Abstract
Over the last two decades, statistics educators have made important changes to introductory courses. Current guidelines emphasize developing statistical thinking in students and exposing them to the entire investigative process in the context of interesting research questions and real data. As a result, many concepts (confounding, multivariable models, study design, etc.) previously reserved only for higher-level courses now appear in introductory courses. Despite these changes, causality is rarely discussed in introductory courses, except for warning students “correlation does not imply causation” or covering the special case of randomized controlled experiments. In this article, we argue causal inference concepts align well with statistics education guidelines for introductory courses by developing statistical and multivariable thinking, exposing students to many aspects of the investigative process, and fostering active learning. We discuss how to integrate causal inference concepts into introductory courses using causal diagrams and provide an illustrative example with youth smoking data. Through our website, we also provide a guided student activity and instructor resources. Supplementary materials for this article are available online.
1 Introduction
Undergraduate statistics education has undergone important changes in the last two decades. Today, guidelines for introductory courses emphasize developing statistical thinking, gaining experience with the investigative process, multivariable thinking, and using real data with research questions of interest to students, with less focus on calculations, mathematical derivations, and probability theory (Carver et al. Citation2016). As a result, introductory courses now include many concepts (study design, multivariable models, confounding) previously reserved for higher-level courses (Wild and Pfannkuch Citation1999; Cobb Citation2007; Rossman and Chance Citation2014; Horton Citation2015). During this same period, advances in causal inference have influenced the practice of statistics and how we think about causality (Pearl Citation1995; Hernán et al. Citation2002; Greenland, Pearl, and Robins Citation1999; Hernán and Robins Citation2006, Citation2018; Pearl and Mackenzie Citation2018). Despite its potential to contribute to multivariable thinking and providing necessary context for topics such as confounding, causal inference is rarely discussed in introductory courses, except for warning students “correlation does not imply causation” or mentioning the special case of randomized controlled experiments. In this article, we argue investigating causal applications and using causal diagrams support statistics education guidelines for introductory courses by fostering multivariable thinking and other educational goals. We provide an example of a student activity and instructor guide appropriate for introductory statistics courses. This article is novel in two ways. It is the first evaluation in the literature of causal inference’s potential to foster statistical thinking at the introductory level. Next, the activity is the first in the statistics education literature to demonstrate causal inference integrated into a typical introductory course.
In 2016, the American Statistical Association published updated guidelines for introductory, undergraduate courses in the GAISE College Report (Carver et al. Citation2016). According to these guidelines, the primary goal of introductory courses should be to develop statistical thinking in students. Effective statistical thinkers consider the investigative process as a whole (Chance Citation2002), can critically assess statistical findings (Utts Citation2003), and exercise sound judgment in approaching ambiguous, real-world scenarios (De Veaux and Velleman Citation2008). Multivariable thinking—understanding how several variables can be interrelated in complex ways—is an important component of statistical thinking (Carver et al. Citation2016). To develop statistical thinking, students must gain experience with all steps of a statistical analysis using real scenarios (Cobb and Moore Citation1997; De Veaux and Velleman Citation2008; Gould Citation2010). To meet these goals, concepts traditionally reserved for higher-level courses, such as study design, data production and management, and simulation-based inference, are included in many introductory courses, with less focus on traditional topics in probability theory and “cookbook” style approaches to teaching normal-based inference methods (Wild and Pfannkuch Citation1999; Cobb Citation2007; Garfield et al. Citation2011; Lock et al. Citation2014; Rossman and Chance Citation2014; Horton, Baumer, and Wickham Citation2015; Horton Citation2015; Tintle et al. Citation2018). Investigating causal questions and using causal diagrams complement these ongoing changes to the undergraduate statistics curriculum.
The goal of causal inference is to estimate the effect in a population of intervening on one variable, the treatment, on another variable, the outcome. Causal inference is important because many questions we investigate, both inside and outside the classroom, are about causality:
“What is the effect of youth smoking on lung function?”
“How much does adding a bedroom to my house increase its sales price?”
“Does studying an extra hour for my exam increase my grade?”
“How does policy X affect crime in major cities?”
In observational studies, measures of association relating the treatment and outcome are typically not causal effects due to confounding, which can occur in the presence of one or more common causes of the treatment and outcome. When confounding variables are known and measured, they can be adjusted for or conditioned upon, resulting in valid estimates of causal effects. When confounding variables are not measured, valid estimates of causal effects cannot be obtained. Furthermore, it is not possible to determine from the data if there is unmeasured confounding. Researchers must identify potential confounding variables and specify assumptions about the causal relationships between them to determine an appropriate set of variables to adjust for confounding (Pearl, Glymour, and Jewell Citation2016).
Causal diagrams, also called directed acyclic graphs (DAGS), are easily understood tools researchers employ in this process and they serve two important roles. First, they facilitate researcher and subject-matter expert discussions of causal relationships among variables by providing a visual representation of the system. Second, following some simple heuristics, causal diagrams allow researchers to identify potential confounders and nonconfounders, helping to determine appropriate study design and methodology (Pearl Citation1995, Citation2009; Pearl, Glymour, and Jewell Citation2016).
Causal inference supports statistics education guidelines for introductory courses in several ways. First, an important component of statistical thinking is understanding when to be skeptical about causal conclusions drawn from observational studies. Causal inference develops this thinking by requiring students to explicitly state and justify relationships between variables using nonstatistical knowledge. During this process, they develop their own opinions on whether it is appropriate to make causal conclusions. Formally introducing confounding and visually depicting it in causal diagrams helps structure this thinking in introductory students, resulting in potentially deeper understanding than just “correlation does not imply causation.” Second, causal inference gives students experience with the entire investigative process. In causal inference, students must specify relationships between variables during study design, prior to collecting data, thus gaining an appreciation for statistics as a way of thinking and not a set of steps to follow. Third, in causal inference, effect estimates have clear interpretations because researchers (students) selected the study design and methods with this purpose in mind. Fourth, causality is the basis for understanding confounding, an important concept already covered in many popular introductory statistics textbooks (Lock et al. Citation2013; De Veaux, Velleman, and Bock Citation2015; Tintle et al. Citation2015)
Instructors can integrate causal inference topics into their introductory courses without important changes to existing topic coverage. We informally introduce diagrams at the beginning of courses, using them to depict relationships between variables and encouraging students to do the same. Initially, we avoid overwhelming students with formal rules for drawing causal diagrams, instead focusing on students’ understanding that variables are often related in complex ways and an appreciation for the value of visually representing these relationships. Later in the course, after discussing confounding and the difference between causal and associational relationships, we provide students with more formal rules for drawing causal diagrams and identifying confounding variables. Lastly, we introduce adjusting for confounding variables when covering multivariable models (typically multiple regression). For courses not covering multiple regression, conducting separate analyses after stratifying on the confounding variable accomplishes similar learning objectives.
The organization of this article is as follows. First, we review some basic concepts in causal inference. Second, we present rules for drawing causal diagrams and the heuristics for interpreting them. For both sections, we emphasize basic concepts necessary to incorporate causal inference applications into existing introductory courses; the target audience for these sections is undergraduate statistics instructors with no experience with causal inference. Third, we present a student activity appropriate for introductory courses. Our activity uses a causal inference approach to the activity in Kahn (Citation2005) relating smoking to lung function in youths. The full activity and an instructor guide are available in supplementary materials. Lastly, we discuss in greater detail the value of causal inference concepts in developing statistical thinking.
2 An Overview of Causal Inference and Diagrams
The goal of causal inference is to estimate the effect of a treatment on an outcome of interest. A causal effect compares the outcome in the population if a treatment is present to the outcome in the population if the treatment is not present. In the presence of confounding variables, such as in observational studies, measures of association are not causal effects because the observed treated and untreated subjects are different in ways that are themselves causes of the outcome. For example, consider a researcher investigating the effect of attending an elite college (the treatment) on adult earnings (the outcome). The researcher administers a survey asking a large sample of college graduates where they went to college and how much they earn. If the researcher observes a positive association, we could conclude there is either a beneficial effect of elite college attendance or a spurious association from confounding. Parental socioeconomic status is a likely confounder because (1) children in families of higher socioeconomic status are more likely to attend elite colleges and (2) children in families of higher socioeconomic status have, on average, higher earnings as adults, regardless of whether they attended an elite college.
When confounding variables are known, researchers can eliminate confounding through study design or by adjusting for it during analysis. In the elite college example, the researcher could ask participants their parents’ household income (or some other measure of socioeconomic status) and adjust for it during analysis. If there are no other confounding variables and no sources of bias (such as selection bias), then valid estimates of causal effects can be obtained. When confounding variables are unmeasured, measures of association are biased estimates of causal effects (in other words, “correlation does not imply causation”). There is no way to detect the presence of unmeasured confounding variables in the data; researchers have to use expert knowledge to identify them during study design. Thus, a key component of causal inference is identifying potential confounding variables and qualitatively evaluating the validity of causal assumptions using expert knowledge, not the data. In the elite college example, we would have to evaluate the quality of the assumption that parents’ household income is the only confounding variable.
There are several challenges central to performing causal inference. First, we must assume there are no unmeasured confounding variables. In many cases, this is a very strong assumption and one that can only be made with domain knowledge and consideration of the full study design. In the elite college example, to say an association between elite college attendance and income is causal, we must know and measure enough variables to eliminate confounding. However, the list of potential confounders is very long (quality of childhood education, parental involvement, participation in youth activities, etc.). Even if we measured them all (and did so accurately), we still would not know if we had eliminated all confounding. Our analysis also relies upon the quality of our causal assumptions. In many cases though, it is difficult to determine the direction of causality between two variables. For example, is crime a cause of lower socioeconomic status or is lower socioeconomic status a cause of crime? Second, every individual in the study must have a positive probability of having been treated. This condition is referred to as positivity. In a sample, we can determine if positivity holds by checking if there are treated and untreated subjects within each stratum of confounding variables. In the elite college example, we need some subjects from wealthy backgrounds and some subjects from poor backgrounds at both elite and nonelite schools. If the positivity condition does not hold, we cannot obtain valid estimates of causal effects, which frequently occurs when there are many confounding variables.
Causal diagrams are common tools for identifying confounding variables and visualizing causal relationships (Pearl Citation1995; Greenland, Pearl, and Robins Citation1999). They represent the researcher’s assumptions about the causal model that generated the data (Pearl, Glymour, and Jewell Citation2016). Causal diagrams are directed-acyclic graphs with nodes representing variables and directed edges (arrows) representing causal relationships between the parent nodes and their child nodes. The lack of an edge between two variables indicates the researcher believes neither variable causes the other. Only diagrams containing all common causes of variables in the diagram are causal diagrams.
is a causal diagram with two variables, A and Y. The arrow from A to Y indicates the researcher’s belief that A is a cause of Y. There are no common causes of A and Y in . This diagram would be appropriate if A was a randomly assigned treatment in an experiment and Y was the outcome of interest. The randomization eliminates any edges coming into A, making other variables unnecessary to depict.
Graphically, confounding occurs when there is a so-called “backdoor path” between A and Y. In is a confounder of the effect of A on Y. The backdoor path is A to C to Y. We refer to this path as a backdoor path because C is a parent (cause) of A and Y. Adjusting for or stratifying on C blocks the backdoor path from A to Y and eliminates confounding. In the elite college example, there is a backdoor path from elite college attendance (A) through socioeconomic status (C) to adult earnings (Y). We would want to adjust for socioeconomic status in our analysis.
Alternatively, if A was a cause of C instead, then C is no longer a confounder. In the elite college example, let’s say the variable C is being awarded a Rhodes scholarship. Being awarded a Rhodes scholarship is a consequence of college attendance and part of the effect we want to measure. We do not want to adjust for a Rhodes scholarship. For more complex diagrams with multiple confounders, we adjust for a set of variables that blocks all backdoor paths from the treatment to the outcome. After adjusting for these variables, the causal diagram is said to meet the “backdoor criterion.” A full specification of the backdoor criterion is outside the scope of this article. The interested reader can find it in Pearl (Citation1995).
Another type of variable in a causal diagram is a collider. A collider is a child of both treatment and outcome. In other words, the treatment and outcome are both causes of a collider. When the treatment has no causal effect on the outcome, the two variables can be associated in analyses conditioning upon a collider. depicts the collider Z. In this case, the treatment A has no causal effect on the outcome Y. If we perform analyses conditional upon Z, it is likely we will observe a statistical association between A and Y even though there is no causal effect of A on Y.
Fig. 3 A causal diagram depicting treatment A, outcome Y, collider Z, and no causal effect of A on Y.
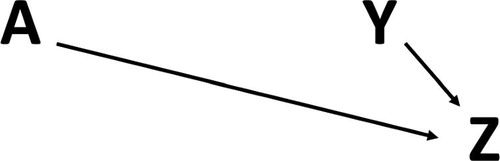
Pearl and Mackenzie (Citation2018) demonstrate conditioning on a collider using a catchy example about the relationship between beauty and talent in famous Hollywood actors. Let’s assume, all else being equal, beautiful people are no more or less likely to be talented in the general population. In other words, beauty (A) is not a cause of talent (Y). Therefore, assuming no confounding, we would not expect to observe an association between beauty and talent in the general population. However, if we only look at famous Hollywood actors, beauty will be negatively associated with talent. A person with below average looks is more likely to be talented because the person must have had some reason for becoming a famous Hollywood actor. In this example, being a famous Hollywood actor is the collider (Z). When we condition on it, we observe an association between beauty and talent even though there is no causal relationship.
More seriously, selection bias can be represented as conditioning on a collider (Hernán, Hernández-Díaz, and Robins Citation2004). For example, we can observe an association between two diseases unrelated in the general population when we only look at patients admitted to a hospital. In this case, an indicator of hospital admission is the collider and we observe an association between the two diseases in hospital patients because patients without the first disease are more likely to have the second because they were admitted to the hospital for some reason. This type of selection bias is referred to as Berkson’s bias. For additional examples of colliders, see Cole et al. (Citation2009).
Frequently, researchers indicate they will adjust for a variable during analysis by placing a box around it. depicts conditioning on the confounder C and not conditioning on the collider Z. We say the backdoor path from A to Y is blocked, and assuming there are no unmeasured confounders, we can obtain valid estimates of the causal effect of A on Y.
Students should be skeptical of drawing causal conclusions from observational data. Unmeasured confounding is a common cause of so-called spurious associations we find in associational studies. In causal diagrams, we depict unmeasured confounding variables as “backdoor” paths from the treatment to the outcome. In , the relationship of interest is from A to Y. We have adjusted for a known confounder C. However, the diagram depicts U, a set of variables unmeasured by the researchers. U is the source of spurious associations because there is a backdoor path from A to Y and we cannot obtain valid estimates of the effect of A on Y. This diagram would be appropriate for the elite college example above where the variables measured were A—whether the person attended an elite college, Y—adult earnings, and C—parents’ household income. It is not reasonable to assume there are no other confounding variables, and we would use U in the causal diagram to depict unmeasured variables that could be the source of spurious associations.
3 An Example Activity
In this activity, students assume the role of researchers investigating the effect of youth smoking on lung function. Tager et al. (Citation1979, Citation1983) conducted analyses of the effects of smoking and exposure to second-hand smoke on pulmonary functions in youths. These articles provide useful context for students and we encourage students to read them. The dataset fev.dat.txt available at http://jse.amstat.org/jse_data_archive.htm is a cross-sectional subset of data from the Tager studies and contains information on 654 subjects aged 3–19 participating in the Childhood Respiratory Disease Study in East Boston, Massachusetts in the late 1970s. These data first appeared in Rosner (Citation1995). Previously, Kahn (Citation2005) demonstrated how these data could be used in introductory courses to illustrate traditional statistical concepts. Here, we demonstrate how principles of casual inference can also be easily explained to introductory students using the same data. A student handout and instructor resources related to this activity are available in the supplementary materials.
To structure the activity, we use the six step investigative process of Tintle et al. (Citation2015): (1) ask a research question, (2) design a study and collect data, (3) explore the data, (4) draw inferences, (5) formulate conclusions, and (6) look back and ahead. While this investigative process is not specific to causal inference, we have found that the tenets apply to much of what we propose.
Students begin by discussing how they would conduct a study on the effects of youth smoking on lung function. We encourage them to read one of the original studies and understand some basics of how researchers measure lung function. They also must address why an observational study is necessary in this case and the limitations of such a study. They identify potential confounding variables and discuss how each is associated with the treatment (youth smoking) and the outcome (lung function).
To scope this analysis for an introductory course, we focus on the variables in for the remainder of the activity. The outcome is FEV, forced expiratory volume (liters), and the treatment is SMOKE, whether the subject has ever smoked. The other variables are potential confounders.
Table 1 Description of variables in this study.
Students draw a causal diagram describing the relationships between variables in . They determine these relationships with their knowledge of the subject, and we encourage them to cite evidence, such as the Center for Disease Control or American Cancer Society websites. depicts a causal diagram for the variables. Commonly, students note that AGE is likely positively associated with SMOKE as older teenagers are more likely to smoke than younger youths. Further discussions among students also tend to point out that AGE is also positively associated with FEV. When we ask them to explore this further, they find that the typical person’s FEV will more than double from age 10 to age 20 (Stanojevic et al. Citation2008). As the students will discover, the strong associations between AGE and SMOKE and between AGE and FEV have important implications in this study.
The remaining associations that the students should discover are that SEX is associated with SMOKE, HEIGHT, and FEV because boys are more likely to smoke, be taller, and have higher FEV. HEIGHT is positively associated with FEV, as taller people have higher lung capacity. The relationship between SMOKE and HEIGHT presents a nice opportunity to illustrate the challenge of conducting causal inference. In this case, valid arguments could be made for different relationships between the two variables. There is some evidence smoking slows growth (Stice and Martinez Citation2005), potentially justifying an arrow from SMOKE to HEIGHT. Others may argue that youth smokers are more likely to have parents that smoked and it is parental smoking that slows childhood growth. In this case, an appropriate diagram would have an arrow in the opposite direction and the addition of another variable (parental smoking). We encourage these discussions as they introduce students to the challenges of causal inference. For the remainder of this article, we assume the first case (SMOKE is a cause of HEIGHT).
Using the backdoor path criterion, it is next possible for students to identify confounders in their causal diagrams. In , there are four backdoor paths from SMOKE to FEV: (1) SMOKE, AGE, FEV; (2) SMOKE, AGE, HEIGHT, FEV; (3) SMOKE, SEX, FEV; (4) SMOKE, SEX, HEIGHT, FEV. Therefore, SEX and AGE are confounders of the SMOKE and FEV relationship. HEIGHT is a consequence of SMOKE, so it does not meet the definition of a confounder (we say HEIGHT lies on the causal pathway from SMOKE to FEV).
The next step is to create tables of summary statistics. In this example, the students are expected to generate a table such as the one given in . Through the summary statistics, it becomes clear to the students that smokers are older and taller. Females were more likely to be smokers, which was not expected. Most interestingly, smokers have a much higher FEV, and many students will misinterpret this result as showing smoking has a beneficial effect.
Table 2 Mean (standard deviation) by smoking status and sex.
Next, students fit a statistical model adjusting for confounders AGE and SEX. gives the effect of SMOKE on FEV for two models (matching and regression) adjusting for AGE and SEX. In the matching model, students match smokers with all nonsmokers of the same AGE and SEX. Each AGE/SEX combination is called a subclass. Weights are assigned to each observation such that the total weight for both smokers and nonsmokers in each subclass is equal to one. Then, the students compute the weighted least squares estimate for the parameter associated with FEV. The R MatchIt package makes this analysis straightforward for students to perform (Ho et al. Citation2011). Alternatively, students can adjust for AGE and SEX by including these variables in a regression model without weighting. Both adjusted models show there is clearly not a beneficial effect of smoking and potentially a small harmful effect. The average FEV of smokers was 0.15 L less than nonsmokers in the regression model (95% CI: –0.31, 0.00). Further discussion can then highlight that this is approximately a 5% reduction in lung capacity for the typical 15-year-old who smokes.
Table 3 Estimates of the change in FEV (liters) comparing smokers to nonsmokers for two models.
Students should be skeptical of interpreting estimates in as causal effects, given the observational study. We recommend having students develop alternative explanations for the observed associations in . For example, participation in sports could make young people less likely to smoke (due to less smoking in their peer group and better adult role models) and more likely to have higher FEV (due to increased levels of physical activity). In this case, we would expect to see an association between smoking and lung capacity even in the absence of a causal effect.
4 Discussion
This article, we demonstrated an activity using causal inference methods that is appropriate for developing statistical thinking in introductory courses. Students identified relationships between smoking, lung capacity, and other variables, and had to justify these relationships using outside knowledge. During this process, they make important discoveries about study design, confounding, and multivariable thinking. Causal diagrams provide both a systematic and visual way to structure this process. In addition, this activity exposes students to many aspects of the investigative process. Students construct causal diagrams during the design phase, prior to investigating data. Many introductory courses focus too narrowly on data analysis and statistical models, instead of broader issues in study design. This activity forces students to consider the broader context of the study, providing a richer context for students to interpret their statistical analysis. Lastly, this activity fosters active learning. Causal diagrams are a great discussion source, as students frequently arrive at different diagrams. Asking them to defend their diagrams is an important part of the activity, and provides an easy way to increase participation in classroom discussions.
Some might argue causal inference is too advanced for introductory courses. Leveraging causal diagrams is the key; they require no mathematical theory or notation to employ and are easily understood visual tools. Correctly drawing and interpreting a causal diagram only requires students follow a few simple rules. For this reason, epidemiologists use them extensively to communicate with medical and public health experts during study design. An instructor can easily teach these rules in one lesson, leaving the instructor with a very effective pedagogical tool for structuring discussions of multivariable relationships for the remainder of the course. Others may be concerned it will take too much time—introductory courses already have many topics fighting for space. We argue incorporating causal inference concepts requires little additional topic coverage. Instructors should already give students experience with the investigative process (Carver et al. Citation2016). The only change is to ask causal questions and use causal diagrams. For example, instead of asking “what are important factors affecting the lung function of young people?,” a question lending itself to prediction, an instructor could ask, “what is the effect of smoking on lung function in young people?” Then, use causal diagrams to identify confounding variables. There are online courses, such as Hernan (Citation2018), that can help to reinforce classroom instruction.
Instructors could extend this activity in several ways depending on the course. For example, this activity could demonstrate the importance of full reporting and transparency, a point recently emphasized by the American Statistical Association (Wasserstein and Lazar Citation2016), by having students fit several models for smoking and lung capacity adjusting for different variables and discussing the ethical implications of only reporting the statistically discernible (significant) results. These issues are very salient in causal analyses. In more advanced courses, instructors could explore direct and indirect effects of smoking on lung function with height as a mediator (Robins and Greenland Citation1992; Pearl Citation2001). Lastly, online activities and games, such as those by Kuiper and Sturdivant (Citation2015), may be effective in demonstrating the difference between association and causation, as the game could generate both treated and untreated outcomes for each virtual subject.
Supplementary Materials
Student lab:A printable student lab based on the activity described in this article. (pdf)
Instructor guide:Additional information and suggestions for instructors using the student lab. (pdf)
Website:R code and additional resources for this activity. (https://github.com/kfcaby/causalLab)
Data:Data for this activity is available at the Journal of Statistics Education data archive. (http://jse.amstat.org/datasets/fev.dat.txt)
Supplemental Material
Download PDF (129.8 KB)Supplemental Material
Download PDF (251.9 KB)Acknowledgments
Thanks to Dr. Michael Kahn for allowing us to use this dataset. Thanks to Dr. Shonda Kuiper for letting us borrow many of her ideas on structuring labs and instructor materials.
References
- Carver, R., Everson, M., Gabrosek, J., Horton, N., Lock, R., Mocko, M., Rossman, A., Rowell, G., Velleman, P., Witmer, J., and Wood, B. (2016), “Guidelines for Assessment and Instruction in Statistics Education: College Report 2016,” American Statistical Association.
- Chance, B. L. (2002), “Components of Statistical Thinking and Implications for Instruction and Assessment,” Journal of Statistics Education, 10. DOI: 10.1080/10691898.2002.11910677.
- Cobb, G. W. (2007), “The Introductory Statistics Course: A Ptolemaic Curriculum?,” Technology Innovations in Statistics Education, 1, available at https://escholarship.org/uc/item/6hb3k0nz.
- Cobb, G. W., and Moore, D. S. (1997), “Mathematics, Statistics, and Teaching,” The American Mathematical Monthly, 104, 801–823. DOI: 10.1080/00029890.1997.11990723.
- Cole, S. R., Platt, R. W., Schisterman, E. F., Chu, H., Westreich, D., Richardson, D., and Poole, C. (2009), “Illustrating Bias Due to Conditioning on a Collider,” International Journal of Epidemiology, 39, 417–420. DOI: 10.1093/ije/dyp334.
- De Veaux, R. D., and Velleman, P. F. (2008), “Math Is Music; Statistics Is Literature (or, Why Are There No Six-Year-Old Novelists?),” Amstat News, 375, 54–58.
- De Veaux, R. D., Velleman, P., and Bock, D. E. (2015), Intro Stats, Boston: Pearson.
- Garfield, J., Zieffler, A., Kaplan, D., Cobb, G. W., Chance, B. L., and Holcomb, J. P. (2011), “Rethinking Assessment of Student Learning in Statistics Courses,” The American Statistician, 65, 1–10. DOI: 10.1198/tast.2011.08241.
- Gould, R. (2010), “Statistics and the Modern Student,” International Statistical Review, 78, 297–315. DOI: 10.1111/j.1751-5823.2010.00117.x.
- Greenland, S., Pearl, J., and Robins, J. M. (1999), “Causal Diagrams for Epidemiologic Research,” Epidemiology, 10, 37–48. DOI: 10.1097/00001648-199901000-00008.
- Hernan, M. (2018), “Causal Diagrams: Draw Your Assumptions Before Your Conclusions,” available at https://www.edx.org/course/causal-diagrams-draw-assumptions-harvardx-ph559x.
- Hernán, M. A., Hernández-Díaz, S., and Robins, J. M. (2004), “A Structural Approach to Selection Bias,” Epidemiology, 15, 615–625. DOI: 10.1097/01.ede.0000135174.63482.43.
- Hernán, M. A., Hernández-Díaz, S., Werler, M. M., and Mitchell, A. A. (2002), “Causal Knowledge as a Prerequisite for Confounding Evaluation: An Application to Birth Defects Epidemiology,” American Journal of Epidemiology, 155, 176–184.
- Hernán, M. A., and Robins, J. M. (2006), “Estimating Causal Effects From Epidemiological Data,” Journal of Epidemiology & Community Health, 60, 578–586.
- Hernán, M. A., and Robins, J. M. (2018), Causal Inference, Boca Raton, FL: Chapman and Hall/CRC.
- Ho, D. E., Imai, K., King, G., and Stuart, E. A. (2011), “MatchIt: Nonparametric Preprocessing for Parametric Causal Inference,” Journal of Statistical Software, 42, 1–28. DOI: 10.18637/jss.v042.i08.
- Horton, N. J. (2015), “Challenges and Opportunities for Statistics and Statistical Education: Looking Back, Looking Forward,” The American Statistician, 69, 138–145. DOI: 10.1080/00031305.2015.1032435.
- Horton, N. J., Baumer, B. S., and Wickham, H. (2015), “Setting the Stage for Data Science: Integration of Data Management Skills in Introductory and Second Courses in Statistics,” CHANCE, 28, 40–50. DOI: 10.1080/09332480.2015.1042739.
- Kahn, M. (2005), “An Exhalent Problem for Teaching Statistics,” Journal of Statistics Education, 13. DOI: 10.1080/10691898.2005.11910559.
- Kuiper, S., and Sturdivant, R. X. (2015), “Using Online Game-Based Simulations to Strengthen Students’ Understanding of Practical Statistical Issues in Real-World Data Analysis,” The American Statistician, 69, 354–361. DOI: 10.1080/00031305.2015.1075421.
- Lock, R. H., Lock, P. F., Morgan, K. L., Lock, E. F., and Lock, D. F. (2013), Statistics: Unlocking the Power of Data, Hoboken, NJ: Wiley.
- Lock, R. H., Lock, P. F., Morgan, K. L., Lock, E. F., and Lock, D. F. (2014), “Intuitive Introduction to the Important Ideas of Inference,” in Proceedings of the Ninth International Conference on Teaching Statistics.
- Pearl, J. (1995), “Causal Diagrams for Empirical Research,” Biometrika, 82, 669–688. DOI: 10.1093/biomet/82.4.669.
- Pearl, J. (2001), “Direct and Indirect Effects,” in Proceedings of the Seventeenth Conference on Uncertainty in Artificial Intelligence, Morgan Kaufmann Publishers Inc., pp. 411–420.
- Pearl, J. (2009), Causality, Cambridge: Cambridge University Press.
- Pearl, J., Glymour, M., and Jewell, N. P. (2016), Causal Inference in Statistics: A Primer, New Jersey: Wiley.
- Pearl, J., and Mackenzie, D. (2018), The Book of Why: The New Science of Cause and Effect, New York: Basic Books.
- Robins, J. M., and Greenland, S. (1992), “Identifiability and Exchangeability for Direct and Indirect Effects,” Epidemiology, 3, 143–155. DOI: 10.1097/00001648-199203000-00013.
- Rosner, B. (1995), Fundamentals of Biostatistics, New York: Duxbury Press.
- Rossman, A. J., and Chance, B. L. (2014), “Using Simulation-Based Inference for Learning Introductory Statistics,” Wiley Interdisciplinary Reviews: Computational Statistics, 6, 211–221. DOI: 10.1002/wics.1302.
- Stanojevic, S., Wade, A., Stocks, J., Hankinson, J., Coates, A. L., Pan, H., Rosenthal, M., Corey, M., Lebecque, P., and Cole, T. J. (2008), “Reference Ranges for Spirometry Across All Ages: A New Approach,” American Journal of Respiratory and Critical Care Medicine, 177, 253–260. DOI: 10.1164/rccm.200708-1248OC.
- Stice, E., and Martinez, E. E. (2005), “Cigarette Smoking Prospectively Predicts Retarded Physical Growth Among Female Adolescents,” Journal of Adolescent Health, 37, 363–370. DOI: 10.1016/j.jadohealth.2004.10.017.
- Tager, I. B., Weiss, S. T., Muñoz, A., Rosner, B., and Speizer, F. E. (1983), “Longitudinal Study of the Effects of Maternal Smoking on Pulmonary Function in Children,” New England Journal of Medicine, 309, 699–703. DOI: 10.1056/NEJM198309223091204.
- Tager, I. B., Weiss, S. T., Rosner, B., and Speizer, F. E. (1979), “Effect of Parental Cigarette Smoking on the Pulmonary Function of Children,” American Journal of Epidemiology, 110, 15–26. DOI: 10.1093/oxfordjournals.aje.a112783.
- Tintle, N., Chance, B. L., Cobb, G. W., Rossman, A. J., Roy, S., Swanson, T., and VanderStoep, J. (2015), Introduction to Statistical Investigations, Wiley Online Library.
- Tintle, N., Clark, J., Fischer, K., Chance, B., Cobb, G., Roy, S., Swanson, T., and VanderStoep, J. (2018), “Assessing the Association Between Precourse Metrics of Student Preparation and Student Performance in Introductory Statistics: Results From Early Data on Simulation-Based Inference vs. Nonsimulation-Based Inference,” Journal of Statistics Education, 26, 103–109. DOI: 10.1080/10691898.2018.1473061.
- Utts, J. (2003), “What Educated Citizens Should Know About Statistics and Probability,” The American Statistician, 57, 74–79. DOI: 10.1198/0003130031630.
- Wasserstein, R. L., and Lazar, N. A. (2016), “The ASA’s Statement on p-Values: Context, Process, and Purpose,” The American Statistician, 70, 129–133. DOI: 10.1080/00031305.2016.1154108.
- Wild, C. J., and Pfannkuch, M. (1999), “Statistical Thinking in Empirical Enquiry,” International Statistical Review, 67, 223–248. DOI: 10.1111/j.1751-5823.1999.tb00442.x.