Abstract
We performed a secondary analysis of the Moving to Opportunity (MTO) social experiment to investigate the impact of different types of housing assistance and neighborhood environments on long-term patterns of healthcare use for specific conditions and across different types of healthcare services. MTO participants, who were randomized at baseline, were linked to up to 21 years’ worth of all-payer hospital discharge and Medicaid data. Among the 9,170 children at the time of randomization, those who received a voucher had subsequent hospital admissions rates that were 36% lower for asthma and 30% lower for mental health disorders compared with the control group; rates of psychiatric services, outpatient hospital services, clinic services, and durable medical equipment were also lower among the voucher groups. Findings for adults were not statistically significant. The results suggest that housing policies that reduce neighborhood poverty exposure as a child are associated with lower subsequent healthcare use for specific clinical conditions and types of services.
The connection between housing and quality of life has long been recognized in the United States, including early attempts in housing policy to deconcentrate crowding and improve the quality of tenements at the start of the 20th century (Schwartz, Citation2021). More recently, there has been an increasing emphasis on leveraging housing subsidies to promote access to lower poverty neighborhoods. Studies have shown long-term economic benefits of enabling low-income children to move to lower poverty neighborhoods (Chetty, Hendren, & Katz, Citation2016), and existing programs demonstrate the feasibility of assisting families to overcome barriers to renting homes in lower poverty neighborhoods (Bergman et al., Citation2020; Weismann, Rolfe, Kye, & Knudsen, Citation2020). For example, the 2019 and 2020 budgets from Congress allocated a total $50 million to implement approaches to helping families with housing assistance move to “opportunity” neighborhoods (HUD.gov, Citationn.d.), and President Biden’s fiscal year 2022 discretionary request for the U.S. Department of Housing and Urban Development (HUD) included funds designated to support housing mobility (Executive Office of The President Office of Management and Budget, Citation2021).
Concurrently, those in the health policy world have seen increased attention to social factors, including housing and neighborhood environments, as a root cause of health and health disparities. Drivers include changes to provider payment models, a widening focus on different types of community benefit spending by nonprofit hospitals, and the expanding evidence on the link between social factors and health (American Public Health Association, Citation2014; Association of American Medical Colleges, Citation2016; Centers for Disease Control and Prevention, Citation2016; Daniel, Bornstein, & Kane, Citation2018; National Academies of Sciences, Engineering, and Medicine, Citation2017; Sims et al., Citation2020). Emerging healthcare initiatives include the Center for Medicare and Medicaid Services’ Accountable Health Communities Model, which screens individuals for housing-related needs and links them to community-based services (Alley, Asomugha, Conway, & Sanghavi, Citation2016; RTI International, Citation2020), Medicaid reforms to allow investments in housing-related support services (Medicaid and CHIP Payment and Access Commission, Citation2018), and health system direct investments in social determinants including affordable housing and neighborhood revitalization (Horwitz, Chang, Arcilla, & Knickman, Citation2020).
As programs are increasingly designed to help low-income families move to lower poverty neighborhoods and as interest in the social determinants of health and healthcare grows, two key challenges remain in delineating the impact of housing and its surrounding neighborhood environment on health and healthcare use. First, family decisions about where to live are not random. These decisions are often influenced by factors including the availability of affordable housing and discrimination in the housing market (DeLuca, Garboden, & Rosenblatt, Citation2013; DeLuca, Wood, & Rosenblatt, Citation2019), and may be affected by one’s underlying health status and expected healthcare use. Thus, observational studies that examine variation in health and healthcare across neighborhoods and by housing type are prone to confounding, making it difficult to disentangle the contribution of these factors. Consequently, there is a need for experimental data that can provide causal evidence for these potential interventions’ effects on healthcare use. The second key challenge is that studies on housing, neighborhood environments, and health often focus on short-term outcomes (Clampet-Lundquist, Citation2004; Fauth, Leventhal, & Brooks-Gunn, Citation2004; Rosenbaum & Harris, Citation2001), leaving the long-term impacts of investments in housing and housing-related services unknown. It is possible that the potential benefits of housing and neighborhood interventions may not materialize immediately and instead develop over time.
The goal of this article is to delve further into the relationship between housing, neighborhood environments, and healthcare by leveraging healthcare claims and data from the Moving to Opportunity (MTO) experiment. Building on the study team’s prior findings, which observed reductions in overall rates of hospitalization among children whose family received a housing voucher without differences in emergency department (ED) visits (Pollack et al., Citation2019a; Pollack, Du, Blackford, & Herring, Citation2019b), this study explores whether access to lower poverty neighborhoods was associated with long-term differences in healthcare use for specific clinical conditions (e.g., asthma, mental health, diabetes), and across broad categories of healthcare use.
Background on Moving to Opportunity
MTO offers a critical chance to address challenges in studying the link between housing, neighborhood environments, and healthcare use (Sanbonmatsu, Ludwig, & Katz et al., Citation2011). MTO had a rigorous experimental design and because MTO was conducted in the 1990s, enough time has passed to allow researchers to examine its long-term effects.
MTO randomized families with children living in public housing located in high-poverty neighborhoods into (a) a group receiving a voucher that could only be used in a low-poverty neighborhood, (b) a group receiving a traditional housing voucher with no restrictions, or (c) a control group. Families who received either of the two types of housing vouchers reported improvements in housing quality, such as increasing the proportion of households who rated their housing conditions as good or excellent and reducing specific housing problems (e.g., vermin and peeling paint; Sanbonmatsu et al., Citation2011). Exposure to neighborhood poverty was also reduced among families in the two voucher groups compared with the control group (with similar average amounts for the two voucher groups). However, these differences were attenuated by several factors, including suboptimal lease-up rates among the voucher groups, many families with vouchers moving back to higher poverty neighborhoods, and a reduction of neighborhood poverty in the control group because of HOPE VI and other public housing assistance (Briggs, Popkin, & Goering, Citation2010; DeLuca, Clampet-Lundquist, & Edin, Citation2016).
Nonetheless, prior analyses of MTO found significant differences in health and healthcare use across the study groups. Analyses of the follow-up survey found that adults who received a housing voucher experienced reductions in overweight/obesity status and diabetes (Ludwig et al., Citation2011) and changes in maternal mental health (Orr et al., Citation2003), and children reported differences in mental health that varied by gender (Clampet-Lundquist, Kling, Edin, & Duncan, Citation2011; Kessler et al., Citation2014).
Previous work linking MTO participants to administrative health data allows for more objective measures of healthcare use and has found that children living in households that received a housing voucher—either the low-poverty or the traditional voucher—experienced lower rates of all-cause hospitalizations and had lower hospital spending during long-term follow-up compared with the control group (Pollack et al., Citation2019a). However, additional analyses found no significant differences in ED use among children between study groups and no significant differences among adults in the rates of either hospitalizations or ED visits between study groups (Pollack et al., Citation2019b).
Our Contribution
This project extends our prior analyses of MTO participants linked to their healthcare claims in two main ways. The first is that we examine whether the receipt of housing assistance to enable access to lower poverty neighborhoods was associated with long-term differences in hospitalization and ED visits for specific clinical conditions. Prior research has identified several types of health conditions that may be plausibly impacted by the housing and neighborhood environment—including asthma, injury, mental health, obesity, diabetes, and hypertension (DiGuiseppi, Jacobs, Phelan, Mickalide, & Ormandy, Citation2010; Edwards, Chauvin, & Blanchet, Citation2019; Ellen, Dragan, & Glied, Citation2020; Fisk, Eliseeva, & Mendell, Citation2010; Institute of Medicine (U.S.) Committee on Damp Indoor Spaces and Health, Citation2004; Kirby, Citation2017; Ludwig et al., Citation2012; MacMillan, 2018; Matsui et al., Citation2017; Sheehan et al., Citation2010; WHO Housing and Health Guidelines, Citation2018). Examining these specific conditions allows us to explore heterogeneity within the previously reported main effects (overall hospitalizations and ED visits). Additional evidence regarding the impact of housing quality on specific health conditions may subsequently enable policymakers to direct resources most appropriately.
The second goal of the analyses presented here is to examine whether housing assistance enabling access to low-poverty neighborhoods affects broad categories of healthcare use not limited to care received within hospital settings. Prior research has tended to focus on hospitalizations and ED visits, which represent only about one third of total healthcare spending (Bui et al., Citation2017; Lassman, Hartman, Washington, Andrews, & Catlin, Citation2015), with the majority of spending going to various types of outpatient services. Many studies group healthcare use—inclusive of inpatient, ED, and outpatient use—into types representing broad classifications as a way to document the distribution of care across populations, trends over time, and changes as a result of reforms (e.g., Berenson–Eggers Type of Service [BETOS] Codes); however, these categories have not, to our knowledge, been examined with respect to housing and neighborhood environments over a long-term follow-up period (CMS.gov, Citationn.d.).
Housing- and Neighborhood-Sensitive Conditions
Solely examining whether individuals are hospitalized or visit the ED may belie important variation in the underlying clinical conditions for which adults and children receive medical care. Prior research has found that that healthcare for specific clinical conditions may be strongly associated with the physical conditions in the home environment. For example, integrated pest management may reduce allergen exposures (Matsui et al., Citation2017; Sheehan et al., Citation2010), which, in turn, may decrease asthma symptoms (Kirby, Citation2017); mold remediation may reduce respiratory symptoms and infections (Fisk et al., Citation2010); structural improvements to buildings may reduce risk of injury (DiGuiseppi et al., Citation2010; Edwards et al., Citation2019); heating and insulation may affect mental health (Kessler et al., Citation2014; Ludwig et al., Citation2012; WHO Housing and Health Guidelines, Citation2018); and reductions in home temperatures and dampness may lower one’s blood pressure (Institute of Medicine (US) Committee on Damp Indoor Spaces and Health, Citation2004). In addition to changes in the physical home environment, several other aspects of the home and neighborhood environment have been shown to impact specific clinical conditions. For example, stress is related to home and neighborhood environments, through exposure to violence, neighborhood disorder, and other mechanisms (Booth, Ayers, & Marsiglia, Citation2012; Ross & Mirowsky, Citation2009). The home and neighborhood environment has been shown to be associated with asthma symptoms and may be a potential mediator between asthma triggers and exacerbations (Landeo-Gutierrez, Forno, Miller, & Celedón, Citation2020; Tobin et al., Citation2016; Wright, Citation2006). The home and neighborhood environments have also been linked with physical activity and diet (MacMillan et al., Citation2018) and, as noted above, receiving the MTO voucher has been shown to affect diabetes and obesity (Ludwig et al., Citation2011).
Based on the existing evidence, Ellen and colleagues identified a set of conditions that they termed “housing-sensitive” to study the impact of renovating public housing on children’s health (Ellen et al., Citation2020, p. 228). The authors found that renovations of public housing through a public–private partnership resembling the Rental Assistance Demonstration program were significantly associated with Medicaid claims for an index measure of these clinical conditions.
Our study similarly focuses on categories of housing- and neighborhood-sensitive conditions, to delve deeper into the associations between MTO study group assignment and hospitalization and ED visits. Based on MTO’s prior findings, which show changes in mental health and in diabetes and obesity, and with observed changes in housing quality and neighborhood conditions, we hypothesize that the associations between MTO study group assignment and hospitalizations will be concentrated among these housing- and neighborhood sensitive conditions.
Categories of Healthcare Use
In addition to studying healthcare use for particular clinical conditions, we examined broad categories of healthcare use beyond hospitalization and ED visits. Over the past four decades, the delivery of care has partially shifted from inpatient to outpatient utilization (National Center for Health Statistics, Citation2017). Differences in the use of these services vary across settings, with a rising number of ED visits for mental health diagnoses and substance use disorders. These changes are closely tied to a person’s physical and/or socioeconomic environment, which affect utilization via the need for care, the propensity to use services, and barriers to accessing these services (Chen, Vargas-Bustamante, Mortensen, & Ortega, Citation2016; Levesque, Harris, & Russell, Citation2013; National Academies of Sciences, Engineering, and Medicine, Citation2018; National Center for Health Statistics (U.S.), Citation2015, Citation2017).
It is largely unknown to what extent housing and neighborhood conditions impact these broad categories of healthcare use, such as psychiatric services, lab and X-ray services, dental services, clinic-based services, transportation services, targeted case management, and durable medical equipment. For instance, with documented effects of housing and neighborhood conditions on mental health (Kessler et al., Citation2014), it is possible that psychiatric services (inclusive of hospitalizations, outpatient visits, and day programs) may have decreased as families in MTO moved to lower poverty neighborhoods. Another example is that transportation barriers may be greater for families living in low-poverty neighborhoods (Bullard, Citation2013), where there may be less access to public transportation (Caldwell, Ford, Wallace, Wang, & Takahashi, Citation2016; Hensley et al., Citation2018; Syed, Gerber, & Sharp, Citation2013; Yang, Zarr, Kass-Hout, Kourosh, & Kelly, Citation2006), thereby leading to increased reliance on medical transport.
Methods
MTO Data
Developed by HUD, MTO enrolled families living in selected public housing in high-poverty neighborhoods within Baltimore, Maryland; Boston, Massachussets; Chicago, Illinois; Los Angeles, California; and New York, New York. From 1994 to 1998, participants were randomized to one of three study groups: (a) an experimental housing voucher which could only be used in a low-poverty neighborhood (defined as <10% of families in poverty), (b) a traditional Section 8 housing voucher without restrictions on neighborhood poverty, and (c) a control group. After 1 year, participants who received the experimental voucher could lease a home without restrictions on neighborhood poverty. In each group, housing assistance limited spending on rent and utilities to approximately 30% of a household’s income.
Study Sample
A total of 15,892 MTO participants (4,602 adults and 11,290 children) were matched to various administrative health data (described below) based on Social Security numbers, date of birth, gender, and study site. Individuals without known Social Security numbers (N = 1,984) or who died prior to the years of available administrative data (N = 16) were excluded.
For all analyses, participants were grouped as adults or children at the time of randomization, recognizing that the child sample became young adults during the study’s follow-up period. Based on prior MTO findings, the child sample was also stratified into two subsamples for secondary analyses: younger versus older children (<13 years old at the time of randomization vs. 13+) (Chetty et al., Citation2016) and boys versus girls (Clampet-Lundquist et al., Citation2011; Kessler et al., Citation2014). The Johns Hopkins School of Medicine Institutional Review Board approved the current analyses.
Administrative Health Data
Participants were linked to two sources of data: state all-payer hospital and ED data (which include potential utilization from all MTO participants, regardless of whether they had insurance or, if they did, their type of coverage) and Medicaid data for inpatient, ED, and outpatient service utilization and program enrollment (). The all-payer data were obtained directly from the relevant states: California (1995–2015 data for hospitalizations; 2005–2015 data for ED use), New York (1995–2015 data for hospitalizations; 2003–2015 data for ED use), and Massachusetts (2004–2014 data for hospitalization only). The Medicaid data were obtained from the Research Data Assistance Center (ResDAC) for California (1999–2014), Illinois (1999–2012), Maryland (1999–2012), and New York (1999–2013). Because MTO’s randomization occurred over a 5-year period, time is presented in our analyses as the number of years since a participant was randomized rather than according to the calendar year. For example, New York randomized families between 1994 and 1997, so its hospital discharge data start at the first year since randomization and extend through 18 to 21 years after randomization.
Consistent with our prior analyses (Pollack et al., Citation2019a, Citation2019b), the primary set of analyses examining hospitalizations and ED use for specific conditions leverages the all-payer data when available and is supplemented by the additional years of Medicaid data; combining these data sources is done to maximize their sample size (see the Supplementary material). When analyzing the types of service as the outcome, only the Medicaid data is able to be used.
Dependent Variables
Housing/Neighborhood-Sensitive Condition Outcomes
The first set of primary outcomes includes the number of hospitalizations per year for a given clinical condition and the number of ED visits per year for a given clinical condition. The analyses focused on the seven clinical conditions identified in prior research as sensitive to the housing or neighborhood environment: asthma, acute respiratory infections, injuries, mental health (including anxiety, adjustment, attention-deficit/hyperactivity, conduct, and mood disorders), diabetes, obesity, and hypertension. The analyses of hospital and ED use tied to diabetes, obesity, and hypertension are limited to adults, because of their low prevalence among children. The codes defining each condition were obtained from the Agency for Healthcare Research and Quality Clinical Classification Software (Agency for Health are Research and Quality, Citation2017 see the Supplementary material), and conditions were identified from the corresponding International Classification of Diseases (ICD)-9 and ICD-10 codes in the linked claims from ResDAC. In the primary analyses, hospitalizations and ED visits were classified based solely on the principal diagnostic code. The results from analyses are interpreted as the primary cause of given hospitalization or ED visit. In secondary analyses, all diagnostic codes were used. Results using all diagnostic codes give an interpretation of the underlying prevalence of an admission or ED visit with any particular The inpatient and ED visit samples differ because of variation in available years of data.
Type of Service Outcomes
The second set of primary outcomes comprised the annual utilization counts for the type of service, which are available in the Medicaid Analytic Extract (MAX) Other Therapy file from ResDAC; these included physician services, outpatient hospital utilization, lab/X-ray, clinic services, home health, and hospice (Type of Service Code, Citation2019). ResDAC’s Type of Service (TOS) code in the MAX data classifies lines into 34 national, uniform groups of service that describe where services were delivered (e.g., outpatient hospital), by whom (e.g., physician, other practitioners), or the services delivered (e.g., abortion services, transportation services, durable medical equipment); 22 of those 34 codes were present in the MTO sample used here. To increase the stability, consistency, and salience of the model’s estimates, these analyses were limited to the 11 categories that were present (a) at a rate greater than 5 per 100 person-years in each sample (so that both sets of count data are sufficiently prevalent to yield stable estimates) and (b) in at least 5% of adults and children, separately, over the entire follow-up period (as some of the TOS outcomes can be large counts concentrated in a relatively small number of people). These 11 categories were lab and X-ray, physicians, psychiatric services, outpatient hospital, dental, clinic, other services, transportation services, targeted case management, durable medical equipment and supplies, and other practitioners.
Main Independent Variable
Following an intention-to-treat framework, the primary independent variable in the regression analyses was an indicator for being assigned to one of the two study groups compared with the control group. The experimental and traditional voucher groups had similar average reductions in neighborhood poverty compared with the control group, and prior studies have generally not found significant differences in outcomes between the two voucher groups. Consistent with prior MTO analyses (Nguyen, Acevedo-Garcia, Schmidt, & Osypuk, Citation2017; Pollack et al., Citation2019a, Citation2019b; Rudolph et al., Citation2018; Rudolph et al., Citation2018), the two voucher groups were combined in these main analyses. Sensitivity analyses with separate indicators for the two voucher groups were also conducted.
Covariates
Covariates from the baseline MTO survey included: age; gender; race/ethnicity; head of household educational, employment, and marital status; and whether the head of household was under age 18 at the birth of his/her first child. Additional covariates included study site, year of randomization, calendar year (corresponding to the annual utilization count), and indicators for Medicaid status (managed care and partial-year enrollment).
Statistical Analyses
Using the person-year (PY) as the unit of observation, separate negative binomial regression models were used to estimate incidence rate ratios (IRRs) for the voucher group relative to the control group for each outcome. Because a participant could be enrolled in Medicaid for less than a full calendar year, an offset term indicating the total months of available data (set to 12 for the all-payer data) was included in each model. Observations were weighted by the National Bureau of Economic Research’s MTO survey sample weights to control for possible confounding induced by the varying randomization probabilities over the study’s accrual period (Sanbonmatsu et al., Citation2011). Cluster-robust standard errors were estimated using generalized estimating equations to account for repeated observations over time and clustering of children within families. Additionally, the consistency of the association between the housing voucher intervention and the outcomes was tested over different periods in follow-up, using interactions between the voucher groups and time, defined both linearly and as specific categories of time. Among children at the time of randomization, interaction terms were also used to test whether the associations differed by age at randomization or by gender. All outcome variables were top-coded at the 99th percentile of nonzero values, with separate state-specific thresholds for adults and for children to reduce the influence of outlier values.
Two additional sets of analyses were performed to help illustrate the magnitude of the findings. First, a set of estimates presents the change in healthcare use associated with a 10-percentage-point decrease in neighborhood poverty (Kling, Liebman, & Katz, Citation2007; Ludwig et al., Citation2011). To do so, the above empirical models for healthcare utilization replaced the voucher indicator with a measure of predicted neighborhood poverty as the key independent variable. This was done in two stages. Average neighborhood poverty experienced during the first 3 years after randomization was estimated in a first-stage model based on study group assignment, sites, and their interaction; this first-stage model generates an exogenous estimate of predicted neighborhood poverty. This measure of predicted neighborhood poverty exposure was then used as the key independent variable in a second-stage model for healthcare use. Second, a set of treatment-on-treated estimates was produced using a first-stage model to examine voucher take-up (with separate models for each voucher compared with the control), conditional on study group assignment, sites, and their interaction. Predicted voucher take-up was then used in a second-stage model examining healthcare use, conditional on voucher use. Multiple additional sensitivity analyses are described in the Supplementary material.
All p values are two-sided; we present both an unadjusted p value (where significance is defined as <.05) and a Benjamini–Hochberg adjusted p value in the tables and figures. The Benjamini–Hochberg procedure is used to adjust for multiple comparisons and sets a false discovery rate based on the total number of tests in each family of analyses (i.e., groups of comparisons in each table; Benjamini & Hochberg, Citation1995). The lowest false discovery rate thresholds at which the result would be considered statistically significant are specified in the tables/figures based on the total number of comparisons (Benjamini, Heller, & Yekutieli, Citation2009). Analytical files were constructed using SAS version 9.4 (SAS Institute, INC), and regression analyses were conducted using Stata version 15.1.
Results
Descriptive Statistics
Among those randomized as adults, 4,142 (90.0%) were linked to the all-payer and Medicaid hospital data, giving a median of 13.4 years of follow-up time (up to 21 years after randomization; N = 56,462 PY). Overall, the median age at randomization was 32 years, 98% were female, and 64% identify as black or African American (see ). Among those randomized as children, 9,169 (81.2%) were linked to the all-payer and Medicaid hospital data (N = 127,300 PY); the median age at randomization was 8, 50% were female, and 66% identify as black. There were no significant differences in sociodemographic characteristics between adults or children assigned to different study groups. The all-payer ED samples included 39,981 PY for adults and 95,469 PY for children. A total of 2,927 individuals who were adults at the time of randomization were linked with the Medicaid data used in the type of service analysis, providing a median of 8 years of follow-up time up to 19 years after randomization (N = 26,077 PY). Among the 6,986 children at the time of randomization who linked with Medicaid data, the median follow-up was 9 years (N = 68,807 PY).
Table 1. Baseline characteristics of the matched MTO study population.a
Take-up of the vouchers was 49% among households in the low-poverty voucher group and 62% in the traditional voucher group. On average, households experienced an adjusted rate of neighborhood poverty of 36% during the first 3 years after randomization in the low-poverty voucher group, 38% in the traditional voucher group, and 47% in the control group.
Intention-to-Treat Analyses for Housing/Neighborhood Sensitive Conditions
For those who were adults at randomization, the rates of hospitalizations or ED visits for the housing/neighborhood-sensitive conditions as defined by a primary diagnosis code were not significantly different between study groups (see ). For those who were children at randomization, the rates of hospital admissions based on the primary diagnostic code were lower in the voucher groups compared with the control groups for asthma (0.19 vs. 0.36 per 100 PY, IRR 0.64, 95% confidence interval [CI] [0.41, 1.00]) and for mental health disorders (0.33 vs. 0.46 per 100 PY, IRR 0.70, 95% CI [0.51, 0.95]); these nine CIs are based on the unadjusted p values. There were no differences in hospital admissions for the other clinical conditions and no differences in any of the ED visits.
Figure 1. Inpatient hospitalizations and ED visits based on primary diagnosis among voucher groups versus control group.
Note. Mean hospitalizations and emergency department (ED) visits represent counts per 100 person-years and were estimated from intercept-only negative binomial regression models, with an offset term for the total months of available data for a person-year and survey sample weights to account for varying sample probabilities over the accrual period. Incidence rate ratios are expressed as the voucher groups relative to the control group and were derived from negative binomial regression models for the count of hospitalizations or ED visits with the person-year as the unit of observation and adjustments made for the set of covariates described in the text. The models included survey sample weights to account for varying sampling probabilities over the accrual period and accounted for the family unit by clustering all standard errors by family. The size of each square point is proportional to the number of person-years of data available. Diabetes, obesity, and hypertension are not included for children because of their low prevalence.
*Adjusted p values represent the lowest false discovery rate threshold at which the test could be considered significant, using the Benjamini–Hochberg procedure to keep the false discovery rate to less than 1 out of 8, corresponding to the number of comparisons per group of analyses for adults, and 1 out of 5, corresponding to the number of comparisons per group of analyses for children; an adjusted p value is statistically significant if < .125 for adults and if < .20 for children
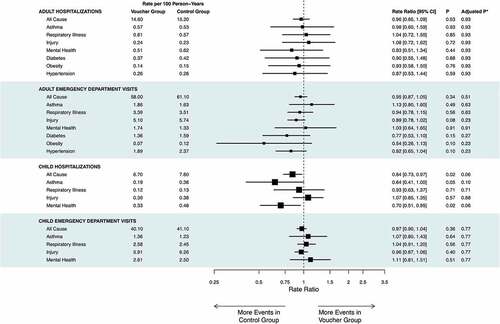
Figure 2. Type of service utilization among voucher groups versus control groups.
Note. Mean types of service utilization represent counts per 100 person-years and were estimated from intercept-only negative binomial regression models with an offset term for the total months of available data for a person-year and survey sample weights to account for varying sample probabilities over the accrual period. Incidence rate ratios are expressed as the voucher groups relative to the control group and were derived from negative binomial regression models, with an offset term indicating the total months of available data for the person and adjustments made for the set of covariates described in the text. The models included survey sample weights to account for varying sampling probabilities over the accrual period and accounted for the family unit by clustering all standard errors by family. The size of each square point is proportional to the number of person-years of data available.
*Adjusted p values represent the lowest false discovery rate threshold at which the test could be considered significant, using the Benjamini–Hochberg procedure to control the false discovery rate to less than 1 of 11, corresponding to the number of comparisons per group of analyses; an adjusted p value is statistically significant if <.09
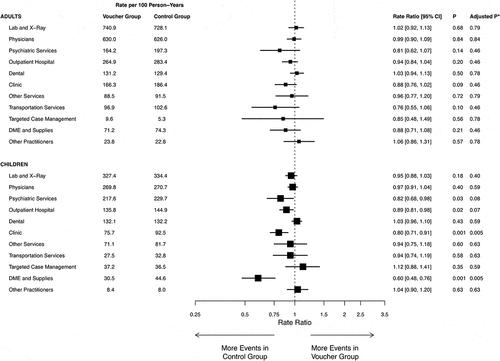
For children at the time of randomization, the interaction analysis for outcomes based on the primary diagnostic code indicated that the significant association between study group and hospitalizations did not vary over time (see the Supplementary material). Further, the interaction analysis for younger versus older children at the time of randomization indicated that the lower rate of asthma admissions compared with the control group was concentrated among the younger children (younger children: IRR 0.58; 95% CI [0.36, 0.93]; older children: IRR 1.80, 95% CI [0.71, 4.59]). There were no differences in the associations with outcomes observed by child gender.
The next set of analyses uses all available diagnostic codes associated with a hospitalization or ED visit to classify the clinical conditions, as opposed to the analyses that rely solely on the primary code as described in the preceding paragraphs. In these analyses based on all available diagnostic codes, there were still no significant associations between study group and hospitalizations among adults (see ). For ED visits, adults in the voucher groups had significantly lower rates for diabetes (IRR 0.60, 95% CI [0.43, 0.84]) and obesity (IRR 0.57, 95% CI [0.37, 0.87]) compared with the control group. Adults in the voucher groups had significantly lower rates of ED visits with a code of injury (IRR 0.86, 95% CI [0.76, 0.98]). For children at the time of randomization, there were again lower rates of hospitalizations with a diagnostic code for asthma and for mental health in the voucher compared with control groups; there were no differences with respect to ED visits.
Table 2. Housing/neighborhood-sensitive hospitalizations and emergency department (ED) visits based on all diagnostic codes among voucher groups vs. control groupa: adults and children
Intention-to-Treat Analyses for Types of Services
For the analyses of the types of service categories, there were no significant differences in the rates of any of the 11 categories between those in the voucher versus control groups for adults (see). Among children at the time of randomization, there were significantly lower rates of service use in the voucher versus control groups for 4 of the 11 categories: psychiatric services (217.6 vs. 229.7 per 100 PY, IRR 0.82, 95% CI [0.68, 0.98]), outpatient hospital services (135.8 vs. 144.9 per 100 PY, IRR 0.89, 95% CI [0.81, 0.96]), clinic services (75.7 vs. 92.5 per 100 PY, IRR 0.80, 95% Cl [0.71, 0.91]), and durable medical equipment and supplies (30.5 vs. 44.6 per 100 PY, IRR 0.60, 95% CI [0.48, 0.76]). The associations between study group and these outcomes did not vary over time, nor did they vary for the child-specific subgroups of younger versus older children or boys versus girls (see the Supplementary material).
Poverty-Based Estimates and Treatment-on-Treated Estimates
The additional analyses presenting the results from a two-stage model with predicted neighborhood poverty were generally consistent with the results of the intention-to-treat analyses for hospital and ED use (see ). A decrease in neighborhood poverty was not associated with subsequent hospitalizations or ED visits for a primary diagnosis of specific clinical conditions among adults. For children at the time of randomization, a 10-percentage-point reduction in neighborhood poverty was associated with 25% lower rates of mental health hospitalizations (IRR 0.75, 95% CI [0.56, 0.99]).
Table 3. Inpatient hospitalizations and emergency department (ED) visits based on primary diagnosis: magnitudes are shown as associations with a 10-percentage-point decrease in neighborhood poverty
The poverty-related estimates by TOS category were also generally consistent with the results of the intention-to-treat analyses (see ). For adults at the time of randomization, a 10-percentage-point decrease in neighborhood poverty was associated with lower use of transportation services (IRR 0.76, 95% CI [0.60, 0.97]). For children at the time of randomization, a 10-percentage-point decrease in neighborhood poverty was associated with a decrease in clinic services (IRR 0.86, 95% CI [0.79, 0.94]) and in durable medical equipment and supplies (IRR 0.71, 95% CI [0.59, 0.84]). The treatment-on-treated analyses accounting for incomplete voucher take-up are also generally consistent with the intention-to-treat models (see the Supplementary material).
Table 4. Magnitudes of the association with types of service as a 10-percentage-point decrease in neighborhood poverty+
Sensitivity Analyses
For the intention-to-treat analyses, the results were generally robust to separating out the two voucher groups and altering the top-coding of outcome measures. Some differences were observed when analyzing the all-payer hospital data and Medicaid hospital data separately. For children at the time of randomization, the results were generally consistent across the two data sets for mental health hospitalizations; asthma hospitalizations differed across study groups with the all-payer data subsample but not with the Medicaid data subsample. Lengthening the time period for neighborhood poverty exposure did not alter the findings from the two-stage analyses producing poverty-related magnitudes of the estimates.
Discussion
In exploring the relationship between receipt of a housing voucher and healthcare use among a low-income population enrolled in federal housing assistance, several key findings can be observed. Among adults, receipt of a housing voucher enabling a move to a lower-poverty neighborhood was not associated with inpatient hospital, ED, or outpatient utilization; however, when expanding the definition of housing/neighborhood-sensitive conditions to include all diagnostic codes, lower rates of ED visits for diabetes and obesity among adults who received a voucher were observed. Children whose families received a voucher experienced lower rates of asthma and mental health hospitalizations and overall lower rates of psychiatric, outpatient hospital, clinical, and durable medical equipment/supplies service use versus those in the control group. These results have important implications for considering the ways in which housing and neighborhood environments are linked to different components of healthcare use.
The generally null findings for adults coincide with prior work showing little difference in rates of hospitalizations or ED visits and no significant difference in economic outcomes. That said, the final MTO survey performed 10–15 years after randomization found reductions in diabetes (i.e., glycated hemoglobin) and severe obesity for adults in the low-poverty voucher group compared with the control (Ludwig et al., Citation2011). These prior results align somewhat with the current findings, although the significant reductions in ED visits for obesity and diabetes were only observed when including both the primary diagnosis and secondary diagnoses, suggesting the potential for these factors to complicate visits for a range of reasons. Because Orr and colleagues found a significant association between MTO study group and measures of adult mental health, the lack of association with mental health-related hospitalizations and ED visits also warrants some scrutiny (Orr et al., Citation2003). It is possible that observed differences based on MTO surveys were not severe enough to translate into differences in actual emergency or inpatient mental healthcare use (Orr et al., Citation2003). Multiple other barriers exist toward mental healthcare use including access and affordability (Beronio, Glied, & Frank, Citation2014), geographic accessibility (Cummings, Allen, Clennon, Ji, & Druss, Citation2017; Fortney, Rost, Zhang, & Warren, Citation1999), and stigma (Thornicroft, Citation2008).
The current findings for children extend prior work on the MTO cohort, which has found that children who received a voucher at a young age had higher earnings and increased likelihood of college attendance as young adults (Chetty et al., Citation2016), and had lower overall rates of hospitalizations (Pollack et al., Citation2019a). This work sheds light on other possible benefits of voucher receipt among children with respect to asthma and mental health and certain types of service, although the potential mechanisms underlying these new findings warrant further analysis.
Our finding that children whose families received a voucher had lower rates of asthma-related hospitalizations differs from the findings of the MTO final impact evaluation. That evaluation, conducted 10–15 years after randomization, did not find significant differences between study groups in self-reported asthma exacerbations or wheezing that caused individuals to limit activities or miss school/work in the preceding year (Sanbonmatsu et al., Citation2011). These survey measures may reflect a wide range of asthma symptomatology and may be prone to recall bias. In contrast, our claims-based measures used here reflect severe asthma requiring hospitalizations.
It is possible that the lower rate of asthma-related hospitalizations among children who received a voucher reflect differences in environmental exposures known to cause asthma exacerbations among inner-city and high-poverty populations, including mouse and cockroach allergens (Keet et al., Citation2015; Matsui et al., Citation2017). The MTO final impact evaluation showed that families who received a voucher reported fewer specific problems with their housing units, including “vermin” (Sanbonmatsu et al., Citation2011). Research has also identified parental stress as a potential mediator of the relationship between allergen exposure and asthma outcomes (Lee et al., Citation2017; Magnus et al., Citation2018; Matsui, Citation2014); stress may vary with respect to voucher receipt, moving, and neighborhood context. The stronger association with voucher receipt as a relatively younger child may underscore the notion of a life-course perspective with critical windows for these interventions to reduce asthma morbidity (Pearlin, Schieman, Fazio, & Meersman, Citation2005).
Regarding the lower rate of mental health-related hospitalizations and psychiatric types of service among children, MTO’s follow-up surveys found that boys whose families received vouchers tended to have higher rates of depression, posttraumatic stress disorder, and conduct disorder as adolescents, whereas girls tended to have lower rates of conduct disorder compared with the control group (Kessler et al., Citation2014). In contrast, we did not observe significant variation by gender, which may be due to healthcare use (as presented here) potentially reflecting more severe manifestation of mental health disorders, differences in evaluation and referral patterns overall and by gender (Green, Clopton, & Pope, Citation1996), the longer duration of follow-up in the current study, or other reasons. The measure of psychiatric types of services incorporates a range of services related to mental health and/or substance use, including counseling, residential care, and psychological testing. More generally, housing and neighborhood environments have been linked with child mental health through a range of mechanisms, including reduced exposure to violence and policing (Geller, Fagan, Tyler, & Link, Citation2014; Geller, Fagan, & Tyler, Citation2017; Geller & Fagan, Citation2019; Geller, Garfinkel, Cooper, & Mincy, Citation2009), peer group effects, and a more robust support system and social network (Dahal, Swahn, & Hayat, Citation2018), within both neighborhoods and higher resource schools.
The findings for lower rates of durable medical equipment use among children are challenging to interpret. Five of the top 10 procedure codes in this category refer to vision services (including frames and lenses), whereas three are for diabetic supplies (including syringes and lancets). The use of vision screenings, especially among children, is linked to improved health outcomes through early detection of amblyopia and its risk factors (Hartmann, Block, & Wallace, Citation2015; U.S. Preventive Services Task Force, Citation2011). Although prior studies have found that children in low-income neighborhoods are less likely to receive vision screening, this could be, at least partially, offset by programs in schools and local health departments (e.g., Vision for Baltimore, no-cost eye exams and glasses in New York and California), which may prompt higher use of durable medical equipment/supplies among children remaining in high-poverty neighborhoods. Utilization of these school-based programs may not appear in the Medicaid claims data.
The rates of outpatient hospital services and clinic visits were also significantly lower among children whose families received a voucher. These categories are broad, potentially reflecting the ability of the housing and neighborhood environment to impact outpatient care in addition to hospital-based care, as observed in recent work. Additional work would be required to delve into the specific types of providers and visit conditions driving these relationships.
Limitations
Our analyses have several limitations that may affect the interpretation of our findings. First, there were limitations of MTO as a social experiment. Only 23% of eligible families opted to participate in MTO, thereby limiting its generalizability (Fauth et al., Citation2004). Additionally, a relatively low proportion of families who received vouchers successfully used them to move, many who leased homes in low-poverty neighborhoods did not remain in these neighborhoods over time, and a large proportion of families in the control group also lived in lower-poverty neighborhoods over time, factors that together diminish the ability of researchers to observe meaningful differences between study groups. Contemporary mobility programs are more successful in helping families move to and remain in low-poverty neighborhoods (Bergman et al., Citation2019; Juracek et al., Citation2018). In addition, MTO was not initially conceptualized as a health experiment and, as such, baseline health data were not collected. Second, there are limitations inherent in using these all-payer and Medicaid data, although the quality of the data (e.g., incomplete data linkage to health claims from the original MTO participants) was unlikely to be differential across study groups. That said, observing Medicaid utilization is conditional on being enrolled in Medicaid, and MTO could potentially impact either eligibility or enrollment; however, our analyses indicated that differential enrollment by study group was not significant. Third, the condition-related outcome measures analyzed here were designed to reflect areas with evidence suggesting a prior link with the housing and neighborhood context, but the grouping may be imprecise, and the underlying diagnostic coding may be subject to misclassification—although, again, any errors are unlikely to be differential across study groups. Fourth, the ways that states code the TOS data may vary across states, although this would not vary by study group within a state. Fifth, observed changes in utilization may be either due to differences in underlying health status (e.g., differences in the prevalence and severity of certain conditions related to differences in housing and neighborhood factor) or due to differences in access to care conditional on one’s underlying health status (for example, due to geographic proximity, affordability, and acceptability). Although the analyses here are unable to disentangle the two effects, the findings nonetheless suggest important avenues for future research and intervention, especially with regards to asthma and mental health hospitalizations in children. Finally, we present many outcomes that were not prespecified either at the time of MTO enrollment or upon our subsequent data linkage to health claims; thus, together with the issue of testing multiple outcomes, these results should be considered exploratory.
Conclusion
Overall, approximately 10 million individuals, including 4 million children, live in households receiving federal housing assistance; they live in neighborhoods with a mean poverty rate of 27% (U.S. Department of Housing and Urban Development Office of Policy Development and Research, Citation2018). Initiatives that help families move to opportunity neighborhoods through, for example, financial counseling, security deposit assistance, and landlord outreach are increasingly being considered in light of the expected long-term economic benefits for children (Bergman et al., Citation2019; Executive Office of The President Office of Management and Budget, Citation2021; HUD.gov, Citationn.d.). Although the per-family costs of these mobility initiatives are quite small in relation to per-family costs of subsidized housing generally, it is important to consider the full range of potential benefits to help offset these costs of mobility. Our findings provide information on these long-term cost implications and indicate that there would be some modest decreases in healthcare use for specific clinical conditions and different types of services.
Increasingly, Medicaid programs are considering housing-related services as part of 1,115 waivers (Hinton, Artiga, Musumeci, & Rudowitz, Citation2019; Maryland Department of Health, Citationn.d.). Existing waivers covering services that focus on housing have been designed to support residential stability and homelessness reduction, for example through tenancy support services. They have not, to our knowledge, considered the neighborhood location of housing assistance. Given the potential for lower inpatient and certain types of outpatient service use among Medicaid beneficiaries in the long term, those waivers might consider the potential for future programs to incorporate residential services and counseling that help direct families to lower poverty neighborhoods. At the same time, as HUD evaluates mobility programs enabling voucher holders to move to lower poverty neighborhoods, policymakers should consider the potential spillover effects on healthcare use when determining the programs’ value.
Appendix: Using the Moving To Opportunity Experiment to Investigate the Long-Term Impact of Neighborhoods on Healthcare Use by Specific Clinical Conditions and Type of Service
Download MS Word (300 KB)Acknowledgments
The authors thank Adam Tapply, JD, MPH and Sylvia Hobbs, MPH of Massachusetts’s Center for Health information and Analysis, John Piddock and Colleen Fiato of New York’s Statewide Planning and Research Cooperative System, Jonathan Teague, MCRP and Karen Henderson, BA of California’s Office of Statewide Health Planning and Development, the Research Data Assistance Center, Lisa Sanbonmatsu, PhD of the National Bureau of Economic Research, and Mark Shroder, PhD and Lydia Taghavi, BS of the Department of Housing and Urban Development for assistance with data preparation and linkage; Shawn Du, PhD of the Analysis Group and Eric Roberts, PhD of the University of Pittsburgh for assistance with data preparation, linkage, and analysis; Erika Poethig, MA of the Urban Institute, Barbara Samuels, JD of the ACLU of Maryland, Josh Sharfstein, MD of Johns Hopkins, and Phil Tegeler, JD of the Poverty and Race Action Council for serving on the project’s policy advisory committee; and Oktawia Wojcik, PhD of the Robert Wood Johnson Foundation for her helpful comments and advice.
Disclosure Statement
Craig Pollack reports stock ownership in Gilead Pharmaceuticals and was an unpaid member of the Enterprise Community Partners Health Advisory Committee. In September 2019 Johns Hopkins University entered into a contract with the Department of Housing and Urban Development (HUD) for Pollack to work part time on a temporary assignment, assisting the department on housing and health issues. The opinions expressed by the authors do not necessarily represent the policies of the Department of Housing and Urban Development or opinions of the other funders.
Supplemental data
Supplemental data for this article can be accessed here.
Additional information
Funding
Notes on contributors
Craig Evan Pollack
Craig Evan Pollack is the Katey Ayers Associate Professor of Health Policy and Management, Nursing, and Medicine at the Johns Hopkins Bloomberg School of Public Health and School of Nursing. He is a practicing primary care physician whose research investigates the impact of housing policies on health and health care among adults and children.
Debra G. Bozzi
Debra G. Bozzi is a senior researcher at the Health Care Cost Institute, focused on studying geographic variation in health care prices and utilization, provider behavior, and health disparities. She completed her PhD in Health Economics from the Johns Hopkins Bloomberg School of Public Health.
Amanda L. Blackford
Amanda L. Blackford is a principal biostatistician in the Division of Biostatistics and Bioinformatics in the Department of Oncology in the Johns Hopkins University School of Medicine. Her research focuses on cancer outcomes in clinical trials and through leveraging claims-based data sources.
Stefanie DeLuca
Stefanie DeLuca is the James Coleman Professor of Sociology and Social Policy at Johns Hopkins University. Her research uses mixed methods and interdisciplinary perspectives to inform housing policy, with a focus on understanding housing decisions and interventions to increase residential choice and neighborhood quality.
Rachel L. J. Thornton
Rachel L. J. Thornton is Associate Professor of Pediatrics and the inaugural Executive Director of Clinical Services for the Johns Hopkins Medicine Office of Population Health. Her research examines child and family health and seeks to inform policies and practices across healthcare and other sectors with a focus on equity related considerations.
Bradley Herring
Bradley Herring is the Forrest D. McKerley Professor of Health Economics at the University of New Hampshire’s Paul College of Business and Economics. Dr. Herring’s research primarily focuses on several economic and policy issues related to health insurance markets and healthcare reform.
References
- Agency for Health Care Research and Quality. (2017, March). Clinical classifications software (CCS) for ICD-9-CM. Retrieved from https://www.hcup-us.ahrq.gov/toolssoftware/ccs/ccs.jsp
- Alley, D. E., Asomugha, C. N., Conway, P. H., & Sanghavi, D. M. (2016). Accountable health communities--addressing social needs through Medicare and Medicaid. The New England Journal of Medicine, 374(1), 8–11.
- American Public Health Association. (2014, November 18). Support for social determinants of behavioral health and pathways for integrated and better public health. Retrieved from https://www.apha.org/policies-and-advocacy/publichealth-policy-statements/policydatabase/2015/01/28/14/58/support-for-social-determinants-ofbehavioral-health
- Association of American Medical Colleges. (2016). Achieving health equity: How academic medicine is addressing the social determinants of health. Retrieved from https://www.aamc.org/download/460392/data/sdoharticles.pdf
- Benjamini, Y., Heller, R., & Yekutieli, D. (2009). Selective inference in complex research. Philosophical Transactions A, 367(1906), 4255–4271.
- Benjamini, Y., & Hochberg, Y. (1995). Controlling the false discovery rate: A practical and powerful approach to multiple testing. Journal of the Royal Statistical Society. Series B (Methodological), 57(1), 289–300.
- Bergman, P., Chetty, R., DeLuca, S., Hendren, N., Katz, L., & Palmer, C. (2019). Creating moves to opportunity: Experimental evidence on barriers to neighborhood choice. Cambridge, MA: National Bureau of Economic Research. Retrieved from https://www.nber.org/papers/w26164
- Berkman, P., Chetty, R., DeLuca S., Hendren N., Katz, L. F., & Palmer, C. (2020). Creating moves to opportunity: Experimental evidence on barriers to neighborhood choice. National Bureau of Economic Research. https://www.nber.org/system/files/working_papers/w26164/w26164.pdf
- Beronio, K., Glied, S., & Frank, R. (2014). How the affordable care act and mental health parity and addiction equity act greatly expand coverage of behavioral health care. The Journal of Behavioral Health Services & Research, 41(4), 410–428.
- Booth, J., Ayers, S. L., & Marsiglia, F. F. (2012). Perceived neighborhood safety and psychological distress: Exploring protective factors. The Journal of Sociology & Social Welfare, 39(4), 137–156.
- Briggs, X., Popkin, S. J., & Goering, J. (2010). Moving to Opportunity: The story of an American experiment to fight ghetto poverty illustrated edition. New York: Oxford University Press.
- Bui, A. L., Dieleman, J. L., Hamavid, H., Birger, M., Chapin, A., Duber, H. C., … Murray, C. J. (2017). Spending on children’s personal health care in the United States, 1996–2013. JAMA Pediatrics, 171(2), 181–189.
- Bullard, R. D. (2013). Addressing urban transportation equity in the United States. Fordham Urban Law Journal, 31(5), 1183–1209.
- Caldwell, J. T., Ford, C. L., Wallace, S. P., Wang, M. C., & Takahashi, L. M. (2016). Intersection of living in a rural versus urban area and race/ethnicity in explaining access to health care in the United States. American Journal of Public Health, 106(8), 1463–1469.
- Centers for Disease Control and Prevention. (2016). Home improvement loans and grants. Retrieved from https://www.cdc.gov/policy/hst/hi5/homeimprovement/index.html
- Chen, J., Vargas-Bustamante, A., Mortensen, K., & Ortega, A. N. (2016). Racial and ethnic disparities in health care access and utilization under the Affordable Care Act. Medical Care, 54(2), 140–146.
- Chetty, R., Hendren, N., & Katz, L. F. (2016). The effects of exposure to better neighborhoods on children: New evidence from the Moving to Opportunity experiment. The American Economic Review, 106(4), 855–902.
- Clampet-Lundquist, S. (2004). Moving over or moving up? Short-term gains and losses for relocated HOPE VI families. Cityscape, 7(1), 57–80.
- Clampet-Lundquist, S., Kling, J. R., Edin, K., & Duncan, G. J. (2011). Moving teenagers out of high-risk neighborhoods: How girls fare better than boys. American Journal of Sociology, 116(4), 1154–1189.
- CMS.gov. (n.d.). Berenson-Eggers type of service (BETOS) codes. Retrieved from https://www.cms.gov/Research-Statistics-Data-and-Systems/Statistics-Trends-and-Reports/MedicareFeeforSvcPartsAB/downloads/betosdesccodes.pdf
- Cummings, J. R., Allen, L., Clennon, J., Ji, X., & Druss, B. G. (2017). Geographic access to specialty mental health care across high- and low-income U.S. communities. JAMA Psychiatry, 74(5), 476–484.
- Dahal, S., Swahn, M. H., & Hayat, M. J. (2018). Association between neighborhood conditions and mental disorders among children in the U.S.: Evidence from the national survey of children’s health 2011/12. Psychiatry Journal, 2018, 5914315.
- Daniel, H., Bornstein, S. S., & Kane, G. C.; Health and Public Policy Committee of the American College of Physicians. (2018). Addressing social determinants to improve patient care and promote health equity: An American College of Physicians position paper. Annals of Internal Medicine, 168(8), 577–578.
- DeLuca, S., Clampet-Lundquist, S., & Edin, K. (2016). Coming of age in the other America. New York: Russell Sage Foundation.
- DeLuca, S., Garboden, P. M. E., & Rosenblatt, P. (2013). Segregating shelter: How housing policies shape the residential locations of low-income minority families. The Annals of the American Academy of Political and Social Science, 647(1), 268–299.
- DeLuca, S., Wood, H., & Rosenblatt, P. (2019). Why poor families move (and where they go): Reactive mobility and residential decisions. City & Community, 18(2), 556–593.
- DiGuiseppi, C., Jacobs, D. E., Phelan, K. J., Mickalide, A. D., & Ormandy, D. (2010). Housing interventions and control of injury-related structural deficiencies: A review of the evidence. Journal of Public Health Management and Practice: JPHMP, 16(5 Suppl), S34–S43.
- Edwards, N., Chauvin, J., & Blanchet, R. (2019). Advocating for improvements to building codes for the population’s health. Canadian Journal of Public Health [Revue canadienne de sante publique], 110(4), 516–519.
- Ellen, I. G., Dragan, K. L., & Glied, S. (2020). Renovating subsidized housing: The impact on tenants’ health. Health Affairs, 39(2), 224–232.
- Executive Office of The President Office of Management and Budget. (2021). Summary of the president’s discretionary funding request. Retrieved from https://www.whitehouse.gov/wp-content/uploads/2021/04/FY2022-Discretionary-Request.pdf
- Fauth, R. C., Leventhal, T., & Brooks-Gunn, J. (2004). Short-term effects of moving from public housing in poor to middle-class neighborhoods on low-income, minority adults’ outcomes. Social Science & Medicine, 59(11), 2271–2284.
- Fisk, W. J., Eliseeva, E. A., & Mendell, M. J. (2010). Association of residential dampness and mold with respiratory tract infections and bronchitis: A meta-analysis. Environmental Health: A Global Access Science Source, 9(1), 72.
- Fortney, J., Rost, K., Zhang, M., & Warren, J. (1999). The impact of geographic accessibility on the intensity and quality of depression treatment. Medical Care, 37(9), 884–893.
- Geller, A., & Fagan, J. (2019). Police contact and the legal socialization of urban teens. Russell Sage Foundation Journal of the Social Sciences, 5(1), 26–49.
- Geller, A., Fagan, J., Tyler, T., & Link, B. G. (2014). Aggressive policing and the mental health of young urban men. American Journal of Public Health, 104(12), 2321–2327.
- Geller, A., Fagan, J., & Tyler, T. R. (2017). Police contact and mental health. Columbia law school scholarship archive. Retrieved from https://scholarship.law.columbia.edu/cgi/viewcontent.cgi?article=3079&context=faculty_scholarship
- Geller, A., Garfinkel, I., Cooper, C. E., & Mincy, R. B. (2009). Parental incarceration and child wellbeing: Implications for urban families. Social Science Quarterly, 90(5), 1186–1202.
- Green, M. T., Clopton, J. R., & Pope, A. W. (1996). Understanding gender differences in referral of children to mental health services. Journal of Emotional and Behavioral Disorders, 4(3), 182–190.
- Hartmann, E. E., Block, S. S., & Wallace, D. K. (2015). Vision and eye health in children 36 to <72 months. Optometry and Vision Science, 92(1), 24–30.
- Hensley, C., Heaton, P. C., Kahn, R. S., Luder, H. R., Frede, S. M., & Beck, A. F. (2018). Poverty, transportation access, and medication nonadherence. Pediatrics, 141(4), e20173402.
- Hinton, E., Artiga, S., Musumeci, M., & Rudowitz, R. (2019). A first look at North Carolina’s section 1115 medicaid waiver’s healthy opportunities pilots. KFF. Retrieved from https://www.kff.org/medicaid/issue-brief/a-first-look-at-north-carolinas-section-1115-medicaid-waivers-healthy-opportunities-pilots/
- Horwitz, L. I., Chang, C., Arcilla, H. N., & Knickman, J. R. (2020). Quantifying health systems’ investment in social determinants of health, by sector, 2017–19. Health Affairs (Project Hope), 39(2), 192–198.
- HUD.gov. (n.d.). Housing choice voucher (HCV) mobility demonstration. Retrieved from https://www.hud.gov/program_offices/public_indian_housing/programs/hcv/mobilitydemo
- Institute of Medicine (U.S.) Committee on Damp Indoor Spaces and Health. (2004). Damp indoor spaces and health. Washington, DC: National Academies Press (U.S.).
- Juracek, A., Bell, A., Rolfe, N., Tegeler, N., Kurniawan, H., & Herskind, M. (2018). Housing mobility programs in the U.S. PRRAC. Retrieved from https://prrac.org/pdf/mobilityprogramsus2018.pdf
- Keet, C. A., McCormack, M. C., Pollack, C. E., Peng, R. D., McGowan, E., & Matsui, E. C. (2015). Neighborhood poverty, urban residence, race/ ethnicity,and asthma: Rethinking the inner-city asthma epidemic. The Journal of Allergy and Clinical Immunology, 135(3), 655–662.
- Kessler, R. C., Duncan, G. J., Gennetian, L. A., Katz, L. F., Kling, J. R., Sampson, N. A., … Ludwig, J. (2014). Associations of housing mobility interventions for children in high-poverty neighborhoods with subsequent mental disorders during adolescence. JAMA, 311(9), 937–948.
- Kirby, T. (2017). Effect of pest management on asthma symptoms. Lancet Respiratory Medicine, 5(5), 380.
- Kling, J., Liebman, J., & Katz, L. (2007). Experimental analysis of neighborhood effects. Econometrica, 75(1), 83–119.
- Landeo-Gutierrez, J., Forno, E., Miller, G. E., & Celedón, J. C. (2020). Exposure to violence, psychosocial stress, and asthma. American Journal of Respiratory and Critical Care Medicine, 201(8), 917–922.
- Lassman, D., Hartman, M., Washington, B., Andrews, K., & Catlin, A. (2015). U.S. health spending trends by age and gender: Selected years 2002–10. Health Affairs, 33(5), 815–822.
- Lee, A. G., Chiu, Y. M., Rosa, M. J., Cohen, S., Coull, B. A., Wright, R. O., … Wright, R. J. (2017). Association of prenatal and early childhood stress with reduced lung function in 7-year-olds. Annals of Allergy, Asthma & Immunology: Official Publication of the American College of Allergy, Asthma, & Immunology, 119(2), 153–159.
- Levesque, J. F., Harris, M. F., & Russell, G. (2013). Patient-centred access to health care: Conceptualising access at the interface of health systems and populations. International Journal for Equity in Health, 12(1), 18.
- Ludwig, J., Duncan, G. J., Gennetian, L. A., Katz, L. F., Kessler, R. C., Kling, J. R., & Sanbonmatsu, L. (2012). Neighborhood effects on the long-term well-being of low-income adults. Science, 337(6101), 1505–1510.
- Ludwig, J., Sanbonmatsu, L., Gennetian, L., Adam, E., Duncan, G. J., Katz, L. F., & McDade, T. W. (2011). Neighborhoods, obesity, and diabetes—a randomized social experiment. New England Journal of Medicine, 365(16), 1509–1519.
- MacMillan, F., George, E. S., Feng, X., Merom, D., Bennie, A., Cook, A., … Astell-Burt, T. (2018). Do natural experiments of changes in neighborhood built environment impact physical activity and diet? A systematic review. International Journal of Environmental Research and Public Health, 15(2), 217.
- MACPAC.gov. (2018). Medicaid’s role in housing. Medicaid and CHIP payment and access commission. Retrieved from https://www.macpac.gov/wp-content/uploads/2018/10/Medicaid%E2%80%99s-Role-in-Housing.pdf
- Magnus, M. C., Wright, R. J., Røysamb, E., Parr, C. L., Karlstad, Ø., Page, C. M., … Nystad, W. (2018). Association of maternal psychosocial stress with increased risk of asthma development in offspring. American Journal of Epidemiology, 187(6), 1199–1209.
- Maryland Department of Health. (n.d.). Assistance in community integration services pilot. Retrieved from https://mmcp.health.maryland.gov/Pages/Assistance-in-Community-Integration-Services-Pilot.aspx
- Matsui, E. C. (2014). Environmental exposures and asthma morbidity in children living in urban neighborhoods. Allergy, 69(5), 553–558.
- Matsui, E. C., Perzanowski, M., Peng, R. D., Wise, R. A., Balcer-Whaley, S., Newman, M., … Phipatanakul, W. (2017). Effect of an integrated pest management intervention on asthma symptoms among mouse-sensitized children and adolescents with asthma: A randomized clinical trial. JAMA, 317(10), 1027–1036.
- National Academies of Sciences, Engineering, and Medicine, Health and Medicine Division, Board on Population Health and Public Health Practice, Committee on Community-Based Solutions to Promote Health Equity in the United States; A. Baciu, Y. Negussie, A. Geller, & J. N. Weinstein (Eds.). (2017). Communities in action: Pathways to health equity. Washington, DC: National Academies Press (U.S.).
- National Academies of Sciences, Engineering, and Medicine, Health and Medicine Division, Board on Health Care Services, & Committee on Health Care Utilization and Adults with Disabilities. (2018). Health-care utilization as a proxy in disability determination. Washington, DC: National Academies Press (U.S.).
- National Center for Health Statistics (U.S.). (2015). Health, United States, 2015: With special feature on racial and ethnic health disparities. Hyattsville, MD: National Center for Health Statistics (U.S.); 2016 May.
- National Center for Health Statistics (U.S.). (2017). Health, United States, 2016: With chartbook on long-term trends in health. Hyattsville, MD: National Center for Health Statistics (U.S.).
- Nguyen, Q. C., Acevedo-Garcia, D., Schmidt, N. M., & Osypuk, T. L. (2017). The effects of a housing mobility experiment on participants’ residential environments. Housing Policy Debate, 27(3), 419–448.
- Orr, L., Feins, J. D., Jacob, R., Beecroft, E., Sanbonmatsu, L., Katz, L., … Kling, J. R. (2003). Moving to Opportunity interim impacts evaluation. Washington, DC: U.S. Department of Housing and Urban Development, Office of Policy Development and Research.
- Pearlin, L. I., Schieman, S., Fazio, E. M., & Meersman, S. C. (2005). Stress, health, and the life course: Some conceptual perspectives. Journal of Health and Social Behavior, 46(2), 205–219.
- Pollack, C. E., Blackford, A. L., Du, S., DeLuca, S., Thornton, R. L. J., & Herring, B. (2019a). Association of receipt of a housing voucher with subsequent hospital utilization and spending. JAMA, 322(21), 2115–2124.
- Pollack, C. E., Du, S., Blackford, A. L., & Herring, B. (2019b). Experiment to decrease neighborhood poverty had limited effects on emergency department use. Health Affairs, 38(9), 1442–1450.
- Rosenbaum, E., & Harris, L. E. (2001). Low-income families in their new neighborhoods: The short-term effects of moving from Chicago’s public housing. Journal of Family Issues, 22(2), 183–210.
- Ross, C. E., & Mirowsky, J. (2009). Neighborhood disorder, subjective alienation, and distress. Journal of Health and Social Behavior, 50(1), 49–64.
- RTI International. (2020). Accountable health communities (AHC) model evaluation. Retrieved from https://innovation.cms.gov/data-and-reports/2020/ahc-first-eval-rpt
- Rudolph, K. E., Schmidt, N. M., Glymour, M. M., Crowder, R., Galin, J., Ahern, J., & Osypuk, T. L. (2018). Composition or context: Using transportability to understand drivers of site differences in a large-scale housing experiment. Epidemiology, 29(2), 199–206.
- Rudolph, K. E., Sofrygin, O., Schmidt, N. M., Crowder, R., Glymour, M. M., Ahern, J., & Osypuk, T. L. (2018). Mediation of neighborhood effects on adolescent substance use by the school and peer environments. Epidemiology, 29(4), 590–598.
- Sanbonmatsu, L., Ludwig, J., Katz, L. F., Gennetian, L. A., Duncan, G. J., Kessler, R. C., Adam, E., McDade, T. W., & Lindau, S. T. (2011). Moving to Opportunity for fair housing demonstration program—final impacts evaluation. Washington, DC: U.S. Department of Housing and Urban Development. Retrieved from https://www.huduser.gov/publications/pdf/MTOFHD_fullreport_v2.pdf.Published
- Schwartz, A. F. (2021). Housing policy in the United States. New York: Routledge.
- Sheehan, W. J., Rangsithienchai, P. A., Wood, R. A., Rivard, D., Chinratanapisit, S., Perzanowski, M. S., … Phipatanakul, W. (2010). Pest and allergen exposure and abatement in inner-city asthma: A work group report of the American Academy of Allergy, Asthma & Immunology Indoor Allergy/Air Pollution Committee. The Journal of Allergy and Clinical Immunology, 125(3), 575–581.
- Sims, M., Kershaw, K. N., Breathett, K., Jackson, E. A., Lewis, L. M., Mujahid, M. S., & Suglia, S. F.; American Heart Association Council on Epidemiology and Prevention and Council on Quality of Care and Outcomes Research. (2020). Importance of housing and cardiovascular health and well-being: A scientific statement from the American Heart Association. Circulation, Cardiovascular Quality and Outcomes, 13(8), e000089.
- Syed, S. T., Gerber, B. S., & Sharp, L. K. (2013). Traveling towards disease: Transportation barriers to health care access. Journal of Community Health, 38(5), 976–993.
- Thornicroft, G. (2008). Stigma and discrimination limit access to mental health care. Epidemiologia E Psichiatria Sociale, 17(1), 14–19.
- Tobin, E. T., Zilioli, S., Imami, L., Saleh, D. J., Kane, H. S., & Slatcher, R. B. (2016). Neighborhood stress, depressive symptoms, and asthma morbidity in youth. Journal of Pediatric Psychology, 41(9), 952–960.
- Type of Service Code. (2019). ResDAC. Retrieved from https://www.resdac.org/cms-data/variables/type-service-code
- U.S. Department of Housing and Urban Development Office of Policy Development and Research. (2018). Picture of subsidized households. Retrieved from https://www.huduser.gov/portal/datasets/assthsg.html
- U.S. Preventive Services Task Force. (2011). Vision screening for children 1 to 5 years of age: U.S. preventive services task force recommendation statement. Pediatrics, 127(2), 340–346.
- Weismann, G., Rolfe, N., Kye, P., & Knudsen, B.; Housing Mobility Programs in the U.S. (2020). PRRAC. Retrieved from https://prrac.org/pdf/housing-mobility-programs-in-the-us-2020.pdf
- WHO Housing and Health Guidelines. (2018). Low indoor temperatures and insulation. Geneva: World Health Organization. Retrieved from https://www.ncbi.nlm.nih.gov/books/NBK535294/
- Wright, R. J. (2006). Health effects of socially toxic neighborhoods: The violence and urban asthma paradigm. Clinics in Chest Medicine, 27(3), 413–v.
- Yang, S., Zarr, R. L., Kass-Hout, T. A., Kourosh, A., & Kelly, N. R. (2006). Transportation barriers to accessing health care for urban children. Journal of Health Care for the Poor and Underserved, 17(4), 928–943.