Abstract
Background: Research is needed on how substance use patterns develop over time in the general adolescent population. This knowledge is crucial in calibrating prevention and other interventions. Method: The study concerns use of cigarettes, alcohol, and cannabis in a nationally representative cohort of Swedish adolescents (n = 3999). Two waves (9th and 11th school grade) from the Futura01 study were analyzed using latent transition analysis (LTA) and multinomial regression analysis. Results: Four substance use patterns, were identified, ranging from Non-user, Alcohol experienced, Alcohol User to Co-user of cigarettes, alcohol, and cannabis. Statuses thus conveyed a continuum from no use to more advanced use. Half of the individuals remained in their original status between time-points, and half transitioned, most often one step on the continuum. Alcohol user was the most stable status over time (0.78), and the Non-user status the least (0.36). The probability of remaining in the Alcohol experienced status was 0.57, and 0.45 for the Co-user status. There was a low probability of transitioning from alcohol to cannabis use. Females were more likely to belong to Alcohol experienced and males to Co-user statuses, but these associations weakened over time. Conclusions: The study identified transitions across substance use statuses between time-points. These usually concerned different levels of alcohol use, and not into more advanced substance use that included the illegal substance cannabis. The study corroborates that young Swedes belong to a “sober” generation and usually do not transition from legal into illegal substance use during late adolescence, though with some gender differences.
Introduction
Legal and illegal substance use have long been part of adolescence, considered a means to explore limits and have fun (Jackson, Citation2004; Parker et al., Citation1998). However, adolescent substance use has also been framed in terms of deviance and dangerousness, with emphasis placed on the potential negative effects this use can have on both current and future generations of adolescents (Lindgren, Citation1993). The consumption of legal and illegal substances poses several health risks (Abrams et al., Citation2018; Gobbi et al., Citation2019; Marshall, Citation2014). Use of more than one substance has generally been associated with a greater risk of harm than the use of a single substance (Connor et al., Citation2013, Citation2014; Conway et al., Citation2013; Halladay et al., Citation2020), and early use of more than one substance is considered to be particularly harmful (Ciairano et al., Citation2009; Moss et al., Citation2014; Poudel & Gautam, Citation2017). With this as a starting point, it is crucial to study how substance use develops over time concerning both legal substances and illegal substancesFootnote1 in representative samples of adolescents from the general population.
After the prevalence of substance use peaked during the end of the 20th century, the past decades have shown decreased use of legal substances among adolescents across Europe, and the use of cannabis has decreased somewhat during this time period as well (ESPAD, Citation2019). On the international level, it appears as if use patterns vary with gender (Chiauzzi et al., Citation2013; Choi et al., Citation2018; Halladay et al., Citation2020; Measham & Shiner, Citation2009). The decline in alcohol use has been steeper among males than among females (Pape et al., Citation2018), but males seem more prone to transition forward into the use of illegal substances (Choi et al., Citation2018).
Swedish adolescents have followed the decreasing trend in legal substance use (Gripe, Citation2021; Zetterqvist & Ramstedt, Citation2019). Simultaneously, the mean age of onset of both alcohol and cigarette use has increased (Guttormsson & Zetterqvist, Citation2019). The prevalence of cannabis use has been stable rather than decreasing, but remains low compared to most European countries (Englund, Citation2019; ESPAD, Citation2019). There is an association between cigarettes and cannabis use among Swedish adolescents, but the question of causality remains unclear (Guttormsson & Zetterqvist, Citation2019).
Efforts have been made to understand why changes in substance use patterns occur among adolescents (Caluzzi et al., 2022; Vashishtha et al., Citation2020). It has been suggested that the explanation should be sought on the population level, rather than on the individual level. This includes considering both the somewhat vague concept of “youth culture” as well as different paths into adulthood (Pape et al., Citation2018).
There is a lack of research on what the characteristics are of different groups of adolescent substance users in Sweden, as well as how their substance use patterns develop over time (e.g., Karlsson et al., Citation2018). Previous international studies have identified different groups of substance users with person-centered approaches, such as latent class analysis (LCA). In population-based studies, these patterns typically include no or limited use, moderate use, and extensive use (Connor et al., Citation2013). A systematic review identified four main patterns: alcohol; alcohol with cannabis and/or tobacco; tobacco with alcohol; alcohol, tobacco, and cannabis, with or without other illegal substances (Halladay et al., Citation2020). For example, a nationally representative cohort study of 10th graders in the United States identified four predominant classes of users: a Non-user group; an alcohol user group; a marijuana user group; and a polysubstance user group (Conway et al., Citation2013). Moreover, Choi and colleagues identified three classes in a sample of adolescents in Texas, USA: mild alcohol use; alcohol and moderate marijuana use; and polysubstance use (Choi et al., Citation2018).
Longitudinal approaches, for instance latent transition analysis (LTA), can identify shifts in status membership over time (Collins & Lanza, Citation2003), as well as transitions backward in use, as in individuals who use a substance at baseline but not at follow-up (Velicer et al., Citation1996). Increased understanding of such transitions is important when informing prevention and intervention strategies (Connor et al., Citation2014; Lanza & Rhoades, Citation2013; Tomczyk, Isensee, et al., 2016; Tomczyk, et al., 2016). However, the use of LTA to explore substance use among adolescents over time is scarce (Merrin et al., Citation2018). There is a need for research on youth substance use in different contexts, including also in low-prevalence settings (Sznitman et al., Citation2013) such as Sweden.
To contribute to this important field of research, we conducted an LTA study on a large, nationally representative longitudinal sample of Swedish school adolescents born in 2001. The aim of this study is to identify patterns of cigarette; alcohol and cannabis use in the general population of adolescents and describe transitions between use patterns over time. A secondary aim is to examine whether status membership and transitions over time vary with gender.
Data and methods
Sample and setting
We use data from the two first waves of Futura01, a prospective cohort study among a nationally representative sample of Swedish adolescents born in 2001 (Dennermalm et al., Citation2022; Raninen et al., Citation2022; Sjödin et al., Citation2021, Citation2022). Futura01 uniquely follows Swedish adolescents’ paths into adulthood, with a focus on trying to understand declining drinking levels (Raninen et al., Citation2022).
The first wave of data was collected in 2017, when participants were 15–16 years of age and attended 9th grade (n = 5576), and a second wave of data was collected two years later in 11th grade. Out of 500 randomly selected schools at the first wave, 344 schools (69%) participated, with one school class per school filling out the questionnaires. The first wave was collected manually on paper in the classroom, and the second wave was collected through postal services or an online questionnaire. After excluding incomplete surveys and surveys without ID numbers, the response rate at baseline was 82% among the students who were offered to participate. This study included those who participated in both waves and had complete values on all the items used in the analyses (i.e., using listwise deletion, n = 3999).
The Swedish Ethical Review Authority granted permission 2020–01272. The authors of this study did not collect the data but complied with all study protocols to ensure the protection of the data and the anonymity of the participants.
Measures
Items included were dichotomous variables relating to substance use during 9th and 11th grade. They were current use of cigarettes (daily/almost daily) and current use of alcohol, measured by the Alcohol Use Disorders Identification Test (AUDIT-C). AUDIT-C measures problem drinking with three questions: “How often did you have a drink containing alcohol in the past year” (“Never,” “Monthly or less,” “Two to four times a month,” “Two to three times per week,” “Four or more times a week”), “How many drinks did you have on a typical day when you were drinking in the past year?” (“1 or 2,” “3 or 4,” “5 or 6,” 7 to 9,” 10 or more”) and “How often did you have six or more drinks on one occasion in the past year?” (“Never,” “Less than monthly,” “Monthly,” “Weekly,” “Daily or almost daily”). The summed score ranges from 0 to 12 points. There are no set cut-points between different levels of use or risk in AUDIT-C and some suggest that the scale should be interpreted differently depending on age, gender, and other factors (Liskola et al., Citation2018), making universal cut-points for different behaviors less relevant. We used AUDIT-C with cut-points set at one and five, respectively, to explore different levels of alcohol experience. A score of one or higher tapped having tried alcohol and a score of five or higher tapped a more advanced alcohol use. We decided upon cut-points that were deemed theoretically relevant at both time-points. Cannabis consumption was measured by two questions, differentiating between use on one or two occasions and 10 or more times. The data we had access to measured lifetime prevalence of cannabis use at 9th grade and past year use at 11th grade. Additionally, we included gender as a covariate in the later multinomial regression analyses. The items and descriptive statistics are shown in .
Table 1. Substance use at two time-points (%).
Latent transition analysis
LTA is a person-centered statistical method for longitudinal modeling (Velicer et al., Citation1996). It is based on LCA, a method that aims to estimate latent variables based on responses to a set of dichotomous or categorical manifest items (Collins & Lanza, Citation2003; Velicer et al., Citation1996). The idea is that the latent variable causes responses to the observed variables and that the former can be estimated based on the response patterns to the latter. As such, LCA is similar to factor analysis in which a continuous latent variable is assumed to determine the responses to a range of continuous indicators (Collins & Lanza, Citation2003). However, the main difference is that LCA is person-centered, and is as such more useful for identifying distinct groups of individuals. LCA also makes it possible to assign persons into latent classes and to predict class membership from covariates. Two sets of parameters are central to LCA: the unconditional probability of being a member in each latent class (i.e., the prevalence of each class), and the probability of responding in a certain way to each of the indicators included for each latent class (i.e., conditional probabilities) (Collins & Lanza, Citation2003). For longitudinal data, LTA builds on the logic of LCA but can be used to explore the probability of individuals’ movements between statuses over time (Collins & Lanza, Citation2003; Velicer et al., Citation1996).
Key to these approaches is model fit, that is, determining the ideal number of classes in LCA or statuses in LTA. This choice is guided by two overarching principles: model fit using a range of fit indices (Asparouhov & Muthén, Citation2012; Nylund et al., Citation2007), and interpretability (Collins & Lanza, Citation2003; Nylund et al., Citation2007). However, there is inconclusive evidence on which measure is more robust when evaluating model fit (Asparouhov & Muthén, Citation2012; Nylund et al., Citation2007), and the literature is also inconsistent regarding procedures (Ryoo et al., Citation2018). This study drew inspiration from (Ryoo et al., Citation2018) and included the following six steps: We began by deciding upon dichotomous items, based on assumptions of usefulness at both time-points. Second, we diagnosed and explored cross-sectional data using 1–6-class solutions in the LCA to identify which solution is favored with respect to the fit indices Akaike Information Criterion (AIC), Bayesian Information Criterion (BIC), Adjusted BIC, Lo-Mendell-Rubin Likelihood Ratio Test (LMR LRT), Bootstrap LRT and Entropy. We then tested if measurement invariance across time-points held for the status solutions suggested in the second step. In the following step, we decided upon the ideal number of statuses based on interpretability and a range of fit indices (AIC, BIC, and the Likelihood Ratio Test). Then we identified transition probabilities. (6) Lastly, we ran multinomial regression analysis with gender as covariate and substance use status as the outcome.
We used Mplus version 8.7 (Los Angeles, CA, USA) for the LCA and LTA and IBM SPSS statistics version 26 (IBM Corp., Armonk, NY) for the multinomial regression analysis.
Results
shows proportions responding “Yes” to the items used in the analysis by time-point. The total proportions are presented, as well as stratified by gender. The prevalence of daily or almost daily use of cigarettes increased from 2.8% in 9th grade to 6.3% in 11th grade. The percentage of individuals who scored one or more on the AUDIT-C scale increased from 28.5% to 67.9% and the proportion of individuals who scored five or more increased from 8.4% to 21.6%. Similarly, there was an increase in reported use of cannabis once or twice from 5.0% to 9.2% and 10 times or more from 1.2% to 2.2%. Females were more likely to have used cigarettes daily or almost daily and to have scored one or more on the AUDIT-C scale, while males were more likely to have scored five or more on the AUDIT-C and to have experimented with or used cannabis.
presents fit indices for the LCA analyses with different number of classes, separately for 9th and 11th grade. These provided initial suggestions on the ideal number of statuses to be included in the LTA. Lower AIC, BIC, and adjusted BIC values indicate relatively better model fit. Though there is no consensus on where to set the cut-point for good class separation, Asparouhov and Muthén (Citation2014) argue that entropy values of >0.6 indicate good class separation.
Table 2. Fit statistics and entropy for LCA, 1–6-class models.
The fit indices in are inconclusive, suggesting that either a 3- or 4-class solution fits the data best. Therefore, we chose to move forward using both the 3- and 4-class solutions in the LTA, where we began with assessing whether measurement invariance held across 9th and 11th grade for the 3- and the 4-class solution (see ).
Table 3. Tests of longitudinal measurement invariance.
The AIC and BIC values in indicated better model fit for the 4-status solution, compared to the 3-status solution, regardless of whether measurement invariance was assumed or not. The fit indices, however, were inconsistent as to whether a model with measurement invariance fitted the data better or not. Because the meaning of the transitions in LTA is easier to interpret if measurement invariance holds (Collins & Lanza, Citation2003), we continued with a 4-class solution assuming such invariance. The item response probabilities (IRPs) for the final 4-status solution LTA are presented in and . Because measurement invariance is assumed, the IRPs are the same for both 9th and 11th grade.
Table 4. Item response probabilities at both time-points by latent statuses.
Based on our interpretation of the characteristics of the IRPs, we chose the following names for the latent statuses: Non-user, Alcohol experienced (high probability of scoring ≥1 on AUDIT-C), Alcohol user (high probability of scoring ≥ 5 on AUDIT-C), and Co-user (high probability of scoring on both AUDIT-C scores and having tried cannabis and daily use of cigarettes).
The size of each status varied greatly, both between statuses and between time-points. Notably, daily or almost daily use of cigarettes did not define a status on its own, nor did it come to dominate the Co-user status.
displays the prevalence of the different statuses at 9th and 11th grade, and the flow between these. The numbers to the left of the diagram represent the prevalences in 9th grade and the number to the right the prevalences in 11th grade. As can be seen, the majority of the participants were assigned to the Non-user status at 9th grade (67.8%) and considerably smaller shares to the other statuses: Alcohol experienced (20.1%), Alcohol user (9.4%), and Co-user (2.7%). At 11th grade, the largest status shifted from Non-user (24.3%) to Alcohol experienced (44.4%). The proportion of those belonging to the Alcohol user status three-folded to 27.0%, and the Co-user status increased to 4.3%.
Figure 2. Diagram illustrating the prevalence of the different statuses at 9th and 11th grade, and the flow between these. The thickness of the bars illustrates the transition prevalence.
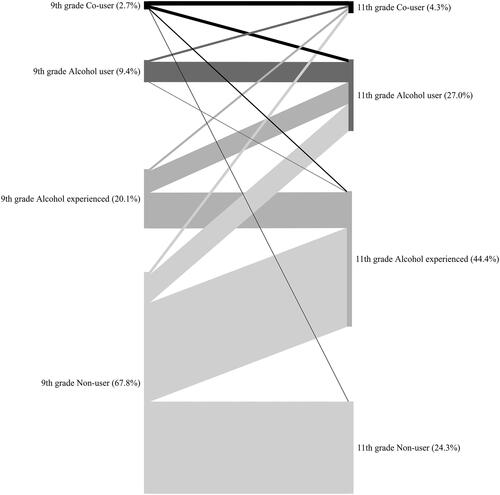
As can be seen from the thickness of the bars in , the majority either stayed in the status assigned to them at 9th grade or transitioned to a status in close proximity. A substantial part of the individuals stayed in the status they were assigned to at 9th grade (48.6%, not shown in the figure). Approximately, half of the individuals transitioned forward (49.9%, not shown in the figure), predominately from Non-user to the two alcohol statuses. Interestingly, the individuals who were assigned to the Co-user status at 11th grade derived equally from the Co-user status, the two alcohol statuses, and the Non-user status. This suggests that the individuals with experience of cannabis at 11th grade had diverse previous substance use experiences.
Shifting to transition probabilities, the LTA provides conditional transition probabilities for each of the statuses (). In determining how to label the magnitude of the transition probabilities, we worked inductively to identify measures which we considered fit the data best. In the article, we based the measures on terciles: this measures the study’s latent transition probabilities 0.00 − 0.16 as “low stability,” 0.36 − 0.57) as “medium stability,” and finally, 0.78 as “high stability.” As shown in , individuals in the Non-user status were medium stable (0.36), with a very low probability of transitioning to other statuses than Alcohol experienced (0.47). Alcohol-experienced individuals were also a medium stable group with a 0.57 chance of remaining in their initial status. The relatively small share of individuals in the Alcohol user status was the most stable (0.78), with a low probability of transitioning to either the Alcohol experienced or Co-user statuses. Individuals assigned to the Co-user status stayed in their initial status (0.45) or transitioned backward to the Alcohol user status (0.36). The total probability of transitioning from the Co-user status was 0.55 (1–0.45), showing that the majority of those with experience of cannabis use in 9th grade had no cannabis experience the past year in 11th grade.
Table 5. Latent transition probabilities.
Next, we performed multinomial regression analyses at both time-points, with gender as covariate. The dependent variable was membership in each of the four latent statuses, separate for 9th and 11th grade (see ). The Non-user status was used as reference category. Females had 23% higher odds of belonging to the Alcohol experienced status than males in 9th grade and 32% higher odds in 11th grade, compared to the reference category (OR 1/0.81 vs. OR 1/0.76). The estimates were significant and stable across time-points. Males were more likely to belong to the Co-user status at both time-points, compared to the reference category, although to a lesser degree at 11th grade (OR 1.93 vs. OR 1.44). The results were statically significant. The association between gender and being assigned to the Alcohol user status was small and non-significant.
Table 6. Cross-sectional multinomial regression analysis with gender as co-variate (male = 1) and the Non-user status as reference category.
Discussion
Main results based on different substances
Using LTA on a nationally representative cohort of Swedish adolescents, this study identified four substance use statuses, ranging from Non-user, Alcohol experienced, Alcohol user, to Co-user. The majority was assigned to the Non-user status or the two alcohol statuses, which corresponds with previous research (Halladay et al., Citation2020; Tomczyk, Isensee, et al., 2016). The size of the statuses varied greatly, both between the statuses and between time-points. The low prevalence of cigarette and cannabis use in Sweden may explain why this study, similar to (Choi et al., Citation2018; Merrin et al., Citation2018), but in contrast to (Lanza et al., Citation2010), did not identify a cigarette-only or a cannabis-only status.
The study identified two distinct alcohol statuses. Our interpretation is that the Alcohol experienced status consisted of individuals who had minor experience with alcohol, with a high item response probability (IRP) for AUDIT-C ≥ 1 and IRP of zero on the AUDIT-C ≥ 5 item. The members of this status had used 1–2 units of alcohol monthly or less, but not experienced drunkenness or more frequent use. In contrast, the Alcohol user status included individuals who scored five or more on AUDIT-C and thereby had a more diverse experience of alcohol. It has been suggested (Liskola et al., Citation2018) that three is an adequate cut-point for early detection of problematic drinking among adolescents. Although early detection of problematic use was beyond the scope of this study, one in five scored an AUDIT-C of five or more in 11th grade. If applying cutoffs suggested by Liskola et al. (Citation2018), our study would indicate that a fairly large proportion of Swedish adolescents are at risk of problematic drinking. This does not corroborate current research on adolescent alcohol use, where the vast majority of the adolescent population uses less and has a later onset than previous generations (e.g., Guttormsson & Zetterqvist, Citation2019), and seems to be an overly inclusive definition of alcohol problems.
The numerically smallest status, the Co-user status, was defined by a high IRP for alcohol and cannabis experience and medium-high IRP for use of cigarettes (0.43), alcohol (0.63), and cannabis (0.48). Our interpretation is that this status encompassed a heterogenous group of adolescents with different combinations of substance use experiences. Thus, they shared being alcohol and cannabis experienced, with some having additional use of cigarettes, and somewhat more frequent alcohol, and/or cannabis use. Our findings suggest that participants who used alcohol often used it without the presence of other substances, while individuals who used cannabis also used alcohol (Choi et al., Citation2018; Merrin et al., Citation2018; Raninen et al., Citation2018). The lack of a cannabis-only status challenges the idea of cannabis being a replacement substance for cigarettes or alcohol (Raninen et al., Citation2018), since cannabis is often used alongside these substances (Merrin et al., Citation2018).
Transitions between substance use statuses
As mentioned, the LTA identifies transitions between statuses over time (Velicer et al., Citation1996). The international literature is inconclusive as to the prevalence of transitions between substance use statuses (Choi et al., Citation2018; Chung et al., Citation2013; Merrin et al., Citation2018; Mistry et al., Citation2015). Our LTA revealed significant transitions between time-points, although usually to a nearby status, similar to what has been reported previously (Choi et al., Citation2018). As shown in prior research, more profound changes over statuses are often only observed over longer time periods (Merrin et al., Citation2018). In this study, the stability of the statuses varied from 0.36 (Non-user) to 0.78 (Alcohol user). Also, transitioning forward to a more distal status was uncommon, as were transitions backward from the alcohol statuses.
In contrast to Chung et al. (Citation2013), Lanza et al. (Citation2010), and Merrin et al. (Citation2018), this study shows that individuals with an early onset of alcohol use were more likely to continue using alcohol, compared to individuals with early onset of cannabis, of which more than half did not maintain cannabis use by 11th grade. In other words, an early onset of cannabis in this sample of adolescents from the Swedish normal population did not predict cannabis use in later school years.
Approximately, half of the individuals remained in their original status and half transitioned forward into a status of more extensive or advanced use. As half of the individuals transitioned forward, this finding could be interpreted as support for the gateway hypothesis regarding moves from “softer” to “harder” substance use (Yamaguchi & Kandel, Citation1984). However, the probability of transitioning to the Co-user status from other statuses was low, suggesting that movement primarily occurred within the boundaries of legal substances. Additionally, following the gateway hypothesis, one would expect that a decrease in the use of legal (gateway) substances would result in a decrease in the use of cannabis. However, cannabis use among Swedish adolescents remains low and stable (Englund, Citation2019), raising questions about the ability of the gateway hypothesis to inform the understanding of use among Swedish adolescents.
Another question that this study raises is how to understand transitions forward into more extensive use or more substances compared to transitions backward into less use and fewer substances. Transitioning forward may be associated with negative circumstances or risk factors (del Palacio-Gonzalez & Pedersen, Citation2022) or may be a normal part of current youth culture (Parker et al., Citation1998), while transition backward from cannabis or alcohol use may potentially be the consequence of negative experiences or school-based prevention. However, evaluations of these school-based prevention efforts are scarce and previous research indicates low impact due to poor quality of programming and poor use of resources (Beckman et al., Citation2017; Karlsson, Citation2006). The characteristics of this numerically small group of backward transitioners are of interest for the development of more high-quality programs and should be the subject of future research.
Substance use patterns and gender
Prior research is somewhat inconclusive as to the association between gender and substance use (Chiauzzi et al., Citation2013; Choi et al., Citation2018; Halladay et al., Citation2020; Tomczyk, Isensee, et al., 2016). This study showed a statistically significant association between being female and belonging to the alcohol-experienced group at both time-points. As to the use of cannabis, a LCA-specific systematic review found no clear association between gender and use of illegal substances (Tomczyk, Isensee, et al., 2016). In contrast, our analysis showed that males were more likely to use several substances, including cannabis, which agrees with previous research (Choi et al., Citation2018). However, the results suggest that the association between gender and Co-use use weakened somewhat over time. Gender thus seems to be an instable predictor (Dennermalm et al., Citation2022) and appears of less importance for understanding advanced substance use over time. A gender-specific LTA could explore aspects of gender’s influence on substance use over time, this could be the subject of future research.
Strengths and limitations
A key strength of this study is its longitudinal design, which makes it possible to elucidate transitions in substance use patterns over time. Another strength is that the sample is nationally representative. One important limitation is the different time frames of the items capturing cannabis use. This is not standard practice when doing LTA, where both the items, number of statuses, and IRPs, preferably, should be identical across time-points. Another limitation concerns the possibility of social desirability bias when participants filled out the questionnaire during class. Finally, we would like to emphasize the limitation of using the same cutoff points for males and females in AUDIT-C since gender may affect drinking patterns and outcomes.
Conclusion
This study concludes that a majority of those assigned to the Co-user status in 9th grade transitioned backward to use of legal substances, raising questions about the idea of legal substances as gateways to cannabis use. It also shows that Swedish adolescents are rarely daily users of cigarettes, their use of alcohol is not very extensive and they tend to not use cannabis. Moreover, the vast majority of transitions in substance use statuses between 9th and 11th grade occurred within the boundaries of legal substances. Young Swedes appear to belong to a “sober” generation, and they usually do not transition into cannabis use during late adolescence. This challenges the extent to which youth substance use in Sweden in fact lives up to its politico-professional status as a “high-profile problem” (see Månsson & Ekendahl, Citation2015).
Acknowledgments
Thanks to Jonas Raninen who provided us with the Futura01 data.
Disclosure statements
The authors report there are no competing interests to declare.
Additional information
Funding
Notes
1 In Sweden, some substances are categorized as illegal (e.g., cannabis, cocaine, and opioids), and others legal (tobacco and alcohol). While use of tobacco and alcohol among adolescents under the age of 18 is not criminalized, it is illegal to sell to minors (FRA, Citation2017; Zetterqvist & Ramstedt, Citation2019).
References
- Abrams, D. B., Glasser, A. M., Pearson, J. L., Villanti, A. C., Collins, L. K., & Niaura, R. S. (2018). Harm minimization and tobacco control: Reframing societal views of nicotine use to rapidly save lives. Annual Review of Public Health, 39, 193–213. https://doi.org/10.1146/annurev-publhealth-040617-013849
- Asparouhov, T., & Muthén, B. (2014). Auxiliary variables in mixture modeling: Three-step approaches using mplus. Structural Equation Modeling: A Multidisciplinary Journal, 21(3), 329–341. https://doi.org/10.1080/10705511.2014.915181
- Asparouhov, T., & Muthén, B. O. (2012). Using mplus TECH11 and TECH14 to test the number of latent classes. Mplus Web Notes, 14, 1–17. https://www.statmodel.com/examples/webnotes/webnote14.pdf
- Beckman, L., Svensson, M., Geidne, S., & Eriksson, C. (2017). Effects on alcohol use of a Swedish school-based prevention program for early adolescents: a longitudinal study. BMC Public Health, 17(1), 1–9. https://doi.org/10.1186/s12889-016-3947-3
- Chiauzzi, E., Dasmahapatra, P., & Black, R. A. (2013). Risk behaviors and drug use: A latent class analysis of heavy episodic drinking in first-year college students. Psychology of Addictive Behaviors: Journal of the Society of Psychologists in Addictive Behaviors, 27(4), 974–985. https://doi.org/10.1037/a0031570
- Choi, H. J., Lu, Y., Schulte, M., & Temple, J. R. (2018). Adolescent substance use: Latent class and transition analysis. Addictive Behaviors, 77, 160–165. https://doi.org/10.1016/j.addbeh.2017.09.022
- Chung, T., Kim, K. H., Hipwell, A. E., & Stepp, S. D. (2013). White and black adolescent females differ in profiles and longitudinal patterns of alcohol, cigarette, and marijuana use. Psychology of Addictive Behaviors: Journal of the Society of Psychologists in Addictive Behaviors, 27(4), 1110–1121. https://doi.org/10.1037/a0031173
- Ciairano, S., Molinengo, G., Bonino, S., Miceli, R., & van Schuur, W. (2009). Age of initiation with different substances and relationships with resources and vulnerabilities: A cross-national study. European Journal of Developmental Psychology, 6(6), 666–684. https://doi.org/10.1080/17405620701626368
- Collins, L. M., & Lanza, S. T. (2003). Latent class and latent transition analysis with applications in the social, behavioral, and health sciences. In D. J. Balding, N. A. C. Cressie, G. M. Fitzmaurice, I. M. Johnstone, G. Molenberghs, D. W. Scott, A. F. M. Smith, R. S. Tsay, S. Weistberg, V. Barnett, J. S. Hunter, & J. L. Teugels (Eds.), Handbook of psychology. John Wiley & Sons, Inc.
- Connor, J. P., Gullo, M. J., Chan, G., Young, R. M. D., Hall, W. D., & Feeney, G. F. X. (2013). Polysubstance use in cannabis users referred for treatment: Drug use profiles, psychiatric comorbidity and cannabis-related beliefs. Frontiers in Psychiatry, 4, 79. https://doi.org/10.3389/fpsyt.2013.00079
- Connor, J. P., Gullo, M. J., White, A., & Kelly, A. B. (2014). Polysubstance use: Diagnostic challenges, patterns of use and health. Current Opinion in Psychiatry, 27(4), 269–275. https://doi.org/10.1097/YCO.0000000000000069
- Conway, K. P., Vullo, G. C., Nichter, B., Wang, J., Compton, W. M., Iannotti, R. J., & Simons-Morton, B. (2013). Prevalence and patterns of polysubstance use in a nationally representative sample of 10th Graders in the United States. The Journal of Adolescent Health: Official Publication of the Society for Adolescent Medicine, 52(6), 716–723. https://doi.org/10.1016/j.jadohealth.2012.12.006
- del Palacio-Gonzalez, A., & Pedersen, M. U. (2022). Youth’s personal relationships, psychological symptoms, and the use of different substances: A population-based study. Nordisk alkohol- & narkotikatidskrift , 39(3), 322–337. https://doi.org/10.1177/14550725211050768
- Dennermalm, N., Karlsson, P., & Ekendahl, M. (2022). Risk factors for substance use in Swedish adolescents: A study across substances and time points. Nordisk alkohol- & narkotikatidskrift , 39(5), 535–552. https://doi.org/10.1177/14550725221108792
- Englund, A. (2019). Skolelevers drogvanor 2019 CAN rapport 187. The Swedish Council for Information on Alcohol and Other Drugs.
- ESPAD. (2019). ESPAD report 2019 results from the European school survey project on alcohol and other drugs. European Monitoring Centre for Drugs and Drug Addiction.
- FRA. (2017). Purchasing and consuming alcohol. European Union For Fundamental Rights. https://fra.europa.eu/sv/publication/2017/mapping-minimum-age-requirements-concerning-rights-child-eu/purchasing-and-consuming-alcohol
- Gobbi, G., Atkin, T., Zytynski, T., Wang, S., Askari, S., Boruff, J., Ware, M., Marmorstein, N., Cipriani, A., Dendukuri, N., & Mayo, N. (2019). Association of cannabis use in adolescence and risk of depression, anxiety, and suicidality in young adulthood: A systematic review and meta-analysis. JAMA Psychiatry, 76(4), 426–434. https://doi.org/10.1001/jamapsychiatry.2018.4500
- Gripe, I. (Ed.) (2021). CAN: s nationella skolundersökning 2021. Ungas erfarenheter av alkohol, narkotika, doping, tobak och spel CAN Rapport 205 [CAN’s national school survery 2021. Experience of alcohol, drugs, tobacco, athletic performance-enhancing drugs and gambling among. The Swedish Council for Information on Alcohol and Other Drugs.
- Guttormsson, U., & Zetterqvist, M. (2019). Det går uppåt i åldrarna - Substansdebut bland skolungdomar 1999–2019. The Swedish Council for Information on Alcohol and Other Drugs.
- Halladay, J., Woock, R., El-Khechen, H., Munn, C., MacKillop, J., Amlung, M., Ogrodnik, M., Favotto, L., Aryal, K., Noori, A., Kiflen, M., & Georgiades, K. (2020). Patterns of substance use among adolescents: A systematic review. Drug and Alcohol Dependence, 216, 108222. https://doi.org/10.1016/j.drugalcdep.2020.108222
- Jackson, P. (2004). Inside clubbing sensual experiments in the art of being human. Berg.
- Karlsson, P. (2006). Margins of Prevention – On Older Adolescents’ Positive and Negative Beliefs about Illicit Drug Use. Stockholm University.
- Karlsson, P., Ekendahl, M., Gripe, I., & Raninen, J. (2018). Individual and school-class correlates of youth cannabis use in Sweden: A multilevel study. Nordisk alkohol- & narkotikatidskrift, 35(2), 131–146. https://doi.org/10.1177/1455072518763426
- Lanza, S. T., Patrick, M. E., & Maggs, J. L. (2010). Latent transition analysis: Benefits of a latent variable approach to modeling transitions in substance use. Journal of Drug Issues, 40(1), 93–120. https://doi.org/10.1177/002204261004000106
- Lanza, S. T., & Rhoades, B. L. (2013). Latent class analysis: An alternative perspective on subgroup analysis in prevention and treatment. Prevention Science: The Official Journal of the Society for Prevention Research, 14(2), 157–168. https://doi.org/10.1007/s11121-011-0201-1
- Lindgren, S. Å. (1993). Den hotfulla njutningen Att etablera drogbruk som samhällsproblem 1870-1970 [Doctoral theses]. SymposionGraduale
- Liskola, J., Haravuori, H., Lindberg, N., Niemelä, S., Karlsson, L., Kiviruusu, O., & Marttunen, M. (2018). AUDIT and AUDIT-C as screening instruments for alcohol problem use in adolescents. Drug and Alcohol Dependence, 188, 266–273. https://doi.org/10.1016/j.drugalcdep.2018.04.015
- Månsson, J., & Ekendahl, M. (2015). Protecting Prohibition. Contemporary Drug Problems, 42(3), 209–225. https://doi.org/10.1177/0091450915599348
- Marshall, E. J. (2014). Adolescent alcohol use: Risks and consequences. Alcohol and Alcoholism (Oxford, Oxfordshire), 49(2), 160–164. https://doi.org/10.1093/alcalc/agt180
- Measham, F., & Shiner, M. (2009). The legacy of “normalisation”: The role of classical and contemporary criminological theory in understanding young people’s drug use. The International Journal on Drug Policy, 20(6), 502–508. https://doi.org/10.1016/j.drugpo.2009.02.001
- Merrin, G. J., Thompson, K., & Leadbeater, B. J. (2018). Transitions in the use of multiple substances from adolescence to young adulthood. Drug and Alcohol Dependence, 189, 147–153. https://doi.org/10.1016/j.drugalcdep.2018.05.015
- Mistry, R., Heinze, J. E., Cordova, D., Heish, H. F., Goldstick, J. E., Ayer, S. M., & Zimmerman, M. A. (2015). Transitions in current substance use from adolescence to early-adulthood. Journal of Youth and Adolescence, 44(10), 1871–1883. https://doi.org/10.1007/s10964-015-0309-x
- Moss, H. B., Chen, C. M., & Yi, H. (2014). Early adolescent patterns of alcohol, cigarettes, and marijuana polysubstance use and young adult substance use outcomes in a nationally representative sample. Drug and Alcohol Dependence, 136(1), 51–62. https://doi.org/10.1016/j.drugalcdep.2013.12.011
- Nylund, K. L., Asparouhov, T., & Muthén, B. O. (2007). Deciding on the number of classes in latent class analysis and growth mixture modeling: A Monte Carlo simulation study. Structural Equation Modeling: A Multidisciplinary Journal, 14(4), 535–569. https://doi.org/10.1080/10705510701575396
- Pape, H., Rossow, I., & Brunborg, G. S. (2018). Adolescents drink less: How, who and why? A review of the recent research literature. Drug and Alcohol Review, 37(1), S98–S114. https://doi.org/10.1111/dar.12695
- Parker, H., Aldridge, J., & Measham, F. (1998). Illegal leisure: The normalization of adolescent recreational drug use. Routledge.
- Poudel, A., & Gautam, S. (2017). Age of onset of substance use and psychosocial problems among individuals with substance use disorders. BMC Psychiatry, 17(1), 10. https://doi.org/10.1186/s12888-016-1191-0
- Raninen, J., Karlsson, P., Svensson, J., Livingston, M., Sjödin, L., & Larm, P. (2022). Reasons not to drink alcohol among 9th graders in Sweden. Substance Use & Misuse, 57(11), 1747–1750. https://doi.org/10.1080/10826084.2022.2102189
- Raninen, J., Livingston, M., Karlsson, P., Leifman, H., Guttormsson, U., Svensson, J., & Larm, P. (2018). One explanation to rule them all? Identifying sub-groups of non-drinking Swedish ninth graders. Drug and Alcohol Review, 37, S42–S48. https://doi.org/10.1111/dar.12663
- Ryoo, J. H., Wang, C., Swearer, S. M., Hull, M., & Shi, D. (2018). Longitudinal model building using latent transition analysis: An example using school bullying data. Frontiers in Psychology, 9(675), 675. https://doi.org/10.3389/fpsyg.2018.00675
- Sjödin, L., Larm, P., Karlsson, P., Livingston, M., & Raninen, J. (2021). Drinking motives and their associations with alcohol use among adolescents in Sweden. Nordisk alkohol- & narkotikatidskrift, 38(3), 256–269. https://doi.org/10.1177/1455072520985974
- Sjödin, L., Livingston, M., Karlsson, P., Larm, P., & Raninen, J. (2022). Associations between trust and drinking among adolescents. Drug and Alcohol Review, 41(1), 221–229. https://doi.org/10.1111/dar.13338
- Sznitman, S. R., Kolobov, T., Bogt, T. t., Kuntsche, E., Walsh, S. D., Boniel-Nissim, M., & Harel-Fisch, Y. (2013). Exploring substance use normalization among adolescents: A multilevel study in 35 countries. Social Science & Medicine (1982), 97, 143–151. https://doi.org/10.1016/j.socscimed.2013.08.038
- Tomczyk, S., Isensee, B., & Hanewinkel, R. (2016a). Latent classes of polysubstance use among adolescents-a systematic review. Drug and Alcohol Dependence, 160, 12–29. https://doi.org/10.1016/j.drugalcdep.2015.11.035
- Tomczyk, S., Pedersen, A., Hanewinkel, R., Isensee, B., & Morgenstern, M. (2016b). Polysubstance use patterns and trajectories in vocational students - A latent transition analysis. Addictive Behaviors, 58, 136–141. https://doi.org/10.1016/j.addbeh.2016.02.027
- Vashishtha, R., Livingston, M., Pennay, A., Dietze, P., MacLean, S., Holmes, J., Herring, R., Caluzzi, G., & Lubman, D. I. (2020). Why is adolescent drinking declining? A systematic review and narrative synthesis. Addiction Research & Theory, 28(4), 275–288. https://doi.org/10.1080/16066359.2019.1663831
- Velicer, W. F., Martin, R. A., & Collins, L. M. (1996). Latent transition analysis for longitudinal data. Addiction, 91(s12), S197–S209. https://doi.org/10.1111/j.1360-0443.1996.tb02339.x
- Yamaguchi, K., & Kandel, D. (1984). Patterns of drug use from adolescence to young adulthood: II. Sequences of progression. American Journal of Public Health, 74(7), 668–672. https://doi.org/10.2105/AJPH.74.7.668
- Zetterqvist, M., & Ramstedt, M. (2019). Tobaksvanor i Sverige 2003–2019. CAN rapport 194 [Tobacco trends in Sweden 2003–2019. CAN report 194]. The Swedish Council for Information on Alcohol and Other Drugs.