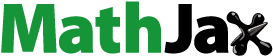
Abstract
It is commonly known that education reproduces, rather than diminishes, social inequality, but how this takes place may vary between educational systems. This paper uses data from a unique longitudinal cross-national project to analyze differences in pathways through the upper years of secondary education between Bergen (Norway), Ghent (Flanders, Belgium) and Barcelona (Spain). Analyses show that social background has the greatest impact on destination in both Years 11 and 12 of upper secondary education, and engagement does not mediate this relationship. Further, we find more commonalities than differences between the cities, despite them representing different types of educational systems.
Introduction
A major finding in sociology of education research is that social background features such as socio-economic status determine educational outcomes beyond ability (Bourdieu & Passeron, Citation1990). As educational outcomes are related to higher education and future job opportunities, the social determination of educational outcomes is consequential for social inequality in society. Although most educational systems do reproduce inequality, there are important variations between systems (Breen & Jonsson, Citation2005). Using data from large-scale international databases, including PISA or TIMSS, studies show that educational systems with early tracking (Gamoran, Citation2009), less standardization (Van de Werfhorst & Mijs, Citation2010) and higher school selectivity (Perry, Citation2018) are characterized by more educational inequality.
However, previous cross-national research comparing social reproduction across systems remains limited to investigating social differences in standardized test scores at a given point in time—e.g., based on PISA test scores. Standardized test scores fail to reflect students’ actual mastery of their curriculum (Perry, Citation2018), thereby biasing our current understanding of between-system differences. The curriculum or track being followed is pivotal in determining later life chances (Shavit & Müller, Citation2000). Moreover, in some systems, educational pathways may be co-determined—directly or indirectly—by teacher evaluations and recommendations (Thys & Van Houtte, Citation2016), which can be socially biased (Jussim & Harber, Citation2005). Students with differing social and ethnic backgrounds, therefore, may have different future trajectories, even when scoring equally on standardized tests at a given point in time (Bernardi & Cebolla-Boado, Citation2014). Limiting scholarly attention to standardized test scores thus leads to an underestimation of educational inequality.
The current study has two aims: firstly, to assess the relative impact of social background on actual pathways through upper secondary education, when controlling for academic factors, and secondly, to understand engagement influence how social background and grades affect pathways. More specifically, we account for three pathway outcomes: attendance of an academic or vocational track, a delayed school career, and dropping out of the educational system altogether. Hence, our first research question investigates differences in pathways through upper secondary education between the educational systems.
To categorize educational systems, we use the heterogeneity management model categorization as developed by Mons (Citation2007). She distinguishes between four heterogeneity management models (that is, the separation model, and individualized, uniform, and à la carte integration models, further explained below). This categorization has been shown to relate to social reproduction (Dupriez et al., Citation2008). In short, social reproduction would be highest in systems utilizing the separation model. However, the study by Dupriez et al. (Citation2008) used cross-sectional PISA data, which fails to account for track location and/or curriculum followed, thereby potentially underestimating social inequality.
In the current study, we investigated differences in social reproduction between three educational systems: the Flemish (the Dutch-speaking, northern part of Belgium) system in Ghent, the Spanish system in Barcelona, and the Norwegian system in Bergen using longitudinal data. Respectively, these systems are seminal examples of a separation model, a uniform integration model, and an individualized integration model, the three most common models in continental Europe.
The second research question is linked to engagement, as it is a crucial non-cognitive factor underlying success or failure (Fredricks et al., Citation2004), and engagement in fact is the most important determinant of school dropout (Janosz et al., Citation2008). Moreover, engagement is connected both to prior achievement (Fredricks et al., Citation2004) and to social background factors such as socioeconomic status and ethnicity (Demanet & Van Houtte, Citation2014). Therefore, we ask to what extent engagement mediates the influence of academic and social determinants on students’ pathways in upper secondary education?
The paper is organized as follows: starting out with a section outlining the theoretical background of the paper, ending in hypotheses corresponding to the two research questions, followed by a section describing data and methods, as well as the measures used to operationalize the concepts in the paper. Thereafter, results are presented and finally the findings are discussed in relationship to the hypotheses.
Background
Educational Pathways: A Mechanism for Reproduction?
Students’ educational pathways are commonly described as sequences of transitions (Lucas, Citation2001). Initially this was understood as a binary divide, distinguishing between those who stay at school and those who drop out in a given year of schooling (Mare, Citation1980). However, Lucas (Citation2001) pointed out that a more “varied set of options” better reflects the actual choices students in upper secondary school face today (Lucas Citation2001: 1650). Not only do students have to decide whether to continue their education (versus dropping out), in most educational systems they must also decide between an academic or vocational track (Breen & Jonsson, Citation2000; Lucas, Citation2001), and progressing at the normal pace instead of repeating their current grade (Jimerson, Citation2001).
Boudon (Citation1974) highlighted the role of social differences in choices of pathway. He distinguished between the primary and secondary effects of social class. The primary effect of social stratification refers to differences in achievement as the result of socioeconomic status. However, social background affects educational decisions regardless of achievement (i.e. secondary effect)—as students from different backgrounds with similar levels of achievement might still not follow the same educational pathways. Further, low SES students are more likely to end up in vocational tracks (Thys & Van Houtte, Citation2016), to drop out (Mare, Citation1980) and to experience grade repetition (Jimerson & Ferguson, Citation2007). Research shows that the decisions made at each transition point are pivotal for students’ pathways and thus for their future educational careers (Breen & Jonsson, Citation2000) and labor market opportunities (Gambetta Citation1987, Iannelli & Smyth Citation2008).
Heterogeneity Management Models
Students and their parents make choices within the educational structures available to them (Breen & Jonsson, Citation2000). Hence, as educational systems vary in structure, the possible pathways students may take within the systems also vary. The most used distinction is a dichotomous one, namely between comprehensive and tracked educational systems (e.g., Bol et al., Citation2014). However, this binary divide does not represent the complexity of heterogeneity management models—that is, the various ways systems deal with differences in ability, achievement, and future interests (Dupriez et al., Citation2008). Mons (Citation2007) identified four models by which educational systems handle academic heterogeneity, which is placed in a quadrant alongside two axes: the first ax distinguish between comprehensive education and ability grouping/tracking, and the other between automatic promotion, or contingent promotion by letting students repeat their current grade in the following academic year (e.g., Demanet & Van Houtte, Citation2016) (see ).
The “separation model” (Germany, Flanders) employs rigid tracking and uses grade repetition extensively to cope with low achieving students. Its opposite, the “individualized integration model” (Norway, Poland) is comprehensive and repetition of grades does not occur. The “à la carte integration model” (UK, US) combines ability grouping with low levels of retaking a year, and the “uniform integration model” (France, Spain) is comprehensive and employs repetition of grades (Janmaat & Mons, Citation2011; Mons, Citation2007). The differences between models will be discussed in more detail below, focusing on the three models corresponding to the three countries used in the paper.
First, the extent to which tracking is applied is an important feature in differentiating between educational systems (Allmendinger, Citation1989; Hanushek & W Ößmann, Citation2006; Lavrijsen, Citation2013; Mons, Citation2007). Tracking refers to the assignment of students to different groups, who are offered different curricula based on their academic ability (Gamoran & Mare, Citation1989). In most systems, students must choose between different tracks at some point in their educational career, but in some systems, the distinction between tracks is more rigid than in others. The separation model, for example, is known for its rigid tracking (Mons, Citation2007). In such systems, the curricula in the vocational and academic tracks differ both in the content that is offered and in the way this content is taught. Curricula in vocational tracks are less demanding and more focused on applied skills linked to labor market needs. In contrast, the academic track is the more demanding track, which prepares students for higher education (Stevens & Vermeersch, Citation2010). Due to the extensive tracking in separation models, it becomes difficult to change from the lower-esteemed vocational track to the academic track, resulting in less upward mobility (LeTendre et al., Citation2003). In the individual integration model, there is no tracking, and students are given the same curriculum until the start of upper secondary education, at age 16 (Imsen & Volckmar, Citation2014). As a result, between-track mobility in the individual integration model happens only during upper secondary education. The uniform integration model constitutes a middle ground between the separation model and the individual integration model, giving students a common core curriculum complemented with optional subjects. In contrast to the individual integration model, the uniform integration model applies ability grouping to manage academic heterogeneity, albeit to a lesser extent than the separation model (Mons, Citation2007). Thus, in the uniform integration model, the choices that students must make when starting upper secondary education are less restricted than in separation models, yet more restricted than in individual integration models (Bernardi, Citation2012).
Second, educational models differ in the way they deal with low achievers. Roughly, two different approaches can be distinguished, namely, automatic progression to the next grade with intensive individual guidance and teaching or holding the student back for a year in order to improve their achievement (Jimerson, Citation2001). In separation models and uniform integration models, students are held back for a year, and it can be applied quite extensively (Goos et al., Citation2013). However, in an empirical test of the pathways of students in the four distinguished educational models, Dupriez et al. (Citation2008) show that tracking and grade retention function as “connected vessels.” Indeed, grade retention occurs relatively less in a stringently tracked system, and authors find that grade retention, therefore, is most extensively applied in the uniform integration model. Proponents believe that giving students “the gift of time” will put them back on track for normal educational growth (for a review, see Jimerson, Citation2001). Individual integration models are characterized by automatic promotion (Dupriez et al., Citation2008). Previous research established that retention is an important predictor of academic and nonacademic outcomes in the future (Martin, Citation2011). For example, those who must repeat a year are more likely to disengage behaviorally from school and to experience social rejection (Demanet & Van Houtte, Citation2013, Citation2016). Grade retention is also the most single important predictor of unqualified dropout (Jimerson et al., Citation2002; Lamote et al., Citation2014).
Heterogeneity Management Models and Social Inequality
As we noted above, we know that in most educational systems social background determines academic attainment, at least to a certain extent. However, research suggests that the extent to which social inequality is reproduced in educational systems depends on the educational model that is applied (Dupriez & Dumay, Citation2006). For instance, Dupriez and Dumay (Citation2006) demonstrate that students’ educational achievement in school systems that utilize more integrative models, such as the individual and uniform integration model, depend less upon their socioeconomic backgrounds. In contrast, social reproduction is higher in educational systems characterized by separation models (Dupriez et al., Citation2008; Mons, Citation2007).
First, we know that track placement is highly dependent on students’ socioeconomic background (Dupriez & Dumay, Citation2006). Low socioeconomic status students are directed toward technical and vocational tracks, partly because of self-selection processes (Boone & Van Houtte, Citation2013a) and partly because of biased teacher recommendations (Boone & Van Houtte, Citation2013b; Thys & Van Houtte, Citation2016). Especially when the choice between tracks occurs early on in students’ educational career, the influence of social background would be larger (Dupriez & Dumay, Citation2006). As such, we expect the role of social factors in track choice to be larger in separation models, as compared to individual and uniform integration model, where the choice occurs at a higher age (Dupriez & Dumay, Citation2006).
Second, also grade retention is subject to inequality. Specifically, research shows that not all poor performers are equally likely to be retained. For example, teacher attitudes, such as their preference for retention (Bonvin et al., Citation2008; Goos et al., Citation2013), affect teachers’ decision to retain students. Importantly, some socio-demographic student characteristics, such as students’ gender and socioeconomic background (Jimerson et al, Citation1997), affect the likelihood of grade retention. Given the important cognitive and non-cognitive outcomes of retention as discussed above, such inequality can be quite consequential for educational opportunities. Given the strong links of retention to drop-out (Jimerson, Anderson, & Whipple, Citation2002; Stearns et al., Citation2007), this might be a reason why research finds social risk factors to be so important in drop-out as well (Rumberger, Citation1987, Citation1995). To the best of our knowledge, no cross-national research has yet considered whether social inequality in grade retention and dropout differs between educational systems cross-nationally. Based on the previously discussed research we hypothesize the links to be stronger in the separation model. As such, we reach the first hypothesis:
H1: Social inequality in students’ pathways, in terms of selection in tracks, grade retention, and dropout, is most pronounced in Ghent (separation model), and least pronounced in Bergen (individualized integration model, with Barcelona (uniform integration model) occupying the middle position.
Specifically, we expect students with social risk factors to be more likely to end up in the vocational track, to be delayed, and to drop out of education in Ghent, as compared to the other two systems.
The Role of Engagement
It is one thing to establish that there is social inequality in pathways, but quite another to explain why certain groups of students are more likely to succeed or fail. An important concept that can enlighten such linkages is engagement (Fredricks et al., Citation2004). Engagement is a multi-faceted construct, consisting of three dimensions. Behavioral engagement captures attendance, active participation in class, or (mis)conduct on school premises. Emotional engagement consists of the emotional reactions and feelings students have regarding learning, studying, and the school environment. Cognitive engagement is captured by students’ willingness to invest time and effort in studying and learning and is analogous to (intrinsic) motivation (see Fredricks et al., Citation2004, for an influential review of these constructs). It is important to understand the determinants of engagement, as behavioral (Roeser & Eccles, Citation1998), emotional (Klem & Connell, Citation2004), and cognitive engagement (Schunk & Zimmerman, Citation2013), all of which are linked to self-regulated learning, and hence to academic success.
Scholars explain the origins of disengagement by referring to the perceived control theory (Demanet & Van Houtte, Citation2014; You et al., Citation2011). In short, this theory states that students will disengage when they perceive their own efforts at school to be pointless in improving their scholastic attainment. Such findings of perceived powerlessness are more prevalent among certain groups of students. First, academically-lagging students are more likely to disengage, because they tend to have lower academic self-esteem (Régner & Loose, 2006). Second, because of their lower educational opportunities, students from lower socio-economic status and ethnic minority groups are more likely to feel powerless at school, which explains why students from these backgrounds are more likely to disengage alongside the three dimensions (Fredricks et al., Citation2004). As such, students exposed to academic and social risk factors are especially prone to disengaging at school. Notwithstanding the influence of these determinants, engagement is seen as a malleable student feature and therefore as a pivotal mechanism which policy makers, school administrators, and teachers can use to curb failure and expedite educational success (van Uden et al., Citation2014).
This is particularly important as engagement is connected to students’ pathways. Most research in this area highlights the effects of dropping out, noting the higher chances of leaving the educational system unqualified by behaviorally, emotionally, and cognitively disengaged students (Lamote et al., Citation2014; Janosz et al., Citation2008; Steinmayr & Spinath, Citation2009). Dropping out is understood as the culmination of a long process, which sometimes started in primary school, in which students lose interest in their education and disconnect from it (Janosz et al., Citation2008), which is captured in the concept of disengagement (Fredricks et al., Citation2004). Studies by Lamote et al. (Citation2014) and Janosz et al. (Citation2008) demonstrate how (dis)engagement trajectories are linked to eventually dropping out. As such, they classify students in different types of engagement trajectories and chart these groups’ chances of eventually dropping out. These studies portray the need to chart engagement longitudinally throughout secondary education. However, they do not explain how engagement shapes the immediate trajectories in secondary education. Given the importance of engagement for academic success, we expect that engagement will be important for students’ immediate secondary education pathways. Moreover, given that results reported in earlier literature found engagement to be affected by academic and social risk factors, we reach our second hypothesis:
H2: Engagement reduces the influence of academic and social determinants on students’ pathways in upper secondary education, in all educational systems.
Data and Methods
Data
Data from the ISCY project (www.iscy.org), a longitudinal international project undertaken in 14 cities around the world, were used in this study. The intention when gathering the data set was to explore student completion, drop out and trajectories through upper secondary education, with the opportunity to account for attitudes, motives and engagement. The starting point in all countries was a common survey, distributed to students in Grade 10, carried out in 2013–2014, which cover issues of motivation, engagement, and attitudes. Later, this initial survey was followed up by data from new surveys of the same cohort, or by using register data covering the same cohort, creating a longitudinal data set that is comparable across countries. We followed students’ pathways for two full years after Grade 10.
For the analyses in this paper, we used data from three cities: Bergen (Norway), Ghent (Belgium) and Barcelona (Spain). The main reason for choosing these three educational systems was because they represent good examples of Mons' typology, and as systems they are also the most prominent ones within Europe. Norway (Bergen) would be within the "individual integration model” of the Nordic countries which, as explained above, consists of comprehensive lower secondary education with automatic promotion of students. Belgium (Ghent) is an example of the “separation model,” with a division between academic and vocational tracks, at the beginning of lower secondary education and with the use of grade repetition as a strategy for managing heterogeneity. Spain (Barcelona) would be the example of the “uniform integration model,” with a formally comprehensive lower secondary education but with an ability grouping, and with an intensive use of grade repetition. A second reason for choosing these three systems is the unique comparable data set, based on longitudinal monitoring of the sample of 10th-grade students in all cities.
Our aim in the paper is to compare student pathways from Grade 10—the modal grade for 15-year olds—through the two following school years, Year 11 and 12. For students who are on a continuation pathway, this corresponds to Grades 11 and 12. However, students who were held back or who changed their path through upper secondary education may be in a lower grade than the year they should have been in. These students are labeled as delayed (see below). Even though the three datasets are made to match each other, there are some challenges linked to the differences in the data. Information in the Norwegian data set is based on register data with little missing data, while the information in the Belgian and Spanish data sets is based on panel data (from surveys), with slightly more missing data. However, this challenge to the comparison is handled through the set-up of analyses (see below).
Measures
Outcome
In order to analyze pathways, we created two measures to chart students’ positions in the 11th and 12th years of schooling respectively. In order to account for various sets of options (Lucas Citation2001), we joined three components in this measure, namely the track the student was in (academic vs vocational), as well as if the student was progressing at the intended pace or was held back in a grade below (on time or delayed), and if students had dropped out of the educational system. Eventually, this led to a measure with five categories: if students were in the academic or the vocational track if they had been held back in compulsory education, if they were delayed or if they were outside upper secondary education. It should be noted that to make this measure meaningful across systems, this operationalization necessarily neglects some of the complexities of the specific systems. For instance, in Ghent (Flanders), students belong to four different tracks (academic, artistic, technical, and vocational; see Demanet et al., Citation2019), which were collapsed into two tracks in the analyses, to match the structure in the other two countries. More specifically, given the orientation toward higher education, students from the artistic and academic track in Ghent make up one category, and students from the technical and vocational track the other one. As students in Ghent had already chosen their track at the baseline-point in this study (Year 10), we registered that 69% of students in Ghent were in the academic track, while 31% were in the vocational track in Year 10. displays the initial comparative outcome categories used in the data.
However, due to country differences which caused an empty cell problem (as visible in ), the measure used for Year 11 distinguished between three different positions: on time in the academic track, on time in the vocational track, and the other three categories collapsed into one called “delayed/outside.” For the analyses of Year 12, we separated out delayed students (which also encompassed students who have been held back) from students who were outside the system and thus have four different positions in the analyses.
Independent Variables
In the analyses we distinguish between our variables in focus, city and background resources as parental education level and grades, control variables and mediating variables. Bergen is set as reference category in the analyses.
For parental education we distinguished between two groups: those who come from families where at least one of the parents have education above ISCED 3 level (post-secondary education), and those coming from a family where upper secondary education certificate (ISCED 3) is the highest level of educationFootnote1. For simplicity will we in the text and tables talk about the first group as “parents with higher education.” Academic results were measured by one of the questions in the initial questionnaire, in which students stated that they expected good or less good (pass or poor) results in Grade 10. The control variables are gender, immigrant background (born abroad or both parents born abroad) and if the father worked full-time or not. The reason for the last control is that working life patterns are quite different in the three countries compared, and to control for this we measure the parental labor market situation by a variable indicating if the father worked full-time or not, coded as 1 for those not working full-time. Our mediating variables are different forms of engagement, which were measured through indexes of behavior engagement, cognitive engagement, and emotional engagement, as used by Fredricks et al. (Citation2004). All these measures are centered on the mean, to make interpretation easier. Frequencies for the independent variables are presented in and .
Table 1. Frequency for dichotomous independent background variables, by city.
Table 2. Indexes used as independent variables, measures of engagement.
Data Analyses
We used multinomial logistic regression to analyze the two dependent variables. Multinomial logistic regressionFootnote2 is a type of regression in which the outcome variable has three or more categories (Long & Freese Citation2006).
The outcome variables analyzed for Year 11 and Year 12 were based on common denominators of the three educational systems at that point in a student’s educational career. In the analysis of Year 11, the outcome variable had three categories: if the student was in the academic track, in the vocational track and the three other categories collapsed into one category, “delayed/outside.” In the second regression, on the student’s status in Year 12, we can distinguish between four outcomes: on time in the academic track, on time in the vocational track, delayed (regardless of track) and outside education. Hence, in Year 12 we also control for dropout in all three systems.
We conducted three analyses, the first two on destination was run on data from all cities, with one model with controls for city and interactions between city and all variables,Footnote3 while the last analysis also have a second model, the first one controlling for social and demographic factors. In the second model we also controlled for engagement, to investigate mediation (Baron & Kenny, Citation1986). In order to test the mediation effect in the nested models we have used the khb-test (Karlson et al., Citation2012). In all regressions are significance of regression coefficients (unstandardized B and standard errors displayed in table) calculated compared to the academic track.
The coefficients in the tables presenting the regression analyses are not directly interpretable and must thus be recalculated into probabilities, using average marginal effects (AME). The AMEs calculated as a part of the STATA output are presented as figures in which each line sums to 100%, which thus gives the likelihood of a person with the specified trait being in one of the outcomes. T-tests (calculated in STATA) is used to establish if differences are statistically significant.
Results
We will first analyze the first hypothesis, by focusing on students’ pathway into year 11 and year 12 respectively, and then present an analysis focusing on the mediating effect of engagement.
Year 11 Destination
The first transition we study is students’ transition into Year 11, focusing on student position or destination in the educational system at Year 11. For students in Bergen and Barcelona this also meant students had to choose between the academic or vocational track. As students in Ghent were already tracked in Year 10, they had to choose whether to remain in their current track, or to change. The regression including all three cities in one model is displayed in .
Table 3. Multinomial regression analysis, on destination in Year 11 of upper secondary education.
To investigate the first hypothesis, we focus on parents’ educational level and students’ grades. The pattern is quite similar for the three countries among more resourceful students: particularly when comparing those who have parents with higher education, but also when comparing those who expect good grades. The pattern for students who either come from a family without higher education experience or who is expecting less good grades is more varied.
illustrates differences between the three systems, controlling for means of other variables. Across the more resourceful students the differences across the three systems are quite small, as a clear majority of students are in the academic track. The share in the academic track is significantly higher in Barcelona, compared to the other two cities. However, if we instead compare families where parents do not have higher education, the differences are much larger and all are significant, both for being in the academic and in the vocational track. Students in Barcelona are still very likely to be in the academic track, and only a minority, 11% are in the vocational track, and 20% are delayed or outside. Over half of the students in Ghent are in the vocational track, while the share of student in Bergen in the vocational track is 46%. If we instead compare the marginal effects among students who expect less good grades, we see that students in Bergen are most likely to choose the vocational track, 52%, compared to 35 in Ghent and 12 in Barcelona. Students in Barcelona more likely to be delayed or outside, rather than being in the vocational track. All differences are statistically significant. Apart from less resourceful students in Barcelona are only a very small percentage of students delayed or outside the educational system in the 11th year of education.
Year 12 Destination
In the analyses of the transition to Year 12, we distinguished between four categories: academic on track, vocational on track, delayed or outside, and the regression is displayed in for all cities.
Table 4. Multinomial regression analysis, on destination in Year 12 of upper secondary education.
As in the regression for Year 11, the focus in the analyses is on city, parents’ educational level and grades, and the other background variables only functioning as controls. Again, we find an effect of not expecting good grades and not having parent’s with higher education, as this increases the chances of being in the vocational track or delayed. There are also city differences, and to ease interpretation these differences are presented as AMEs in . All differences are statistically significant.
Figure 4. AME of being in the academic track, the vocational track, delayed or outside in Year 12, by parents’ level of education, grades and city. Baseline: Bergen—academic track.
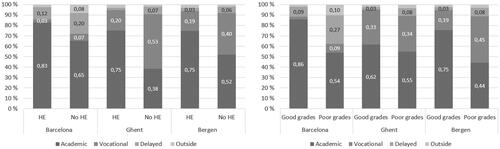
The regression for Year 12 showed the same pattern as the regression for Year 11, but as stated, those who were delayed were separated from those who were outside upper secondary education in Year 12. As displayed in , being outside education at this stage was quite uncommon, as this only applies to 2–3% among all groups apart from less resourceful students in Barcelona. But this finding might to some extent be linked to panel attrition in the data. The general pattern is the same as for Year 11, more resourceful students, from educated families and with good grades are more likely to be in the academic track, while the pattern is more varied for students who either come from families without higher education or who expect lesser grades. It is only among less resourceful students in Barcelona (either no HE or low grades) we find a higher share of students outside as well as a higher share of students who are delayed. Thus, the overall differences between the educational systems are quite stable at the two transition points.
In sum, the two regressions indicated that having parents with a low level of education is the variable that had the strongest impact on all outcomes. Variables traditionally used in the analysis of educational inequalities, such as gender or the employment status of parents, only had minor effect. Regarding gender, it is more likely that inequalities are not so much between pathways as within them, but this question is beyond the focus of this article.
Mediating Effect of Engagement
In , we test the mediating effect of engagement, by entering the indexes as z-scores in Model 2 of the regression and investigating the change in result on parents’ educational level and grades. As indicated in the table, the unstandardized B does not change much, and there is almost no change to the main independent variables when entering the indexes of engagement. It only results in 1–2% points change in AMEs. Tests of nested models determine that these variables have very little effect on the influence of other independent variables, only changing the one of the control variables (grades) slightly. This indicate that there is no mediating effect of engagement on pathway.Footnote4
Table 5. Multinomial regression analysis, on destination in Year 11, with second model controlling for mediating variables.
Discussion
Cross-national research on how and when social inequality affects the educational pathways in different educational systems is rather limited as most international studies are cross-sectional.
Our first research question, therefore, was to investigate whether social inequality affected the pathways that students followed throughout their upper secondary education, and we set out a hypothesis stating that social inequality in students’ pathways (either as selection in tracks, grade retention, and dropout), is most pronounced in Ghent, least pronounced in Bergen, with Barcelona occupying the middle position. Additionally, we argued in the second hypothesis that cognitive, behavioral, and emotional engagement might serve as an underlying mechanism that potentially reduces the influence of students’ socioeconomic background on their educational pathways.
As noted in the first hypothesis, this comparative study makes it possible to analyze the similarities, but also the differences in pathways between educational systems. One similarity across all three systems is that students from highly educated families seem to prefer the academic track. This finding cuts across educational systems, as a clear majority of students in Barcelona, Bergen and Ghent who come from educated families choose to enter the academic track, regardless of if they have good grades or not. This is true also for young people with parents who have higher education, even if they are expecting lower grades (Bernardi, Citation2014; Bernardi & Cebolla-Boado, Citation2014). Even though the three cities represent three different models according to Mons (Citation2007), the pattern is strikingly similar across educational systems. Hence, we did not find support for our first hypothesis, that social reproduction is most pronounced in Ghent, and social mobility is highest in Bergen. Rather it seems as if choice of pathway is least influenced by parental background in Barcelona, as most students seem to prefer the academic path. Among students with lesser grades the pattern is more varied, at least for students in Ghent and Bergen. In Ghent does it seem as if students from families without higher education in general are more prone to choose the vocational track instead of the academic, while this pattern is only true for students who have parents with no higher education and who are expecting lesser grades.
In general, dropping out of school during the first two years of upper secondary education is quite rare, and so is being delayed, apart from in Barcelona, where it is slightly more common (see also Dupriez et al., Citation2008). This can be explained by the institutional design of the Spanish education system, as students must obtain a certificate which enables the student to choose the academic or the vocational pathway for further education at the end of lower secondary school. Students who do not obtain this certificate have no choice but to leave the education system. This contributes to explaining why the percentage of young people in vocational training is lower in Barcelona and is particularly evident among young people with poor grades. In Ghent, on the other hand, compulsory education lasts until age 18 (usually in 12th grade). As a result, it is logical that dropping out occurs much less frequently in the Flemish system.
Regarding the second research question, we found that engagement does not mediate social background effects on students’ pathways, as the few found effects are small. This counters empirical studies that put engagement as a key element in relationship to social origin and educational outcomes, such as drop-out, retention and mobility (Fredricks et al., Citation2004). We do find minor significant effects of engagement, but as these are generally very small, they are not responsible for the observed influence of social background factors.
While not consistent with our hypothesis, this is a far-reaching finding. The fact that engagement was not able to mediate the effect of social background on educational transitions indicates that social inequality in students’ educational pathways can be explained by structural features rather than school processes such as engagement. Efforts to target engagement to improve students’ educational chances, as van Uden et al. (Citation2014) propose, will thus not be successful when policy makers do not account for structural reproduction of inequality in educational systems. One possible explanation is that disengagement no longer exclusively is a property of lower-class youth, but is beginning to have a generational character, transversal to the different social strata. This fact should be a reason for reflection about educational policies.
As is common for all research, our study has several limitations which should be mentioned. First, although a cross-national longitudinal perspective provides many analytical opportunities, it also leads to some limitations. Because our analyses were limited to three cities, we could not include the country level in the analyses. It was therefore not possible to check whether our results are due to system-specific features or to more general characteristics of the country, but as we contextualize, we can hypothesize about this. Another challenge linked to country differences is that it puts restrictions on our outcome variable, as we had to reduce the number of outcomes to what was comparable across countries. This reduces the complexity we can consider. A third limitation is attrition in the data for Ghent and Barcelona, as some of the students answering the first survey were not present for the follow-up surveys. The Norwegian data do not have this challenge, as we had access to register data for all students completing the first survey. However, the challenge of panel attrition is common in longitudinal data sets. The likely effect of panel attrition is a slight underestimation of dropout in the Spanish and Belgian systems.
Our research raises many questions and options for future research. It would be interesting to further analyze differences in engagement within different post-secondary pathways, and to analyze factors external to the education system, particularly the implications of the relationship between the labor market and vocational training, and how this plays out in various countries.
In sum, our study found that parental educational level and expected grades have the greatest impact on Year 11 and Year 12 destination in student’s pathway through upper secondary education, and both variables are important. Further, the pattern of pathways is quite similar across the three cities for students who have better-educated parents and who are expecting to get good grades, while there are more differences for students at the other end of the scale, those from less educated families or with poorer grades. This may imply that the heterogeneity management model presented by Mons (Citation2007) is more appropriate for describing differences among less resourceful students, than among more resourceful students (see also Dupriez et al., Citation2008). Thus, future research should consider focusing on low resourced students, as students from families with higher education seem to do well in all systems.
Acknowledgments
An earlier version of this paper has been presented at the Transition in Youth (TIY) workshop in Mannheim in September 2018.
Data availability statement
More information on the research network the data derives from can be found here: http://www.iscy.org/
Additional information
Funding
Notes on contributors
Elisabeth Hovdhaugen
Elisabeth Hovdhaugen is a research professor at NIFU, Nordic Institute for Studies in Innovation, Research and Education, and holds a PhD in Sociology from University of Oslo. Her research focuses on students in general, and much of it has centred on completion and retention in upper secondary and higher education.
Isis Vandelannote
Isis Vandelannote, PhD in Sociology, is member of the research group Cultural Diversity: Opportunities and Socialization (CuDOS), at the Department of Sociology at Ghent University (Belgium). Her research scope of interest includes social inequality in education, with particular attention for educational transitions, tracking and school effects.
Rafael Merino Pareja
Rafael Merino, lecturer at the Department of Sociology, Autonomus University of Barcelona. He is the coordinator of Research Group of Education and Work, his research focuses on transition from school to work, vocational training and educational policy. He was the coordinator of the International Study of City Youth in Spain.
Jannick Demanet
Jannick Demanet, PhD in Sociology, is associate professor at the Department of Sociology, research team CuDOS, Ghent University, Belgium. He studies contextual effects on anti-school attitudes and behavior, grade retention and higher education outcomes. He is vice-coordinator of RN 10 (Sociology of Education) of the European Sociological Association (ESA).
Notes
1 The questionnaire was constructed in 2011, thus using the ISCED 1997 classification of educational levels.
2 Example of equations used:
3 Mood (Citation2010) recommended using this way of handling interactions, as regular interactions might be problematic in logistic regression analyses.
4 The same type of model was run on Year12, rendering the same results. Output is not shown in paper.
References
- Allmendinger, J. (1989). Educational systems and labor market outcomes. European Sociological Review, 5(3), 231–250. https://doi.org/https://doi.org/10.1093/oxfordjournals.esr.a036524
- Baron, R. M., & Kenny, D. A. (1986). The moderator–mediator variable distinction in social psychological research: Conceptual, strategic, and statistical considerations. Journal of Personality and Social Psychology, 51(6), 1173–1182.
- Bernardi, F. (2012). Unequal transitions: Selection bias and the compensatory effect of social background in educational careers. Research in Social Stratification and Mobility, 30(2), 159–174. https://doi.org/https://doi.org/10.1016/j.rssm.2011.05.005
- Bernardi, F. (2014). Compensatory advantage as a mechanism of educational inequality: A regression discontinuity based on month of birth. Sociology of Education, 87(2), 74–88. https://doi.org/https://doi.org/10.1177/0038040714524258
- Bernardi, F., & Cebolla-Boado, H. (2014). Previous school results and social background: Compensation and imperfect information in educational transitions. European Sociological Review, 30(2), 207–217. https://doi.org/https://doi.org/10.1093/esr/jct029
- Bol, T., Witschge, J., Van de Werfhorst, H. G., & Dronkers, J. (2014). Curricular tracking and central examinations: Counterbalancing the impact of social background on student achievement in 36 countries. Social Forces, 92(4), 1545–1572. https://doi.org/https://doi.org/10.1093/sf/sou003
- Bonvin, P., Bless, G., & Schuepbach, M. (2008). Grade retention: Decision-making and effects on learning as well as social and emotional development. School Effectiveness and School Improvement, 19(1), 1–19.
- Boone, S., & Van Houtte, M. (2013a). In search of the mechanisms conducive to class differentials in educational choice: A mixed method research. The Sociological Review, 61(3), 549–572. https://doi.org/https://doi.org/10.1111/1467-954X.12031
- Boone, S., & Van Houtte, M. (2013b). Why are teacher recommendations at the transition from primary to secondary education socially biased? A mixed-methods research. British Journal of Sociology of Education, 34(1), 20–38. https://doi.org/https://doi.org/10.1080/01425692.2012.704720
- Boudon, R. (1974). Education, opportunity, and social inequality: Changing prospects in western society. Wiley.
- Bourdieu, P., & Passeron, J.-C. (1990). Reproduction in education, society and culture. Sage.
- Breen, R., & Jonsson, J. O. (2000). Analyzing educational careers: A multinomial transition model. American Sociological Review, 65(5), 754–772. https://doi.org/https://doi.org/10.2307/2657545
- Breen, R., & Jonsson, J. O. (2005). Inequality of opportunity in comparative perspective: Recent research on educational attainment and social mobility. Annual Review of Sociology, 31(1), 223–243. https://doi.org/https://doi.org/10.1146/annurev.soc.31.041304.122232
- Demanet, J., Van den Broeck, L., & Van Houtte, M. (2019). Socioeconomic school composition and school misconduct: Disentangling structural and cultural explanations in Ghent. In J. Demanet & M. Van Houtte (Eds.), Resisting education: A cross-national study on systems and school effects (pp. 135–153). Springer.
- Demanet, J., & Van Houtte, M. (2013). Grade retention and its association with school misconduct in adolescence: A multilevel approach. School Effectiveness and School Improvement, 24(4), 417–434. https://doi.org/https://doi.org/10.1080/09243453.2012.727834
- Demanet, J., & Van Houtte, M. (2014). Social–ethnic school composition and disengagement: An inquiry into the perceived control explanation. The Social Science Journal, 51(4), 659–675. https://doi.org/https://doi.org/10.1016/j.soscij.2014.09.001
- Demanet, J., & Van Houtte, M. (2016). Are flunkers social outcasts? A multilevel study of grade retention effects on same-grade friendships. American Educational Research Journal, 53(3), 745–780. https://doi.org/https://doi.org/10.3102/0002831216646867
- Dupriez, V., & Dumay, X. (2006). Inequalities in school systems: Effect of school structure or of society structure? Comparative Education, 42(2), 243–260. https://doi.org/https://doi.org/10.1080/03050060600628074
- Dupriez, V., Dumay, X., & Vause, A. (2008). How do school systems manage pupils’ heterogeneity? Comparative Education Review, 52(2), 245–273. https://doi.org/https://doi.org/10.1086/528764
- Fredricks, J. A., Blumenfeld, P. C., & Paris, A. H. (2004). School engagement: Potential of the concept, state of the evidence. Review of Educational Research, 74(1), 59–109. https://doi.org/https://doi.org/10.3102/00346543074001059
- Gambetta, D. (1987). Were they pushed or did they jump? Individual decision mechanisms in education. Cambridge University Press.
- Gamoran, A. (2009). Tracking and inequality: New directions for research and practice (WCER Working Paper No. 2009-6). University of Wisconsin–Madison, Wisconsin Center for Education Research.
- Gamoran, A., & Mare, R. D. (1989). Secondary school tracking and educational inequality: Compensation, reinforcement, or neutrality? American Journal of Sociology, 94(5), 1146–1183. https://doi.org/https://doi.org/10.1086/229114
- Goos, M., Van Damme, J., Onghena, P., Petry, K., & de Bilde, J. (2013). First-grade retention in the Flemish educational context: Effects on children's academic growth, psychosocial growth, and school career throughout primary education. Journal of School Psychology, 51(3), 323–347.
- Hanushek, E. A., & W Ößmann, L. (2006). Does educational tracking affect performance and inequality? Differences‐in‐differences evidence across countries. The Economic Journal, 116(510), C63–C76. https://doi.org/https://doi.org/10.1111/j.1468-0297.2006.01076.x
- Iannelli, C., & Smyth, E. (2008). Mapping gender and social background differences in education and youth transitions across Europe. Journal of Youth Studies, 11(2), 213–232. https://doi.org/https://doi.org/10.1080/13676260701863421
- Imsen, G., & Volckmar, N. (2014). The Norwegian school for all: Historical emergence and neoliberal confrontation. In The Nordic education model (pp. 35–55). Springer.
- Janmaat, J. G., & Mons, N. (2011). Promoting ethnic tolerance and patriotism: The role of education system characteristics. Comparative Education Review, 55(1), 056–081. https://doi.org/https://doi.org/10.1086/657105
- Janosz, M., Archambault, I., Morizot, J., & Pagani, L. S. (2008). School engagement trajectories and their differential predictive relations to dropout. Journal of Social Issues, 64(1), 21–40. https://doi.org/https://doi.org/10.1111/j.1540-4560.2008.00546.x
- Jimerson, S. R. (2001). Meta-analysis of grade retention research: Implications for practice in the 21st century. School Psychology Review, 30(3), 420–437. https://doi.org/https://doi.org/10.1080/02796015.2001.12086124
- Jimerson, S. R., Anderson, G. E., & Whipple, A. D. (2002). Winning the battle and losing the war: Examining the relation between grade retention and dropping out of high school. Psychology in the Schools, 39(4), 441–457. https://doi.org/https://doi.org/10.1002/pits.10046
- Jimerson, S. R., & Ferguson, P. (2007). A longitudinal study of grade retention: Academic and behavioral outcomes of retained students through adolescence. School Psychology Quarterly, 22(3), 314–339. https://doi.org/https://doi.org/10.1037/1045-3830.22.3.314
- Jimerson, S., Carlson, E., Rotert, M., Egeland, B., & Sroufe, L. A. (1997). A prospective, longitudinal study of the correlates and consequences of early grade retention. Journal of School Psychology, 35(1), 3–25.
- Jussim, L., & Harber, K. (2005). Teacher expectations and self-fulfilling prophecies: Knowns and unknowns, resolved and unresolved controversies. Personality and Social Psychology Review, 9(2), 131–155. https://doi.org/https://doi.org/10.1207/s15327957pspr0902_3
- Karlson, K. B., Holm, A., & Breen, R. (2012). Comparing regression coefficients between same-sample nested models using logit and probit: A new method. Sociological Methodology, 42(1), 286–313. https://doi.org/https://doi.org/10.1177/0081175012444861
- Klem, A. M., & Connell, J. P. (2004). Relationships matter: Linking teacher support to student engagement and achievement. The Journal of School Health, 74(7), 262–273.
- Lamote, C., Pinxten, M., Van Den Noortgate, W., & Van Damme, J. (2014). Is the cure worse than the disease? A longitudinal study on the effect of grade retention in secondary education on achievement and academic self-concept. Educational Studies, 40(5), 496–514. https://doi.org/https://doi.org/10.1080/03055698.2014.936828
- Lavrijsen, J. (2013). Characteristics of educational systems. How they influence outcomes in the short and the long run. Steunpunt SSL. rapport nr. SSL_2012.04/1.1.1.
- LeTendre, G. K., Hofer, B. K., & Shimizu, H. (2003). What is tracking? Cultural expectations in the United States, Germany, and Japan. American Educational Research Journal, 40(1), 43–89. https://doi.org/https://doi.org/10.3102/00028312040001043
- Long, J. S., & Freese, J. (2006). Regression models for categorical dependent variables using Stata. Stata Press.
- Lucas, S. R. (2001). Effectively maintained inequality: Education transitions, track mobility, and social background effects. American Journal of Sociology, 106(6), 1642–1690. https://doi.org/https://doi.org/10.1086/321300
- Mare, R. D. (1980). Social background and school continuation decisions. Journal of the American Statistical Association, 75(370), 295–305. https://doi.org/https://doi.org/10.1080/01621459.1980.10477466
- Martin, A. J. (2011). Holding back and holding behind: Grade retention and students’ non‐academic and academic outcomes. British Educational Research Journal, 37(5), 739–763. https://doi.org/https://doi.org/10.1080/01411926.2010.490874
- Mons, N. (2007). Les nouvelles politiques éducatives: La France fait-elle les bons choix?. Presses universitaires de France.
- Mood, C. (2010). Logistic regression: Why we cannot do what we think we can do, and what we can do about it. European Sociological Review, 26(1), 67–82. https://doi.org/https://doi.org/10.1093/esr/jcp006
- Perry, L. (2018). Using PISA to examine educational inequality. Orbis Scholae, 2(2), 77–86. https://doi.org/https://doi.org/10.14712/23363177.2018.235
- Régner, I., & Loose, F. (2006). Relationship of sociocultural factors and academic self-esteem to school grades and school disengagement in North African French adolescents. British Journal of Social Psychology, 45(4), 777–797.
- Rumberger, R. W. (1987). High school dropouts: A review of issues and evidence. Review of Educational Research, 57(2), 101–121.
- Rumberger, R. W. (1995). Dropping out of Middle School - a Multilevel Analysis of Students and Schools. American Educational Research Journal, 32(3), 583–625.
- Roeser, R. W., & Eccles, J. S. (1998). Adolescents' perceptions of middle school: Relation to longitudinal changes in academic and psychological adjustment. Journal of Research on Adolescence, 88(1), 123–158.
- Schunk, D. H., & Zimmerman, B. J. (2013). Self-regulation and learning. In W. M. Reynolds, G. E. Miller, & I. B. Weiner (Eds.), Handbook of psychology: Educational psychology (pp. 45–68). John Wiley & Sons, Inc.
- Shavit, Y., & Müller, W. (2000). Vocational secondary education, tracking, and social stratification. In M. T. Hallinan (Ed.), Handbook of the sociology of education (pp. 437–452). Kluwer/Plenum Press.
- Stearns, E., Moller, S., Blau, J., & Potochnick, S. (2007). Staying back and dropping out: The relationship between grade retention and school dropout. Sociology of Education, 80(3), 210–240.
- Steinmayr, R., & Spinath, B. (2009). The importance of motivation as a predictor of school achievement. Learning and Individual Differences, 19(1), 80–90. https://doi.org/https://doi.org/10.1016/j.lindif.2008.05.004
- Stevens, P. A., & Vermeersch, H. (2010). Streaming in Flemish secondary schools: Exploring teachers’ perceptions of and adaptations to students in different streams. Oxford Review of Education, 36(3), 267–284. https://doi.org/https://doi.org/10.1080/03054981003629862
- Thys, S., & Van Houtte, M. (2016). Ethnic composition of the primary school and educational choice: Does the culture of teacher expectations matter? Teaching and Teacher Education, 59, 383–391. https://doi.org/https://doi.org/10.1016/j.tate.2016.06.011
- Van de Werfhorst, H. G., & Mijs, J. J. B. (2010). Achievement inequality and the institutional structure of educational systems: A comparative perspective. Annual Review of Sociology, 36(1), 407–428. https://doi.org/https://doi.org/10.1146/annurev.soc.012809.102538
- van Uden, J. M., Ritzen, H., & Pieters, J. M. (2014). Engaging students: The role of teacher beliefs and interpersonal teacher behaviour in fostering student engagement in vocational education. Teaching and Teacher Education, 37, 21–32. https://doi.org/https://doi.org/10.1016/j.tate.2013.08.005
- You, S., Hong, S., & Ho, H. Z. (2011). Longitudinal effects of perceived control on academic achievement. The Journal of Educational Research, 104(4), 253–266. https://doi.org/https://doi.org/10.1080/00220671003733807