Abstract
The Common Core State Standards for Mathematics have a widespread impact on children’s statistical learning opportunities. The Grade 6 standards are particularly ambitious in the goals they set. In this critique, experiences helping children work toward the Grade 6 Common Core statistics expectations are used in conjunction with previous research to identify ways in which the Grades 4–6 standards might be supplemented or revised to help maximize learning. It is suggested that opportunities for children to perceive datasets as aggregates and to draw reasonable conclusions about statistical data by attending to context should be purposefully introduced in Grades 4–5. Currently, the Common Core does not have explicit learning standards for these activities in fourth and fifth grade. It is also suggested that teachers help students question their natural tendencies to focus extensively on the mode when summarizing data. The current standards do not specifically mention the mode. Revising or supplementing the Common Core in the suggested ways holds potential to make the Grade 6 statistical learning standards more attainable for children and to help teachers better anticipate the statistical thinking tendencies that are likely to emerge during classroom discourse.
1 Introduction
Curriculum standards for Grades K-12 prioritize what is most valued and important to teach and learn. Different standards documents reflect different sets of value judgments. For example, documents from different countries reflect disagreement about the amount of emphasis to be put on student-posed statistical questions, probability language, and variability (Groth Citation2018). There can be diversity across standards even within a single country; at the outset of this century, there were substantive differences in the appropriate grade levels and roles for many statistical and mathematical concepts across state standards documents in the United States (Smith Citation2011). Differences across states in the United States led directly to the writing of Curriculum Focal Points (National Council of Teachers of Mathematics [NCTM] 2006), which advocated greater coherence and uniformity among state standards. The Focal Points, in turn, were used to help construct the Common Core State Standards for Mathematics (CCSSM, Common Core State Standards Initiative [CCSSI] 2010) for Grades K-8.
The CCSSM are currently the official learning standards for 41 states, the District of Columbia, four U.S. territories, and the Department of Defense Education Activity (CCSSI 2018). Some states that have rescinded or not adopted the CCSSM nonetheless have standards documents that strongly resemble them (Garland Citation2016; Loewus Citation2015). Hence, the CCSSM exert a sizeable influence in U.S. schools. Given their influence, it is advisable to continuously examine the learning opportunities they provide students and revise or supplement them as needed. When carefully done, such examination and critique can contribute to improved learning opportunities for students. In this article, I offer a constructive critique of the Grades 4–6 statistics standards of the CCSSM by drawing upon my experience conducting research related to this portion of the CCSSM and findings from previous literature. The standards to be considered appear in the Appendix. I argue that, collectively, evidence from these sources suggests that the CCSSM would benefit from revisions to the manner in which they address aggregate distributions, the mode, and statistical contexts.
2 Source of Empirical Data for the Critique
The writing of this critique was motivated by recurring student thinking patterns I observed while leading two rounds of design-based research. Design-based research involves setting learning goals for students, charting a tentative path for them to achieve the goals, designing lessons to help lead students along the path, and continuously analyzing classroom data to revise the path as necessary to be responsive to the thinking students exhibit (Bakker and van Eerde Citation2015; Cobb, Jackson, and Sharpe Citation2017). I used this process on two occasions to guide undergraduate researchers in helping groups of children attain goals set forth in the Grade 6 statistics portion of the CCSSM. On each occasion, the research spanned a 10-week period. During the first week, children were interviewed to help set a viable starting point for instruction. During weeks 2–8, the undergraduates and I worked collaboratively to design weekly lessons for the children. Post-assessment interviews were conducted during the ninth week, followed by retrospective analyses during the 10th. Each interaction with children was video recorded and transcribed, and children’s written work was retained for analysis. This article uses examples of student thinking patterns from the first round of design-based research (Groth Citation2017; Groth, Kent, and Hitch Citation2015) as well as the second round of design-based research to form a constructive critique of the CCSSM.
The undergraduates involved in the research were enrolled in secondary teacher preparation programs. They were charged with implementing the lessons we collaboratively designed. Undergraduates participating in the first round of design-based research described in this report were Rachel and Shantel (pseudonyms). The undergraduates during the second round were Jacob and Olivia (pseudonyms). Each pair of undergraduates worked together over the course of the project to plan, implement, and analyze lessons for groups of four children. The four children in Rachel and Shantel’s group were Jonah, Rhonda, Mary, and Tyrone (pseudonyms). The four children in Jacob and Olivia’s group were Allison, Andrew, Brian, and Claire (pseudonyms). contains background information about the children. In selecting children to participate in the research, we aimed for diversity along the dimensions of race, gender, and schooling experiences. All eight children would enter sixth grade within a month after participating; the research aimed to prepare them for concepts they would soon encounter in their school curricula.
Table 1 Background information about children participating in the research.
3 Aggregate Distributions
3.1 Research on Students’ Thinking About Aggregates
Learning to perceive aggregates in datasets is important because characteristics such as average and skewness belong to aggregate collections rather than individual data points (Konold et al. Citation2015). Describing and analyzing distributions using such aggregate characteristics are essential parts of statistical reasoning (Bakker and Hoffmann Citation2005). Accordingly, the Grade 6 CCSSM expect students to “Understand that a set of data collected to answer a statistical question has a distribution which can be described by its center, spread, and overall shape” and “Recognize that a measure of center for a numerical dataset summarizes all of its values with a single number, while a measure of variation describes how its values vary with a single number” (CCSSI 2010, p. 45).
One robust finding in statistics education research is that it is challenging for beginning learners of statistics to shift their focus from individual data points to aggregate distributions. Aridor and Ben-Zvi (Citation2017) observed, “Young students tend to see data as individual cases and measurement values as inseparable from an object or person measured” (p. 38). At times, the graphical representations children use when asked to display data lend themselves to an individual case view rather than an aggregate view. For example, during the first lesson of the first round of our research, children had the task of displaying the scores they obtained during a game that involved rolling pairs of dice. Mary and Tyrone used one bar to represent each roll rather than grouping similar data values together (). Their use of this sort of graphical display is not unique. During both rounds of research, other students at times used the same approach, and this graphing strategy has also been observed in previous studies (Bakker and Gravemeijer Citation2004; Cobb Citation1999). The graphs shown in illustrate the use of case value bars (Konold Citation2002) to represent data. Although case value bars represent data correctly and support students’ reasoning at times, they can make it difficult to see some distributional features that are more prominent in graphs such as dotplots that aggregate data more compactly.
Fig. 1 Case value bars produced by Mary and Tyrone to represent game scores obtained after rolling a pair of dice 10 times. Mary’s graph indicated that she scored 4 on the first trial, 7 on the second and third, 6 on the forth, etc. Similarly, Tyrone’s graph shows scoring 9 on the first trial, 6 on each of the next three trials, etc.
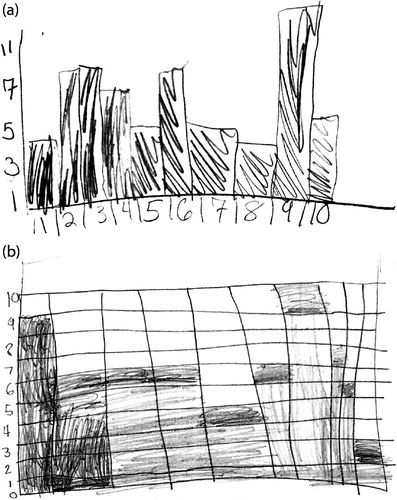
When students do not perceive datasets as aggregates, they lack a basis from which to describe aggregate characteristics such as typical value. During her pre-interview, Claire responded to an item from the National Assessment of Educational Progress (NAEP) asking for the typical number of customers at a bike shop over a 5 day span. Claire examined the data and chose the day with the highest number of customers rather than looking for a value near the middle of the others. Mary, Rhonda, Jonah, and Andrew also at times looked for the highest values when asked to determine typical values. Notably, some of these students were able to produce compact aggregate displays such as dotplots during their interviews. However, they did not automatically see dotplots as useful starting points for the task of describing aggregate features. Instead, they initially used them as tools for looking up individual points such as the greatest value, which Konold et al. (Citation2015) characterized as a case value view of data. Their abilities to produce displays to compactly aggregate data did not automatically translate to the ability to actually perceive aggregates and their features.
During our research, we prioritized helping children adopt more than just a case value approach when reading dotplots. We wanted them to see dotplots as portraits of aggregate distributions rather than just reference tools for looking up and reading individual values. Toward that end, during both rounds of research, we encouraged students to use words such as mountain, hill, peak, hillside, gap, cluster, hole, and outlier to informally describe characteristics of distributions. Doing so helped students think about grouping vertical stacks of data together rather than just looking at individual points and stacks when analyzing dotplots. For example, during one lesson, Brian circled multiple stacks in a given dotplot to indicate they appeared to be a hillside. We gradually focused students’ attention toward clusters of stacks near the centers of data distributions, leading some of them to identify central clusters in data on their own, as Brian did during his post-interview () when asked to describe a distribution as part of an Illustrative Mathematics item showing data about the birth weights of 25 puppies (https://www.illustrativemathematics.org/content-standards/tasks/1026).
Some work remained to be done, however, to help all students associate the central cluster of a distribution with its typical value. Although Brian produced a helpful graph for the puppy weight item and circled its central cluster, he did not refer to the central cluster when asked to identify a typical puppy weight. Instead, he justified his choice of 18 as the typical weight by saying, “18 is the most popular number.” Although 18 is a reasonable estimate of typical value in this situation, we hoped he would justify his choice by talking about how 18 is within the central cluster, and that an estimate such as 17 would also be reasonable because it is still within the cluster. Claire’s reasoning on post-interview items was generally not as developed as Brian’s. She still chose the highest value when asked for the typical number of customers in a bike shop over the course of a week, as she did during pre-interviews. Others, however, did justify their choice of typical value in reference to clusters of data. Allison, for example, justified her choice of median as a good indicator of typical attendance at a movie theater during a week by talking about how more of the data were grouped around the median than the mean.
3.2 Reflections on CCSSM Standards Related to Aggregate Distributions
During both rounds of research, the prospective teachers collaborating with me were surprised at the amount of time it took to help students perceive aggregate features and use them to describe the data. Ideally, the fourth and fifth grade standards would help lead students toward an aggregate view to make sixth grade work more manageable. However, the Grade 4 standard on representing and interpreting data only states that students should:
Make a line plot to display a dataset of measurements in fractions of a unit (1/2, 1/4, 1/8). Solve problems involving addition and subtraction of fractions by using information presented in line plots. For example, from a line plot find and interpret the difference in length between the longest and shortest specimens in an insect collection (CCSSI 2010, p. 31).
This Grade 4 standard and sample item do not go beyond encouraging a case value view of data, since students simply select individual data points and compare them to one another. The Grade 5 standard on representing and interpreting data builds on the Grade 4 standard only by broadening the types of fraction operations students are to do beyond just addition and subtraction. Hence, the Grades 4 and 5 standards miss opportunities to help students begin to develop aggregate reasoning, which children can begin to build at this age (Aridor and Ben-Zvi Citation2017; Frischemeier Citation2018). Revising or supplementing these standards to have students identify clusters, gaps, and outliers in data they have graphed would help lay the groundwork for Grade 6, when students are to forge connections among the ideas of central cluster, typical value, mean, and median. Without this necessary groundwork on developing an aggregate view of data, helping students develop deep understanding of the content described in the Grade 6 standards is likely to occupy a much greater timespan than generally anticipated or available.
4 The Mode
4.1 Research on Students’ Attraction to the Mode
A step toward developing an aggregate view of data is to group cases with the same value together. In a dotplot, for example, this is done with stacks; each stack represents a group with the same value. Konold et al. (Citation2015) considered this sort of grouping to be indicative of viewing data as a classifier, and noted that students are often attracted to the tallest stack of data when operating from such a perspective. We noticed the same student tendency to focus on the mode during each round of research, especially during the earliest lessons in each sequence. When describing typical values in distributions represented as dotplots, our students often chose the tallest stack of data. During the first round of research, students eventually kept track of game scores they obtained when rolling two dice by using dotplots. When asked to describe the typical score, they consistently chose whichever stack of data was highest. During the second round of research, students opened several packs of Skittles and kept track of the number of red candies in each pack using a dotplot. When we asked them to predict the number of reds that would be in a pack we had not yet opened, they all used the tallest stack of data to decide. The same attraction to the mode resurfaced when students described typical values in the context of graphs showing the numbers of siblings for each person in a group.
Given students’ tendencies to gravitate toward the mode, we found it necessary to design problems to focus their attention on other graph features. During the first round of research, we altered the game of rolling two dice. Rather than giving each of the four students a pair of fair dice to roll, we gave three of the students fair dice and one student a pair of trick dice weighted to consistently roll 12s. When students pooled their scores from rolling their dice seven times each, the tallest stack in the class dotplot was at 12 rather than being within the main cluster of data. This started a discussion about when the mode is not helpful for describing typical value. During the second round of research, to stop students from focusing only on the tallest stack, we showed them a set of data with no stacks (). We focused class discussion on establishing the meanings of words like cluster, gap, and outlier in the “stackless” dataset because they had mainly considered only individual vertical stacks to be “clusters” in other dotplot displays. Having no vertical stacks prompted them to start to group points representing different, but proximal, values into clusters. When we reintroduced different-sized stacks (), students finally began to speak of multiple proximal vertical stacks as constituting clusters. We then were able to move on to asking students to decide if the tallest stack fell inside or outside the main cluster of stacks when we posed questions about typical value.
4.2 Reflections on the CCSSM Stance Toward the Mode
Our experiences with students’ attraction to the mode, along with those of other researchers (Konold et al. Citation2015; Mokros and Russell Citation1995) indicate that this student thinking tendency must be addressed. The mode is not explicitly mentioned in CCSSM. Mean and median are mentioned in Grade 6, where CCSSM state that students should summarize datasets by,
Giving quantitative measures of center (median and/or mean) and variability (interquartile range and/or mean absolute deviation), as well as describing any overall pattern and any striking deviations from the overall pattern with reference to the context in which the data were gathered (CCSSI 2010, p. 45).
At minimum, it seems advisable to build the mode into the statement of this standard, given that many students will gravitate toward it, whether directed to do so or not. Additionally, if students began to identify clusters, gaps, and outliers in Grades 4 and 5, as recommended earlier, it could help put sixth grade teachers in position to ask students if the mode lies inside or outside the central cluster of data they are analyzing. Such discussions could help students make sound decisions about measures that are suitable for summarizing data in a given situation rather than automatically opting for the mode.
5 Statistical Contexts
5.1 Research on Students’ Interactions With Context
Another student thinking tendency that consistently surprised my undergraduates was that children at times completely ignored the data in problems and responded solely on the basis of their beliefs about its context. This tendency first surfaced during pre-interviews each summer. For instance, when Tyrone was asked to give the typical puppy weight in the Illustrative Mathematics item described earlier (https://www.illustrativemathematics.org/content-standards/tasks/1026), he responded, “When a dog is born, a puppy weighs 5 pounds.” He did not consult the dataset, which showed weights between 13 and 20 ounces, in making this decision. Instead, his judgment was based on an incorrect personal conception of how much dogs usually weigh when born. When Andrew was asked to decide on the typical number of customers over the course of five days at a bike shop, he stated, “I think there would be 87 average for the customers coming.” When asked why he chose 87, he responded, “Because bike stores are kind of popular.” Again, the data in the problem did not seem to play a role in his decision-making.
The tendency to seemingly ignore the data was not limited to pre-interviews. At times, children did the same thing during instructional sessions, even after they had begun to view the data as an aggregate. One instance of this phenomenon occurred as children discussed the data on soccer teams (). Claire came up to the board and identified a gap and a cluster in . She described the gap as “the big open area” and the cluster as where “they are all grouped together,” indicating that she had begun to focus on properties of graphs as wholes. However, she struggled when given the following problem:
A player from Belgium was injured during the game. Using the data from the graph, write three good predictions for how tall you think the substitute player will be. Why did you choose these numbers?
Claire wrote 79, 77, and 76 inches as her predictions, even though these values fell in the gap she had identified earlier. She reasoned that being one of these heights would help players “make more goals.” She was not alone in choosing values in the gap. Brian chose 71, 72, and 73 inches because they were “not too tall and not too short.” Andrew chose 75, 76, and 77 inches, stating only, “because I think it is good.” For Claire, and for some of her classmates, viewing the data as an aggregate was a distinct cognitive process from making inferences by coordinating attention to data and context.
5.2 Reflections on Attention to Context in CCSSM
Statistical thinking involves “continual shuttling backward and forward between thinking in the context sphere and the statistical sphere” (Wild and Pfannkuch Citation1999, p. 228). This is not a trivial process for students to master. Our study is just one among others showing that students sometimes rely solely upon personal beliefs or experiences and disregard data when making statistical inferences (Jones et al. Citation2000; Mooney Citation2002). The thinking processes involved in shuttling back and forth between data and context need concentrated attention in school. The CCSSM do mention the importance of this thinking process in the third standard for mathematical practice, “Construct viable arguments and critique the reasoning of others,” which states that students should, “reason inductively about data, making plausible arguments that take into account the context from which the data arose” (CCSSI 2010, p. 7). However, this idea is not drawn upon explicitly in the statistics standards until Grade 6, when students are to “summarize datasets in relation to their context” (CCSSI 2010, p. 45) using formal measures of center and spread. As mentioned earlier, the statistics standards for Grades 4 and 5 concentrate upon extracting quantities from graphs and then performing mathematical calculations on them. This is unfortunate, since research shows that children can begin to engage in meaningful conversations about data in relation to its context at this age and earlier (Makar Citation2018).
Research can inform the setting of learning goals that help children begin to balance attention to data and context. In one study of children’s use of context knowledge in statistics, Langrall et al. (Citation2011) noted,
We found that students used context knowledge to (a) bring new insight or additional information to the task, (b) explain the data, (c) provide justification or qualification for claims, (d) identify useful data for the task at hand, and (e) state facts that may enhance the picture of the data but are irrelevant to the process of analyzing the data (p. 47).
Findings (a)–(d) can be translated fairly directly into learning goals. Finding (e), on the other hand, points to a thinking process teachers need to be aware of helping students avoid; a learning goal based upon it might ask students to identify contextual details that are irrelevant to the process of analyzing data. In regard to finding (c), it should be noted that justifications students provide for claims can, at times, be based upon inaccurate beliefs about context, as we found working with our own students. Students have difficulty making decisions from data when the data contradict their existing beliefs about a given context (Masnick, Klahr, and Morris Citation2007). So, it would be desirable to set a goal that students should learn to synthesize statistical and contextual knowledge, at times confronting data that contradict their beliefs about a context.
6 Conclusion
Overall, it is encouraging that the CCSSM include attention to statistical thinking in Grades 4–6. However, the Grade 6 expectations seem overwhelming in light of the minimal statistics learning standards in the previous two grade levels. It would be helpful for students to begin to identify aggregates and shuttle back and forth between data and context before they reach sixth grade. Such activities could be done as they construct the types of statistical displays mentioned in the fourth and fifth grade CCSSM. Assigning learning standards to such activities could increase the likelihood they will be done widely across classrooms. By engaging in such activities, students can be in better position to satisfy Grade 6 learning standards, such as deciding on the most appropriate measure of center for a given dataset and context.
Revising the CCSSM in the ways that have been discussed could also help the enterprise of teacher education. As noted, the struggles children had discerning aggregates, reasoning about the mode, and working productively with context surprised the prospective teachers with whom I worked each year. Helping prospective teachers anticipate children’s thinking patterns is an essential part of teacher education (Jacobs and Spangler Citation2017). The ability to anticipate can help teachers design lesson plans that are responsive to students’ needs (Matthews, Hlas, and Finken Citation2009) and guide classroom discourse in productive directions (Stein et al. Citation2008). Surprising student thinking patterns become less surprising the more visible they are in the standards documents teachers are charged with implementing. So, revisions to the CCSSM that better reflect and develop common patterns of children’s statistical thinking can help students and teachers alike.
Even if the recommendations in this critique are not incorporated in future versions of the CCSSM, teachers, curriculum developers, and researchers can look for opportunities to help students develop the essential thinking processes described herein. Many of these opportunities occur in Grades 4–5 as students construct CCSSM-prescribed graphs. As they do so, we can look for opportunities to question children in ways that help them see the aggregate and its specific features, such as central cluster. We can also ask them to justify their conclusions about the data in reference to the data generation context, challenging assumptions that are based solely on personal beliefs. In the process, students gain opportunities to develop essential statistical thinking processes over a longer period of time, making the ambitious goals of the Grade 6 CCSSM more attainable.
Funding
Additional information
Funding
References
- Aridor, K., and Ben-Zvi, D. (2017), “The Co-Emergence of Aggregate and Modelling Reasoning,” Statistics Education Research Journal, 16, 38–63.
- Bakker, A., and Gravemeijer, K. P. E. (2004), “Learning to Reason about Distribution,” in The Challenge of Developing Statistical Literacy, Reasoning, and Thinking, eds. D. Ben-Zvi and J. Garfield, Dordrecht, The Netherlands: Kluwer, pp. 147–168.
- Bakker, A., and Hoffmann, M. (2005), “Diagrammatic Reasoning as the Basis for Developing Concepts: A Semiotic Analysis of Students’ Learning about Statistical Distribution,” Educational Studies in Mathematics, 60, 333–358. DOI: 10.1007/s10649-005-5536-8.
- Bakker, A., and van Eerde, D. (2015), “An Introduction to Design-Based Research with an Example from Statistics Education,” in Doing Qualitative Research: Methodology and Methods in Mathematics Education, eds. A. Bikner-Ahsbahs, C. Knipping, and N. Presmeg, New York: Springer, pp. 429–466.
- Cobb, P. (1999), “Individual and Collective Mathematical Development: The Case of Statistical Data Analysis,” Mathematical Thinking and Learning, 1, 5–43. DOI: 10.1207/s15327833mtl0101_1.
- Cobb, P., Jackson, K., and Sharpe, C. (2017), “Conducting Design Studies to Investigate and Support Mathematics Students’ and Teachers’ Learning,” in Compendium for Research in Mathematics Education, ed. J. Cai, Reston, VA: National Council of Teachers of Mathematics, pp. 208–233.
- Common Core State Standards Initiative (CCSSI) (2010), Common Core State Standards for Mathematics, Washington, DC: National Governors Association Center for Best Practices and the Council of Chief State School Officers.
- Common Core State Standards Initiative (CCSSI) (2018), Standards in Your State, Washington, DC: National Governors Association Center for Best Practices and the Council of Chief State School Officers.
- Frischemeier, D. (2018), “Design, Implementation, and Evaluation of an Instructional Sequence to Lead Primary School Students to Comparing Groups in Statistical Projects,” in Statistics in Early Childhood and Primary Education: Supporting Early Statistical and Probabilistic Thinking, eds. A. Leavy, M. Meletiou-Mavrotheris, and E. Paparistodemou, Singapore: Springer, pp. 217–238.
- Garland, S. (2016), “In Texas, New Math Standards Look a Whole Lot Like Common Core,” The Hechinger Report, May 26.
- Groth, R. E. (2017), “Developing Statistical Knowledge for Teaching During Design-Based Research,” Statistics Education Research Journal, 16, 376–396.
- Groth, R. E. (2018), “Unpacking Implicit Disagreements among Early Childhood Standards for Statistics and Probability,” in Statistics in Early Childhood and Primary Education: Supporting Early Statistical and Probabilistic Thinking, eds. A. Leavy, M. Meletiou-Mavrotheris, and E. Paparistodemou, Singapore: Springer, pp. 149–162.
- Groth, R. E., Kent, K., and Hitch, E. (2015), “Journey to Centers in the Core,” Mathematics Teaching in the Middle School, 21, 295–302.
- Jacobs, V. R., and Spangler, D. A. (2017), “Research on Core Practices in K-12 Mathematics Teaching,” in Compendium for Research in Mathematics Education, ed. J. Cai, Reston, VA: National Council of Teachers of Mathematics, pp. 766–792.
- Jones, G. A., Thornton, C. A., Langrall, C. W., Mooney, E. S., Perry, B., and Putt, I. J. (2000), “A Framework for Characterizing Children’s Statistical Thinking,” Mathematical Thinking and Learning, 2, 269–307. DOI: 10.1207/S15327833MTL0204_3.
- Konold, C. (2002), “Teaching Concepts Rather Than Conventions,” New England Journal of Mathematics, 34, 69–81.
- Konold, C., Higgins, T., Russell, S. J., and Khalil, K. (2015), “Data Seen through Different Lenses,” Educational Studies in Mathematics, 88, 305–325. DOI: 10.1007/s10649-013-9529-8.
- Langrall, C., Nisbet, S., Mooney, E., and Jansem, S. (2011), “The Role of Context Expertise when Comparing Data,” Mathematical Thinking and Learning, 13, 47–67. DOI: 10.1080/10986065.2011.538620.
- Loewus, L. H. (2015), “Nebraska’s New Math Standards: A Comparison to the Common Core,” Education Week, September 15.
- Makar, K. (2018), “Theorising Links between Context and Structure to Introduce Powerful Statistical Ideas in the Early Years,” in Statistics in Early Childhood and Primary Education: Supporting Early Statistical and Probabilistic Thinking, eds. A. Leavy, M. Meletiou-Mavrotheris, and E. Paparistodemou, Singapore: Springer, pp. 3–20.
- Masnick, A. M., Klahr, D., and Morris, B. J. (2007), “Separating Signal from Noise: Children’s Understanding of Error and Variability in Experimental Outcomes,” in Thinking with Data, eds. M. C. Lovett and P. Shah, Mahwah, NJ: Lawrence Erlbaum, pp. 3–26.
- Matthews, M. E., Hlas, C. S., and Finken, T. M. (2009), “Using Lesson Study and Four-Column Lesson Planning with Preservice Teachers,” Mathematics Teacher, 102, 504–508.
- Mokros, J., and Russell, S. J. (1995), “Children’s Concepts of Average and Representativeness,” Journal for Research in Mathematics Education, 26, 20–39. DOI: 10.2307/749226.
- Mooney, E. S. (2002), “A Framework for Characterizing Middle School Students’ Statistical Thinking,” Mathematical Thinking and Learning, 4, 23–63. DOI: 10.1207/S15327833MTL0401_2.
- National Council of Teachers of Mathematics (NCTM) (2006), Curriculum Focal Points for Prekindergarten through Grade 8 Mathematics: A Quest for Coherence, Reston, VA: NCTM.
- Smith, J. P., ed. (2011), Variability is the Rule: A Companion Analysis of K-8 State Mathematics Standards, Charlotte, NC: Information Age.
- Stein, M. K., Engle, R. A., Smith, M. S., and Hughes, E. K. (2008), “Orchestrating Productive Mathematical Discussions: Five Practices for Helping Teachers Move Beyond Show and Tell,” Mathematical Thinking and Learning, 10, 313–340. DOI: 10.1080/10986060802229675.
- Wild, C. J., and Pfannkuch, M. (1999), “Statistical Thinking in Empirical Enquiry,” International Statistical Review, 67, 223–265. DOI: 10.1111/j.1751-5823.1999.tb00442.x.