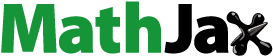
ABSTRACT
We introduce an improved Bonferroni method for testing two primary endpoints in clinical trial settings using a new data-adaptive critical value that explicitly incorporates the sample correlation coefficient. Our methodology is developed for the usual Student’s t-test statistics for testing the means under normal distributional setting with unknown population correlation and variances. Specifically, we construct a confidence interval for the unknown population correlation and show that the estimated type-1 error rate of the Bonferroni method with the population correlation being estimated by its lower confidence limit can be bounded from above less conservatively than using the traditional Bonferroni upper bound. We also compare the new procedure with other procedures commonly used for the multiple testing problem addressed in this paper.
1. Introduction
Pivotal clinical trials for new treatments that are designed to evaluate two primary efficacy endpoints face the so-called ‘multiplicity problem’, which, if not addressed, may cause inflation of type-1 error. Accordingly, regulatory agencies require that analysis plans contain a statistical methodology for type-1 error control. Moreover, since controlling type-1 error may also impact type-2 error (i.e., decrease power), regulators stress that one should examine the trade-off between the two types of error and carefully choose type-1 error controlling methodology. The multiplicity problem is further exacerbated by the inherent dependencies among various endpoints. While these dependencies can be qualitatively characterized in the sense that outcomes associated with the endpoints exhibit similar tendencies, albeit, with different magnitudes, there are situations where they can be quantitatively assessed from sample correlations among the examined variables.
Several statistical methodologies have been put forward to deal with the need to control type-1 error, with the aim of ultimately identifying at least one endpoint, and preferably both, for which the new treatment is better than the control. Among them, the most commonly used are the Bonferroni method for global testing and its step-down extension, Holm’s (Citation1979) method, for multiple testing. Because these methods utilize the Bonferroni inequality that relies only on the marginal p-values, they are dependency-free, and hence can be quite conservative when the p-values or the corresponding test statistics are highly dependent. Šidák (Citation1967) and Simes (Citation1986) have introduced improvements of the Bonferroni method for global testing. They control the type-1 error rate under independence and under a type of positive dependency that arises in some practical applications (Hochberg and Rom (Citation1995), Samuel-Cahn (Citation1996), Sarkar and Chang (Citation1997), and Sarkar (Citation1998)). Šidák’s (Citation1967) global test has been used by Holland and Copenhaver (Citation1987) to develop a step-down method, whereas Simes (Citation1986) has been used by Hochberg (Citation1988) to develop a step-up multiple testing method and by Hommel (Citation1988) to develop a closed testing method based on the ‘Closure Principle’ of Marcus et al. (Citation1976). Gou et al. (Citation2014) proposed a class of hybrid Hochberg-Hommel procedures which tend to be more powerful than either the Hochberg or Hommel procedure.
Šidák’s (Citation1967) and Simes (Citation1986) improved versions of the Bonferroni global test and their multiple testing extensions only qualitatively capture the underlying positive dependency, as they are still based on marginal p-values while continuing to maintain the type-1 error rate control even under such positive dependency. Unfortunately, they can be quite conservative, and hence can lose power, when such dependency is moderately high. Moreover, they can fail to control the type-1 error under negative dependency. While these two tests are widely used, theoretical results regarding the validity of their application have only been done in the case of normal statistics with certain correlation structure [(Hochberg and Rom (Citation1995), and Samuel-Cahn (Citation1996)], or t-statistics with same denominator representing an estimate of the common population standard deviations [Sarkar and Chang (Citation1997), and Sarkar (Citation1998)]. These assumptions do not hold in the two-endpoint problem addressed here because the endpoints almost always have different population variabilities.
Under normal distributional settings, which are most commonly used for global testing in practical applications and where the dependency among test statistics is parametrically represented through correlation coefficients, it is possible to capture the dependency quantitatively, and hence more fully than the Šidák’s (Citation1967) and Simes (Citation1986) tests, while improving the Bonferroni method. However, this idea of improving the Bonferroni method has so far been limited to the case where the population correlations are assumed known (see, e.g., Xie (Citation2012) and the references therein). Of course, one can consider replacing the known correlations in these methods with their suitable estimates to make them fully data-adaptive, but there is no theoretical justification that these would ultimately control the type I error rate. With correlations being rarely known in practice, tightening the Bonferroni type-1 error rate control through explicit use of sample correlations and providing a theoretical justification of such control would be an important objective.
In this paper, we consider achieving the above-mentioned objective by considering the two-mean testing problem under a normal distributional setting with unknown population correlation and variances. Our goal is to test the two hypotheses, with the aim of rejecting at least one, and preferably both. This testing scenario commonly arises in pharmaceutical studies. We propose a new procedure in this setting that utilizes the Bonferroni test based on the usual (marginal) Student’s-t test statistics but uses a data-adaptive critical value that explicitly incorporates the sample correlation coefficient. The confidence interval approach of Berger and Boos (Citation1994) is employed to make use of the sample correlation. More specifically, we first theoretically prove that the type-1 error rate of the Bonferroni method based on Student’s-t statistics (or their absolute values) with any fixed critical value is strictly decreasing in the unknown correlation coefficient (or its absolute value). These decreasing properties allow us to estimate the type-I error rates for both one- and two-sided testing problems, without relying on computations generally required in the application of the Berger and Boos (Citation1994) approach. We simply are substituting the unknown correlation coefficient (or its absolute value) with its lower confidence limit, given a fixed confidence coefficient, into the error rate formulas. Bounding these estimated error rates from above by the nominal level α allows us to produce correlation-adaptive critical values that are smaller than the traditional Bonferroni critical values but still control the type-1 error rate. The fact that such adaptive Bonferroni methods can provide much tighter control of the type-1 error rate than their regular, non-adaptive versions over a wide range of choices for the confidence coefficient and level of significance is demonstrated numerically.
It is important to note that the Berger and Boos (Citation1994) approach to estimating population correlation using its interval estimate in multiple testing scenarios was taken before in Tamhane et al. (Citation2012). However, it was for a different problem, namely, the development of a two-stage group sequential design for testing primary and secondary endpoints controlling familywise error rate (FWER). Moreover, unlike here, they considered large-sample settings, which allowed them to assume the -test statistics to be normally distributed and use large-sample confidence interval for the unknown correlation. Additionally, these authors only showed a directional relationship between the FWER and the correlation via numerical analysis as they were unable to show the relationship analytically.
The paper is organized as follows. Section 2 introduces our proposed ‘correlation-adaptive Bonferroni’ methodologies for both one- and two-sided testing problems. The process of computing the correlation-adaptive critical values in these methods is described in Section 3. In Section 4, we present these critical values for a wide range of sample sizes, before numerically showing in Section 4 how our methods compare with the corresponding traditional, non-adaptive Bonferroni methods in terms of type-1 error rate control and power. Concluding remarks are made in Section 5. These remarks include comments on (i) the novelty of theoretical results we obtain in this article towards application of the Berger and Boos (Citation1994) approach, and (ii) possible extension of the proposed correlation-adaptive Bonferroni to its Holm-type stepdown analog for simultaneous testing. Detailed proofs of the technical results needed to develop our proposed method are provided in the Appendix 1.
2. Proposed methodologies
In our setting, a test treatment is compared to a control treatment on two outcome measures and
that are jointly distributed as a bivariate normal with a covariance matrix
.
and with the following pair of means:
Given pairs of observations
,
for the test group, and
pairs of observations
,
for the control group, our problem is to test the intersection
of the following two one-sided null hypotheses:
against the union of one-sided alternative hypotheses:
or the intersection of the following two null hypotheses:
against the union of two-sided alternative hypotheses:
subject to a control of the type-1 error rate at .
Note that for the one-sided testing problem, the least favorable configurartion, i.e., the point in the parameter space of for which type-1 error is maximized is
. Therefore in the one-sided testing problem, we can control type-1 error if we define and test the null hypotheses exactly as in the two-sided testing problem, i.e.,
Let
where is the sample mean corresponding to
, for
, and
We seek to improve the Bonferroni test by adapting it to the correlation between and
through
, with
.
the pooled sample correlation between and
. More specifically, we attempt to find a critical value
, depending on
, such that
or such that
depending on whether is tested against a one-sided alternative
or against a two-sided alternative
.
Towards finding and
, we first note the following distributional results:
independently distributed as and
respectively, with
which equals under
From these results, we obtain the theorem below:
Theorem 1. The following results hold:
The probability depends on the nuisance parameters,
only through
and is strictly increasing in
, for any fixed
.,
The probability depends on the nuisance parameters,
only through
and is strictly increasing in
, for any fixed
.
This theorem, a proof of which is presented in Appendix 1, facilitates the calculation of and
using a slight modification of the confidence interval approach of Berger and Boos (Citation1994). Specifically, let
, and
be a lower confidence limit for
based on
with confidence coefficient
. Then, since
and is strictly increasing in
, we have
=
=
The desired guaranteeing (2.1) then can be obtained by equating
to
, that is, by solving the equation below for
, for any fixed
:
It is worth noting that , and so
is less than or equal to the Bonferroni critical value
satisfying
. In other words, the resulting modification of the Bonferroni test for testing
against
will have a larger rejection region.
The satisfying (2.2) can be obtained in the same manner by using the fact that
and
is strictly increasing in
, and utilizing a lower confidence limit
of
based on
with confidence coefficient
. More specifically,
can be obtained by solving the equation below for
, for any fixed
:
Since is smaller than the Bonferonni critical value
satisfying
for testing
against
, our modification of the Bonferroni test will have a larger rejection region, and hence more power, than the usual Bonferroni test.
3. Data-adaptive critical values
This section describes the process of calculating and
, given
from the pooled sample covariance matrix with
degrees of freedom (d.f.). A pseudocode of these calculations appears in Appendix 2. Subsequently, we derive the critical values
and
by solving the corresponding Equationequations (2.3
(2.3)
(2.3) ) and (Equation2.4
(2.4)
(2.4) ) for
. The calculation of
and
involves expressing the probabilities
and
and estimating them by substituting ρ and |ρ| with their respective
lower confidence limit
and
3.1. Expressions of 
and 

Let be the cumulative distribution function of
) having standard bivariate normal distribution with correlation
. Then, from the above-mentioned joint distribution of
and
under
, we see that
and
(3.2)
where is the density of
, the diagonal elements of a
Wishart matrix with
d.f. and covariance matrix
Since
with ,
, and
being distributed independently as
,
and
, respectively (see, e.g., Odell and Feiveson (Citation1966)), we see that
can be expressed as follows:
where ,
and
are the densities of
,
and
, respectively, and
3.2. Lower confidence limits 
and

Although these confidence limits can be approximated by using Fisher’s transformation of , we consider calculating them exactly using the following distribution of
(from sample covariance matrix with
d.f.), obtained from Hotelling (Citation1953):
2F1
,
where is the gamma function and 2
is the Gaussian hypergeometric function:
2F1 =
, with
A lower confidence limit
for
is calculated by solving the following equation for
:
=
. (3.3)
Similarly, a lower confidence limit
for
can be calculated by solving the following equation for
:
=
, (3.4)
where
3.3. Calculation of 
and 

We estimate , given
,
by replacing
with its lower confidence limit
to obtain
=
,
where is calculated using the cumulative distribution function of central Student’s t with
d.f. The
is then obtained by solving the equation
for
.
Similarly, is calculated by estimating
, given
,
by replacing
with its lower confidence limit
to obtain
=
,
where is calculated using the cumulative distribution function of central Student’s t with
d.f. and solving the equation
for
.
4. Critical values
present the critical values of our proposed correlation-adaptive Bonferroni procedures, respectively, for one- and two-sided testing problems. For each configuration of sample size and observed sample correlation coefficient , the table entries are the solutions of the process described in Section 3 for
(one-sided tests) and
(two-sided tests). These solutions were obtained by iteratively changing the critical values and numerically integrating the left-hand side of each equation until a solution was found so that the right-hand side of each equation was within 0.000001 of
.
Table 1. One-sided critical values (= 0.025; β = 0.05 for n < 1,000, β = 0.01 for n ≥ 1,000)
Table 2. Two-sided critical values (= 0.05; β = 0.05 for n < 1,000, β = 0.01 for n ≥ 1,000)
We are providing values for a wide range of observed sample correlation coefficient or its absolute value
, depending on whether the testing problem is one- or two-sided, and for some choices of total sample size
. For sample sizes below 1000, we have used
0.05, while for sample sizes of 1000 and above,
0.01 was used. We elaborate on these choices in Section 5.
Note that for the two endpoints problem, with a two-sided or a one-sided
, the Bonferroni critical values are simply half of their respective
namely 0.025 (= 0.05/2) and 0.0125 (= 0.025/2) for the two- or one-sided testing problems, no matter what the sample correlation coefficient is. As expected, the newly derived critical values increase as the sample size or the sample correlation coefficient increases. This is due to the tighter range of the confidence interval with increased sample size, and the decreasing property of the type-1 error rate with increasing population correlation (approximately equaling the sample correlation for large sample size). Of note is that the new critical values remain close to the corresponding usual Bonferroni critical values when the sample correlation is in the range of −1 to 0.
displays power comparisons between the correlation adaptive Bonferroni, the standard, non-adaptive Bonferroni, Simes, and Šidák procedures. Estimated power calculation was done via 1,000,000 random samples. Comparisons were made for a few configurations of effect sizes for the two endpoints, ranging from equal to substantially different. For meaningful comparisons, configurations of effect sizes were designed to facilitate power comparisons in the range of 80-90%. As expected, the Adaptive Bonferroni has a power advantage over the non-adaptive Bonferroni test that increases with sample size and population correlation. The differences are noticeable, being in the range from 1% to 4%. Šidák’s test gives only a minor improvement over Bonferroni. Simes’s test has its best advantage for effect sizes that are equal. In those cases, its advantage over the adaptive Bonferroni can be in the range of 0.5–1%. On the other hand, when the effect sizes are different, the adaptive Bonferroni has an advantage that can be in the range of 2–2.5%. As we stated before, and elaborated in the next section, the Simes’ test has not been shown to control type-1 error for the testing problem addressed here, namely when the t-statistics are constructed with separate estimates of the population standard deviations, and therefore its validity for this problem is not known.
Table 3. Power (%) comparison. One-sided = 0.025
5. Discussion and concluding remarks
The multiplicity problem addressed in this paper is quite common in clinical trial settings where two treatments are compared on two primary endpoints and evidence of superiority on one of these endpoints is sufficient to obtain regulatory marketing approval. Current solutions to this problem in terms of controlling the type-1 error rate are typically based on dependency-free methodologies (such as Bonferroni test and its various extensions) or on those that only qualitatively utilize positive dependencies (such as Šidák’s (Citation1967) and Simes (Citation1986) tests and their extensions). However, it is generally understood that test procedures that utilize more data-embedded information, such as dependencies among variables, tend to be more powerful. Our proposed data-adaptive version of the Bonferroni method utilizing information through the sample correlation is such a procedure. It is indeed more powerful than its non-adaptive counterpart, as numerically verified.
It is important to note that Simes’ and Šidák’s inequalities were not proven to hold in the testing problem described here and therefore the validity of multiple testing procedures based on these two tests is questionable. Hochberg and Rom (Citation1995) and Samuel-Cahn (Citation1996) have shown that Simes’ test controls type-1 error when the test statistics are jointly bivariate normal for two-sided testing, and with non-negative correlation for one-sided testing. Sarkar and Chang (Citation1997), and Sarkar (Citation1998) have obtained similar results when the test statistics are jointly bivariate t whose marginal t-statistics have been constructed with the same estimate of the standard deviation (sometimes referred to as ‘the standard bivariate t of the Dunnett type’). For the problem at hand, the marginal t-statistics do not share the same estimate of the standard deviation, and therefore, the resulting bivariate t-distribution is not of the Dunnett type. It is unknown whether the results proven in Sarkar and Chang (Citation1997), and Sarkar (Citation1998) hold for this problem. Moreover, it has been shown in Hochberg and Rom (Citation1995), and Samuel-Cahn (Citation1996) that Simes’ test has an inflated type-1 error for negatively correlated normal statistics with one-sided testing; and since the value of the population correlation is rarely known and can be negative, the validity of the Sime’s test in the testing problem described here is questionable.
The arguments above regarding one-sided testing also apply to Šidák’s inequality. Nevertheless, the results obtained in this paper allow us to state the following: 1. The Adaptive Bonferroni method is never less powerful than Šidák’s method for two-sided testing since our method allows us to replace the unknown correlation with a less conservative correlation resulting from the use of the confidence interval for the unknown population correlation. If the confidence interval does not cover zero, then our critical values will be less conservative than Šidák’s critical values, otherwise, they will be the same. By implication, we have proven that 1: Šidák’s inequality holds for the absolute values of two t statistics whose joint distribution is of the form described here (the standard deviations have separate estimates), an important result on its own; and 2: For the one-sided testing problem, it is generally (but not always) true that for positively correlated statistics, our method will result in less conservative critical values than those obtained by assuming that the correlation is zero (independence in the normal case) as is done by Šidák. However, Šidák’s method can inflate type-1 error if the population correlation is negative, while our method is valid for that case.
One might consider using Hotelling’s T2 to test the global null hypothesis for our setting. However, the resulting test does not possess the “Consonance” property of Gabriel (Citation1969); that is, following the rejection of the global null hypothesis, the rejection of any of the individual hypotheses is not guaranteed, and they must each be tested and rejected by their own a -level test. This may lead to loss of power for the rejection of any of the individual null hypotheses. On the other hand, the Bonferroni as well as our adaptive version of it, being in the class of Union Intersection (UI) tests, are consonant, and therefore do allow for the rejection of at least one individual null hypothesis whenever the global null hypothesis is rejected. A UI test allows for the allocation of different portions of type-1 the error to the marginal Student’s t-test statistics, thereby adapting the test to the possible difference in effect sizes between the two endpoints. Also, it is amenable to its applications as a stepwise procedure, starting with the global test and, depending on the rejection of the global null hypothesis (and so at least one individual hypothesis), allocating the full nominal type-1 error to the other hypotheses, thereby increasing the power to reject the second hypothesis.
The monotonicity of the type I error rate for Bonferroni global testing involving one-sided (or two-sided) tests with respect to the population correlation (or the absolute value of the population correlation) is an important theoretical result in the process of carrying out the main maximization step in the Berger and Boos (Citation1994) approach without computations. While this property is known in the literature for multivariate (or absolute-valued multivariate) normal random variables, they are not available for the joint distribution of the marginal’s (or absolute-valued marginal
’s) in Hotelling’s T2, and so these results proven in the bivariate case in this paper are important in their own right. Tamhane et al. (Citation2012) have made use of a similar monotonicity property for normally distributed test statistics, although for a different problem, in the aforementioned step of the Berger and Boos (Citation1994) approach without computations. However, they verified this property numerically.
The proposed correlation-adaptive Bonferroni method for global testing can be used to develop a Holm-type stepdown method for simultaneous testing of the individual null hypotheses in the present context. For instance, let us consider the one-sided testing problem. With and
denoting the null hypotheses corresponding to
and
, respectively, we can describe this so-called correlation-adaptive Holm method controlling the (familywise) type-1 error rate at
as follows:
Do not reject or
if
Do not reject but reject
if
Reject both and
if
A correlation-adaptive Holm method for the two-sided testing problem can be similarly proposed in terms of
and
.
The correlation-adaptive Bonferroni methodology can be further extended to more than two endpoints, although a difficulty arises due to the increased dimensionality. One may need to resort to some efficient Monte-Carlo numerical integration methods to address the testing of more than two endpoints. This extension will also require some additional theoretical results. A more pragmatic approach to reduce the dimensionality problem is to use the bivariate results obtained here and to devise an upper bound for the case of more than two endpoints. The first method can readily be described for the case of three endpoints as follows (one-sided bounds are described here with obvious changes to two-sided testing):
and since
This bound relies on the univariate and bivariate probabilities only. We can then replace each of the bivariate probabilities on the righthand side of (2.5) using the lower confidence limit of the correlation between the respective statistics and apply the Berger and Boos (Citation1994) method as was done for the two-endpoint problem. Two types of extensions of (2.5) can be made for more than three endpoints: The first is based on extending (2.5) to endpoints using Kounias (Citation1968) inequality:
and using a lower confidence bound for each (bivariate) correlation and the Berger and Boos (Citation1994) method in (2.5).
A second approach is to utilize the closure principle of Marcus et al. (Citation1976) to test all intersection hypotheses of cardinality at level
. In this approach, any intersection hypothesis
of cardinality
, can be rejected at level
by testing and rejecting all intersection hypotheses of cardinality
implying
at level
. Applying this idea recursively to testing
endpoints, the following procedure will control type-1 error rate:
Reject any hypothesis
corresponding to endpoint
provided all intersection hypotheses of cardinality
implying
have been tested and rejected at level
We use (2.5) to test all hypotheses of cardinality
.
The tightness (i.e., how far the above bounds are from the exact type-1 error) of the above approaches depends on the correlation matrix among the endpoints which in turn determines whether higher dimensional probabilities are diminishingly small compared to the two-dimensional probabilities. Our preliminary evaluation suggests that for small to moderate correlations, the univariate and bivariate probabilities do provide a tight upper bound on type-1 error. Further work is currently undertaken to examine the above bounds. As an example of this point, consider a setting with three endpoints, and sample sizes of 500 in each of two groups, with all observed sample correlations being 0.5. With a one-sided type-1 error of 0.025, the Bonferroni test will use a critical value of 0.025/3 =0.0083 for testing each of the three hypotheses. Applying (2.5) with a lower
=0.0001) confidence limit and the Berger and Boos (Citation1994) method, we get a critical value of 0.00867 which is a slight improvement over the Bonferroni test. If we were to consider the asymptotic critical value (
using a three-dimensional normal with all correlations equal to 0.5 to approximate the joint distribution of the test statistics, we would use a critical value of 0.0095 (estimated using a Monte Carlo simulation) making our critical value 0.00867 slightly conservative. Note that the use of the asymptotic critical value may cause some type-1 error inflation due to the use of the normal distribution instead of the t-distribution, and the use of the observed correlations to replace the unknown correlations. Thus, the conservatism of our critical value is no more than the difference derived from the asymptotic distribution, and practically can be much lower.
A similar problem with observed sample correlations of 0.9 gives a critical value of 0.01205 from our method while the Bonferroni test remains unchanged with a critical value of 0.0083. Again, considering the asymptotic distribution as a three-dimensional normal with all correlations being 0.9, the critical value is 0.0145 (estimated from a Monte Carlo simulation), making our method with a critical value of 0.01205 slightly conservative but much less conservative than the Bonferroni test.
The method described in this paper can be extended more easily to situations where, following the rejection of either of the primary endpoints, it is desired to test secondary endpoints. The dependencies between the primary and secondary endpoints can then be readily incorporated using the methodology described in this article to devise an improved sequential testing.
Supplemental Material
Download MS Word (68.2 KB)Acknowledgments
We would like to thank Ajit Tamhane for his helpful suggestions on an earlier version of this manuscript. We also thank the anonymous referee and the editor for their insightful comments which helped strengthen this manuscript. We thank Michael Pol for meticulously programming all tables. An R code for generating critical values is available from the authors upon request
Supplemental data
Supplemental data for this article can be accessed on the publisher’s website.
Correction Statement
This article has been republished with minor changes. These changes do not impact the academic content of the article.
References
- Berger, R. L., and D. D. Boos. 1994. P values maximized over a confidence set for the nuisance parameter. Journal of the American Statistical Association 89 (427):1012–1016.
- Gabriel, K. R. 1969. Simultaneous test procedures–some theory of multiple comparisons. Annals of Mathematical Statistics 41 (1):224–250. doi:https://doi.org/10.1214/aoms/1177697819.
- Gou, J., A. C. Tamhane, D. Xi, and D. Rom. 2014. A class of improved hybrid hochberg-hommel type step-up multiple test procedures. Biometrika 101 (4):899–911. doi:https://doi.org/10.1093/biomet/asu032.
- Hochberg, Y. 1988. A sharper Bonferroni procedure for multiple tests of significance. Biometrika 75 (4):800–802. doi:https://doi.org/10.1093/biomet/75.4.800.
- Hochberg, Y., and D. M. Rom. 1995. Extensions of multiple testing procedures based on Simes’ test. Journal of Statistical Planning and Inference 48 (2):141–152. doi:https://doi.org/10.1016/0378-3758(95)00005-T.
- Holland, B. S., and M. D. Copenhaver. 1987. An improved sequentially rejective Bonferroni test procedure. Biometrics 43 (2):417–423. doi:https://doi.org/10.2307/2531823.
- Holm, S. 1979. A simple sequentially rejective multiple test procedure. Scandinavian Journal of Statistics 6 (2):65–70.
- Hommel, G. A. 1988. A stagewise rejective multiple test procedure based on a modified Bonferroni test. Biometrika 75 (2):383–386. doi:https://doi.org/10.1093/biomet/75.2.383.
- Hotelling, H. 1953. New light on the correlation coefficient and its transforms. Journal of the Royal Statistical Society 15 (2):193–232.
- Kounias, E. J. 1968. Bounds for the probability of a union, with applications. The Annals of Mathematical Statistics 39 (6):2154–2158. doi:https://doi.org/10.1214/aoms/1177698049.
- Marcus, R., E. Peritz, and K. R. Gabriel. 1976. On closed testing procedures with special reference to ordered analysis of variance. Biometrika 63 (3):655–660. doi:https://doi.org/10.1093/biomet/63.3.655.
- Odell, P. L., and A. H. Feiveson. 1966. A numerical procedure to generate a sample covariance matrix. Journal of the American Statistical Association 61 (313):199–203. doi:https://doi.org/10.1080/01621459.1966.10502018.
- Samuel-Cahn, E. 1996. Is the Simes improved Bonferroni procedure conservative? Biometrika 83 (4):928–933. doi:https://doi.org/10.1093/biomet/83.4.928.
- Sarkar, S. K. 1998. Some probability inequalities for ordered MTP2 random variables: A proof of the Simes conjecture. Annals of Statistics 26 (2):494–504. doi:https://doi.org/10.1214/aos/1028144846.
- Sarkar, S. K., and C.-K. Chang. 1997. The simes method for multiple hypothesis testing with positively dependent test statistics. Journal of the American Statistical Association 92 (440):1601–1608. doi:https://doi.org/10.1080/01621459.1997.10473682.
- Šidák, Z. K. 1967. Rectangular confidence regions for the means of multivariate normal distributions. Journal of the American Statistical Association 62 (318):626–633.
- Simes, R. J. 1986. An improved Bonferroni procedure for multiple tests of significance. Biometrika 73 (3):751–754. doi:https://doi.org/10.1093/biomet/73.3.751.
- Tamhane, A. C., Y. Wu, and C. R. Mehta. 2012. Adaptive extensions of a two-stage group sequential procedure for testing primary and secondary endpoints (II): sample size re-estimation. Statistics in Medicine 31 (19):2041–2054. doi:https://doi.org/10.1002/sim.5377.
- Xie, C. 2012. Weighted multiple testing correction for correlated tests. Statistics in Medicine 31 (4):341–352. doi:https://doi.org/10.1002/sim.4434.