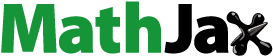
ABSTRACT
In the context of recently emerged pandemic of COVID-19, we have performed two-dimensional quantitative structure-activity relationship (2D-QSAR) modelling using SARS-CoV-3CLpro enzyme inhibitors for the development of a multiple linear regression (MLR) based model. We have used 2D descriptors with an aim to develop an easily interpretable, transferable and reproducible model which may be used for quick prediction of SAR-CoV-3CLpro inhibitory activity for query compounds in the screening process. Based on the insights obtained from the developed 2D-QSAR model, we have identified the structural features responsible for the enhancement of the inhibitory activity against 3CLpro enzyme. Moreover, we have performed the molecular docking analysis using the most and least active molecules from the dataset to understand the molecular interactions involved in binding, and the results were then correlated with the essential structural features obtained from the 2D-QSAR model. Additionally, we have performed in silico predictions of SARS-CoV 3CLpro enzyme inhibitory activity of a total of 50,437 compounds obtained from two anti-viral drug databases (CAS COVID-19 antiviral candidate compound database and another recently reported list of prioritized compounds from the ZINC15 database) using the developed model and provided prioritized compounds for experimental detection of their performance for SARS-CoV 3CLpro enzyme inhibition.
Introduction
Severe acute respiratory syndrome corona virus 2 (SARS-CoV-2) is a positive-sense single stranded RNA virus, informally known as the corona virus [Citation1,Citation2]. The virus was first identified in Wuhan, China by the Centres for Disease Control and Prevention (CDC) in the year of 2019 [Citation2,Citation3]. Since then, the virus has spread to others countries by infected people, and now this is a threat to global health [Citation3,Citation4].The virus spreads from person to person through close contact with someone who has the infection [Citation5]. The disease is most infectious when a person is symptomatic [Citation6]. However it is possible for someone without symptoms to spread the virus [Citation6]. A new study suggests that 10% of infections are from people exhibiting no symptoms [Citation7]. The diagnosed people experience fever, cough, potential loss of taste or smell and trouble breathing [Citation8]. However, many of people with COVID-19 recover with proper care and treatment [Citation6]. It may be noted that SARS-CoV-2 is a new virus and many researches are still ongoing; therefore, it may possible that coronavirus can spread in many other ways that are still unknown. According to the report of WHO (World Health Organization), the two groups of people most at risk of experiencing severe illness due to a SARS-CoV-2 infection are older adults, defined as over 60 years old and persons with other health issues that compromise their immune system [Citation6,Citation7]. Currently there is no vaccine available for the complete treatment of this disease. So there is an urgent need to develop a new effective treatment strategy for the proper and complete treatment of this disease. Viral protease inhibitors are extremely effective at blocking the replication of viruses [Citation9,Citation10]. In this context, 3C-like protease (3CLpro) has become one of the most important SARS-CoV-2 protein targets due to its essential role in processing of replicase polypeptides 1a and 1ab into functional proteins, playing an important role in viral replication [Citation11–Citation14]. Thus, 3CLpro enzyme can be considered as an attractive target for developing effective inhibitors against SARS-CoV-2. In addition, 3CLpro protease of SARS-CoV-2 shares over 95% of sequence similarity with that of SARS-CoV [Citation4]. Thus, the latter may be used as the surrogate enzyme for development of inhibitors against SAR-CoV-2 3CLpro.
In the current state, computational biology and chemoinformatics have established their great prospective in designing leads for complex diseases. Currently, these in silico methodologies are playing an important role in the drug discovery process, and they are usually implemented in the search of novel drugs or for the optimization of therapeutic activity (or pharmacokinetic properties) of chemical series [Citation15]. Among these methodologies, quantitative structure-activity relationship (QSAR) and molecular docking have great applications in the field of in silico search. In the present report, we have developed a multiple linear regression (MLR) based 2D-QSAR model using 3CLpro enzyme inhibitors for the search of significant structural features for the inhibition of 3CLpro in SARS-CoV. We have used 2D descriptors due to their ease and speed of calculation, simplicity and reproducibility as they do not need exhaustive computation involving conformer generation and energy minimization. Moreover, the developed model is expected to be easily used by other groups of researchers for the screening purposes. Prior to the development of the final model, we have performed the multilayer variable section strategy as discussed in the next section, to extract the important descriptors for the bioactivity. The results of the validated model showed acceptable values for various internal and external validation metrics. The model was developed by following the OECD (Organization for Economic Co-operation and Development) guidelines for QSAR model development [Citation16]. The obtained model suggested important structural requirements or molecular properties necessary to design effective inhibitors. The study has identified the structural attributes in small molecules that confer 3CLpro enzyme inhibitory activities and their correlation to ligand-receptor interactions for further development of new generation drugs for SARS-CoV infections. Additionally, we have also performed the molecular docking studies with the most and least active compounds from the dataset and tried to validate the contributions of different descriptors/features as evident from the 2D-QSAR model. Moreover, we have also performed virtual screening, using the developed model, of two different databases (the details are discussed in the next sections) containing a total of 50,437 compounds in search of novel inhibitors against 3CLpro enzyme for the treatment of SARS-CoV infections.
Materials and methods
The details of the methodologies adopted in this study are discussed below and depicted in .
Figure 1. Schematic work flow for the methodologies adopted in this study. MLR = Multiple linear regression, SR = Stepwise regression, BSS = Best subset selection, GAs = Genetic algorithms.
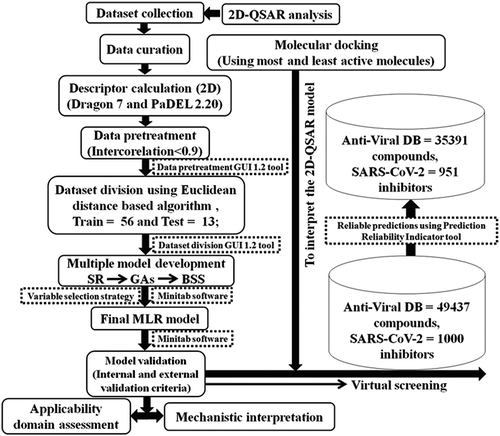
2D-QSAR analysis
Dataset selection
In present work, we have collected a set of 69 molecules (see supplementary information S1 sheet 1) as 3C-like Protease inhibitors against infectious bronchitis virus (SARS-CoV virus) from the binding database [Citation17] reported by the ‘The Scripps Research Institute Molecular Screening Center’. It may be noted that we omitted 16 compounds present in the original source (85 compounds) due to their outlier behaviour in the initial modelling exercises (see sheet 2 in Supplementary information S2). However, we have later used the developed model for the prediction of these omitted 16 compounds to show the applicability of the model on the omitted compounds. The used dataset comprises diverse classes of heterocyclic compounds, and the experimental activity of each compound is expressed in IC50 (nM) values, derived following the same bioassay protocol (QFRET-based dose response biochemical high throughput screening assay) [Citation18]. For the purpose of the model development, we have converted the IC50values to pIC50 (pIC50 = -logIC50) values. All the compounds were drawn using MarvinSketch [Citation19], followed by cleaning of molecules. Then, hydrogen was added and the file saved in.sdf format. Prior to descriptor calculation, we have carefully checked all structures in the dataset for development of significant 2D-QSAR model.
Descriptor calculation and pretreatment
We have computed only 2D descriptors using two software, namely Dragon 7 [Citation20] (covering functional group counts, constitutional, topological indices, ring descriptors, connectivity index, atom centred fragments, 2D atom pairs, atom type E-states and molecular properties) and PaDEL-Descriptor 2.20 [Citation21] (for extended topochemical atom indices). After descriptor calculation, we have implemented data pretreatment utilizing the tool Pretreatment V-WSP version 1.2 (available at http://dtclab.webs.com/software-tools) in order to remove the descriptors with at least one missing value or near constant values.
Dataset division
In the current analysis, we have divided the whole dataset into training and test sets based on the Euclidean distance based algorithm using the ‘Dataset Division GUI’ developed by our group (available at http://dtclab.webs.com/software-tools). The 2D-QSAR model was developed by the training set and validated using new chemical entities, i.e. the test set to check the predictive quality of the developed model.
Multi-layered variable selection and 2D QSAR model development
To identify the significant descriptors from the large pool of initial descriptors using different variable selection methodologies is an important step in QSAR modelling. Based on this concept, we have implemented multi-layered variable selection strategy prior to development of the final model. In the multi-layered variable selection, first we have applied stepwise regression in successive iterations using Minitab software [Citation22] followed by genetic algorithm (GA) using GA software (available at http://dtclab.webs.com/software-tools), and afterwards we have applied best subset selection (available at http://dtclab.webs.com/software-tools), and the final model was built using multiple linear regression (MLR) technique. The detailed multi-layered variable selection strategy is depicted in Figure S1 (see supplementary information S3).
Statistical validation criteria of the developed model
Statistical validation is an important step in QSAR modelling to judge the robustness and predictive quality of the developed models. In this investigation, we have applied various statistical methodologies like internal and external validation methods to assure the significant quality of the developed model. In case of internal validation, we have determined different statistical metrics such as determination coefficient (r2), leave-one-out cross-validated correlation coefficient (Q2(LOO)), Avg rm2(LOO) and Δrm2 values [Citation23]. Higher values of the metrics r2, Q2(LOO) and Avg rm2(LOO) indicate a better fit of the model, but all these parameters are not appropriate to evaluate the robustness and predictivity of a significant model [Citation23]. Thus, we have determined other statistical validation parameters (external validation parameters), like Q2F1, Q2F2, rm2 parameters like average rm2 (test) and Δrm2 and concordance correlation coefficient (CCC) to assure the significance of the developed models [Citation23]. Furthermore, we have also performed Y-randomization test, checked applicability domain criteria etc. to examine the robustness of developed model. The Y-randomization test was performed using the MLRPlusValidation1.3 tool (available at http://dtclab.webs.com/software-tools) through randomly reordering (100 permutations) the dependent variable [Citation24].
Virtual screening and activity prediction of unknown compound database
It is time consuming and expensive to experimentally determine various biological/chemical/toxicological endpoint values of all possible compounds in the large chemical space. In this regard, we must have some computational tools to derive prediction data for the real or hypothetical compounds for possible screening purposes, and we should have some measures to estimate the reliability of predictions. This will help us in prioritizing potential compounds for costly experiments. There are several tools reported to provide measures of confidence estimates of QSAR predictions. Among them, one of the reliable tools for the prediction of response data for an external set is ‘Prediction Reliability Indicator’ tool [Citation25] available at http://dtclab.webs.com/software-tools. The tool was developed to categorize the quality of predictions for the test set or external based on the three rules, Rule 1: Scoring based on the quality of leave-one-out predictions of closest 10 training compounds to a test/external compound, Rule 2: Scoring based on the similarity-based AD using the standardization method and Rule 3: Scoring based on the proximity of predictions to the training set observed/experimental response mean [Citation25]. On the basis of composite score tool, the predictions for the external compounds are classified in to three groups: good (with composite score 3), moderate (with composite score 2) and bad (with composite score 1) [Citation25,Citation26]. In this research, we have screened two databases: the first dataset has been reported by the Ton et al., very recently in 2020 [Citation27], and composed of 1000 screened compounds derived by the Deep Docking protocol applied on the ZINC15 database; the second dataset of 49,437 compounds was downloaded from the Chemical Abstracts Service (CAS) [Citation28] reported as COVID-19 antiviral candidate compounds for the identification novel 3C-like Protease inhibitors. We have screened and predicted the activities of the compounds of the mentioned two databases, with consideration of applicability domain of our MLR based 2D-QSAR model, applying the Prediction Reliability Indicator tool developed by our group (available at http://dtclab.webs.com/software-tools).
Molecular docking analysis
In this investigation, we have applied molecular docking studies to investigate the interaction pattern of molecules (most and least actives from the dataset) with their respective enzyme (3C-like Protease). The structure of the enzyme was retrieved from the protein databank with the PDB id: 6lu7 (crystal structure of COVID-19 main protease in complex with an inhibitor N3) [Citation29]. The molecular docking study was performed by using Autodock tool 1.5.6 [Citation30] platform as discussed by the Rizvi et al. [Citation31] in 2013. Prior to the docking, we have prepared the target enzyme and selected inhibitors using the protein and ligand preparation protocol available in Autodock tool 1.5.6 [Citation30]. The active site in the enzyme was defined by the providing explicit coordinates of active amino acids residues obtained from the co-crystal ligand in the enzyme using PDBsum web server [Citation32]. The size and the exact position of the grid was adjusted by providing the coordinates using the protocol ‘Grid preparation’ available in Autodock tool 1.5.6 [Citation30]. After receptor and ligand preparation and binding site definition, docking runs were launched from the command line. In the docking analysis, we have sorted the generated poses as per binding interaction energy, and the top scoring poses (most negative) were kept for further analysis. The obtained poses were validated using the bound ligand present in the crystal structure of the enzyme. On the basis of number of interactions and active residues interacting with the bound ligand, we have selected the final pose for the further study. From the ligplot (See Figure S2 in supplementary information S3), we can see the number of interactions and active residues responsible for the significant interaction in the crystal structure of COVID-19 main protease with the bound ligand.
Results and discussion
2D-QSAR analysis
In this investigation, we have developed a MLR based 2D-QSAR model for the identification of important structural features responsible for the inhibition of 3C-like protease (3CLpro) enzyme in SARS-CoV-2 infections. The model (equation 1) has been developed with 8 significant descriptors. The details of the different validation metric values for the model are summarized in Equation 1. The results obtained from the model recommended that the developed model was acceptable in terms of stability, predictive ability and fitness. The descriptors appearing in the model describe the structural and functional requirements which can improve the inhibitory activity of molecules against 3CLpro enzyme. The scatter plot () defines the closeness of the observed and predicted values for the 3CLpro enzyme inhibitors. Additionally, we have also performed the Y-Randomization test using the MLRPlusValidation1.3 tool (available at http://dtclab.webs.com/software-tools) to make sure whether the model was obtained by any chance or not. The results obtained from randomized models (Avg. r2 = 0.155 and Avg. Q2 = −0.240) suggested that the developed model was not obtained by any chance correlation as shown in Sheet 1 (see supplementary information S4). We have also predicted the set of 16 initially omitted molecules using our developed model, and the results are shown in Supplementary Information S2. The absolute predicted residual of all compounds except one is less than 1 log unit. Thus, the model will not misclassify (active/inactive) these compounds even if the predictions are not very precise. The MAE (predictions) for these compounds is also not very high (0.432) suggesting the applicability of our model for these compounds. The observed and predicted values of these compounds are given in Sheet 2 of Supplementary Information S2).
Figure 2. Scatter plot of observed and predicted values from the developed MLR model against 3CLpro enzyme.
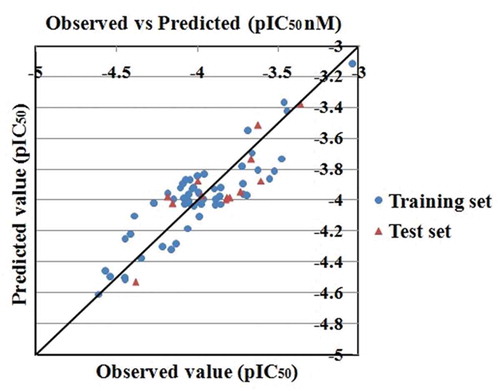
2D-QSAR model and its statistical validation parameters obtained from the developed model against 3CLpro enzyme (Eq. 1).
Internal validation parameters:
,
,
, EL = 8
External validation parameters:
ntest, Q2F1
, Q2F2
, Avgrm2 = 0.610, Δrm2 = 0.110, MAE = 0.127.
Mechanistic interpretation of modelled descriptors obtained from the developed 2D-QSAR model against 3CLpro enzyme
Here we have discussed the importance of each descriptor against 3CLpro enzyme and analysed them with their appropriate examples.
The descriptor F06[O-S] is the most important feature contributing positively to the response in the model. It belongs to the 2D atom pair descriptor family and represents the frequency of O–S at the topological distance 6 in the molecules. The positive contribution of this descriptor indicates that the compounds containing higher number of O–S fragments at the topological distance 6 show higher 3CLpro enzyme inhibitory activity as evidenced by () compounds #1 (pIC50: −3.035), #4 (pIC50: −3.446) and #5 (pIC50: −3.463) (their corresponding descriptors values are 2, 1 and 1 respectively). On the other hand, molecules which do not contain such feature have lower 3CLpro enzyme inhibitory activity as shown in () compounds #84 (pIC50: −4.607), #83 (pIC50: −4.565) and #81 (pIC50: −4.534).
The functional group count descriptor, nPyridines, describes the number of pyridine rings present in the compounds. The negative contribution of this descriptor indicates that the presence of pyridine rings in the compounds may lower the inhibitory activity against 3CLpro enzyme as found in () compounds #55 (pIC50: −4.080), #61 (pIC50: −4.159) and #76 (pIC50: −4.409) (containing 1 pyridine ring in each case), whereas the absence of such fragment in the molecules shows higher inhibitory activity as observed in case of () compounds #1 (pIC50: −3.035), #4 (pIC50: −3.446) and #5 (pIC50: −3.463).
Another functional group count descriptor, nThiophenes, describes the number of thiophene rings present in the compounds. The therapeutic role of the thiophene ring and their derivatives in drug design and synthesis of pharmacologically important molecules have been discussed by Shah and Verma [Citation33] and Kamboj and Randhawa [Citation34] in their review articles. In the present context, this feature interacts with the enzyme through π- interactions as discussed in docking analysis portion (discussed later). This descriptor contributes positively towards the 3CLpro enzyme inhibitory activity in the model. Thus, the molecules bearing higher number of thiophene rings may have higher 3CLpro enzyme inhibitory activity as shown in () compounds #21 (pIC50: −3.717), #4 (pIC50: −3.446) and #5 (pIC50: −3.463) (containing descriptor values 2, 1 and 1 respectively), whereas in the contrary, compounds #84 (pIC50: −4.607), #83 (pIC50: −4.565) and #81 (pIC50: −4.534) which do not contain any such fragment showed less 3CLpro enzyme inhibitory activity. From this observation, it can be concluded that the number of thiophene rings should be higher for better inhibitory activity against 3CLpro enzyme.
The topological descriptor, PW4or path/walk 4 – Randic shape index of molecules, can be considered as a shape descriptor. Path/walk Randic shape indices (PWk) are calculated by summing the ratios of the atomic path count over the atomic walk count of the same order k and then dividing by the number of non-H atoms [Citation35]. The shape of compounds plays an important role in protein ligand interactions to fit in the binding cavity of target protein. The positive contribution of this descriptor suggests that the higher values of molecular topology and symmetry accounting Randic shape index descriptor PW4 (path/walk 4) are favourable for 3CLpro enzyme inhibitory activity as evidenced by () the compounds #33 (pIC50: −3.89), #8 (pIC50: −3.55) and #5 (pIC50: −3.03) (containing descriptor values 0.203, 0.202 and 0.201 respectively), whereas vice versa is observed in case of compounds #65 (pIC50: −4.212), #77 (pIC50: −4.444) and #58 (pIC50: −4.126) (containing descriptor values 0.153, 0.156 and 0.157 respectively),
C-007 is an atom-centred fragment type descriptor representing the fragment CH2X2, which describes the number of methylene groups connected with the highly electronegative atoms like oxygen, nitrogen, sulphur, phosphorus and various halogens [Citation36]. This feature negatively influences the inhibitory activity against 3CLpro enzyme as suggested by the developed model, which indicates that this feature does not enhance the 3CLpro enzyme inhibitory activity of molecules as found in compounds () #84 (pIC50: −4.607) (containing descriptor values 1). On the hand, the compounds which do not contain such feature have higher 3CLpro enzyme inhibitory activity as found in compounds () #1 (pIC50: −3.035), #4 (pIC50: −3.446) and #5 (pIC50: −3.463).
Another functional group count descriptor, nN(CO)2, describes the number of imides (including thioimides) group present in the compounds. The negative contribution of this descriptor suggests that presence of this group is inversely proportional to the 3CLpro enzyme inhibitory activity as observed in case of compounds () #72 (pIC50:-4.341) and #81 (pIC50:-4.534) (both compounds have descriptor value 2), while the absence of such fragment in the compounds () leads to a higher 3CLpro enzyme inhibitory activity as observed in the compounds #1 (pIC50: −3.035), #4 (pIC50: −3.446) and #5 (pIC50: −3.463).
Another 2D atom pair descriptor, B02[N-O], stands for the presence/absence of the N-O fragment at the topological distance 2. The negative contribution of this descriptor indicates that the presence of the N-O fragment at topological distance 2 may be detrimental for the inhibitory activity against the 3CLpro enzyme as found in () compounds #84 (pIC50: −4.607), #83 (pIC50: −4.565) and #81 (pIC50: −4.534) (containing descriptor value of 1 for all the cases). On the other hand, compounds with absence of such fragment show higher inhibitory activity as observed in () compounds #6 (pIC50: −3.471), #7 (pIC50: −3.523) and #14 (pIC50: −3.661).
Another topological descriptor, LOC, is simply characterized by the lopping centric index in the compounds. The positive contribution of this descriptor suggests that a higher value of the LOC descriptor is favourable for the inhibitory activity against the 3CLpro enzyme as evidenced by the () compounds #4 (pIC50: −3.446), #5 (pIC50: −3.463) and #14 (pIC50: −3.661) (containing descriptor values 1.28, 1.07 and 1.28 respectively). On the other hand, the compounds () with lower descriptor values such as #81 (pIC50: −4.534) and #68 (pIC50: −4.267) (containing descriptor values 0.548 in both cases) show lower inhibitory activity against the 3CLpro enzyme. From the analysis, it was observed that the long chain acyclic compounds show higher values for the descriptor and in the same way higher values for the endpoint.
Applicability domain criteria for the validation dataset
In addition we have also performed the applicability domain (AD) study using the standardization approach (MLRPlusValidation1.3 tool available at http://dtclab.webs.com/software-tools) with an objective to define the hypothetical domain in the chemical space for a particular QSAR model, within which the model predictions are considered reliable [Citation16]. From the analysis, we have observed that (see sheet 2 in supplementary information S4), there is no compound in the test set located outside the AD.
Molecular docking analysis
In the current investigation, we have performed the molecular docking studies using the most and least active compounds from the dataset. We have taken the three most active compounds, i.e. #1, #4 and #5 and two least active compounds #83 and #84 from the dataset to identify the molecular interactions with the active site of 3CLpro enzyme. The details of docking interaction, binding score and their relation with the features obtained from the developed 2D-QSAR model are depicted in . Here, we have discussed the details of docking interactions with the active residues of the enzyme below.
Table 1. Docking results and correlation with 2D-QSAR model against 3CLpro enzyme.
Molecular docking analysis of the most active compounds from the dataset
In this analysis, three most active compounds (#1, #4 and #5) from the dataset (pIC50 = −3.035, −3.446 and – 3.463 respectively) interacted with the active site amino acid residues, i.e. CYS A:145, MET A: 49, GLU A: 166, LEU A: 141, LEU A: 167, GLN A: 192, GLN A: 189, THR A: 190, MET A: 165 and PRO A: 168 through interacting forces like hydrogen bonding (conventional and carbon hydrogen bonds), π-bonding (π-alkyl, π-anion, π-cation, π-sulphur, amide-π-stacked), alkyl, and halogen interactions.
One of the most active compounds from the dataset, compound #1 (), interacts with the active site amino acid residues of the enzyme through hydrogen bonding (GLU A: 166, LEU A: 141), π-cation (HIS A: 41), π-anion (GLU A: 166), π-sulphur (CYS A: 145), Alkyl bond (MET A: 49 and CYS A: 145) and π-alkyl (MET A: 49 and HIS A: 41) interactions.
Figure 6. Docking interactions of the most (Compound #1) and least (Compound #84) active compounds from the dataset of 3CLpro enzyme inhibitors.
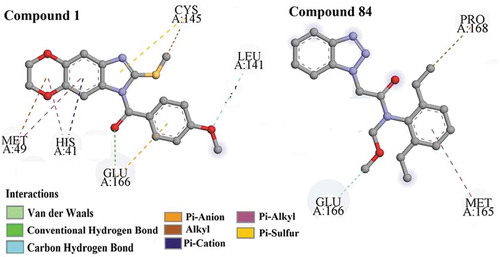
The next most active compound from the dataset, compound #4 (see Figure S3 in supplementary information S3), interacts with active site amino acid residues, such as GLU A: 166, GLN A: 189 and GLN A: 192 via hydrogen bonding, MET A: 49 through π-alkyl bonding, GLN A: 189 via amide-π-stacked, MET A: 165 via π-sulphur and THR A: 190 and GLN A: 189 through the halogen atom interaction such as fluorine.
Another most active compound from the dataset, compound #5 (see Figure S4 in supplementary information S3), interacts with the active amino acid residues of the enzyme like GLU A: 166, GLN A: 189 (through hydrogen bonding), LEU A: 167, MET A: 165 and PRO A: 168 (hydrophobic alkyl bond) and PRO A: 168 (through hydrophobic π-alkyl bond).
Molecular docking analysis of the least active compounds from the dataset
In this investigation, two least active compounds (compounds #83 and #84) from the dataset (pIC50 = −4.565 and −4.607 respectively) interacted with the active site amino acid residues such as CYS A: 145, HIS A: 163, MET A: 165, THR A: 190, PRO A: 168 and GLU A: 166 through interacting forces like hydrogen bonding (carbon hydrogen bonds), π-bonding (π-alkyl) and alkyl hydrophobic bonding.
One of the least active compounds from the dataset, compound #83 (Figure S5 in supplementary information S3), interacts with amino acid residues like THR A: 190 through carbon hydrogen bonding, HIS A: 163, MET A: 165 through hydrophobic π-alkyl bonds and CYS A: 145 through alkyl bonding.
shows that compound #84, another least active compound from the dataset, interacts with the active amino acid residues of enzyme such as GLU A: 166 via carbon hydrogen bonding, PRO A: 168 via hydrophobic alkyl bonding and MET A: 165 through hydrophobic π-alkyl bonds.
Correlation of docking analysis results with the developed 2D-QSAR model
From the above study, we have concluded that the formation of hydrogen bonding and π-interaction between the ligand and receptor play a crucial role in the interactions. Hydrogen bonding may correlate with the descriptors such as F06[O-S], LOC and PW4 of the developed 2D-QSAR model. The descriptors nThiophenes is well corroborated with interactions formed via π- interactions (amide-π-stacked, π-alkyl π-anion, π-cation and π-sulphur) between the receptor and ligand. All these descriptors contributed positively in the developed model and are essential features for the inhibitory activity against the 3CLpro enzyme. The above mentioned features are observed in most active compounds from the dataset such as #1, #4 and #5 ( and Figure S3 and S4 in supplementary information S3 of docking). In contrast, the descriptors nPyridines, contributed negatively in the 2D-QSAR model, and thus might be detrimental for the inhibitory activity, and this has been observed in the least active compound number #83 (Figure S5 in supplementary information S3 of docking). Thus, from above investigation, we can conclude that features obtained from molecular docking studies and 2D-QSAR model are in agreement, and these are essential for the inhibitory activity against 3CL-pro enzyme.
Application of developed QSAR model for the activity prediction and virtual screening
The developed 2D-QSAR model was used to predict the activity of compounds of two different datasets (as discussed in the methods section above) having no quantitative observed response values in the source files. First, the developed MLR model was used to derive the predicted values of external dataset compounds; the model could predict the activity of most of the compounds with confidence as suggested by ‘Prediction reliability indicator’ tool available at https://sites.google.com/site/dtclabdc. In the second step, we have ranked the screened compounds on the basis of the predicted values (highest to lowest). In case of the dataset of 1000 screened compounds reported by the Ton et al. [Citation27], we have found that 951 compounds are located inside the AD (applicability domain) whereas only 49 compounds found outside the AD of the model. In case of COVID-19 antiviral candidate compound database [Citation28], we have found that 35,391 compounds are located inside the AD and 14,046 compounds fall outside the AD, out of the listed 49,437 compounds. The list of top 100 compounds with significant predicted inhibitory activity is listed in Table S1 (See supplementary information S3). The details of external set evaluations are reported in sheets 1 and 2 (see supplementary information S5).
Conclusion and future prospective
In the current search, we have applied chemoinformatic tools to investigate a dataset of 69 heterocyclic molecules [Citation17] with defined 3C-like protease enzyme inhibitory activity, in order to discover the significant structural requirements essential for enzyme inhibition. Prior to modelling, we have computed simple, meaningful and easily interpretable 2D descriptors for the purpose of development of an easily reproducible and transferable model which can be used for prediction and screening of external compounds. Before the development of the final model, we have applied a multilayered variable selection strategy using stepwise regression using Minitab software [Citation22] followed by genetic algorithm (GA) (available at http://dtclab.webs.com/software-tools) and afterwards best subset selection (available at http://dtclab.webs.com/software-tools). The final model was built by using MLR based technique following OECD guidelines. The statistical validation results obtained from the developed model showed good predictive ability on the basis of both internal and external validation matrices. Based on the observations obtained from developed MLR model, we have concluded that frequency of O – S at the topological distance 6, number of thiophene rings, shape of compounds and presence of a long acyclic chain in the molecules may be more favourable for the inhibitory activity against 3CLpro enzyme. On the other hand, the number of pyridine rings, presence of CH2X2 fragment, number of imides (including thioimides) and presence of the N-O fragment at the topological distance 2 are detrimental to the 3CLpro enzyme inhibitory activity. Due to simple and interpretable nature of the developed model, this can be readily and quickly used for screening of any antiviral databases. Additionally, molecular docking analysis has been performed to understand the interactions between the target protein (COVID-19 main protease enzyme) and inhibitors in this dataset, and the results showed the active compounds (compounds #1, #4 and #5) formed hydrogen bond and hydrophobic π interactions with amino acid residues in the active binding site of the target protein. Furthermore, the results obtained from molecular docking studies well supported the 2D-QSAR analysis results. Moreover, virtual screening has been performed to identify the novel 3CLpro enzyme inhibitors with the developed model using the two different anti-viral drug databases containing a total of 50,437 compounds for the treatment of SARS-CoV-2 infections. From the search, we have identified the 36,342 inhibitors that are located inside the applicability domain of the model. Finally we have concluded that the validated model may be helpful for prediction of the inhibitory activity of new analogues against the 3CLpro enzyme, and the features obtained from the 2D-QSAR analysis and molecular docking studies can be useful for the design of novel inhibitors. The list of prioritized inhibitors obtained from the virtual screening can be used for the further development of novel inhibitors.
S5_Supplementary_information_S4_Prediction_score.xlsx
Download MS Excel (5.7 MB)S4_Supplementary_information_S4_AD_and_Rand_test.xlsx
Download MS Excel (16.2 KB)S3_Supplementary_Information_Figures_and_Table.docx
Download MS Word (3.1 MB)S2_Supplementary_Information_Descriptors_and_omitted_compounds.xlsx
Download MS Excel (35.3 KB)S1_Supplementary_Information_Molecules.xlsx
Download MS Excel (11.3 KB)Acknowledgements
Financial assistance from the Indian Council of Medical Research (ICMR), New Delhi in the form of a senior research fellowship (File No: 5/3/8/27/ITR-F/2018-ITR; dated: 18.05.2018) to VK is thankfully acknowledged. KR thanks SERB, Government of India for financial assistance under the MATRICS scheme (MTR/2019/000008).
Disclosure statement
No potential conflict of interest was reported by the authors.
Supplementary material
Supplemental data for this article can be accessed at: https://doi.org/10.1080/1062936X.2020.1776388.
Additional information
Funding
References
- D. Leslie, P.F. Horve, D.A. Coil, M. Fretz, J.A. Eisen, and K.V. Den Wymelenberg, 2019 novel coronavirus (COVID-19) pandemic: Built environment considerations to reduce transmission, Msystems 5 (2020), pp. 245–20. doi:10.1128/mSystems.00245-20
- D. Kumar, R. Malviya, and P.K. Sharma, Corona virus: A review of COVID-19, EJMO 4 (2020), pp. 8–25. doi:10.14744/ejmo.2020.51418
- D.E. Gordon, G.M. Jang, M. Bouhaddou, J. Xu, K. Obernier, M.J. O’meara, and J.Z. Guo, A SARS-CoV-2-human protein-protein interaction map reveals drug targets and potential drug-repurposing, BioRxiv (2020). doi:10.1101/2020.03.22.002386.
- C. Liu, Q. Zhou, Y. Li, L.V. Garner, S.P. Watkins, L.J. Carter, J. Smoot, and D. Albaiu, Research and development on therapeutic agents and vaccines for COVID-19 and related human coronavirus diseases, ACS Cent. Sci. 6 (2020), pp. 315–331. doi:10.1021/acscentsci.0c00272
- X. Yang, Y. Yu, J. Xu, H. Shu, L. Zhang, L. Zhang, and L. Zhang, Clinical course and outcomes of critically ill patients with SARS-CoV-2 pneumonia in Wuhan, China: A single-centered, retrospective, observational study, Lancet Resp. Med. 8 (2020), pp. 475–481. doi:10.1016/S2213-2600(20)30079-5
- W.X. Xiao, X.X. Wu, X.G. Jiang, K.J. Xu, L.J. Ying, C.L. Ma, and S.B. Li, Clinical findings in a group of patients infected with the 2019 novel coronavirus (SARS-Cov-2) outside of Wuhan, China: Retrospective case series, BMJ 368 (2020), pp. 606. doi:10.1136/bmj.m606
- G. Kampf, D. Todt, S. Pfaender, and E. Steinmann, Persistence of coronaviruses on inanimate surfaces and its inactivation with biocidal agents, J. Hosp. Infect. 104 (2020), pp. 246–251. doi:10.1016/j.jhin.2020.01.022
- J. Shang, G. Ye, K. Shi, Y. Wan, C. Luo, H. Aihara, Q. Geng, A. Auerbach, and F. Li, Structural basis of receptor recognition by SARS-CoV-2, Nature 30 (2020), pp. 1–8. doi:10.1038/s41586-020-2179-y
- W.L. Cheng, F.J. Tsai, C.H. Tsai, C.C. Lai, L. Wan, T.Y. Ho, C.C. Hsieh, and P.D. Lee Chao, Anti-SARS coronavirus 3C-like protease effects of Isatis indigotica root and plant-derived phenolic compounds, Antivir. Res. 68 (2005), pp. 36–42. doi:10.1016/j.antiviral.2005.07.002
- E. Fedorenko, M.K. Behr, and N. Kanwisher, Functional specificity for high-level linguistic processing in the human brain, PNAS 39 (2011), pp. 16428–16433. doi:10.1073/pnas.1601327113
- S. Chen, L.L. Chen, H.B. Luo, T. Sun, J. Chen, F. Ye, J.H. Cai, J.K. Shen, X. Shen, and H.L. Jiang, Enzymatic activity characterization of SARS coronavirus 3C‐like protease by fluorescence resonance energy transfer technique 1, Acta Pharmacol. Sin. 26 (2005), pp. 99–106. doi:10.1111/j.1745-7254.2005.00010.x
- A. Zhavoronkov, V. Aladinskiy, A. Zhebrak, B. Zagribelnyy, V. Terentiev, D.S. Bezrukov, and D. Polykovskiy, Potential COVID-2019 3C-like protease inhibitors designed using generative deep learning approaches, Insilico Med. Hong Kong Ltd A 307 (2020), pp. E1.
- X. Deng, S.E. St John, H.L. Osswald, A. O’Brien, B.S. Banach, K. Sleeman, A.K. Ghosh, A.D. Mesecar, and S.C. Baker, Coronaviruses resistant to a 3C-like protease inhibitor are attenuated for replication and pathogenesis, revealing a low genetic barrier but high fitness cost of resistance, Int. J. Virol. 20 (2014), pp. 11886–11898. doi:10.1128/JVI.01528-14
- R. Ramajayam, K.P. Tan, H.G. Liu, and P.H. Liang, Synthesis and evaluation of pyrazolone compounds as SARS-coronavirus 3C-like protease inhibitors, Bioorg. Med. Chem. 18 (2010), pp. 7849–7854. doi:10.1016/j.bmc.2010.09.050
- S. Ekins, J. Mestres, and B. Testa, In silico pharmacology for drug discovery: Applications to targets and beyond, Br. J. Pharmacol. 152 (2007), pp. 9–20. doi:10.1038/sj.bjp.0707306
- K. Roy, S. Kar, and P. Ambure, On a simple approach for determining applicability domain of QSAR models, Chemom. Intell. Lab. Sys. 145 (2015), pp. 22–29. doi:10.1016/j.chemolab.2015.04.013
- M.K. Gilson, T. Liu, M.G. Baitaluk, G. Nicola, L. Hwang, and J. Chong, BindingDB: A public database for medicinal chemistry, computational chemistry and systems pharmacology, Nucleic Acids Res. 19 (2015), pp. 1045–1053. Available at https://www.bindingdb.org/bind/index.jsp
- J. Jacobs, S. Zhou, E. Dawson, J.S. Daniels, P. Hodder, V. Tokars, A. Mesecar, C.W. Lindsley, and S.R. Stauffer, Discovery of non-covalent inhibitors of the SARS main proteinase 3CLpro.” In Probe Reports from the NIH Molecular Libraries Program [Internet], National Center Biotechnol. Inform. (US). 2013 Feb. 28; Available from https://www.ncbi.nlm.nih.gov/books/NBK133447/
- ChemAxon - Software Solutions and Services for Chemistry & Biology. Available at https://chemaxon.com/products/marvin (accessed December 19, 2019).
- A. Mauri, V. Consonni, M. Pavan, and R. Todeschini, Dragon software: An easy approach to molecular descriptor calculations, Match 2 (2006), pp. 237–248.
- C.W. Yap, PaDEL‐descriptor: An open source software to calculate molecular descriptors and fingerprints, J. Comput. Chem. 32 (2011), pp. 1466–1474. doi:10.1002/jcc.21707
- I.N. Minitab, MINITAB statistical software, Minitab Release. 13 (2000). Available at http://www.minitab.com/en-us/products/minitab/.
- A. Tropsha, Best practices for QSAR model development, validation, and exploitation, Mol. Inform. 29 (2010), pp. 476–488. doi:10.1002/minf.201000061
- R. Veerasamy, H. Rajak, A. Jain, S. Sivadasan, C.P. Varghese, and R.K. Agrawal, Validation of QSAR models-strategies and importance, Int. J. Drug Des. Discov. 3 (2011), pp. 511–519.
- K. Roy, P. Ambure, and S. Kar, How precise are our quantitative structure–activity relationship derived predictions for new query chemicals, ACS Omega 3 (2018), pp. 11392–11406. doi:10.1021/acsomega.8b01647
- K. Khanand and K. Roy, Ecotoxicological QSAR modelling of organic chemicals against Pseudokirchneriella subcapitata using consensus predictions approach, SAR QSAR Environ. Res. 30 (2019), pp. 665–681. doi:10.1080/1062936X.2019.1648315
- T.T. Anh, F. Gentile, M. Hsing, F. Ban, and A. Cherkasov, Rapid identification of potential inhibitors of SARS‐CoV‐2 main protease by deep docking of 1.3 billion compounds, Mol. Inform. (2020). doi:10.1002/minf.202000028.
- COVID-19 antiviral candidates compounds database. Available at https://www.cas.org/covid-19-antiviral-compounds-dataset?utm_source=hootsuite&utm_medium=facebook&utm_term=&utm_content=dbcae960-b771-4732-9e4a-40215709e07a&utm_campaign=COVID-19
- G.M. Alexey, S.E. Brenner, T. Hubbard, and C. Chothia, SCOP: A structural classification of proteins database for the investigation of sequences and structures, J. Mol. Biol. 247 (1995), pp. 40. Available at https://www.rcsb.org/structure/6LU7
- Autodock Vina 1.5.6 tool available at http://autodock.scripps.edu/resources/adt
- S. Rizvi, M. Danish, S. Shakil, and M. Haneef, A simple click by click protocol to perform docking: AutoDock 4.2 made easy for non-bioinformaticians, EXCLIJ. 12 (2013), pp. 831.
- PDBsum available at: http://www.ebi.ac.uk/thornton-srv/databases/cgi-bin/pdbsum/GetPage.pl?pdbcode=2zu4&template=ligands.html&l=1.1
- R. Shah and P.K. Verma, Therapeutic importance of synthetic thiophene, Chem. Cent. J. 12 (2018), pp. 137. doi:10.1186/s13065-018-0511-5
- A. Kamboj and H. Randhawa, Pharmacological action and sar of thiophene derivatives: A review, J. Pharm. Res. 5 (2012), pp. 2676–2682.
- X. Yu, Y. Yu, and Q. Zeng, Support vector machine classification of streptavidin-binding aptamers, PLoS One 9 (2014), pp. e99964. doi:10.1371/journal.pone.0099964
- R. Todeschini and V. Consonni, Molecular Descriptors for Chemoinformatics: Volume I:Alphabetical Listing/volume II: Appendices, References, Vol. 41, John Wiley & Sons, New Jersey, 2009.