Abstract
This work studies O3 pollution for Chon Buri city in the eastern region of Thailand, where O3 has become an increased and serious concern in the last decade. It includes emission estimation and photochemical box modeling in support of investigating the underlying nature of O3 formation over the city and the roles of precursors emitted from sources. The year 2006 was considered and two single-day episodes (January 29 and February 14) were chosen for simulations. It was found that, in the city, the industrial sector is the largest emissions contributor for every O3 precursor (i.e., NOx, non-methane volatile organic compounds or NMVOC, and CO), followed by on-road mobile group. Fugitive NMVOC is relatively large, emitted mainly from oil refineries and tank farms. Simulated results acceptably agree with observations for daily maximum O3 level in both episodes and evidently indicate the VOC-sensitive regime for O3 formation. This regime is also substantiated by morning NMVOC/NOx ratios observed in the city. The corresponding O3 isopleth diagrams suggest NMVOC control alone to lower elevated O3. In seeking a potential O3 control strategy for the city, a combination of brute-force sensitivity tests, an experimental design, statistical modeling, and cost optimization was employed. A number of emission subgroups were found to significantly contribute to O3 formation, based on the February 14 episode, for example, oil refinery (fugitive), tank farm (fugitive), passenger car (gasoline), and motorcycle (gasoline). But the cost-effective strategy suggests control only on the first two subgroups to meet the standard. The cost of implementing the strategy was estimated and found to be small (only 0.2%) compared to the gross provincial product generated by the entire province where the city is located within. These findings could be useful as a needed guideline to support O3 management for the city.
Elevated O3 in the urban and industrial city of Chon Buri needs better understanding of the problem and technical guidelines for its management. With a city-specific emission inventory and air quality modeling, O3 formation was found to be VOC sensitive, and a cost-effective control strategy developed highlights fugitive emissions from the industrial sector to be controlled.
Supplemental Materials: Supplemental materials are available for this paper. Go to the publisher's online edition of the Journal of Air & Waste Management for a list of acronyms, outline of emission estimation, temporal allocation profiles, OZIPR initial and boundary conditions, and comparison of observations and simulated results.
Introduction
Surface ozone (O3) is a secondary pollutant formed in the atmosphere by photochemical reactions associated with its precursors, mainly nitrogen oxides (NO x ), volatile organic compounds (VOC), and carbon monoxide (CO). It is harmful and may have adverse effects on human health and also on plants (CitationColls, 2002). O3 pollution has been studied for cities around the world, for example, in CitationMilford et al. (1989); CitationMolina and Molina (2004); CitationTorres-Jardon and Keener (2006); CitationTang et al. (2008); CitationRan et al. (2009), and CitationOrlando et al. (2010). In Thailand, several cities have experienced high O3 levels (CitationPCD, 2010), and the Pollution Control Department (PCD), Ministry of Natural Resources and Environment, has regulated O3 as a criteria pollutant since 1995. Most city-specific O3 studies have been devoted to Bangkok (the capital of the country) and its vicinity (e.g., CitationKim Oanh and Zhang, 2004; CitationLimpaseni et al., 2003; CitationPCD, 2001). However, continued urbanization and industrialization in Thailand during the past decade have rapidly brought about O3 problems in other cities across the country, and Chon Buri has been a most O3-polluted city. The city is located in the eastern region and is considered an important economic and tourism center of Thailand. It is part of the province of the same name and lies along the coast aligned north–south adjacent to the Gulf of Thailand (). During recent years, the O3 pollution in Chon Buri city has severely intensified and become a great concern by the government and local authorities. According to the series of annual summary reports issued by the PCD (CitationPCD, 2010), the number of hourly exceedances to the national ambient O3 standard (100 ppb, hourly) in 2009 for Chon Buri city doubled (22 hours) that in 2000 and was comparable to (once much less than) that in Bangkok.
Figure 1. Locations of (a) Chon Buri city; (b) modeling domain and monitoring stations. Gray shading in (b) denotes urban, commercial, and industrial areas.
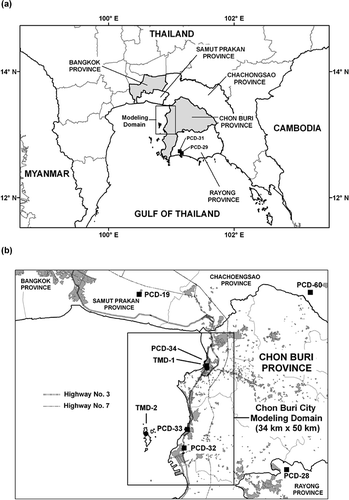
It is commonly known that to properly manage the O3 pollution for a particular city or area requires the knowledge of its precursor emissions and their contributing roles to the problem. O3 can exhibit a nonlinear relationship to NO x and VOC (CitationSillman, 1999), and anthropogenic and biogenic sources can emit various VOC species or groups, each of which may have largely different reactivities to the O3 formation (CitationSeinfeld and Pandis, 1998; CitationAnderson, 2006). Further, the O3 formation is dependent on meteorological conditions (CitationCamalier et al., 2007). These can complicate how O3 is formed and also influence options for its control. To the authors' knowledge, no studies or investigations have been intensively conducted for the O3 pollution in Chon Buri city, and better understanding of the problem is needed to support of air quality management. Given these motivations, this study presents a first dedicated assessment of the O3 pollution for the city, with the aims of examining the relationship of O3 and its precursors emitted from sources based on representatively selected single-day episodes with highly elevated O3 levels and also investigating a potential cost-effective (or least-cost) control strategy to lower peak O3 to be within the standard. The study includes preparation of a city-specific inventory of the O3 precursor's emissions and their processing, photochemical box modeling, and least cost optimization of emission reduction. It is hoped that key findings and perspectives from this study will provide useful guidelines to policymakers and air quality workers for O3 management and help suggest necessary technical areas to be improved for further investigations in the future.
Methods
Study area and observation data
The urban-industrial area defined as Chon Buri city here is geographically located between 13.01°N–13.46°N (latitude) and 100.75°E–101.06°E (longitude) (). Its spatial coverage consists mostly of two major districts (Muang and Sri Racha), of Chon Buri province. The former constitutes most of its upper half, whereas the latter constitutes most of its lower half and is highly industrialized with three industrial estates and one industrial park. There are also several seaports within in the study area, providing services for goods and commodities in the city, and the largest one is Laem Chabang port, which is located in the lower central part of the study area (). In addition, Chon Buri city is regionally known for offering many tourist attractions, including Bang Saen beach. As for Chon Buri province, it is the largest province (in terms of size and population) in the eastern region, with a total land area of 4,363 km2 (CitationChon Buri Provincial Governor's Office, 2011) and a registered population of 1.3 millions (as of 2010) (CitationDOPA, 2011). There are two main highways passing through the city and the province, Highway Nos. 3 and 7 (). The general climate of Chon Buri province is similar to most regions of the country, primarily governed by two monsoons (i.e., northeast and southwest). The northeast monsoon starts in November and ends in mid-March, bringing cool dry air from China. The southeast monsoon starts in mid-May and remains until October, carrying moist air from the Indian Ocean and the Gulf of Thailand to the eastern region and causing plenty of rain.
In the city, three surface air quality and meteorological stations (PCD-32, PCD-33, and PCD-34) are present and routinely operated by the PCD (). Two additional PCD stations (PCD-28 and PCD-60) are also located in nearby provinces. Generally, a PCD station monitors O3, particulate matter (PM), sulfur dioxide (SO2), CO, nitric oxide (NO), nitrogen dioxide (NO2), NO x , total hydrocarbon (THC), methane (CH4), nonmethane hydrocarbon (NMHC), and basic meteorological variables. Observation data from these stations were requested and obtained. In addition, meteorological data from two surface meteorological stations of the Thai Meteorological Department (TMD-1 and TMD-2) in the city were also acquired. Here, we tried to use as many variables relevant to the study from each of these stations as possible unless they contained substantial missing data or were potentially largely influenced by the station's surrounding (e.g., blocked and shadowed by tall buildings and trees) or were considered improper or suspicious (e.g., highly fluctuating or large values that are in contrast with those observed at other nearby stations and not imputable by us). summarizes the information of the monitoring stations and associated monitored variables finally considered. It is noted that the year 2006 was used as the representative year for our O3 study. The primary reason is that this study was planned and begun in 2007–2008, and we were then able to access available data associated with the selected year. Moreover, since O3 is weather dependent, we also checked whether the regional weather pertaining to this year could be considered fairly representative, that is, not highly deviating from typical climatological conditions, especially from interannual variations due to large-scale factors. In the tropics (also Southeast Asia), the most important factor is the El Niño–La Niña–Southern Oscillation (ENSO), and no extreme deviations due to the ENSO occurred in the chosen year (for more information, see http://www.esrl.noaa.gov/psd/enso/mei/rank.html).
Table 1. Monitoring stations and observed variables considered in the study
Estimation of O3 precursor emissions
To support the city-specific study of O3, its precursor emissions were estimated. Here, an anthropogenic inventory of NO x , NMVOC, and CO from six major groups was developed for this area, including industrial facilities (or factories) (i.e., fuel combustion, manufacturing processes, fugitive releases, and seaport), power plants, on-road mobile (both conventional and alternative fuels), nonroad mobile (locomotive only), residential and commercial (liquefied petroleum gas [LPG] consumption, gas service stations, and painting), and waste treatment. These groups were further divided into 36 subgroups (i.e., 34 as anthropogenic and 2 as biogenic) (). For example, the industrial facility group has 17 subgroups according to different types of manufacturing, production, and service. Some of these subgroups (i.e., petrochemical, oil refinery, and tank farm) are related to the petroleum industry, which is also large in Chon Buri city. The power plant group comprises two subgroups (i.e., independent power producer and small power producer) using different fuels in electricity generation (e.g., natural gas, diesel oil, and biofuel). In the waste treatment group, there are two subgroups (solid waste disposal site and wastewater treatment plant). A basic equation to estimate an emission from a source is
Table 2. Estimated annual emissions (tonne) in 2006 over the study domain
For biogenic NMVOC emissions (i.e., released from plants), certain species are known to be reactive to O3 formation (CitationRussell and Dennis, 2000; CitationSillman, 1999). From a recent land cover database (CitationLDD, 2007), many land portions over the study area are covered with crops, forests, and plantations (e.g., rubber, mango, and eucalyptus). Two species were included in the estimation, namely, isoprene and α-pinene (as a dominant monoterpene species). The algorithms in CitationGuenther et al. (1995), the Land Development Department (LDD) land cover data, satellite-based vegetation data, and local meteorological data from the PCD stations () were used. Fires due to open biomass burning are typically minimal in Chon Buri city, which is also the case for 2006 based on satellite data, and their emissions were then not included. Due to the considerable details involved in the estimation process, it is not possible to include them here, and they are instead arranged in Section S1 (Supplemental Information).
In our O3 simulations (using the OZIPR model, discussed later), hourly emissions are required as input over daytime hours. Since the hourly temporal resolution is not possibly resolved in our estimated anthropogenic emissions, it is necessary to scale down the estimated annual emission of each emission subgroup to hourly emissions using a set of representative temporal allocation profiles (TAPs). A TAP represents a time-dependent variation in emission rate, and three TAP time scales used here are monthly, daily, and hourly. With such TAPs, an annual emission can be sequentially allocated to monthly, daily, and hourly emissions. Here, many TAPs were estimated using the obtained activity data, while some were adopted or adapted from CitationPham et al. (2008). In case of no activity data available for a particular time scale, a uniform pattern was assumed. Figure S1 (Supplemental Information) summarizes all nonuniform TAPs assigned to emission subgroups in the study. Apart from the TAPs, NMVOC emitted from each individual emission subgroup needed to be further speciated into lumped carbon species according to the model's chemical mechanism, which is Carbon Bond 4 or CB4 (CitationGery et al., 1989). A number of speciation profiles locally developed (CitationPCD, 2001; CitationSuwattiga and Limpaseni, 2005) for NMVOC from certain anthropogenic emissions were compiled. Some profiles related to biofuels, considered in CitationMilt et al. (2009), were also included. The other profiles were obtained from the SPECIATE database (version 4.2) (CitationU.S. EPA, 2009b). Since the obtained VOC profiles come from different places, many of them contain species incompatible to the defined CB4 lumped species, and they were then rearranged to suit the CB4 application using CitationU.S. EPA (1989a; Citation1989b; Citation2005b). For interested readers, a summary of the VOC profiles finally assigned for all emission groups considered in the study is given in Section S1.
Photochemical box model and simulation setup
The photochemical OZIPR (research-oriented version of ozone isopleth plotting package) model of the U.S. EPA (CitationGery and Crouse, 1990) was used here to assess peak daytime O3 over the study area and how it responds to its precursor emissions in support of developing a cost-effective O3 control strategy. The model was initially developed during late 1980s and early 1990s and was updated occasionally. Although it accounts for many chemical and physical processes in the lower atmosphere, its physical representation is simplified and limited, and only gas-phase chemistry associated with O3 is included. OZIPR has been used as a tool to study O3, generally at a city scale, for example, in CitationJiang et al. (1997); CitationTonnesen (1999); CitationTang et al. (2008); CitationMilt et al. (2009); CitationRan et al. (2009), and CitationOrlando et al. (2010). Two basic modes of simulation available by the model are (1) moving trajectory and (2) city-wide (i.e., stationary box). In the former mode, a well-mixed column of air extending from the ground to the top of the daytime mixed layer moves along with mean horizontal winds. Emissions from sources on the surface are added into the air column when it passes over, and air from above the column is entrained or mixed into the column as the top of mixing height grows during the day. The model also accounts for dry deposition at the surface but in a crude fashion. For the latter mode, a similar concept applies but the air column is fixed (i.e., not moving) and enlarged to cover a wide area or an entire city. This mode is suitable when local winds and emissions are uniform with few variations across the area or when highly spatially resolved meteorology and emissions are not available. The latter condition is the case here. For the used version of the model (downloaded in 2009), Carbon Bond 4 (CB4) (CitationGery et al., 1989) is the chemical mechanism available. It includes nine lumped species of VOC as follows: single-bonded paraffinic carbon atoms (PAR), relatively reactive olefinic double bonds (OLE), slowly reacting olefinic double bonds (ETH), less reactive aromatic compounds (TOL), more reactive aromatic compounds (XYL), formaldehyde (FORM), acetaldehyde and high aldehydes (ALD2), isoprene (ISOP), and nonreactive compounds (NR). In Thailand, many forms of alternative fuels (mainly gasohol, biodiesel, and compressed natural gas [CNG]) have been promoted and used for many years, particularly in the transportation, electricity-generating, and industrial sectors. Here, ethanol (C2H5OH or EtOH) and its associated chemical reactions were added into the original CB4, following CitationMilt el al. (2009).
Our consideration is given only to the dry season, since general meteorological conditions of this season are more favorable for O3 formation than those of the wet season due to high solar radiation, light winds, and minimal rains. For example, in 2006 for Chon Buri city, all O3 exceedance days (i.e., 3 days) and 90% of days with O3 greater than 80 ppb (i.e., 9 out of 10 days) were found in November–February, based on DMaxO3 values averaged over the three PCD stations (PCD-32, PCD-33, and PCD-34) in the study area. Two single-day simulation episodes in 2006 (January 29 and February 14) were representatively chosen. In 2006, highly elevated DMaxO3 (near or greater than 100 ppb) was found for four days (January 28–30 and February 14), and the first episode (January 29) is the middle day of January 28–30. It is noted that the latter episode (February 14) is of most interest and is emphasized here because its DMaxO3 (127 ppb) is the highest observed in 2006, whereas the former episode has a DMaxO3 of 97 ppb (fourth highest of the year). Moreover, February 14 also coincides with Tuesday, representing typical conditions of a working weekday, while January 29 is Sunday, a weekend holiday that has limited commercial and industrial activities. In both episodes, O3 was elevated similarly at all three PCD stations, and the weather conditions are generally fair (i.e., no rains and no solar radiation greatly reduced). Based on a three-day back-trajectory examination using the HYSPLIT model (CitationDraxler and Hess, 1998; http://www.arl.noaa.gov/HYSPLIT.php) (details not shown), the trajectory paths of air mass arriving at the city center in the afternoon of both episodes do not pass through any nearby highly polluted or large cities, that is, Bangkok metropolitan (CitationPCD, 2001) to the northwest of the city, and Rayong (CitationChusai et al., 2012; CitationPhan and Manomaiphiboon, 2012) to the south-southeast. Also, they do not pass through any other large cities in Thailand and neighboring countries. This suggests that O3 development in each episode was not likely to be strongly affected by long-range transport of O3 and its precursors.
As for the modeling domain, its length was set to cover the central district of the province and most major industrial areas along the coast (). We also examined O3 data during 2005–2007 and found high correlations (0.7–0.8) among the three stations. Local recirculation of air (e.g., due to sea breeze) is generally expected over a coastal area under fair weather, which is also the case here for both episodes (i.e., winds being offshore in the morning and turning onshore in later hours). According to this, we used the wind data at PCD-34 to help determine the horizontal extent (in the east–west direction) of the air mass over the study area. Here, the migration path of an air parcel was tracked horizontally, as in CitationPhan and Manomaiphiboon (2012), here from 7:00 a.m. to 5:00 p.m. (which is the peak O3 hour observed in both episodes). The domain's width was given to be moderately large, covering at least 80% of the full paths from both episodes and accounting for such local recirculation to a certain degree. It is noted that other elevated-O3 days in 2006 were also considered at the beginning of the study. However, some do not share the desired characteristics already described, while some do not have the observed air quality/meteorological data as much available over daytime hours as the selected days do.
To run OZIPR, both hourly surface meteorological data (specifically, air temperature, pressure, relative humidity) and initial and final daytime mixing height values are needed. We used the meteorological processor of the advanced air pollutant dispersion model system AERMOD (CitationU.S. EPA, 2004; CitationCimorelli et al., 2005), namely, AERMET, to compute those mixing heights. Before implementing AERMET, certain land-related characteristics of the study area (referenced at around the center of the domain) were prespecified using the AERMOD land-surface preprocessor (namely, AERSURFACE) (CitationU.S. EPA, 2008), together with the LDD land cover data and a 42-yr (1961–2002) daily rain data set (). The latter data were used to specify the relative wetness of the surface in each month/season. AERMET requires both surface meteorological data to drive its surface energy balance module and morning upper-air data for its daytime mixing height calculation in which mixing height increases due to sensible heat transferred from the surface to the atmospheric boundary layer. The configuration and implementation of AERSURFACE and AERMET here follow those in CitationChusai et al. (2012) mostly, with some adjustment to suit our Chon Buri application.
Control strategy development
To develop a cost-effective O3 control strategy for Chon Buri city in this study, it is important to first have a general understanding of O3 formation in the city. Then, attempt to formulate a relationship between DMaxO3 and emissions (here, through simulation experiments and statistical modeling). Lastly, survey and compile information of available control technologies and associated costs, integrate them into the formulated relationship, and perform cost optimization. In doing so, the base case of each selected O3 episode is defined as the typical OZIPR simulation with the original emissions (i.e., without any reduction). A brute-force method was here employed to assess how O3 (i.e., DMaxO3) is sensitive or responds to emissions. This method is practical for the current study because the average run time of our OZIPR simulation for a single-day episode is very small (about a few seconds on a 2-GHz CPU). There are a variety of sensitivity analysis techniques available for and applied to air quality models, and a recent review is given by CitationCohan and Napelenok (2011). An O3 isopleth diagram, which is useful to broadly suggest the underlying nature of O3 formation, can be drawn for each episode using the brute-force technique with different reductions on the original anthropogenic NMVOC and NO x emissions (one species at a time). The O3 formation in the city is shown to be VOC sensitive, suggesting controlling O3 by reducing VOC alone (as opposed to NO x alone or to both NMVOC and NO x together). Here, only anthropogenic NMVOC emissions are to be considered for control.
The next task is to consider and select a set of candidate emission subgroups to be controlled, by examining the following for an individual subgroup: its total NMVOC emitted, O3 sensitivity to NMVOC, and available control technologies and costs. An ideal candidate should have a high O3 sensitivity and a large NMVOC source. In addition, it has feasible NMVOC control technologies suggested. Upon selecting the candidates, a large number of simulation experiments are performed by setting different reductions on their base-case emissions. Results from these simulations are then used to formulate a representative statistical relationship between DMaxO3 (as predictant) and the emissions of selected candidates (as predictors), using response surface methodology or multiple linear regression. The coefficient of each predictor in the established statistical relationship represents the aggregate (or global) sensitivity of DMaxO3, that is, how much DMaxO3 decreases due to unit emission reduced. With the knowledge of the aggregate sensitivities for all candidates, cost minimization with linear programming (here, a simplex method) (CitationNelder and Mead, 1965; CitationPress et al., 1996) can be used in a straightforward fashion to quantify the least cost and its associated emission reductions for all individual candidates. The cost minimization can be mathematically formulated as follows:
Results and Discussion
From the emission estimation, total anthropogenic emissions in Chon Buri province are dominated by those from Chon Buri city (or the study area), that is, 67% for NO x (as NO2), 57% for NMVOC, and 54% for CO, respectively (), despite the fact that the study area comprises only about 17% of the province in terms of land area. Anthropogenic NMVOC contributes 49% to total NMVOC (anthropogenic + biogenic) (34,547 t). The largest anthropogenic NMVOC-emitted subgroups are oil refinery (fugitive) (46% of total anthropogenic NMVOC), tank farm (fugitive) (21%), and motorcycle (9%). Notice that the combined fugitive emissions from the first two subgroups contribute most of the total anthropogenic NMVOC emitted. Biogenic NMVOC is also large (51% total NMVOC), with isoprene being dominant. Total annual NO x is 21,016 t, to which industrial facility, on-road mobile, and power plant groups contribute 52%, 28%, and 20%, respectively. In the industrial facility group, oil refinery (combustion) contributes most (34%). In the on-road mobile group, the heavy-duty diesel vehicle contributes most (21%). For CO, its total annual value is 33,125 t, to which industrial facility and on-road mobile groups contribute most again (61% and 35%, respectively). For a seaport, only Laem Chabang port was included but its contribution was comparatively small (<0.5%) to total emission in every species. Fugitive NMVOC emissions from oil refinery and tank farm subgroups combined were found to be large (68% of total anthropogenic).
In the simulation of each selected episode, the estimated annual emissions were scaled down to hourly emissions (7:00 a.m. to 7:00 p.m.) using the assigned TAPs. It was found that the total anthropogenic emissions (from all daytime hours) in the February 14 episode are larger than those in the January 29 episode (e.g., 1.7 times for NMVOC and 2 times for NO x ) as expected since the January 29 episode coincides with Sunday (a weekend holiday) and the latter episode is Tuesday (a working weekday). After running the simulations, their results were evaluated against observations. It is seen from that the model is acceptably capable of predicting DMaxO3 and its hour of occurrence in both episodes. As recommended in the OZIPR User Manual (CitationU.S. EPA, 1989b), simulated DMaxO3 should be within 30% of observed DMaxO3, which is the case here (6.2 ppb or 6% on January 29 and 0.6 ppb or <1% on February 14). O3 tends to be underestimated on January 29 with MB (mean bias, over all simulated hours) = −7.5 ppb, but overestimated on February 14 with MB = 11.9 ppb. For comparison of other species, see Section S4.
The O3 isopleth diagram corresponding to each episode () was developed by means of a series of OZIPR runs with different reductions on the base-case emissions of NO x and NMVOC. In both episodes, the isopleths diagrams clearly indicate that O3 formation in the city is in a VOC-sensitive (or NO x -saturated) regime where O3 increases with decreasing NO x but decreases with decreasing VOC. Many studies also reported this regime over different cities (especially their central parts) (CitationMilford et al., 1989; CitationSillman, 1999, and references therein; CitationKim Oanh and Zhang, 2004; CitationPCD, 2001; CitationRan et al., 2009; CitationTorres-Jardon and Keener, 2006). CitationSillman (1999) pointed to a simple rule to suggest the underlying regime of O3 formation is to consider morning VOC/NO x ratios, <10 as VOC sensitive and >20 as NO x sensitive. In the VOC-sensitive regime, the rate of O3 formation is primarily regulated by the reaction of hydrocarbon (VOC) and hydroxyl radical (OH), while in the other regime, the formation is regulated by the reaction of alkylperoxy radical (RO2) and hydroperoxy radical (HO2) with NO and its rate increases with increasing NO x (CitationSillman, 1999). To examine the preceding rule, observed NO x and NMVOC concentrations during 6:00–9:00 a.m. in the dry seasons of 2005–2007 at the three PCD stations (i.e., PCD-32, PCD-33, and PCD-34) in the study area were plotted against each other (), showing an NMHC/NO x ratio of 8.4 as the slope of linear regression (p-value < 0.01) (here, equivalent to a NMVOC/NO x ratio of 8.8) and lending additional support to that O3 formation over the city is VOC sensitive. At the PCD stations, NMHC (as opposed to NMVOC) was measured using a flame ionization detection method, and a factor of 1.05 was used to convert it to NMVOC to account for undetected oxygenates (e.g., aldehydes and ketone), as recommended by CitationU.S. EPA (1989b, p. 33).
Figure 3. O3 isopleths as a function of percentages of anthropogenic NMVOC and NO x emission reduction. The base case of emissions in each episode is noted in the upper right corner of each diagram.
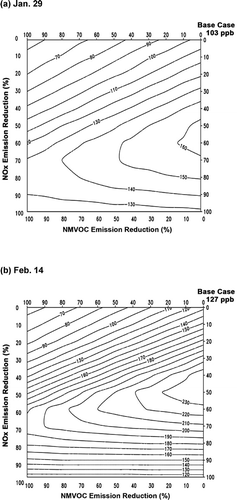
Figure 4. Morning NMHC and NO x levels observed at three PCD stations for all days during the dry seasons of 2005–2007. The solid diamonds denote the all-station-average values for the selected episodes.
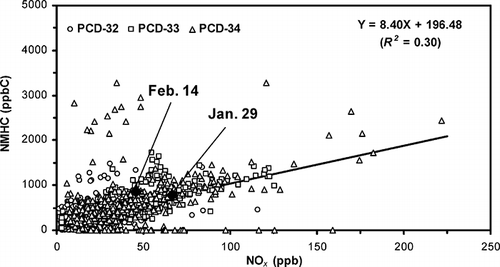
Cost-effective control strategy
According to the preceding results, practical O3 management by NMVOC control alone is suggested for Chon Buri city. In the current case, any slight to moderate NO x control (e.g., <50% reduction in the February 14 episode) would undesirably increase O3. Here, cost-effective control strategy development is based on the February 14 episode as the reference case because this episode represents the worst situation in 2006, while the January 29 episode is complementary in assessing the nature of O3 formation, and O3 observed on that day, though elevated, does not exceed the standard. From , a large NO x reduction alone or a combination with NO x and NMVOC reduction would possibly not lower O3 to meet the standard, whereas an NMVOC reduction of about 30% (applied to all anthropogenic sources of NMVOC) would do. However, such an across-the-board strategy is generally not practical since cost-effectiveness and control technologies need to be considered. It is also worth mentioning that the isopleths diagrams of both episodes show that 100% reductions on both emissions still cannot lower O3 to meet the standard, and a possible explanation for this is the presence of biogenic emissions and nonzero initial and boundary conditions specified in the modeling.
To specify a potential set of candidate emission subgroups to be controlled, the procedure described in the Method section was followed. We began with running a brute-force DMaxO3 sensitivity test for every emission subgroup with nonnegligible emitted NMVOC, using a 10% reduction on the base-case emitted NMVOC as guideline. The results from the tests helped identify five major subgroups with the highest sensitivities in terms of |ΔDMaxO3| due to 10% reduction (here, ≥0.5 ppb), which are (1) oil refinery (fugitive), (2) tank farm (fugitive), (3) passenger car (gasoline), (4) motorcycle (gasoline), and (5) heavy-duty diesel vehicle, with the highest sensitivities found in (1) and (2) (4.2 and 2.1 ppb, respectively). In fact, there are some other emission subgroups with relatively high sensitivities on a per-unit emission basis but their total NMVOC emissions are not substantial. Furthermore, a set of 10% brute-force tests was conducted for the January 29 episode, and agreement was found in that the preceding subgroups were also the major subgroups with the highest sensitivities due to 10% reduction. Next, feasible NMVOC control technologies and their associated costs for the selected candidates were taken into account. A summary of the control technologies and costs for NMVOC representatively used in the study is given in . Their selection are based directly on surveyed information in the literature, mainly CitationAmann et al. (2008) and CitationU.S. EPA (2006), and on technical opinions solicited from two local refinery technicians and a local environmental officer with intensive experience in automobile pollution control. In the table, the heavy-duty diesel vehicle subgroup was not included as a candidate because its control technologies generally emphasize particulate matter and NO x as major pollutants, and no costs for NMVOC control are explicitly suggested according to our literature review. Each cost value shown in the table is of a levelized cost, that is, a total cost incurred from purchase, installation, operation, and maintenance of a technology over its lifetime.
Table 3. NMVOC control technologies and their associated costs
To determine the O3 sensitivities to emissions for the selected four candidates, in total 2,401 (= 74) OZIPR simulations were run, which combine 4 candidates and 7 reduction levels (0%, 10%, 20%, 30%, 40%, 60%, and 80%). Simulated results from these simulations were used to establish a representative statistical model. Here, both response surface methodology and multiple linear regression were considered. The latter, containing only first-order terms, turns out to account for the overall variability of data adequately (R 2 > 0.99) () without any inclusion of second-order and cross terms as in the former approach, which is also expected because the O3 formation falls in the VOC-sensitive regime and the O3 response to NMVOC tends to be approximately linear as suggested by the isopleth diagram (). Given and the knowledge of the estimated emissions, a cost minimization problem was simply set () and numerically solved. To meet the standard with the least cost, oil refinery (fugitive) and tank farm (fugitive) subgroups are required to have 35% and 77% reductions of NMVOC, respectively, whereas no reductions are required for the other subgroups. Notice that the coefficients in the constraint equation are comparable in terms of magnitude (∼3 ppb t−1) among all candidates. Thus, no emission reductions are assigned to passenger car (gasoline) and motorcycle (gasoline) subgroups due to relatively high per-unit-emission costs and relatively low applicable control efficiencies. In terms of annualized levelized cost, the control strategy developed requires a total of 883 MBt (million Bahts) (equivalent to 32.4 MBt ppb−1 O3 lowered), most of which (855 MBt) will be spent on the tank farm. Relative to the overall economic values generated by Chon Buri province in 2006, this amount is quite small, representing only 0.2% of gross provincial product (GPP) and 0.4% of GPP due to industrial-manufacturing activities (CitationNESDB, 2011). The definition of GPP is similar to that of gross domestic product (GDP) but scaled to a province (as opposed to a country).
Table 4. Cost equations and constraints applied in the least-cost optimization based on the February 14 episode as the reference case
Conclusions
A city-specific study on O3 pollution was conducted for Chon Buri, where O3 has become of increased concern over the past decade. It covers anthropogenic and biogenic emission estimation and processing, photochemical box simulations using the OZIPR model, and cost optimization, and the year 2006 was chosen for investigation. The study consists of the following three components: (1) emissions estimation, (2) O3 modeling, and (3) development of a potential control strategy, inferred from the selected two single-day simulation episodes (January 29 and February 14) representing high O3 days in the typical O3 (i.e., dry) season. In the study, an effort to understand the O3 pollution in support of air quality management in Chon Buri city was made. It is hoped that both the technical approaches demonstrated here and the findings can offer needed groundwork to air quality workers, policymakers, and stakeholders for addressing O3-related issues for the city. The important findings from the study and our comments and recommendations are given as follows:
1. | For anthropogenic emissions, Chon Buri city accounts for most emissions within the entire province, for example, 67% for NO x and 57% for NMVOC. For all precursor species, the industrial sector is the largest contributor, followed by the on-road mobile group. The two largest NMVOC emitters pertain to fugitive emissions, which are oil refinery (fugitive) and tank farm (fugitive). The largest NO x emitter is also from the industrial sector. It must be admitted that uncertainty exist in various processes of our emission estimation. Although this task was carefully done, several assumptions were necessarily used to help overcome technical problems often associated with the limitation of data. According to our experience from the study, fugitive emissions from oil refineries and tank farms may contain relatively large uncertainty due to great difficulty in having source-specific data in adequate details. | ||||
2. | The OZIPR setup and simulations for both episodes show acceptable performance in predicting DMaxO3 and fairly capture the daytime evolution of O3 and its precursors. The simulated results evidently show the VOC-sensitive nature of O3 formation, which is also well substantiated by morning NMVOC/NO x ratios observed at the three air-quality monitoring stations located in the city. The O3 isopleths corresponding to both episodes suggest NMVOC control alone (as opposed to NO x control alone or in combination with NO x ) is required to meet the O3 standard. | ||||
3. | Through brute-force sensitivity tests, selection of emission subgroups to be controlled, and an experimental design of OZIPR simulations, a relationship between DMaxO3 and emissions was formulated using multiple linear regression. Important NMVOC sources were identified as significant contributors to elevated O3 in the reference episode (here, February 14), mainly fugitive emissions from the industrial sector and vehicular emissions. The least-cost optimization on the obtained relationship suggests only oil refinery (fugitive) and tank farm (fugitive) subgroups to be required for NMVOC reductions to meet the O3 standard. The corresponding total annualized levelized cost due to this control strategy is 883 MBt (bias-adjusted cost to the year 2006 basis). Given the control strategy pointing to fugitive emissions as the most important contributor, we caution against using the control strategy rigidly and also the emission estimates as being accurate but encourage using them as a first identification or approximation that could be further refined or subject to closer examination in the future. |
uawm_a_716385_sup_28551521.doc
Download MS Word (2.1 MB)uawm_a_716385_sup_29985075.zip
Download Zip (1.6 MB)Acknowledgments
The authors sincerely thank the Pollution Control Department for the air quality and meteorological data, the Thai Meteorological Department for the meteorological data, and the US Environmental Protection Agency for the OZIPR model and the emission software used in the study. We thank Dr. Larry Anderson (University of Colorado Denver) for his useful comments given to the study. The kind provision of technical information and suggestions from local organizations and local experts is acknowledged: Department of Industrial Works, the Regional Environmental Office 13 (Chon Buri), Department of Alternative Energy Development and Efficiency, Department of Highways, Land Development Department, Mr. Nawarat Mitjit (PCD), and Mr. Ittipol Pawarmart (PCD). We also thank members at the JGSEE Computational Laboratory (Bang Khun Tien Campus) for their general assistance. This study was financially supported by the Joint Graduate School of Energy and Environment and the Thailand Research Fund under grant RDG5050016, and in part by the National Research Council of Thailand under grant POR-KOR 2550-46, the Postgraduate Education and Research Development Office (Ministry of Education) under grant JGSEE/PROJECT/002-2011, and the Asahi Glass Foundation.
References
- Amann, M., I. Bertok, J. Borken, A. Chambers, J. Cofala, F. Dentener, C. Heyes, L. Hoglund, Z. Klimont, P. Purohit, P. Rafaj, W. Schöpp, E. Texeira, G. Toth, F. Wagner, and W. Winiwarter. 2008. GAINS-ASIA. Methodology Report. Published by the International Institute for Applied Systems Analysis (IIASA), Laxenburg, Austria http://gains.iiasa.ac.at/index.php/gains-asia (http://gains.iiasa.ac.at/index.php/gains-asia) (Accessed: 21 June 2011 ).
- Anderson , L.G. 2006 . “ Atmospheric chemistry ” . In Encyclopedia of Environmental Science and Engineering Volume I , 5th , Edited by: Pfafflin , J.R. and Ziegler , E.N. Boca Raton , FL : CRC Press .
- Camalier , L. , Cox , W. and Dolwick , P. 2007 . The effects of meteorology on ozone in urban areas and their use in assessing ozone trends . Atmos. Environ , 41 : 7127 – 7137 . doi: 10.1016/j.atmosenv.2007.04.061
- Chon Buri Provincial Governor's Office. 2011. Chon Buri Information, Ministry of Interior, Thailand http://www.chonburi.go.th (http://www.chonburi.go.th) (Accessed: 10 August 2011 ).
- Chusai , C. , Manomaiphiboon , K. , Saiyasitpanich , P. and Thepanondh , S. 2012 . NO2 and SO2 dispersion modeling and relative roles of emission sources over Map Ta Phut Industrial Area, Thailand . J. Air & Waste Manage. Assoc , 62 : 932 – 945 . doi: 10.1080/10962247.2012.687704
- Cimorelli , A.J. , Perry , S.G. , Venkatram , A. , Weil , J.C. , Paine , R.J. , Wilson , R.B. , Lee , R.F. , Peters , W.D. and Brode , R.W. 2005 . AERMOD: A dispersion model for industrial source applications. Part I: General model formulation and boundary layer characterization . J. Appl. Meteorol , 44 : 682 – 693 . doi: 10.1175/JAM2227.1
- Cohan , D.S. and Napelenok , S.L. 2011 . Air quality response modeling for decision support . Atmosphere , 2 : 407 – 425 . doi: 10.3390/atmos2030407
- Colls , J. 2002 . Air Pollution , 2nd , New York : SPON Press, Taylor & Francis .
- DOPA, 2011. Population Data. Department of Provincial Administration, Ministry of Interior, Thailand http://www.dopa.go.th (http://www.dopa.go.th) (Accessed: 21 September 2011 ).
- Draxler , R.R. and Hess , G.D. 1998 . An overview of the HYSPLIT 4 modelling system for trajectories, dispersion, and deposition . Aust. Meteorol. Mag , 47 : 295 – 308 .
- Gery, M.W., and R.R. Crouse. 1990. User's Guide for Executing OZIPR. U.S. EPA, Office of Air Quality Planning and Standards, Research Triangle Park, NC http://www.epa.gov/scram001/userg/other/ozipr.pdf (http://www.epa.gov/scram001/userg/other/ozipr.pdf) (Accessed: 31 August 2008 ).
- Gery , M.W. , Whitten , G.Z. , Killus , J.P. and Dodge , M.C. 1989 . A photochemical kinetics mechanism for urban and regional scale computer modeling . J. Geophys. Res , 94 ( D10 ) : 12925 – 12956 . doi: 10.1029/JD094iD10p12925
- Guenther , A. , Hewitt , C.N. , Erickson , D. , Fall , R. , Geron , C. , Graedel , T. , Harley , P. , Klinger , L. , Lerdau , M. , Mckay , W.A. , Pierce , T. , Scholes , B. , Steinbrecher , R. , Tallamraju , R. , Taylor , J. and Zimmerman , P. 1995 . A global model of natural volatile organic compound emissions . J. Geophys. Res , 100 ( D5 ) : 8873 – 8892 . doi: 10.1029/94JD02950
- Jiang , W. , Singleton , D.L. , Hedley , M. and McLaren , R. 1997 . Sensitivity of ozone concentrations to VOC and NO x emissions in the Canadian Lower Fraser Valley . Atmos. Environ , 31 : 627 – 638 . doi: 10.1016/S1352-2310(96)00284-1
- Kim Oanh , N.T. and Zhang , B. 2004 . Photochemical smog modeling for assessment of potential impacts of different management strategies on air quality of the Bangkok Metropolitan Region, Thailand . J. Air Waste Manage. Assoc , 54 : 1321 – 1338 . doi: 10.1080/10473289.2004.10470996
- LDD . 2007 . Land Use and Land Cover Data for Thailand for the Years 2006–2007 , Thailand : CD-ROM product. Bangkok: Land Development Department, Ministry of Agriculture and Cooperatives .
- Limpaseni , W. , Weeks , I.A. , Galbally , I.E. , Hooper , M.A. , Kivlighon , L.M. , Suwattiga , P. and Chuenarrom , A. Paper presented at 2nd Regional Conference on Energy Technology Towards a Clean Environment . Hydrocarbons related to ozone air pollution problems in Bangkok , Phuket , , Thailand
- Milford , J.B. , Russell , A.G. and McRae , G.J. 1989 . A new approach to photochemical pollution control: Implications of spatial patterns in pollutant responses to reduction in nitrogen oxides and reactive organic gas emissions . Environ. Sci. Technol , 23 : 1290 – 1301 . doi: 10.1021/es00068a017
- Milt , A. , Milano , A. , Garivait , S. and Kamens , R. 2009 . Effects of 10% biofuel substitution on ground level ozone formation in Bangkok, Thailand . Atmos. Environ , 43 : 5962 – 5970 . doi: 10.1016/j.atmosenv.2009.07.062
- Molina , M.J. and Molina , L.T. 2004 . Megacities and atmospheric pollution . J. Air Waste Manage. Assoc , 54 : 644 – 680 . doi: 10.1080/10473289.2004.10470936
- Nelder , J.A. and Mead , R.A. 1965 . Simplex method for function minimization . Comp. J , 7 : 308 – 313 .
- NESDB, 2011. Gross Regional and Provincial Product in 2009. Bangkok: Office of the National Economic and Social Development, The Prime Minister's Office, Thailand http://www.nesdb.go.th/Default.aspx?tabid=96 (http://www.nesdb.go.th/Default.aspx?tabid=96) (Accessed: 22 March 2012 ).
- Orlando , J.P. , Alvim , D.S. , Yamazaki , A. , Corrêa , S.M. and Gatti , L.V. 2010 . Ozone precursors for the São Paulo Metropolitan Area . Sci. Total Environ , 408 : 1612 – 1620 . doi: 10.1016/j.scitotenv.2009.11.060
- PCD . 2001 . Investigation and Analysis of Ozone Precursors for the Mitigation of Photochemical Air Pollution in Bangkok , Vol. 4 , Bangkok , , Thailand : PCD03-040. Prepared by Air & Waste Technology Co., Ltd .
- PCD. 2008. Measurement of Air Pollution from Gasohol-Fueled Vehicles. Final Report (in Thai). PCD03-095. Bureau of Air Quality and Noise Management, Bangkok, Thailand http://infofile.pcd.go.th/air/gasohol.pdf (http://infofile.pcd.go.th/air/gasohol.pdf) (Accessed: 20 May 2011 ).
- PCD. 2010. State and Management of Air and Noise Pollution Problems 2009. Annual Report, Bureau of Air Quality and Noise Management, Bangkok, Thailand. (annual reports published for other years are also available online) http://www.pcd.go.th/public/Publications/en_print_report.cfm (http://www.pcd.go.th/public/Publications/en_print_report.cfm)
- Pengpis , J. 2010 . A Comparative Experiment of Exhaust Emission between Compressed Natural Gas and Gasohol Fuel in Gasoline Car (in Thai) , Bangkok , , Thailand : Automotive Emissions Laboratory, Bureau of Air Quality and Noise Management, Pollution Control Department .
- Phan , T.T. and Manomaiphiboon , K. 2012 . Observed and simulated sea breeze characteristics over Rayong coastal area, Thailand . Meteorol. Atmos. Phys , 116 : 95 – 111 . doi: 10.1007/s00703-012-0185-9
- Pham , T.B.T. , Manomaiphiboon , K. and Vongmahadlek , C. 2008 . Development of an inventory and temporal allocation profiles of emissions from power plants and industrial facilities in Thailand . Sci. Total Environ , 397 : 103 – 118 . doi: 10.1016/j.scitotenv.2008.01.066
- Press, W.H., S.A. Teukolsky, W.T. Vetterling, and B.P. Flannery. 1996. Numerical Recipes in FORTRAN 90. 2nd ed. New York, NY: Cambridge University Press http://apps.nrbook.com/fortran/index.html (http://apps.nrbook.com/fortran/index.html) (Accessed: 20 May 2011 ).
- Ran , L. , Zhao , C. , Geng , F. , Tie , X. , Tang , X. , Peng , L. , Zhou , G. , Yu , Q. , Xu , J. and Guenther , A. 2009 . Ozone photochemical production in urban Shanghai, China: Analysis based on ground level observations . J. Geophys. Res , 114 : D15301 doi: 10.1029/2008JD010752
- Russell , A. and Dennis , R. 2000 . NARSTO critical review of photochemical models and modeling . Atmos. Environ , 34 : 2283 – 2324 . doi: 10.1016/S1352-2310(99)00468-9
- Seinfeld , J.H. and Pandis , S.N. 1998 . Atmospheric Chemistry and Physics: From Air Pollution to Climate Change , New York, NY : John Wiley & Sons .
- Sillman , S. 1999 . The relation between ozone, NO x and hydrocarbons in urban and polluted rural environments . Atmos. Environ , 33 : 1821 – 1845 . doi: 10.1016/S1352-2310(98)00345-8
- Suwattiga , P. and Limpaseni , W. 2005 . Seasonal source apportionment of volatile organic compounds in Bangkok ambient air . Sci. Asia , 31 : 395 – 401 . doi: 10.2306/scienceasia1513-1874.2005.31.395
- Tang , W.Y. , Zhao , C.S. , Geng , F.H. , Peng , L. , Zhou , G.Q. , Gao , W. , Xu , J.M. and Tie , X.X. 2008 . Study of ozone “weekend effect” in Shanghai . Sci. China, Ser. D Earth Sci , 51 : 1354 – 1360 . doi: 10.1007/s11430-008-0088-2
- Tonnesen , G.S. 1999 . Effects of uncertainty in the reaction of the hydroxyl radical with nitrogen dioxide on model-simulated ozone control strategies . Atmos. Environ , 33 : 1587 – 1598 . doi: 10.1016/S1352-2310(98)00375-6
- Torres-Jardon , R. and Keener , T.C. 2006 . Evaluation of ozone–nitrogen oxides–volatile organic compound sensitivity of Cincinnati, Ohio . J. Air Waste Manage. Assoc , 56 : 322 – 333 . doi: 10.1080/10473289.2006.10464459
- U.S. Environmental Protection Agency . 1989a . User's Manual for OZIPM-4 (Ozone Isopleth Plotting with Optimal Mechanisms) , Vol. 1 , Research Triangle Park , NC : Office of Air Quality Planning and Standards, Research . VolumeEPA-450/4-89-009a
- U.S. Environmental Protection Agency . 1989b . Procedures for Applying City-Specific EKMA , EPA-450/4-89-012. www.epa.gov/scram001/userg/regmod/ekmacity.pdf (accessed February 10, 2008)
- U.S. Environmental Protection Agency. 1999. User's Manual for TANKS (Version 4.0) http://www.epa.gov/ttnchie1/software/tanks/tank4man.pdf (http://www.epa.gov/ttnchie1/software/tanks/tank4man.pdf) (Accessed: 10 November 2010 ).
- U.S. Environmental Protection Agency . 2004 . User's Guide for the AERMOD Meteorological Preprocessor (AERMET) , EPA-454/B-03-002. www.epa.gov/scram001/7thconf/aermod/aermetugb.pdf (accessed May 10, 2009)
- U.S. Environmental Protection Agency . 2005a . Landfill Gas Emissions Model (LandGEM) Version 3.02 User's Guide , EPA-600/R-05/047. www.epa.gov/ttncatcl/dir1/landgem-v302-guide.pdf (accessed June 20, 2009)
- U.S. Environmental Protection Agency. 2005b. Updates to the Carbon Bond Chemical Mechanism: CB05. Prepared by G. Yarwood, S. Rao, M. Yocke, G.Z. Whitten, and S. Reyes http://www.camx.com/publ/pdfs/CB05_Final_Report_120805.pdf (http://www.camx.com/publ/pdfs/CB05_Final_Report_120805.pdf) (Accessed: 20 May 2010 ).
- U.S. Environmental Protection Agency. 2006. AirControlNET Database (Version 4.1). Documentation Report http://www.epa.gov/ttnecas1/models/DevelopmentReport.pdf (http://www.epa.gov/ttnecas1/models/DevelopmentReport.pdf) (Accessed: 20 May 2010 ).
- U.S. Environmental Protection Agency . 2008 . AERSURFACE User's Guide , EPA-454/B-08-001. www.epa.gov/scram001/7thconf/aermod/aersurface_ userguide.pdf (accessed May 10, 2009)
- U.S. Environmental Protection Agency. 2009a. Current Methodologies in Preparing Mobile Source Port-Related Emission Inventories. Final Report. Prepared by ICF International http://www.epa.gov/sectors/sectorinfo/sectorprofiles/ports/ports-emission-inv-april09.pdf (http://www.epa.gov/sectors/sectorinfo/sectorprofiles/ports/ports-emission-inv-april09.pdf) (Accessed: 31 August 2010 ).
- U.S. Environmental Protection Agency . 2009b . SPECIATE 4.2. Speciation Database Development Documentation , EPA/600-R-09/038. http://nepis.epa.gov/Exe/ZyPURL.cgi?Dockey=P1004489.txt (accessed January 20, 2009)
- Vallack, H.W., and K. Rypdal. 2007. The Global Atmospheric Pollution Forum Air Pollutant Emissions Inventory Manual, February 2007. York, UK: Stockholm Environmental Institute at York, University of York http://www.sei-international.org/rapidc/gapforum/html/emissions-manual.php (http://www.sei-international.org/rapidc/gapforum/html/emissions-manual.php) (Accessed: 30 September 2008 ).