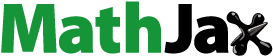
ABSTRACT
Languages differ regarding the depth, structure, and syntactic domains of recursive structures. Even within a single language, some structures allow infinite self-embedding while others are more restricted. For example, when expressing ownership relation, English allows infinite embedding of the prenominal genitive -s, whereas the postnominal genitive of is much more restricted. How do speakers learn which specific structures allow infinite embedding and which do not? The distributional learning proposal suggests that the recursion of a structure (e.g., X1’s-X2) is licensed if the X1 position and the X2 position are productively substitutable in non-recursive input. The present study tests this proposal with an artificial language learning experiment. We exposed adult participants to X1-ka-X2 strings. In the productive condition, almost all words attested in X1 position were also attested in X2 position; in the unproductive condition, only some were. We found that, as predicted, participants from the productive condition were more likely to accept unattested strings at both one- and two-embedding levels than participants from the unproductive condition. Our results suggest that speakers can use distributional information at one-embedding level to learn whether or not a structure is recursive.
1. Introduction
This study investigates the learning mechanism that enables speakers to determine which structures are recursive in a given language. Recursion refers to infinite self-embedding of a particular type of linguistic element or grammatical structure. Many linguists and cognitive scientists agree that the ability for recursion is a crucial part of the language faculty and is universal across languages (e.g., Pinker Citation1994, Hauser et al. Citation2002).Footnote1 However, languages differ regarding the depth, structure, and syntactic domains of recursive structures (e.g., Pérez-Leroux et al. Citation2018). Indeed, even within a single language, some structures are more restricted than others. For example, when expressing ownership relation, English allows infinite embedding with the prenominal s-possessive, (1a), whereas the postnominal of-possessive is much more limited, (1b), (see Levi Citation1978, Biber et al. Citation1999, Rosenbach Citation2014 for extensive discussion). Given the cross- and within-linguistic differences in recursive structures, speakers have to learn from language-specific experience in which syntactic domains the ability of recursion can be applied. Thus, what kind of linguistic experience do they use, and how do they make use of it?
One line of research has proposed that learners determine that a structure can be used recursively by observing evidence for recursive embedding in their input (e.g., Roeper & Snyder Citation2005, Roeper Citation2011). Under this account, the learner starts with the default assumption that a structure cannot be used recursively, and this assumption is only revised when recursive embedding of the structure is observed in the input (e.g., the man’s neighbor’s book). This mechanism prevents overgeneralization of restricted structures like of-possessive (1b), but is challenged by the empirical fact that evidence for recursive embedding is rarely attested in young children’s input. For example, Giblin et al. (Citation2019) examined caregivers’ speech in CHILDES (MacWhinney Citation2000), where they found only 107 recursive s-genitives in 3.1 million English utterances, and no recursive genitives with the productive genitive marker de in three Mandarin corpora. These findings predict the acquisition of recursive structures to be very difficult, if not impossible, under the recursive embedding approach. Yet, despite the paucity of explicit evidence in the input, several behavioral experiments have reported early acquisition of recursive structures. For instance, 4-year-old English- and Mandarin-speaking children can comprehend and produce multi-level recursive s- or de-possessives (e.g., Giblin et al. Citation2019, Li et al. Citation2020) — an unexpected finding if children solely rely on direct evidence of recursive embedding.
Moreover, there is a logical problem of learning recursive structures: no N-level embedding entails even N+1 levels of embedding. Thus, any learning mechanism should explain how native speakers learn that recursive structures can embed deeper than have been observed in the input, and ultimately, how they learn that recursive structures can stack infinitely when examples in the input are always finite.
Recently, an alternative mechanism for learning recursive structures was proposed (Grohe et al. Citation2021, Li et al. Citation2021), which relies on distributional learning (e.g., Maratsos & Chalkley Citation1980, Braine Citation1987). It has been suggested that the recursivity of a structure is related to its productivity in one-level non-embedded data (e.g., Pérez-Leroux et al. Citation2022). The distributional learning proposal (Grohe et al. Citation2021, Li et al. Citation2021) further suggests that recursion can be viewed as structural substitutability. That is, for a structure such as X1’s-X2, where X is the head of the structure and X1 and X2 stand in a selectional relation, it is recursive if position X1 and X2 are productively substitutable, i.e., any noun that appears in one of those positions can also be used in the other position. For example, as demonstrated in Li et al. Citation2021, for the English possessive X1’s-X2, all nouns used in X1 can be used in X2 as well (denoted by X1↦X2), that is, the possessor can always be possessed, thus allowing infinite embedding to be built in this way. Therefore, according to this approach, children learn recursion by learning the lexicon for which structural substitutability holds. For example, if the phrases the mother’s car and the boy’s mother are attested in one’s linguistic input, then the s-possessive is recursive at least for the word mother, and therefore mother’s mother … can infinitely embed. If there are multiple words attested in both positions, then the learner will seek to form generalizations over those attested words: If there is sufficient evidence that structural substitutability is generalizable— that is, if a sufficiently large proportion of words attested in one position are also attested in the other position—then the child will acquire the generalization that all words that can be used in one position (e.g., X1) can also be used in the other (e.g., X2) and therefore the structure can recursively embed for all words eligible for X1; otherwise, the structure is restricted to certain (types of) words attested in both positions in the input. Thus, under the distributional learning account, children discover whether a structure allows recursion in the same way they discover other productive generalizations in their language.
Importantly, Grohe et al. (Citation2021) and Li et al. (Citation2021) argue that the fact that multi-level recursive embedding is rarely attested in input to children is no longer a problem under their distributional learning proposal. Learners can discover structural substitutability (and therefore that a structure allows recursion) by utilizing distributional information at one level of embedding. Grohe et al. (Citation2021) and Li et al. (Citation2021) further argue that the distributional learning proposal addresses the logical problem of learning recursive structures, because it predicts that a structure is either infinitely recursive or must stop at one-level: If structural substitutability holds at one level, then the structure allows infinite embedding for all the words that follow the generalization acquired from one-level data.
While there are other approaches to the structural representation of recursive structures and its relation to acquisition (e.g., Adger Citation2003, Hartmann & Zimmermann Citation2002), the distributional learning proposal is unique in that head and selection are the only structural assumptions required. Li et al. (Citation2021) note that the head requirement is necessary because only when X is the head of the structure does the structure involve self-embedding, which is the definition of recursion. For instance, in the possessive structure N1’s-N2,N2 is the head of the structure (e.g., ‘the neighbor’s book’ is essentially an instance of book), therefore, productive substitutability would lead to recursion under this proposal because the notion of the head establishes an equivalence relation between a head noun and all syntactic objects headed by that noun. In contrast, in English NP1-V-NP2 structures (e.g., ‘dogs chase cats’), for example, neither of the two NPs is the head of the structure, so substitutability would not lead to recursion (e.g., ‘*dogs chase cats chase rats’) although NP1 and NP2 can be substitutable. Importantly, while the distributional learning proposal does not itself rely on any complex syntax/semantics machinery, it does not need to be incompatible with the existing syntactic theories of recursive structures either. Instead, it offers an account for how children learn from their sparse input when recursion is allowed and when it is not.
Li et al. (Citation2021) argue that the distributional learning proposal should apply to all recursive structures that satisfy the head and selection requirements,Footnote2 and have provided initial support for this claim with corpus studies on a range of different structures across languages. Grohe et al. (Citation2021), for example, found that for determiner-adjective1-adjective2-noun strings in English and German input corpora, adjective1 and adjective2 are fully substitutable in both languages according to one measure of productivity: the Tolerance/Sufficiency Principle (TSP; Yang Citation2016);Footnote3 arguing that the productivity and recursion of prenominal adjective stacking can therefore be learned through distributional cues in the two languages.Footnote4 Li et al. (Citation2021) similarly examined productively recursive and restricted possessive structures in Mandarin Chinese, English, and German, and confirmed that the distributional learning proposal can account for the recursivity of such structures. First, for freely recursive structures without any restriction - German von-possessive and Mandarin de-possessive, the study found N1 and N2 are bi-directionally substitutable, so children should learn those structures can be freely embedded. English s-possessive and of-possessive are both one-way substitutable: It’s N1↦N2 for the s-possessive and N2↦N1 for the of-possessive, where N2 is the possessee. Therefore, those structures should only be recursive for the types of words eligible for N1 in the s-possessive and for N2 in the of-possessive, and children need to discover what nouns are eligible for those positions and thus trigger recursion. Through semantic analyses of attested words in the input, Li et al. (Citation2021) found children can discover many of the well-documented restrictions on those structures for recursive embedding: e.g., alienable possession is freely available in the s-possessive (e.g. ‘neighbor’s book’) whereas of-possessive is largely limited to inalienable possession (e.g. ‘end of the story’); and the possessee in the of-possessive must be inanimate as a rule (Levi Citation1978, Biber et al. Citation1999, Rosenbach Citation2014). When those constraints are met, the restricted of-possessive can be embedded as well, for example, ‘the top of the tip of the hat’. Please see Li et al. (Citation2021) for more detailed discussions on the acquisition of recursion with constraints. Finally, for German s-possessive and the possessive without de in Mandarin, the proportion of nouns appearing in both positions fail to meet the threshold of productivity for each direction, so depending on individual’s linguistic experience, those structures will either be recursive only for the highly limited words attested in both positions, or not recursive at all because lexicalization in the absence of productivity requires extensive exposure, which is not guaranteed for all speakers. Indeed, surveys by the authors with native speakers of those languages found considerable individual differences regarding whether those structures can be possibly embedded.Footnote5
In summary, the distributional learning proposal offers a novel account of how speakers learn which structures allow recursion in a given language. Previous corpus studies have provided initial evidence in support of the proposal, showing—across a variety of structures and languages—that there is reliable distributional information in one-level input to acquire recursive structures. However, more work is needed to determine whether such a distributional learning mechanism would indeed enable speakers to discover which structures are recursive in any given language. Certainly, the proposal should be evaluated on a range of linguistic phenomena, not only beyond those including in Grohe et al. (Citation2021) and Li et al. (Citation2021), but also including structures for which the constraints on recursion are undeniably more complex. Equally important, however, is the need to examine human learning behavior, to determine whether learners can make use of distributional information as predicted by the account. In other words, it is not enough to show that a given type of distributional information is available in the learner’s input; one must also demonstrate that human learners can make use of this available information during learning.
In the present study, we use an artificial language learning paradigm to test the proposal in precisely this way: when provided with one-level distributional information and no semantic information, do adults learn recursive structures as predicted by the account? To preview the experiment, in two conditions, participants were exposed to one-level X1-ka-X2 strings in an artificial language. We manipulated the distribution of words in the exposure so that the X1 and X2 positions are productively substitutable in one condition, but not in the other. At test, we asked participants to rate one- and two-level X1-ka-X2 strings that were never attested during exposure, together with attested and ungrammatical controls. If speakers indeed use one-level distributional information to learn recursive structures as predicted by the distributional learning proposal, then participants exposed to productive input should rate the unattested strings higher than participants exposed to unproductive input, since the former group are predicted to be be more likely to acquire the generalization of structural substitutability and extend it to unattested words. We present and discuss the experiments in the following sections.
2. MethodFootnote6
2.1. Participants
Participants were 50 adult native English speakers with typical hearing and vision (or corrected vision). All participants were recruited and run online via Prolific Academic (www.prolific.ac) and paid $9/hour as compensation. The 50 participants were assigned to one of two language conditions, Productive or Unproductive, though 2 participants in the Unproductive condition did not complete the experiment and were excluded from analysis. The final sample of participants includes 48 adults, with 23 in the Unproductive condition (age = 30.48, range = 19-47) and 25 in the Productive condition (age = 27.42,Footnote7 range = 19-40).
2.2. Stimuli
The exposure stimuli in both conditions consisted of 44 strings generated from an artificial grammar of the form X1-ka-X2, where X1 and X2 denote the position in the structure (pre- or post-ka, respectively). In addition to the functional morpheme -ka-, the artificial language contained 12 nonsense words adapted from Ruskin (Citation2014), all of which were mono- or bi-syllabic words that conformed to English phonotactics.
In both conditions, all 12 words were attested in the X1 position during language exposure (see ). Crucially, we manipulated the number of words that were also attested in the X2 position, ensuring there was sufficient evidence for structural substitutability X1↦X2 in the Productive condition (10 of the 12 words attested in X2) but not in the Unproductive condition (6 of the 12 words attested in X2). We selected 10 of 12 in the Productive condition and 6 of 12 in the Unproductive condition because these values are consistent with productivity (or lack of productivity in the Unproductive condition) according to several different metrics. For example, some metrics require a pattern to apply to the majority of types in order to meet the threshold for productive generalization (e.g., Bybee Citation1995). Here, structural substitutability is predicted to be productive if at least 7 of our 12 words are also attested in X2 position. Other metrics require a larger proportion of words to be attested in X2 position in order to be considered productive. For example, the Tolerance/Sufficiency Principle (Yang Citation2016) proposes that a rule R defined over N items productively generalizes if the number of exceptions to the rule is less than or equal to the number of items divided by the natural log of the number of items (e ≤ N/lnN). Here, the Tolerance/Sufficiency Principle permits at most 4 exceptions to structural substitutability (12/ln12 = 4.83), meaning at least 8 of our 12 words must also be attested in X2 position for the rule to generalize. Still other metrics generate an index of productivity—typically a value between 0 and 1—to capture the intuition that the more items a pattern applies to, the more likely it is to be productive. The Word-Form Rule (Aronoff Citation1976, Baayen & Lieber Citation1991), for example, states that the productivity of a given structure can be quantified as the number of items the structure applies to divided by the number of items it could potentially apply to. Here, our values of 6 (Unproductive condition) and 10 (Productive condition) out of 12 words correspond to a productivity index of 0.50 and 0.83, respectively. Importantly, while our conditions are consistent with each of these metrics of productivity, our goal in the current experiment is not to distinguish between these different metrics; instead, our stimuli were designed to meet all of these metrics to ensure only one of our input conditions provides evidence for productivity during exposure.
Table 1. The distribution of words in the 44 string exposure corpus and word frequency in X1/X2 position.
The exposure set was also constructed such that some words were more frequent than others in order to imitate word frequency in natural language input. To keep the two conditions balanced, we kept the total token frequency of each word the same across the two conditions, and ensured the most frequent word was attested in both the X1 and X2 positions in both conditions. We also ensured that both high and low token frequency words were among the words that did not occur in the X2 position. The distribution of the words and their frequencies across conditions and X-positions in the exposure set are shown in .
The test strings were generated to include either one (X1-ka-X2) or two levels (X1-ka-X2-ka-X3) of embedding. At each level, there were three types of test strings: attested, unattested, and ungrammatical, where attested/unattested means whether the words have been attested at the specific position during exposure, not necessarily whether the string as a whole has been attested. Attested strings were strings or combinations of two strings that had been heard during exposure (i.e., were part of the exposure set). For example, as shown in , for a one-level string, it means the exact string (e.g., waso-ka-mito) has been heard during exposure phase; for a two-level string, it means both components (e.g., sane-ka-kewa and kewa-ka-nogi) have been heard. Therefore, all the words have been attested in those positions in relation to ka during exposure. In unattested strings, the post-ka positions (X2 and X3) were occupied by a word that never appeared in X2 position during exposure. Thus, in the unattested strings in , sane, tesa and tana have never been attested after ka. Finally, ungrammatical strings were strings with wrong word order, such as ka-X1-X2 or ka-X1-X2-X3-ka. There were six test strings of each type at each level, leading to 36 test strings in total. We designed our test strings such that in each string type, there were both words of higher frequency and words of lower frequency, in order to avoid the influence of token frequency in the test. The test strings were delivered in random order.
Table 2. Sample test strings in Unproductive condition.
All exposure and test strings were generated by a female voice using an online speech synthesizer, Natural Reader. We generated each unique string separately such that all strings were generated with the same speed, volume, and pitch.
2.3. Procedure
The experiment consisted of two phases: exposure, in which participants were exposed to the artificial language, and test, in which participants were tested on how well they learned and whether they formed a productive generalization. In the exposure phase, participants were told they would hear strings from a new language, and to pay careful attention to the strings, because they would be tested on their knowledge of the language later. During exposure, participants heard two repetitions of the exposure corpus (44 X1-ka-X2 strings) presented in pseudo-random order as they viewed a still, unrelated nature scene (i.e., there was no accompanying referential world). There was 1.5s of silence between each string, and participants were offered a break after each repetition of the 44 strings to prevent task fatigue. In order to make sure that the participants were paying attention, other sounds were randomly dispersed among the linguistic strings, such as bird chirping sounds, and participants were later asked how many such sounds they heard. The random sounds occurred only rarely so as not to interfere with the learning of the language (i.e., 2 or 3 times per block). All participants answered those questions correctly.
Once the exposure phase was completed, the test phase began. On each test trial, participants heard a test string, and were asked to rate the acceptability of the string on a scale of 1 to 5. Participants were told to decide if those strings came from the language that they had just heard (e.g., whether they think a native speaker of the language would have said that particular string). 1 meant the string was definitely not from the language; 2 meant the string may not have come from the language; 3 meant the string may or may not have come from the language; 4 meant the string may have come from the language; 5 meant the string definitely came from the language.
In both conditions, participants are expected to rate attested strings significantly higher than ungrammatical strings at both levels. Of particular interest are the unattested strings. According to the distributional learning proposal, only participants in the Productive condition would learn that X1↦X2 is productive in the X1-ka-X2 structure, and would thus generalize this pattern to unattested words: If a word appeared in position X1 during exposure, it must be able to appear in position X2 as well, even though it was never attested there in the input. On the other hand, X1↦X2 is not productive in the Unproductive condition: for words that only appeared in position X1, participants would be more likely to think that those words cannot appear in position X2. Therefore, it is predicted that the rating score for one-level unattested strings relative to one-level ungrammatical strings by participants in the Productive condition should be higher than that by participants in the Unproductive condition. Furthermore, given the productivity of the structure at level-one, participants in the Productive condition would acquire the generalization that X1↦X2 holds for any level so all of the 12 words can be used in both X1 and X2 positions to create recursive embedding, but for participants in the Unproductive condition, they would be more likely to learn that the words unattested in X2 position cannot appear after ka at any level and that recursive embedding is only possible with the attested words. Thus, participants in the Productive condition are predicted to rate two-level unattested strings higher than participants in the Unproductive condition as well.
3. Results
3.1. Raw Scores
The individual rating scores by condition, embedding level, and test string type are summarized in . We analyzed the results using ordinal regression, with rating score as an ordered factor from 1 to 5, Condition (Unproductive, Productive), Level (as an ordered factor 1 or 2) and test string Type (attested, unattested, or ungrammatical) as fixed effects, and by-participant random intercepts and random slopes for Type. None of Condition, Level or Type is a significant predictor of the rating score, but their three-way interaction is (p < 0.001). Specifically, attested strings were rated significantly higher than unattested strings (β = -1.23, SE = 0.29, z = -4.24, p < 0.001) and ungrammatical strings (β = -3.14, SE = 0.28, z = -11.03, p < 0.001); 1-level strings were rated significantly higher than 2-level strings (β = -1.38, SE = 0.22, z = -6.33, p < 0.001); and 2-level unattested (β = -1.28, SE = 0.44, z = -2.90, p = 0.004) and ungrammatical strings (β = -1.39, SE = 0.45, z = -3.09, p = 0.002) were rated lower in the Unproductive condition. The interaction between Type and Condition is also a significant predictor of the rating score (p = 0.002), suggesting unattested strings were rated higher in the Productive condition. Therefore, as predicted, the results show that participants in the Productive condition rated unattested strings at both levels higher than participants in the Unproductive condition, suggesting that speakers can indeed use one-level distributional information to learn about recursive structures.
Table 3. Individual learning (L) and generalization (G) indices and rating scores for attested (A), unattested (UA), and ungrammatical (UG) sentences, ordered by participant learning score at 1-level embedding.
What we are most interested in this study, though, is not just the raw rating score for each type of test strings and their difference per se, but rather how well participants learned the input language and whether (and how much) they generalized. Therefore, in order to more directly and more informatively capture the phenomena of interest, in addition to our analysis of the raw rating scores, we also calculated and analyzed a learning index and a generalization index, which measured participants learning and generalization, with their ratings for ungrammatical test strings as the baseline. The details are described in the following subsections.
3.2. Learning
To capture how well participants acquired their input language, we calculated a learning index for each participant. We took the difference score of a participant’s mean response on Attested test sentences minus their mean response on Ungrammatical test sentences (see (2)). We calculated this index separately for one-level and two-level test sentences. For one-level test sentences, a positive learning index would suggest that a participant rated X1-ka-X2 sentences they heard during exposure (Attested) as more consistent with the language than ka-X1-X2 sentences that violated the structure of the input grammar (Ungrammatical). For two-level sentences, a positive learning index would suggest that a participant rated two-level sentences whose post-ka positions (X2 and X3) were occupied by words attested in X2 position during exposure (Attested) as more consistent with the input language than two-level sentences with the -ka morpheme in the wrong position (ungrammatical, e.g., ka-X1-X2-X3-ka). Participants were expected to learn the basics of the artificial language regardless of condition, so we do not predict Condition to be a significant predictor of the learning index, which should have a positive score for both conditions.
(2)
shows individual learning indices, and shows the mean learning index by input condition and embedding level. As shown in the figure, participants not only learned the grammar (had a positive learning index on one-level sentences), but also endorsed two-level embedding for words attested in both X1 and X2 position during exposure. Our mixed effects regression model showed that there is no significant main effect of Condition (χ2(1) = 0.49, p = 0.48) or Level (χ2(1) = 0.51, p = 0.48), indicating participants in both conditions learn the grammar equally well. However, there is a significant interaction between Condition and Level (χ2(1) = 9.50, p = 0.002): Participants in the Unproductive condition rated two-level sentences significantly higher (β = 0.74, SE = 0.23, t = 3.17, p = 0.003), suggesting that participants were more willing to endorse two-level recursion for attested sentences in the Unproductive condition. This may not be surprising, given that fewer X words are allowed in both positions in that condition (6 of 12) compared to the Productive condition (10 of 12), so they are easier to learn. Therefore, overall, the results on the learning index indicate the participants in both conditions have learned the basic pattern of the artificial grammar.
3.3. Generalization
To determine whether participants formed a productive generalization permitting words attested in X1 position to also appear in X2 position, we also calculated a generalization index for each participant. Here, we took the difference score of a participant’s mean response on unattested test sentences minus their mean response on ungrammatical test sentences (see (3)). As with the learning index, we calculated the generalization index separately for one- and two-level test sentences. At both levels of embedding, a positive generalization index would suggest that a participant rated unattested sentences (whose post-ka positions, X2 and X3, were occupied by words never attested in X2 position during exposure) as more consistent with the language than ungrammatical sentences that violated the structure of the input grammar. For 1-level strings, this generalization index measures how much participants would generalize substitutability to unattested words. For 2-level strings, this measures how likely participants would accept recursive strings using unattested words based on substitutability at level-one. Since the Unproductive condition did not provide enough evidence for productive substitutability in the input, we predict the generalization index should be higher in the Productive condition than the Unproductive condition at both levels.
(3)
and show individual generalization indices by input condition and embedding level. A mixed effects regression model showed there is a significant main effect of Condition (χ2(1) = 10.07, p = 0.002), which indicates that participants in the Unproductive condition generalized significantly less (β = -0.56, SE = 0.17, t = -3.28, p = 0.002). There is also a significant main effect of Level (χ2(1) = 7.41, p = 0.006), indicating that participants were significantly less likely to generalize at two levels of embedding (β = -0.40, SE = 0.15, t = -2.76, p = 0.008). There is no significant interaction between Condition and Level (χ2(1) = 0.03, p = 0.86). Therefore, the results suggest that as predicted, participants generalized more in the Productive condition than in the Unproductive condition at both levels of embedding. This supports the proposal that speakers can use one-level distributional information to learn about recursive structures. However, in both conditions, they were less likely to generalize for two-level sentences. In the next section, we will discuss this pattern of results in more detail and explore how it relates to findings from natural language.
4. Discussion
In this study, we investigated whether speakers can learn recursive structures purely based on the productivity of structure substitutability in simple one-level embedding data. The distributional learning proposal argues that, for a structure such as X1-ka-X2, if a large enough proportion of words are attested in both the X1 and X2 positions in one-level input, then speakers can acquire the generalization that the two positions are productively substitutable. That means if a word is attested in one position, then it is able to appear in the other position as well, even though it has never been attested in the other position in the input. Furthermore, once a structure is productive at one level, speakers will learn that it can be embedded to any level. In contrast, if the number of words attested in both positions in the input does not reach the productivity threshold, speakers will assume the positions are not substitutable and thus the structure cannot be embedded further, except for specific items that have been attested in the input. We found that as predicted, participants exposed to productive input were significantly more willing to generalize to unattested sentences at both one and two levels than participants exposed to unproductive input. Therefore, our results suggest that learners can indeed access and utilize the distributional information as the distributional learning approach proposes. Together with previous corpus studies which demonstrated the availability and reliability of distributional information for structural substitutability in naturalistic data (Grohe et al. Citation2021, Li et al. Citation2021), the findings indicate that the recursivity of a structure can be learned distributionally from language-specific level-one experience. Therefore, overall, the results imply that recursivity can be viewed as a productive generalization, which can be acquired through distributional learning. This learning mechanism also avoids the logical problem of learning recursive structures, since it does not rely on explicit evidence of deep embedding; instead, it predicts that a structure can be recursively embedded once it is productive at one level. Therefore, this learning mechanism enables speakers to acquire knowledge of infinite embedding from finite input data.
The results of this study add to a body of work that investigates how distributional information can be utilized to acquire higher-order linguistic representations (e.g., Reeder et al. Citation2013, Schuler et al. Citation2017). We would like to make it clear that we are not arguing that children acquire the ability of recursion through distributional learning. Instead, we are interested in whether learners can use distributional information to learn to which specific structures this ability of recursion can be applied, which must be learned from language specific experience. Furthermore, the present study is focused on what speakers can learn about recursive structures from distributional information alone, and our results indicate distributional information itself already allows learners to distinguish structures that can be recursively embedded from restricted structures. However, we do not deny the important role of other factors, such as the well-recorded semantic, pragmatic, and phonetic constraints for the English of-genitive (e.g., Rosenbach Citation2014), in the acquisition of recursive structures. Rather, we consider this work a first step toward future investigations into how learners coordinate and exploit different cues to learn which structures are recursive and the constraints on this recursion in the language they are acquiring. And we agree that other tests of the full range of the distributional learning proposal are welcome and necessary.
One apparent difference between the distributional learning proposal and the current results is that while the proposal predicts that learners will learn that infinite embedding is allowed once there is sufficient evidence for substitutability in one-level input, our participants were less likely to generalize at two-level even in the Productive condition. Indeed, we agree that in principle, the distributional learning account would predict a categorical difference in linguistic knowledge: The unattested strings of both embedding levels in the Productive condition should be completely good, while the unattested strings of both embedding levels in the Unproductive condition should be completely bad. While the distributional learning proposal predicts perfect linguistic ability, participants’ judgement in experiments are naturally imperfect, and influenced by processing factors. Indeed, even experiments with natural language have found that native speakers experience difficulty processing grammatical but recursively embedded structures, and their ratings for the structures get lower with increasing levels of embeddings. For instance, in Christiansen & MacDonald’s (Citation2009) study, participants rated different recursive structures, such as PPs, possessives and central embeddings, and for all the structures, deeper embeddings were rated significantly worse. Further, the pattern to be learned in the study is complex, and the duration of the exposure phase is brief. That is, our participants are new learners of the artificial language. As such we did not expect our learners to be perfect generalizers, even in the Productive condition. Instead, the crucial finding is as predicted by the distribution learning proposal, participants in the productive condition do generalize to both one- and two-level sentences, and they do so significantly more strongly than those in the Unproductive condition. Future studies should try different tasks such as production or forced alternative choice tasks to further investigate the nature of learners’ linguistic knowledge.
Another important question is whether the learners in our experiments acquired a hierarchical structure from the artificial language input or if they simply acquired the linear order of strings. Generalizing the X1-ka-X2 structure to X1-ka-X2-ka-X3 involves tail-recursion, which, in the absence of a referential world, could be accomplished with simple iteration. We agree that our design does not rule out the possibility that learners may not have acquired a hierarchical structure from our language input. However, some artificial language learning studies have found that if human learners can apply certain distributional learning strategy to linear strings, they are also able to apply it to hierarchical structures (Thompson & Newport Citation2007, Takahashi & Lidz Citation2008). Therefore, even though what our participants have learned is a linear structure, we think they are also likely to learn hierarchical structures with the same mechanism. We plan to test this by constructing an explicitly hierarchical language as in Thompson & Newport (Citation2007) and Takahashi & Lidz (Citation2008).
Another possible interpretation of the results is that participants were learning categories: In the Productive condition, they learned all the words belong to one productive category, whereas in the Unproductive condition, they learned the words belong to different categories and are thus uninterchangeable. We suggest this interpretation is not necessarily inconsistent with the distributional learning proposal. For example, the corpus study in Li et al. (Citation2021) showed that for recursive possessive structures in natural languages, all the words appearing in either position can be viewed as belonging to one productive category; in contrast, for restricted structures, the words which can be used in certain position do form semantic subcategories. For instance, for the restricted possessive structure X1’s-X2 in German, words that are attested in X1 are limited to close kinship terms. We will examine the exact relation and distinction between categories and recursion in future research. Another future direction is the role of the structural representation in the distributional learning of recursive structures. In particular, a requirement by the distributional learning proposal is that the substitutable element must be the head of the structure, whereas the current artificial language did not explicitly provide this information. However, given the word length (words in X1 and X2 positions tend to be longer than ka), stress (X1 and X2 words are stressed, ka is never stressed) and word number (there are 12 X words but only 1 ka word) in the current artificial language, we think it is likely that participants will treat X as the head of the structure and ka as a function word, since those cues also apply in natural languages. Moreover, in ongoing work, we are explicitly testing the role of the structural representation by explicitly approximating the distribution of heads in the new artificial languages. Preliminary results suggest that both substitutability and knowledge of the head are necessary for the acquisition of recursion.
Finally, the present experiments have examined adult participants. However, it is unknown whether young learners can also fully utilize such distributional information, given their more limited cognitive abilities. Previous studies have suggested that children and even infants can learn grammatical rules through distributional learning (e.g., Marcus et al. Citation1999, Emond & Shi Citation2021), but the rule to be learned in this study is more abstract than those investigated before. In addition, some studies suggested that distributional learning is an ability available from birth (e.g., Gervain et al. Citation2008, Teinonen et al. Citation2009, Aslin Citation2017). Therefore, it is necessary for future research to examine whether young learners exploit the distributional cues in the same way as the adults in the present study, and at what age this distributional learning is available.
Acknowledgments
We are grateful to the participants in our experiment; to Charles Yang and members of the Child Language Lab and the Language and Cognition Lab at University of Pennsylvania for helpful discussion; and to three anonymous reviewers whose comments improved the paper.
Disclosure statement
The authors report no conflicts of interest. The authors alone are responsible for the content and writing of this article.
Data availability statement
The data that support the findings of this study are available from the corresponding author upon reasonable request.
Additional information
Funding
Notes
1 We are also aware of a long tradition of research on the learning and processing constraints on recursion, e.g., center embedding (e.g., Roth Citation1984, Karlsson Citation2007, Christiansen & MacDonald Citation2009). Our study, though, does not rely on assumptions of the status of recursion, and explores the learnability problem from a different approach, namely how recursive structures can be learned from one-level embedding input.
2 Recursive structures where the crucial elements are not in a selectional relation are not the focus of the distributional learning proposal. Li et al. (Citation2021) proposed that they can also be learned distributionally but in a slightly different way. For instance, for the so-called CP-recursion (e.g., ‘John thinks that Bill said that Mary left’), the crucial element that decides whether a CP can be embedded inside another is whether proper CP-taking verbs are used. Since the two verbs in the configuration (e.g., ‘said’ and ‘think’) are in different CPs, they cannot select each other. Therefore, in order to learn this recursion, learners will need to learn (i) there are verbs that can take CP complements (e.g., ‘John said [CP].’); (ii) those same verbs can be the main verb in an embedded CP (e.g., ‘[John said it].’). Therefore, to learn (i) and (ii), children still need to learn that the two verb positions are substitutable. Thus, the idea of substitutability works for all recursive structures, but applies in slightly different ways depending on whether there is a selectional relation.
3 One reviewer asked about details of the calculation using TSP in Grohe et al. Citation2021 and Li et al. Citation2021. Given the TSP formula e ≤ N/lnN, N is the number of words in a child’s vocabulary that are attested in a certain position of a structure, and e is the number of words out of N that are not attested in the other position of that structure. Both N and e are obtained from input corpus data.
4 Some languages have preferences for the order of adjectives when they stack (e.g., in English, ‘the second green ball’ is usually preferred over ‘the green second ball’). Grohe et al. (Citation2021) and Li et al. (Citation2021) argue that the distributional learning proposal only concerns whether recursion can possibly be allowed by grammar (‘the green second ball’ is not necessarily ungrammatical in felicitous contexts, e.g., given that there are several rows and there is a ‘second ball’ in each row, one wants to stress the green one); the preference will be learned via other mechanisms such as variational or reinforcement learning.
5 More discussion on how the proposal works for structures from a typologically diverse range of languages can be found in Li et al. (Citation2021) and Li & Yang (Citationin prep).
6 An earlier version of this experiment was reported in the Proceedings of the 43rd Annual Meeting of the Cognitive Science Society (Li & Schuler Citation2021).
7 One participant in the productive condition declined to report age.
References
- Adger, David. 2003. Core syntax. Oxford: Oxford University Press.
- Aronoff, Mark. 1976. Word formation in generative grammar. Cambridge, MA: MIT Press.
- Aslin, Richard N. 2017. Statistical learning: A powerful mechanism that operates by mere exposure. WIREs Cognitive Science 8. e1373.
- Baayen, Harald & Rochelle Lieber. 1991. Productivity and English derivation: A corpus-based study. Linguistics 29(5). 801–843.
- Biber, Douglas, Stig Johansson, Geoffrey Leech, Susan Conrad & Edward Finegan. 1999. Longman grammar of spoken and written English. London: Longman.
- Braine, Martin D. S. 1987. What is learned in acquiring word classes – A step toward an acquisition theory. In Brian MacWhinney (ed.), Mechanisms of language acquisition, 65–87. Mahwah, NJ: Lawrence Erlbaum.
- Bybee, Joan. 1995. Regular morphology and the lexicon. Language and Cognitive Processes 10(5). 425–455.
- Christiansen, Mortan H. & Maryellen C. MacDonald. 2009. A usage-based approach to recursion in sentence processing. Language Learning 59. 126–161.
- Emond, Emeryse, & Rushen Shi. 2021. Infants’ rule generalization is governed by the Tolerance Principle. In Danielle Dionne & Lee-Ann Vidal Covas (eds.), Proceedings of the 45th annual Boston University Conference on Language Development [BUCLD 45], 191–204. Somerville, MA: Cascadilla Press.
- Gervain, Judit, Francesco Macagno, Silvia Cogoi, Marcela Pena & Jacques Mehler. 2008. The neonate brain detects speech structure. Proceedings of the National Academy of Sciences of the United States of America [PNAS] 105. 14222–14227.
- Giblin, Iain, Peng Zhou, Cory Bill, Jiawei Shi & Stephen Crain. 2019. The Spontaneous eMERGEnce of recursion in child language. In Megan M. Brown & Brady Dailey (eds.), Proceedings of the 43rd annual Boston University Conference on Language Development [BUCLD 43], 270–285. Somerville, MA: Cascadilla Press.
- Grohe, Lydia, Petra Schulz & Charles Yang. 2021. How to learn recursive rules: Productivity of prenominal adjective stacking in English and German. Paper presented at the 9th biannual conference on Generative Approaches to Language Acquisition – North America, May 7-9, University of Iceland, Reykjavík.
- Hartmann, Katharina & Malte Zimmermann. 2002. Syntactic and semantic adnominal genitive. In Claudia Maienborn (ed.), A-symmetrien – A-symmetries, 171–202. Tübingen: Stauffenburg.
- Hauser, Marc D., Noam Chomsky & W. Tecumseh Fitch. 2002. The faculty of language: What is it, who has it, and how did it evolve? Science 298(5598). 1569–1579.
- Karlsson, Fred. 2007. Constraints on multiple center-embedding of clauses. Journal of Linguist 43. 365–392.
- Levi, Judith. N. 1978. The syntax and semantics of complex nominals. Cambridge, MA: Academic Press.
- Li, Daoxin, Lydia Grohe, Petra Schulz & Charles Yang. 2021. The distributional learning of recursive structures. In Danielle Dionne & Lee-Ann Vidal Covas (eds.), Proceedings of the 45th annual Boston University Conference on Language Development [BUCLD 45], 471–485. Somerville, MA: Cascadilla Press.
- Li, Daoxin & Kathryn Schuler. 2021. Distributional learning of recursive structures. In Proceedings of the 43rd Annual Conference of the Cognitive Science Society [CogSci 2021], 1437-1443.
- Li, Daoxin & Charles Yang. In prep. Productivity and the distributional learning of recursive structures.
- Li, Daoxin, Xiaolu Yang, Tom Roeper, Michael Wilson, Rong Yin, Jaieun Kim, Emma Merritt, Diego Lopez & Austin Tero. 2020. Acquisition of recursion in child Mandarin. In Megan M. Brown & Alexandra Kohut (eds.), Proceedings of the 44th annual Boston University Conference on Language Development [BUCLD 44], 294–307. Somerville, MA: Cascadilla Press.
- MacWhinney, Brian. 2000. The CHILDES project. Mahwah, NJ: Lawrence Erlbaum.
- Maratsos, Michael P. & Chalkley, M. A. 1980. The internal language of children’s syntax: The nature and ontogenesis of syntactic categories. In Keith Nelson (ed.), Children’s language (Vol. 2), 127–214. New York: Gardner Press.
- Marcus, Gary F., S. Vijayan, S. Bandi Rao & P. M. Vishton. 1999. Rule learning by seven-month-old infants. Science 283(5398). 77–80.
- Pérez-Leroux, Ana, Tyler Peterson, Anny Patricia Castilla-Earls, Susana Béjar, Diane Massam & Yves Roberge. 2018. The acquisition of recursive modification in NPs. Language 94(2). 332–359.
- Pérez-Leroux, Ana, Yves Roberge, Alex Lowles & Petra Schulz. 2022. Structural diversity does not affect the acquisition of recursion: The case of possession in German. Language Acquisition 29(1). 54–78.
- Pinker, Steven. 1994. The language instinct. New York: William Morrow and Company.
- Reeder, Patricia A., Elissa L Newport & Richard N. Aslin. 2013. From shared contexts to syntactic categories: The role of distributional information in learning linguistic form-classes. Cognitive Psychology 66(1). 30–54.
- Roeper, Tom. 2011. The acquisition of recursion: How formalism articulates the child’s path. Biolinguistics 5(1–2). 57–86.
- Roeper, Tom, & William Snyder. 2005. Language learnability and the forms of recursion. In Anne M. DiScullo (ed.), UG and external systems: Language, brain and computation, 155-169. Amsterdam: John Benjamins.
- Rosenbach, Anette. 2014. English genitive variation – The state of the art. English Language and Linguistics 18. 215–262.
- Roth, Froma P. 1984. Accelerating language learning in young children. Child Language 11. 89–107.
- Ruskin, David. 2014. Cognitive influences on the evolution of new languages. Rochester, NY: University of Rochester dissertation.
- Schuler, Kathryn D., Patricia A. Reeder, Elissa L. Newport & Richard N. Aslin. 2017. The effect of Zipfian frequency variations on category formation in adult artificial language learning. Language Learning and Development 13. 357–374.
- Takahashi, Eri & Jeffrey Lidz. 2008. Beyond statistical learning in syntax. In A. Gavarró & M. J. Freitas (eds.), Proceedings of GALA 2007: Language Acquisition and Development, 444–454. Cambridge, UK: Cambridge Sch.
- Teinonen, Tuomas, Vineta Fellman, Risto Naatanen, Paavo Alku & Minna Huotilainen. 2009. Statistical language learning in neonates revealed by event-related brain potentials. BMC Neuroscience 10. 21.
- Thompson, Susan P. & Elissa L. Newport. 2007. Statistical learning of syntax: The role of transitional probability. Language Learning and Development 3(1). 1–42.
- Yang, Charles. 2016. The price of linguistic productivity. Cambridge, MA: MIT Press.