingness to pay for organic wine. Journal of Cleaner Production 257 (1):120601. doi:https://doi.org/10.1016/j.jclepro.2020.120601.
- Fang, P.-H. 2019. Customer confusion: Product overlap, ambiguous needs, and information overload. An examination of corporate mental models for derivative product creation and their impact on customers. MSc Thesis in Engineering in Applied Mechanics, National Taiwan University.
- FAO. 2000. Sustainable contribution of fisheries to food security. RAP Publication: 2000/23, Asia-Pacific Fishery Commission Food and Agriculture Organization of the United Nations Regional Office for Asia and the Pacific.
- FAO. 2013. Characteristics, structure and resources of the sector. FAO Cauntry Notes 1380: 1–16.
- FAO. 2018. The state of world fisheries and aquaculture 2018 - Meeting the sustainable development goals. Rome, Italy: FAO.
- FAOSTAT. 2016. Statistical data base. Rome, Italia: Food and Agriculture Organizations of the United Nations.
- Feather, N. T. 1969. Cognitive differentiation, attitude strength, and dogmatism. Journal of Personality 37 (1):111–26. doi:https://doi.org/10.1111/j.1467-6494.1969.tb01734.x.
- Fishbein, M., and I. Ajzen. 1975. Belief, attitude, intention and behaviour: An introduction to theory and research. Boston, MA: Addison-Wesley.
- Fornell, C., and D. F. Larcker. 1981. Evaluating structural equation models with unobservable variables and measurement error. Journal of Marketing Research 18 (1):39. doi:https://doi.org/10.2307/3151312.
- Forza, C., and R. Filippini. 1998. TQM impact on quality conformance and customer satisfaction: A causal model. International Journal of Production Economics 55 (1):1–20. doi:https://doi.org/10.1016/S0925-5273(98)00007-3.
- Frishammar, J., H. Florén, and J. Wincent. 2009. Patterns of uncertainty and equivocality during predevelopment: Findings from process-based firms. Proceedings of the IAMOT 2009: 18th International Conference on Management of Technology, Miami, FL, United States.
- Funge-Smith, S., M. Briggs, and W. Miao. 2012. Regional overview of fisheries and aquaculture in Asia and the Pacific 2012. RAP publication. http://www.fao.org/documents/card/en/c/951b0503-aece-5bdf-a4fa-500b868b55a1/.
- Furnham, A., and M. Avison. 1997. Personality and preference for surreal paintings. Personality and Individual Differences 23 (6):923–35. 0191-8869. doi:https://doi.org/10.1016/S0191-8869(97)00131-1.
- Furnham, A., and T. Ribchester. 1995. Tolerance of ambiguity: A review of the concept, its measurement and applications. Current Psychology 14 (3):179–99. doi:https://doi.org/10.1007/BF02686907.
- Gaskell, G. 2010. Communication, perception and participation of the consumer. The Federal Agency for the Safety of the Food Chain, Nanotechnology in the Food Chain:Opportunities and Risks, Proceedings of the International Symposium in Brussels, Belgium, 24 November 2010, 77–83.
- Gaviglio, A., and E. Demartini. 2009. Consumer attitudes towards farm-raised and wild-caught fish: Variables of product perception New Medit 8:34–40.
- Gentry, R. R., H. E. Froehlich, D. Grimm, P. Kareiva, M. Parke, M. Rust, S. D. Gaines, and B. S. Halpern. 2017. Mapping the global potential for marine aquaculture. Nature Ecology & Evolution 1 (9):1317–24. doi:https://doi.org/10.1038/s41559-017-0257-9.
- Gordon, T. A. C., H. R. Harding, F. K. Clever, I. K. Davidson, W. Davison, D. W. Montgomery, R. C. Weatherhead, F. M. Windsor, J. D. Armstrong, A. Bardonnet, et al. 2018. Fishes in a changing world: Learning from the past to promote sustainability of fish populations. Journal of Fish Biology 92:804–27. doi:https://doi.org/10.1111/jfb.13546.
- Greenspoon, P. J., and D. H. Saklofske. 1998. Confirmatory factor analysis of the multidimensional students’ life satisfaction scale. Personality and Individual Differences 25 (5):965–71. doi:https://doi.org/10.1016/S0191-8869(98)00115-9.
- Grewal, D., J. Gotlieb, and H. Marmorstein. 1994. The moderating effects of message framing and source credibility on the price-perceived risk relationship. Journal of Consumer Research 21 (1):145. doi:https://doi.org/10.1086/209388.
- Guadagnoli, E., and W. F. Velicer. 1988. Relation of sample size to the stability of component patterns. Psychological Bulletin 103 (2):265–75. doi:https://doi.org/10.1037/0033-2909.103.2.265.
- Gupta, M., M. Ahmed, and M. Bimbao. (1992). Socioeconomic impact and farmers’ assessment of Nile tilapia (Oreochromis niloticus) culture in Bangladesh. ICLARM Technical Report, 35, 50.
- Gupta, M., M. A. Mazid, M. S. Islam, and M. Rahman. 1999. Integration of aquaculture into farming systems of the flood prone ecosystem in Bangladesh: An evaluation of adoption and impact. ICLARM Technical Report 56:32.
- Gupta, M., and M. A. Rab. 1994. Adoption and economics of silver barb (Puntius gonionotus) culture in seasonal waters in Bangladesh. Manila, Philippines: International Centre for Living Aquatic Resources Management.
- Hair, J. F., W. C. Black, B. J. Babin, and R. E. Anderson. 2010. Multivariate data analysis. 7th ed. Upper Saddle River, NJ: Pearson Prentice Hall.
- Hair Joseph, F., W. C. Black, B. J. Babin, and R. E. Anderson. 1998. Multivariate data analysis. Prentice Hall 5 (3):207–19.
- Haque, A., N. Anwar, F. Yasmin, A. Sarwar, Z. Ibrahim, and A. Momen. 2015. Purchase intention of foreign products: A study on Bangladeshi consumer perspective. SAGE Open 5 (2):1–12. doi:https://doi.org/10.1177/2158244015592680.
- Hauser, J. R., B. Wernerfelt, and B. Wernerfelt. 1990. An evaluation cost model of consideration sets. The Journal of Consumer Reasearch 16 (4):393–408. doi:https://doi.org/10.1086/209225.
- Hazen, B. T., R. E. Overstreet, L. A. Jones-Farmer, and H. S. Field. 2012. The role of ambiguity tolerance in consumer perception of remanufactured products. International Journal of Production Economics 135 (2):781–90. doi:https://doi.org/10.1016/j.ijpe.2011.10.011.
- Hernandez, R.A., Belton, B., Reardon, T., Hu, C., Zhang, X., Ahmed, A., 2018. The ‘quiet revolution’ in the aquaculture value chain in Bangladesh. Aquaculture 493(1): 456–468. doi:https://doi.org/10.1016/j.aquaculture.2017.06.006.
- Hongskul, V. 1999. Into the next millennium: Fishery perspective. RAP Publication, November, 26. http://www.tistr.or.th/rap/publication/1999/1999_26_high.pdf.
- Hoque, M. S., L. Jacxsens, B. De Meulenaer, and A. K. M. N. Alam. 2016. Quantitative risk assessment for formalin treatment in fish preservation: food safety concern in local market of Bangladesh. Procedia Food Science 6 (Icsusl2015):151–58. doi:https://doi.org/10.1016/j.profoo.2016.02.037.
- Hoque, M. Z. 2020. Sustainability indicators for sustainably-farmed fish in Bangladesh. Sustainable Production and Consumption 27 (115–127):10–22. doi:https://doi.org/10.1016/j.spc.2020.10.020.
- Hoque, M. Z., J. Xie, and S. Nazneen. 2018. Effect of labelled information and sensory attributes on consumers’ intention to purchase milk. South Asian Journal of Business Studies 7 (3):265–86. doi:https://doi.org/10.1108/SAJBS-02-2018-0021.
- Hoque, M. Z., and M. N. Alam. 2020. Consumers’ knowledge discrepancy and confusion in intent to purchase farmed fish. British Food Journal 122 (11):3567–83. doi:https://doi.org/10.1108/BFJ-01-2019-0021.
- Iyengar, S. S., and M. R. Lepper. 2000. When choice is demotivating: Can one desire too much of a good thing? Journal of Personality and Social Psychology 79 (6):995–1006. doi:https://doi.org/10.1037/0022-3514.79.6.995.
- Karim, M., M. Ahmed, R. K. Talukder, and M. A. Taslim. 2006. Dynamic agribusiness- focused aquaculture for poverty reduction and economic growth in Bangladesh. WorldFish Center Discussion Series 1:44.
- Keltner, D., and M. N. Shiota. 2003. New displays and new emotions: A commentary on Rozin and Cohen (2003). Emotion 3 (1):86–91. doi:https://doi.org/10.1037/1528-3542.3.1.86.
- Khan, M. S. 1986. Socio-economic factors in the development of fisheries. Bangladesh Journal of Agricultural Economics 10:43–47.
- Klerck, D., and J. C. Sweeney. 2007. The effect of knowledge types on consumer perceived risk and adoption of genetically modified foods. Psychology & Marketing 24:171–93. doi:https://doi.org/10.1002/mar.20157.
- Lachance, S., R. Ladouceur, and M. J. Dugas. 1999. Elements d’explication de la tendance a s’inquieter. Applied Psychology 48 (2):187–96. doi:https://doi.org/10.1080/026999499377600.
- Lee, S., J. E. Workman, and K. Jung. 2016. Brand relationships and risk: Influence of risk avoidance and gender on brand consumption. Journal of Open Innovation 2:14. doi:https://doi.org/10.1186/s40852-016-0041-0.
- Lewis, D. 1997. Rethinking aquaculture for resource poor farmers: Perspective from Bangladesh. Food Policy 22 (6):533–46. doi:https://doi.org/10.1016/S0306-9192(98)00006-2.
- Litman, J. 2005. Curiosity and the pleasures of learning: Wanting and liking new information. Cognition & Emotion 19 (6):793–814. doi:https://doi.org/10.1080/02699930541000101.
- Little, D. C., R. W. Newton, and M. C. M. Beveridge. 2016. Aquaculture: A rapidly growing and significant source of sustainable food? Status, transitions and potential. Proceedings of the Nutrition Society 75 (3):274–86. doi:https://doi.org/10.1017/S0029665116000665.
- Lysonski, S. 1990. Effects of moderating variables on product managers’ behavior. Psychological Reports 66 (1):295. doi:https://doi.org/10.2466/PR0.66.1.295-306.
- Mac Donald, A. P. 1970. Revised scale for ambiguity tolerance: Reliability and validity. Psychological Reports 26 (3):791–98. doi:https://doi.org/10.2466/pr0.1970.26.3.791.
- Maheswaran, D., and J. Meyers-Levy. 1990. The influence of message framing and issue involvement. Journal of Marketing Research 27 (3):361. doi:https://doi.org/10.2307/3172593.
- Mancuso, T., L. Baldi, and L. Gasco. 2016. An empirical study on consumer acceptance of farmed fish fed on insect meals: The Italian case. Aquaculture International 24:1489–507. doi:https://doi.org/10.1007/s10499-016-0007-z.
- Mazid, M. A. 1999. Rural and coastal aquaculture in poverty reduction. Proceedings of a seminar organized on the occasion of fish week, 79. Dhaka, Bangladesh: Department of Fisheries.
- Mclain, D. L. 1993. The Mstat-I: A new measure of an individual’s tolerance for ambiguity. Educational and Psychological Measurement 53 (1):183–89. doi:https://doi.org/10.1177/0013164493053001020.
- McLain, D. L. 2009. Evidence of the properties of an ambiguity tolerance measure: The Multiple Stimulus Types Ambiguity Tolerance Scale–II (MSTAT–II). Psychological Reports 105 (3):975–88. doi:https://doi.org/10.2466/PR0.105.3.975-988.
- McLain, D. L., E. Kefallonitis, and K. Armani. 2015. Ambiguity tolerance in organizations: Definitional clarification and perspectives on future research. Frontiers in Psychology 6 (MAR):1–7. doi:https://doi.org/10.3389/fpsyg.2015.00344.
- Mitchell, V. W., G. Walsh, and M. Yamin. 2004. Reviewing and redefining the concept of consumer confusion, version-3. Manuscript Manchester School of Management.
- Mitchell, V. W., and V. Papavassiliou. 1999. Marketing causes and implications of consumer confusion. Journal of Product & Brand Management 8 (4):319–42. doi:https://doi.org/10.1108/10610429910284300.
- Mitchell, V.-W., G. Walsh, and M. Yamin. 2005. Association for Consumer Research Towards a Conceptual Model of Consumer Confusion. 32, 143–50. http://www.acrwebsite.org/volumes/9058/volumes/v32/NA-32.
- Mueller, S., and G. Szolnoki. 2010. The relative influence of packaging, labelling, branding and sensory attributes on liking and purchase intent: Consumers differ in their responsiveness. Food Quality and Preference 21 (7):774–83. doi:https://doi.org/10.1016/j.foodqual.2010.07.011.
- Myrland, Ø., T. Trondsen, R. S. Johnston, and E. Lund. 2000. Determinants of seafood consumption in Norway: Lifestyle, revealed preferences, and barriers to consumption. Food Quality and Preference 11 (3):169–88. doi:https://doi.org/10.1016/S0950-3293(99)00034-8.
- Naylor, R. L., R. J. Goldburg, J. H. Primavera, N. Kautsky, M. C. M. Beveridge, J. Clay, C. Folke, J. Lubchenco, H. Mooney, and M. Troell. 2000. Effect of aquaculture on world fish supplies. Nature 405 (6790):1017–24. doi:https://doi.org/10.1038/35016500.
- Nesheim, M. C., and A. L. Yaktine. 2007. Seafood choices: Balancing benefits and risks. Washington: National Academies Press.
- O’Rourke, D., and A. Ringer. 2016. The impact of sustainability information on consumer decision making. Journal of Industrial Ecology 20 (4):882–92. doi:https://doi.org/10.1111/jiec.12310.
- Olesen, I., T. Gjedrem, H. B. Bentsen, B. Gjerde, and M. Rye. 2003. Breeding programs for sustainable aquaculture. Journal of Applied Aquaculture 13 (3–4):179–204. doi:https://doi.org/10.1300/J028v13n03_01.
- Olsen, S. O. 2003. Understanding the relationship between age and seafood consumption: The mediating role of attitude, health involvement and convenience. Food Quality and Preference 14 (3):199–209. doi:https://doi.org/10.1016/S0950-3293(02)00055-1.
- Pallant, J. 2007. SPSS survival manual: A step by step guide to data analysis using SPSS for windows version 15. Maidenhead: Open University Press/McGraw-Hill.
- Rahman, M., S. Ahmed, M. Hosen, and A. K. Talukder. 2012. Detection of formalin and quality characteristics of selected fish from wet markets at Sylhet City In Bangladesh. Bangladesh Research Publications Journal 7 (2):161–69.
- Ramalho Ribeiro, A., T. Altintzoglou, J. Mendes, M. L. Nunes, M. T. Dinis, and J. Dias. 2019. Farmed fish as a functional food: Perception of fish fortification and the influence of origin – Insights from Portugal. Aquaculture 501:22–31. doi:https://doi.org/10.1016/j.aquaculture.2018.11.002.
- Richardson, P. S., A. K. Jain, and A. Dick. 1996. Household store brand proneness: A framework. Journal of Retailing 72 (2):159–85. doi:https://doi.org/10.1016/S0022-4359(96)90012-3.
- Rickertsen, K., F. Alfnes, P. Combris, G. Enderli, S. Issanchou, and J. F. Shogren. 2017. French consumers’ attitudes and preferences toward wild and farmed fish. Marine Resource Economics 32 (1):59–81. doi:https://doi.org/10.1086/689202.
- Roos, N., M. A. Wahab, M. A. R. Hossain, and S. H. Thilsted. 2007. Linking human nutrition and fisheries: Incorporating micronutrient-dense, small indigenous fish species in carp polyculture production in Bangladesh. Food and Nutrition Bulletin 28 (2_suppl2):S280–S293. doi:https://doi.org/10.1177/15648265070282S207.
- Runco, M. A. 2014. Personality and motivation, chapter 9, creativity, 2nd ed, 265–302. San Diego, CA: Academic Press. ISBN 9780124105126. doi:https://doi.org/10.1016/B978-0-12-410512-6.00009-6.
- Schlag, A., and K. Ystgaard. 2013. Europeans and aquaculture: Perceived differences between wild and farmed fish. British Food Journal 115:209–22. doi:https://doi.org/10.1108/00070701311302195.
- Schneider, F. W., J. A. Gruman, and L. M. Coutts. 2012. Applied social psychology: Understanding and addressing social and practical problems. Los Angeles: Sage.
- Schreiber, J. B., A. Nora, F. K. Stage, E. A. Barlow, and J. King. 2006. Reporting structural equation modeling and confirmatory factor analysis results: A review. The Journal of Educational Research 99 (6):323–38. doi:https://doi.org/10.3200/JOER.99.6.323-338.
- Schweizer, M., A. J. Kotouc, and T. Wagner. 2006. Scale development for consumer confusion. Advances in Consumer Research 33 (May2014):184–90.
- Sekaran, U. 2003. Research methods for business: A skill-building approach. 4th ed. New York: John Wiley & Sons.
- Shafir, E., I. Simonson, and A. Tversky. 2000. Reason-based choice. In Choice, val- ues and frames, ed. D. Kahneman and A. Tversky, 597–619. Cambridge, UK: Cambridge University Press.
- Stanley Budner, N. Y. 1962. Intolerance of ambiguity as a personality variable. Journal of Personality 30 (1):29–50. doi:https://doi.org/10.1111/j.1467-6494.1962.tb02303.x.
- Steadman, L., and D. R. Rutter. 2004. Belief importance and the theory of planned behaviour: Comparing modal and ranked modal beliefs in predicting attendance at breast screening. British Journal of Health Psychology 9 (4):447–63. doi:https://doi.org/10.1348/1359107042304579.
- Steginga, S. K., and S. Occhipinti. 2004. The application of the heuristic-systematic processing model to treatment decision making about prostate cancer. Medical Decision Making 24 (6):573–83. doi:https://doi.org/10.1177/0272989X04271044.
- Tabachnick, B. G., and L. S. Fidell. 2001. Using multivariate statistics. 4th ed. Boston: Allyn and Bacon.
- Thompson, P. M., P. Sultana, and F. K. A. Nuruzzaman Md. 2005. Aquaculture extension impacts in Bangladesh: A case study from Kapasia, Gazipur. Penang, Malaysia: WorldFish Center.
- Thong, N. T., and H. S. Solgaard. 2017. Consumer’s food motives and seafood consumption. Food Quality and Preference 56:181–88. doi:https://doi.org/10.1016/j.foodqual.2016.10.008.
- Trivikram, S. 2017. How to prevent customer confusions. Accessed May 02, 2020, https://myventurepad.com/prevent-customer-confusions/.
- Trondsen, T., J. Scholderer, E. Lund, and A. E. Eggen. 2003. Perceived barriers to consumption of fish among Norwegian women. Appetite 41 (3):301–14. doi:https://doi.org/10.1016/S0195-6663(03)00108-9.
- Turnbull, P. W., S. Leek, and G. Ying. 2000. Customer confusion: The mobile phone market. Journal of Marketing Management 16 (1–3):143–63. doi:https://doi.org/10.1362/026725700785100523.
- Van Hook, C. W. 2002. Individual personality characteristics related to suggestibility. Psychological Reports 91 (7):1007. doi:https://doi.org/10.2466/PR0.91.7.1007-1010.
- Vanhonacker, F., T. Altintzoglou, J. Luten, and W. Verbeke. 2011. Does fish origin matter to European consumers? British Food Journal 113 (4):535–49. doi:https://doi.org/10.1108/00070701111124005.
- Verbeke, W., I. Sioen, K. Brunsø, S. De Henauw, and J. Van Camp. 2007. Consumer perception versus scientific evidence of farmed and wild fish: Exploratory insights from Belgium. Aquaculture International 15 (2):121–36. doi:https://doi.org/10.1007/s10499-007-9072-7.
- Verbeke, W., and I. Vackier. 2005. Individual determinants of fish consumption: Application of the theory of planned behaviour. Appetite 44 (1):67–82. doi:https://doi.org/10.1016/j.appet.2004.08.006.
- Walsh, G., T. Hennig-Thurau, and V.-W. Mitchell. 2007. Consumer confusion proneness: Scale development, validation, and application. Journal of Marketing Management 23 (7–8):697–721. doi:https://doi.org/10.1362/026725707X230009.
- Walsh, G., and V. Mitchell. 2010. The effect of consumer confusion proneness on word of mouth, trust, and customer satisfaction. European Journal of Marketing 44 (6):838–59. doi:https://doi.org/10.1108/03090561011032739.
- Walsh, K. 1999. Marketing and public sector management. European Journal of Marketing 28 (3):63–71. doi:https://doi.org/10.1108/03090569410057308.
- Wang, Q., and P. Shukla. 2013. Linking sources of consumer confusion to decision satisfaction: The role of choice goals. Psychology & Marketing 30 (4):295–304. doi:https://doi.org/10.1002/mar.20606.
- Watterson, A., D. Little, J. A. Young, K. Boyd, E. Azim, and F. Murray. 2008. Towards integration of environmental and health impact assessments for wild capture fishing and farmed fish with particular reference to public health and occupational health dimensions. International Journal of Environmental Research and Public Health 5 (4):258–77. doi:https://doi.org/10.3390/ijerph5040258.
- Wittenberg, K. J., and J. C. Norcross. 2001. Practitioner perfectionism: Relationship to ambiguity tolerance and work satisfaction. Journal of Clinical Psychology 57 (12):1543–50. doi:https://doi.org/10.1002/jclp.1116.
- World Bank. 2013. Fish to 2030. Prospects for fisheries and aquaculture. World Bank Report Number 83177-Glb.
- Yurtsever, G. 2001. Tolerance of ambiguity, information, and negotiation. Psychological Reports 89 (1):57–64. doi:https://doi.org/10.2466/pr0.2001.89.1.57.
- Yurtsever, G. 2008. Negotiators’ profit predicted by cognitive reappraisal, suppression of emotions, misrepresentation of information, and tolerance of ambiguity. Perceptual and Motor Skills 106 (2):590–608. doi:https://doi.org/10.2466/pms.106.2.590-608.
- Zander, K., and Y. Feucht. 2018. Consumers’ Willingness to Pay for Sustainable Seafood Made in Europe. Journal of International Food & Agribusiness Marketing 30 (3):251–75. doi:https://doi.org/10.1080/08974438.2017.1413611.
- Zenasni, F., M. Besançon, and T. Lubart. 2008. Creativity and tolerance of ambiguity: An empirical study. Journal of Creative Behavior 42 (1):61–72. doi:https://doi.org/10.1002/j.2162-6057.2008.tb01080.x.
Appendix A.
Ambiguity Tolerance and Confusion Avoidance in the Purchase Intention of Farmed Fish (an Aquaculture Product): A Survey Questionnaire, January 2019
Section 1
Please read the texts below with the visual information carefully, and then go to section 2.Section 2
Please rate the statements that comes to your mind since the rated feelings probably closest to your real purchase in markets.
Section 3: Personal Characteristics a) Age: … … … … … … … … … … … …. b) Gender:Male/Female/Don’t want to mention. c) Income/month (Taka): … … … … … … … … … … … . d) Children (age 1-16) in household:Yes/No e) Number of family members: … … … … … … … … … … … f) Your years of education: … … … … … … … … … … g) Your profession:Self-employed/Employed h) Do you do most of the food shopping for your family?Yes/No i) Overall fish consumption:Less-than once a month/Once a month/Several times a month/Once a weekSeveral times a week/Daily j) I buy fish from:Wet market/Supermarket Both k) Are you a registered member of any environmental (volunteer) club?Yes/No Thanks for your valuable time!