Abstract
The aim of this paper is to propose an exploratory study on simple, accurate and computationally efficient movement classification technique for prosthetic hand application. The surface myoelectric signals were acquired from 2 muscles—Flexor Carpi Ulnaris and Extensor Carpi Radialis of 4 normal-limb subjects. These signals were segmented and the features extracted using a new combined time-domain method of feature extraction. The fuzzy C-mean clustering method and scatter plots were used to evaluate the performance of the proposed multi-feature versus other accurate multi-features. Finally, the movements were classified using the adaptive neuro-fuzzy inference system (ANFIS) and the artificial neural network. Comparison results indicate that ANFIS not only displays higher classification accuracy (88.90%) than the artificial neural network, but it also improves computation time significantly.
Additional information
Notes on contributors
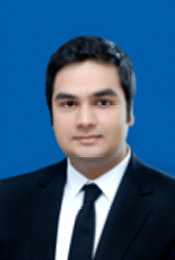
Hessam Jahani Fariman
Hessam Jahani Fariman received B.Sc. degree in Electrical and Electronic Engineering from Ferdowsi University of Mashhad, Iran in 2011 and M.Sc. in Control and Automation Engineering from the University of Putra Malaysia, Malaysia, in 2014. His areas of interest include pattern recognition, machine learning, biomedical engineering and rehabilitation.
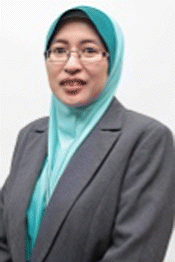
Siti A. Ahmad
Siti Anom Ahmad is a senior lecturer at the Department of Electrical and Electronic Engineering, University Putra Malaysia (UPM). She received her first degree in Electronic/Computer Engineering from UPM, and then obtained MSc in Microelectronics System Design and PhD in Electronics from University of Southampton UK in 2004 and 2009 respectively. Her research interests are biomedical engineering, signal processing and intelligent control system.
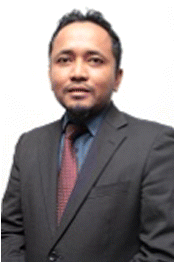
M. Hamiruce Marhaban
Mohammad Hamiruce Marhaban received his degree in Electrical and Electronic engineering from the University of Salford, UK, in 1998 and Ph.D. in Electronic Engineering from the University of Surrey, UK, in 2003. He is an associate professor at the Department of Electrical and Electronic Engineering, UPM. His areas of interest include intelligent control system and computer vision.
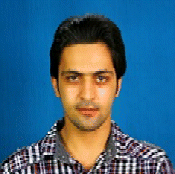
M. Ali Jan Ghasab
Mohammad Ali Jan Ghasab received B.Eng. degree in Electrical and Electronic Engineering from Azad University, Iran in 2010 and M.Sc. in Electronic Engineering from the University of Putra Malaysia, Malaysia, in 2014. His areas of interest include image & signal processing, biomedical engineering and rehabilitation, pattern recognition, machine learning, control system design and optimization.
Paul H. Chappell
Paul H Chappell is an associate professor in the School of Electronics and Computer Science at the University of Southampton 2017 1BJ UK. He is a Chartered Engineer, FIET, FIPEM, SMIEE and MInstP. Paul has published over one hundred and sixty journal articles, conference papers, chapters in books and patents. [email protected]