Abstract
A six-day tunnel field study was conducted in the city of Monterrey, Mexico, during June 2009 to derive on-road emission factors (EFs) for trace gases and fine particulate matter from the local vehicle fleet. The Loma Larga Tunnel (LLT) is a 532-m-long structure that is mainly used by light-duty gasoline-powered vehicles. It is composed of two independent bores that have a semicircular cross section, 17 m in diameter with a 3.5% slope. During the study, a fleet of 108,569 vehicles with average speeds that ranged from 43 to 76 km/hr was sampled. Ambient air samples were taken inside each bore using 6-L SUMMA-polished canisters and low-volume samplers for the quantification of total nonmethane hydrocarbons (TNMHC) and PM2.5, respectively. The effect of road dust resuspension was considered in the computation of PM2.5 EFs. Additional equipment was used to measure real-time levels of CO2 and NOx; CO EFs were estimated using NOx as a surrogate. TNMHC samples and NOx levels were obtained for 2-hr time periods, while PM2.5 samples and CO2 levels were obtained using 2.5-hr time periods, which included the time periods of the TNMHC and NOx measurements. Estimated EFs for TNMHC, CO, NOx, and PM2.5 were 1.16 ± 0.05, 4.83 ± 2.9, and 0.11 ± 0.07 g/km-veh (2-hr average) and 17.5 ± 5.7 mg/veh-km (2.5-hr average), respectively, while CO2 EFs were 182.7 ± 44 g/km-veh for the 2-hr time periods and 170 ± 22 g/veh-km for the 2.5-hr time periods. The average fuel economy estimated from the field data was 12.3 ± 2.3 km/L. The CO2 and TNMHC EFs (on a mass per distance basis) tended to be higher for traffic moving upslope, while the inverse occurred for the PM2.5 EFs. In comparison to other tunnel studies, the CO2 EFs obtained were similar, the NOx and PM2.5 EFs were lower, and the CO and TNMHC EFs were higher.
Mobile source emission factors (EFs) for Mexican cities other than Mexico City are scarce. In Monterrey, Mexico, one of the three major cities in the country, emissions inventories are constructed based on EFs from other locations. However, it is quite relevant to obtain local information to construct reliable inventories. We present what is, to our knowledge, the first tunnel study conducted in a Mexican city other than Mexico City to estimate fleet-average mobile source EFs. This is also the first study that reports PM2.5 EFs derived from a tunnel study in the country.
Introduction
The use of vehicles with internal combustion engines has increased significantly in Mexico during the last few years, particularly in urban areas. For example, in the Monterrey Metropolitan Area (MMA), the third largest urban center in the country (), the number of vehicles that comprise the official vehicle fleet doubled from 1999 to 2005. This has had important environmental implications. On average, mobile sources represent the largest contribution of pollutants emitted to the atmosphere by anthropogenic sources in the country. According to the 2005 official emissions inventory for the MMA (CitationGENL and SEMARNAT, 2008), mobile sources contributed that year 96.5% of the CO, 47.5% of the NOx (NOx = NO + NO2), 47.2% of the volatile organic compounds (VOCs), 3.1% of the PM2.5 (particulate matter with an aerodynamic diameter under 2.5 μm), and 1.5% of the SO2 emitted.
Figure 1. Location of the MMA at the national and state level: shaded in the left panel is the State of Nuevo León and in the right panel all the municipalities that form part of the MMA.
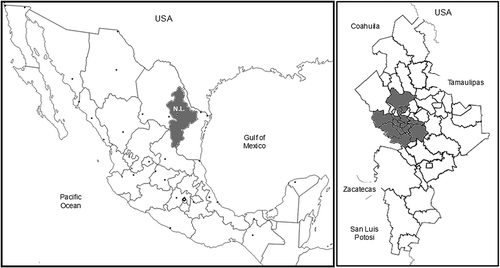
Of the pollutants emitted by gasoline-powered vehicles, PM2.5 and gas-phase emissions, including CO2, NOx, and nonmethane hydrocarbons, are of particular interest due to the environmental and health impacts associated with their release into the atmosphere. For example, some non-methane hydrocarbons can cause serious health problems, including memory loss and irritation of the respiratory tract, while others are well-known carcinogens (CitationDales et al., 2008; CitationOhura et al., 2009). In addition, VOCs and NOx, in the presence of sunlight, are precursors of ozone and secondary organic aerosol.
CO2 emissions from mobile sources are also significant. These emissions are produced by the burning of fossil fuels, of which gasoline, diesel, and natural gas represent major contributors. From 1990 to 2006, the CO2-equivalent emissions in Mexico increased 38%. In 2006, the greatest contribution of CO2-equivalent emissions came from transportation and electrical energy production. The transportation sector accounted for 27.1% of the total CO2-equivalent emissions, of which 66.8% came from mobile sources that use gasoline as fuel (CitationINE and SEMARNAT, 2009).
Although CO, NOx, VOCs, and CO2 seem to be the main emissions from mobile sources, some studies have determined that in the United States, on average, 38% of the observed PM2.5 emissions come from vehicular combustion and 74% of these are produced from gasoline-powered light-duty vehicles (CitationGertler, 2005). Also, these aerosol particles have been related to cardiovascular and respiratory diseases, climate, and visibility effects (CitationDíaz and Domínguez, 2009).
Even though mobile sources have been identified as major contributors to air quality problems in Mexican urban centers (e.g., CitationVelasco et al., 2007), few studies outside Mexico City have been conducted to characterize such emissions in detail. Therefore, given the high contribution of vehicle emissions in urban centers, it is relevant to characterize these emissions. Moreover, given that each region has its own characteristic vehicle fleet and particular conditions that influence vehicular emissions (transportation infrastructure, fuel quality, pavement conditions, climate, driving behavior, etc.), it is important to conduct local studies to obtain local emission factors (EFs). However, it is difficult to estimate fleet-average emissions from single-vehicle laboratory experiments (such as chassis dynamometer tests) because of the differences in vehicle type, fuel, engine conditions, efficiency, and road and driving conditions, and the limited number of tests that could be conducted using this approach. There are several techniques that have been used to estimate fleet-average EFs from mobile sources, including remote sensing (CitationGuo et al., 2007; CitationKuhns et al., 2004; CitationLau et al., 2012; CitationSawyer et al., 2000), usage of mobile laboratories (CitationHung et al., 2005; CitationZavala et al., 2006), and tunnel studies (CitationAllen et al., 2001; CitationEl-Fadel and Hashisho, 2001; CitationJamriska et al., 2004; CitationKristensson et al., 2004; CitationLegreid et al., 2007; CitationMcGaughey et al., 2004).
Of these techniques, tunnel studies have proven to be a robust method when the objective is to obtain fleet-average EFs under real-world conditions, including those for PM2.5 emissions (CitationCheng et al., 2010; CitationGillies et al., 2001). Tunnel experiments have the advantage that the emissions measured come from vehicles that traverse the tunnel and that such emissions have not been subject to photochemical degradation. In addition, tunnel studies deal with well-defined volumes of air. For these types of studies, variables related to the tunnel (geometry, slope, ventilation), vehicle fleet (technology, age, fuel, engine) and driving conditions (traffic density, temperature, maintenance) need to be considered in the experimental setup. Using this technique, EFs can be obtained by performing a mass balance over a control volume delimited by monitoring stations deployed inside the tunnel. These stations are usually located at the entrance and exit of a tunnel in which pollutant concentrations, as well as other parameters such as vehicle speed, fleet count, wind speed, temperature, and pressure, are measured. Here, the difference in pollutant concentration between the “inlet” and “outlet” points is assumed to be the emitted mass from mobile sources that traversed the tunnel during each sampling period.
Few studies have been conducted in Mexico related to measurements in tunnels to derive EFs from vehicle emissions (CitationVega et al., 2000; CitationZavala et al., 2006). In Mexico, it is common to use adjusted EFs and data from the U.S. Environmental Protection Agency (EPA) to estimate emission inventories for different sources. However, this information is inherent to the U.S. conditions and activity patterns. Only recently, EFs based on remote sensing techniques were reported for the MMA vehicular fleet (CitationAguilar-Gómez et al., 2009). Therefore, the purpose of this study was to conduct a monitoring campaign to gather additional local emission data from mobile sources for a typical vehicle fleet of the MMA, using a road tunnel as the experimental setup. This information will help to obtain a better understanding of mobile emissions in the MMA that could then be used when analyzing air pollution abatement strategies in the area.
Methods
Experimental site
The Loma Larga Tunnel (LLT) is one of the main transit connections between the municipalities of Monterrey and San Pedro Garza García, which are part of the MMA (). The tunnel has an approximate length of 532 m and no internal curves. It is composed of two independent bores, each one with a semicircular shape and a diameter of 17 m (). The two bores are almost completely independent; there is a small interconnection near the middle of the tunnel that represents less than 1% of the total area of each bore. Each bore has a four-lane configuration; however, the far right lane in each bore (in the traffic direction) is reserved for emergencies. In addition, each bore has a pedestrian lane that traverses the full length of the tunnel. The Monterrey–San Pedro Garza García bore (north to south direction) has a 3.5% positive slope, and thus the contrary flow is down-slope. The tunnel's ventilation system consists of jet fans positioned along the ceilings of each bore. However, these jet fans were not operational during the sampling periods of this study (ventilation was achieved from the vehicle flow—piston effect—that traversed it).
Sampling setup, instruments, and analysis techniques
The field campaign was conducted during six non-consecutive days between June 22 and June 30, 2009 (). Two sampling periods were selected for each day to account for high- and moderate-density traffic conditions; 2.5-hr and 2.0-hr sampling periods were chosen for the collection of PM2.5 and total nonmethane hydrocarbons (TNMHC) samples, respectively. The TNMHC sampling periods were contained within the interval of the 2.5-hr sampling periods for PM2.5 (i.e., 15 min after the PM2.5 sampling period started, the TNMHC sampling period began, and it ended 15 min before the PM2.5 period ended). Continuous CO2 measurements were conducted throughout the 2.5-hr periods, while continuous NOx measurements were performed just in the 2.0-hr sampling periods. In each period, monitoring equipment was deployed simultaneously at two points located over the walking lane of the bore at approximately 1.5 m from the tunnel wall. These two points, named “inlet” and “outlet,” served as the limits of the control volume over which the mass balances were performed to estimate the corresponding mobile emissions. The distance between sampling points and between the “inlet” sampling point and the actual entrance to the tunnel () were determined based on similar tunnel studies performed elsewhere (CitationValiulis et al., 2002; CitationCheng et al., 2010).
Table 1. Monitoring schedule in the LLT during the summer of 2009
Each sampling location was equipped with a Testo 435 device, which registered 1-min averages of CO2 levels, temperature, relative humidity, and absolute pressure. The equipment's technical characteristics are described in Simultaneously, air velocity at the same location was measured using a Testo 425 thermal anemometer (measurement range: 0–20 m/sec, accuracy ±(0.03 m/sec + 5% mV), resolution 0.01 m/sec). Both types of devices used in the tunnel measurements were calibrated and stabilized before each 2.5-hr sampling period started.
Table 2. Technical specifications of the TESTO 435 device
NOx levels were measured using a NOx-O2 chemiluminescence gas monitor (Shimadzu NOA-7000; NOx range from 0 to 4000 ppm calibrated for low-range measurements). Due to resource constraints, NOx levels were only measured at the “outlet” point during each sampling period. The NOx inlet condition was estimated using the concentration reported by the Obispado air quality station from the routine air quality monitoring system of the MMA, located less than 2 km (linear distance) from the experimental site. The Obispado station is located in downtown Monterrey and is influenced mainly by mobile source emissions, as well as the entrances of the tunnel in both directions. In the same way, equipment malfunction during the field campaign limited the collection of valid samples to measure CO levels. However, it is known that in environments where mobile sources dominate the total local emissions, CO to NOx concentration ratios provide an accurate indicator of such emissions (CitationParrish, 2006). Thus, NOx levels were used as a surrogate for CO concentrations, as reported by the relationship observed in the air quality registries from the Obispado station ():
This method assumes that the NOx and CO concentrations measured at the Obispado station are equivalent to the “inlet” concentrations at the LLT (northern inlet) due to the short distance between the two sites, the prevalence of mobile sources in the area, and the lack of physical barriers that could limit local mixing of emissions. For the southern inlet of the LLT, even though the area surrounding this entrance of the tunnel is also dominated by mobile sources, the Loma Larga Hill (LLH) represents itself a natural barrier that could entail different conditions between the Obispado station and the LLT entrance. The possible inhomogeneity in the concentrations between these last two points was further explored by analyzing the estimated evolution of the mixing height (MH) during the monitoring campaigns. Convectively and mechanically generated MHs were estimated during each monitoring period to establish possible interference in the mixing of pollutants between both sides of the LLH. Convectively generated MHs were estimated using the formulation suggested by CitationCarson (1973) and modified by CitationWeil and Brower (1983). Mechanically generated MHs were estimated from the expression derived by CitationZilitinkevich (1972) and CitationVenkatram (1980).
TNMHC levels were obtained simultaneously at each sampling location through whole air samples taken using 6-L SUMMA-polished stainless-steel canisters (24 samples in total). The sampling train included a canister and a precalibrated mass-flow controller to obtain 2-hr integrated samples with these devices. In addition, the sampling inlet was connected to a Teflon sampling line to elevate the sampling point to the sampling points of the rest of the monitoring devices. The sampling train was subject to vacuum tests before each run to identify possible leaks. Chemical analysis was performed for TNMHC (gas chromatography coupled with flame ionization detection) using the U.S. Environmental Protection Agency (EPA) TO-12 method. Chemical analysis of all canister samples was conducted by TestAmerica laboratory (Austin, TX). All canisters used were cleaned and blank certified according to approved approaches described in U.S. EPA method TO-15; 100% laboratory blanks were used, as well as 10% sample duplicates. Additional details on the analysis techniques used in this study for TNMHC can be found in CitationMendoza et al. (2012).
For the PM2.5, samples were collected simultaneously at the inlet and outlet sites in the tunnel during each sampling period using low-volume filter-based instruments (MiniVol, Airmetrics) for a total of 24 filter samples. To insure that enough mass was collected in each sampling period, 2.5-hr intervals were chosen to operate these pieces of equipment based on test samples obtained prior to conducting the field campaign. Samplers were calibrated before and after each sampling period using a digital manometer (traceable to the National Institute for Standards and Technology) to operate at a 5.5-L/min flow rate. These devices were automatically turned on and off at preset times by an internal timer. The samplers were equipped with a 2.5-μm size-selective impactor and 47-mm Whatman Teflon filters. Prior to sampling, all filters were preweighed and packed in petri slides. All loaded filter samples were stored and transported inside a cooler chilled with blue ice. The collected mass on each filter was determined by gravimetric analysis conducted by Chester LabNet (Tigard, OR). Gravimetric analysis was conducted using a Sartorius ME5 microbalance (1-μg readability), located in a temperature- and humidity-controlled weighing room. All filters were weighted (tare or gross) at a controlled average temperature of 20–23°C (allowable variance of 2°C) over a 24-hr period and average humidity of 30–40% (allowable variance of 5%) over a 24-hr period. Filters were equilibrated to constant temperature and humidity for a minimum of 24 hr prior to tare or gross weighing. In addition, the same filter samples were analyzed for 38 metals (Na to Pb) by x-ray fluorescence (XRF: U.S. EPA Method IO-3.3) as discussed by CitationMancilla et al. (2012). This paper focuses solely on the analysis of the collected mass.
EF estimation
Emission factors (EFs) can be estimated from measurements taken in the interior of a tunnel by performing a mass balance over each pollutant (CitationPierson et al., 1996). Here, the main assumption is that the difference in concentrations between the exit and inlet points of the control volume set inside the tunnel corresponds exclusively to the emissions from mobile sources that went through the tunnel. Thus, the mass emitted per unit time of species k from the vehicles (Mk ) can be expressed as:
EFs for species k can also be estimated in terms of mass emitted per volume of fuel burned (E′ k ) through a carbon mass balance (CitationMartins et al., 2006):
For the particular case of PM2.5, EFs were corrected by field blanks (to reduce the effects of tunnel background) and dust resuspension by vehicle transit inside the tunnel. Dust resuspension tends to be more dependent on environmental conditions, such as temperature, wet or dry weather, vegetation, and tunnel cleaning, than on fleet characteristics. Tunnel studies have obtained contributions of crustal elements in PM2.5 of 2–3% (CitationCheng et al., 2010), 12% (CitationHung-Lung and Yao-Sheng, 2009), and up to 14–17% (CitationHandler et al., 2008). Main elements reported in these PM2.5 emissions include Si, Fe, Ca, Al, and K (CitationLough et al., 2005). Assuming average ambient conditions during the campaign, EFs were corrected, considering that silicon (Si) is one of the key markers of resuspended dust (CitationHandler et al., 2008; CitationWatson and Chow, 2001). Collection of dust samples from the experimental site was accomplished by sweeping the road and nearby surfaces. The collected dust samples were introduced into a resuspension system (). A reverse vacuum system was used to resuspend the dust and force its flow through the chamber. Then PM2.5 samples of this resuspended dust were obtained using a low-volume sampler. As indicated previously, levels of Si and other components were obtained by XRF. A complete description of the chemical characterization of the PM2.5 samples has been reported by CitationMancilla et al. (2012). Finally, the ratio of all components to Si was calculated in the dust samples and this chemical profile was subtracted from the ambient air samples, considering that all the Si found in the air samples came from dust resuspension.
Results and Discussion
Micrometeorological conditions and fleet composition
Average micrometeorological conditions inside the LLT during the field campaign were 22.5°C, 715.4 mm Hg, and 43.3% relative humidity (RH). Considering only the 2-hr sampling periods, in total 87,393 vehicles were sampled during the whole field campaign, while considering the 2.5-hr sampling periods the total number of vehicles that traversed the tunnel during the study was 108,569. Regardless of the sampling time periods or the day or the week, the fleet composition was practically the same, reducing the probability that these variables could influence largely the results. The average fleet composition was as follows: 97 ± 0.7% of the vehicles sampled were gasoline-powered vehicles (56.8 ± 6.2% light-duty vehicles, 8.4 ± 1.9% taxis, 20.2 ± 2.9% sport utility vehicles [SUVs], and 11.7 ± 2.9% gasoline pickup trucks); the remaining 3 ± 0.6% were diesel buses and trucks (2.4 ± 0.6%) and motorcycles (0.6 ± 0.2%). Average vehicle velocities were as low as 41.9 ± 7.18 km/hr (Monterrey–San Pedro bore; June 24, 11–13 hr), and as high as 75.9 ± 9.5 km/hr, (San Pedro–Monterrey bore; June 25, 10–12 hr). The number of vehicles, vehicle velocities and classification were determined using a video recording system.
Evolution of the mixing height
Significant horizontal variations in the MH structure and properties usually arise from surface heterogeneity like changes in surface elevation, as occur in hilly regions (CitationGarrat, 1994). This is relevant for this study, particularly since homogeneous CO and NOx concentrations levels are being assumed between the Obispado air quality station and the southern entrance of the LLT to estimate the corresponding EFs when the measurements occurred in the San Pedro-to-Monterrey bore. If the MH is too shallow (e.g., less than the height of the LLH), then atmospheric mixing could be limited between both sides of the LLT.
illustrates the evolution of the estimated mechanical and convective MH in the proximity of the LLT during the periods in which the San Pedro Garza García to Monterrey bore was being used as the experimental setup. Results indicate that the estimated MH was well over the height of the LLH (∼80 m above the average city ground level) during the sampling periods when the vehicles were driven down-slope. In addition, average wind speeds during these periods ranged from 2 m/sec to 6 m/sec, indicating fair local horizontal advection effects that foster good mixing in both sides of the LLH.
Figure 5. Mechanical (a) and Convective (b) MH profiles for the San Pedro-Monterrey sampling periods.
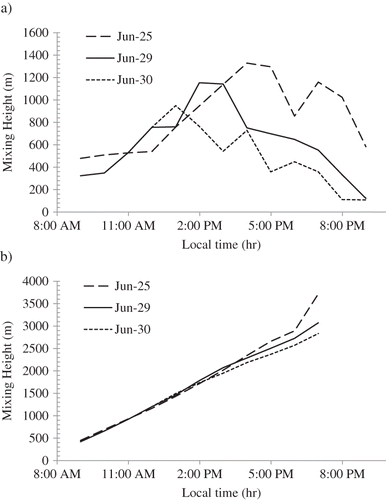
Therefore, from the evolution of the MH in the LLT, it is reasonable to assume that the NOx and CO concentrations measured at the Obispado station can be taken as a surrogate of the levels of the southern entrance. Also, since the municipality of San Pedro Garza García is dominated by mobile sources, the CO to NOx ratio at the southern inlet should not be significantly different from the ratio estimated for the northern side.
CO2 profiles
illustrates a typical CO2 time series from the sampling points inside the LLT. It can be observed that the “outlet” data track the “inlet” data well and that the expected behavior on the relative levels of CO2 was obtained: higher inside the tunnel and lower at the inlet of the tunnel. This implies that the concentration gradients were positive and, therefore, that the difference represents the vehicle emissions. However, in some sampling periods concentration crossover (inlet concentrations greater than outlet concentrations) was observed due to traffic jams (). Profiles differences between the two cases were quite distinctive. During crossover events the whole tunnel was full with vehicles moving in a stop-and-go mode. This low velocity of the vehicles reduces the piston effect inside the tunnel that allows the movement of the air masses inside the tunnel at a point where the inlet and outlet sites experience similar pollutants accumulation and thus similar concentration levels. This state violates the assumptions that support eqs 2–4, making the EF estimates unreliable. When this occurred the period was discarded for further analysis, since estimated EFs turned out to be negative.
The 2.5-hr campaign-average CO2 gradient concentrations up-slope was higher than that down-slope; the ranges of those gradients were 102 to 127 ppm and 78 to 117 ppm, respectively. This gives a first indication of higher EFs when the vehicles are being driven up-slope than down-slope. This is further explored in the section where EFs are presented.
Average CO2 EFs
Average CO2 EFs obtained in the LLT for the 2.0-hr and 2.5-hr sampling periods are shown in The values obtained compare well between sampling periods, though the EFs were higher for the 2.0-hr sampling period. However, an analysis of variance (ANOVA) demonstrated that, at a significance level of 0.01, there is no evidence that there is a difference between 2.0-hr and 2.5-hr EFs means. The F value obtained for the comparison of EFs in terms of g/veh-km and g/L were 0.19 against a critical F value of 3.34 (p = 0.8992) and 3.55 versus a critical F value of 5.56 (p = 0.0424), respectively. These results were obtained with a relatively small sample; additional measurements could give stronger evidence to assure whether or not the means of the EFs are in fact different.
Table 3. Average CO2 EFs obtained in the LLT
When compared by bore, CO2 EFs tended to be higher in the Monterrey–San Pedro bore (positive slope) than in the San Pedro–Monterrey bore (which has a negative slope): 190 ± 52 g/km-veh versus 175 ± 36 g/km-veh for the 2.0-hr sampling periods and 188 ± 22 g/km-veh versus 152 ± 22 g/km-veh for the 2.5-hr sampling period. This difference can be caused by a constant acceleration experienced by the vehicles when they move in a road with a positive slope, which entails a higher fuel consumption and therefore higher emission rates. With the values obtained for CO2 EFs, an average fuel economy of 12.3 ± 2.3 km/L and 12.1 ± 1.9 km/L was determined for the 2.0-hr and 2.5-hr sampling periods, respectively.
Average gaseous and PM2.5 EFs
shows the gaseous pollutants and PM2.5 EFs estimated from the LLT experiments. It can be observed that up-slope CO, NOx, and TNMHC EFs (in terms of g/km-veh) were 3.1, 1.4, and 2.0 times the down-slope EFs, respectively. The constant acceleration of vehicles, when they move on a positive slope, tends to make them use a fuel-rich mixture and thus produce more unburned TNMHCs in the vehicle exhaust gases. This could be one of the reasons of why larger TNMHC EFs are being estimated for up-slope conditions. Even though it would be expected that NOx emissions decrease under fuel-rich conditions, acceleration on a positive slope could derive in higher vehicle speeds, and thus higher engine combustion temperatures and higher availability of oxygen in the combustion chamber, which in turn favor NOx production (e.g., CitationJimenez et al., 1999; CitationKean et al., 2003). CO EFs showed the same trends as the NOx EFs. This is not surprising since estimated CO results are a function of NOx measurements. One would expect that fuel-rich operation would result in higher CO emissions; however, we acknowledge that caution needs to be taken in using the CO EFs obtained here as they were not estimated from direct CO measurements.
Table 4. Average gaseous and PM2. 5 EFs in the LLT
The average PM2.5 EF in the Monterrey–San Pedro bore was 13.3 ± 6.3 mg/km-veh, while in the San Pedro-Monterrey bore it was 21.7 ± 9.5 mg/km-veh. These EFs were corrected for dust resuspension after blank-correcting the collected mass. PM2.5 EF was higher for the down-slope condition than for the up-slope condition, probably because vehicles in negative slopes tend to emit additional PM2.5 from brake wear (CitationBukowiecki et al., 2009). CitationGarg et al. (2000) report PM2.5 brake wear emissions in the range of 2.1–5.5 mg/km-veh (depending on vehicle size). It is well known that Fe, Cu, and Sb are markers of brake linings. For example, CitationMancilla et al. (2012) report for this same study Sb EFs (mg/veh-km) of 0.09 ± 0.16 and 0.04 ± 0.10 for down-slope and up-slope conditions, respectively. This supports the reasoning that brake wear contributed, to a certain extent, to downhill PM2.5 emissions. When comparing the behavior of PM2.5 EFs between up-slope and down-slope conditions, vehicle velocity differences and thus dust resuspension differences could be, besides brake wear, marking a difference between the obtained values. However, as indicated previously, EFs were corrected for dust resuspension. In addition, even though the lowest sampling period-average vehicle velocity occurred in the Monterrey–San Pedro bore (up-slope) and the highest in the San Pedro–Monterrey bore (down-slope), in the LLT, the global vehicle speeds up-slope and down-slope were quite similar (50 km/hr vs. 57 km/hr). Therefore, it is expected that, on average, the contribution from dust resuspension down-slope would be similar that of the up-slope condition. PM2.5 EFs in terms of mass per volume of fuel consumed (g/L) presented the inverse behavior compare to the one just described: higher values for the positive slope conditions compared to the negative slope conditions. Travel over a positive slope implicates higher fuel consumption than on a negative slope, where vehicles tend to brake instead of accelerate.
EF comparison
show a comparison between the gaseous EFs obtained from the LLT against values reported for other tunnel studies. From it can be observed that EFs estimated for CO2 in the LLT in terms of g/km-veh are in the magnitude range of other studies, while those in terms of g/L are slightly lower. In comparing these last EFs one has to consider that the values of ρ g , wc , and the average molecular weight of TNMHC used in eq 4 are not necessarily the same between studies; these values depend mainly on the properties of the gasoline of each region. Our study sampled up to 60 times more vehicles than in the other cited tunnel studies, a condition that favored sampling a representative amount of vehicles, which were in a great majority (97% of the total fleet) light-duty vehicles. The EFs obtained in the LLT can be stated to be a good representation of the average fleet of light-duty vehicles in the MMA that typically commute between the Monterrey and San Pedro municipalities and that would travel under the influence of nonnegligible slopes. The fleet mix, besides the different grades, provides an additional factor that could explain the differences among tunnel studies: that is, 82% and 90% of the vehicles sampled in the Tuscarora and The Fort McHenry tunnels, respectively, were light-duty vehicles. In addition, the mix of light-duty vehicles in each case could influence the average EFs obtained in the different studies, as well as differences in fuel economy and emission regulations among countries.
Table 5. Comparison of CO2 EFs with other tunnel studies
One additional reason why the CO2 EFs differ between studies can be the difference in average velocities of the vehicular fleets sampled in the different tunnel studies. Vehicle speed is an important factor that affects emissions for all pollutants. However, sample correlations between vehicle speed and emissions tend to be weak due to highly dispersed values within a drive cycle (CitationBeevers and Carslaw, 2005). presents average CO2 emission factors for each sampling period plotted against the average vehicle speed for that same period. Results indicate that CO2 EFs and vehicle velocities in the LLT tend to follow a quadratic behavior (R 2 = 0.55): At relative higher or lower velocities, emissions tend to increase, with a minimum around 60 to 65 km/h. Average velocities in the LLT were below 60 km/hr, which could be a reason why EFs were higher (even when the vehicles were moving down-slope) compared to, for example, the Gelizinis Vilkas Tunnel were average velocities were 60 km/h.
Figure 7. Effect of vehicle speed on CO2 emission factors (combined values for 2.0-hr and 2.5-hr measurements) obtained in the LLT.
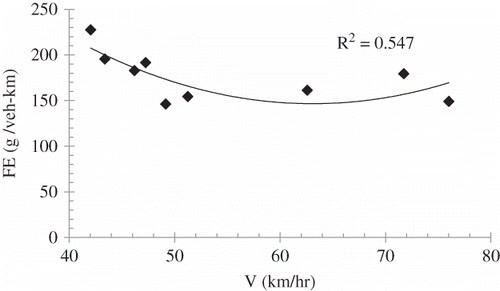
In , the estimated EFs (g/km-veh) for CO and TNMCH based on the LLT data tend to be higher in comparison with other studies (except for the Chung-Cheng tunnel located in Taiwan), while NOx is between two and ten times lower. In the same way, indicates that the LLT EFs (g/L) for CO and TNMHC are between two and four times higher, while the LLT NOx EF is comparable or up to half the value of other tunnel studies. This difference could be attributed again, at least in part, to average speed differences in the vehicles among studies. The average speed in the LLT was in the range of 48–59 km/hr, while in other tunnel studies it was in the 60–96 km/hr range. Under low-speed conditions vehicles tend to emit higher amounts of CO and unburned TNMHC (CitationYli-Tuomi et al., 2005; Corsmeier et al., 2009), while at higher velocities NOx emissions tend to increase (CitationZavala et al., 2006). However, CO and NOx emissions reported here have to be used with caution due to the uncertainty associated with the method used to obtain the value that is being reported. In addition, it is important to consider the difference in time between studies (up to 13 years) and the implications on the availability of modern emission control devices in automobiles, as well as the inherent conditions of the study (type of vehicular fleet, transit conditions, type of fuel, road grade, etc.).
Table 6. Comparison of gaseous vehicle EFs in g/km-veh with other tunnel studies
Table 7. Comparison of gasoline vehicle EFs in g/L with other tunnel studies
As indicated previously, a study by Aguilar et al. (2009) reported composite EFs for the MMA vehicular fleet based on remote sensing data obtained in a June 2008 field campaign at 10 sites within the metropolitan area. In that study, vehicle speeds were mainly between 20 km/hr and 35 km/hr, with most of the vehicles driven in an acceleration mode, which again make the values not fully comparable but do provide a complementary range of expected values. presents a comparison between the emission rates obtained by Aguilar et al. (2009) and our study. In this comparison, we only consider data reported in the remote sensing study for vehicles 1999 and newer, which are the type of vehicles that are typically found in the LLT. The CO EF derived from the LLT is well within the range of values reported in the remote sensing study, while the NOx EF is half the value and the hydrocarbon EF is twice the value with respect to what Aguilar et al. (2009) report. Different vehicle fleet composition could be the reason of the difference in those EFs as well as the dominant driving mode in each study.
Table 8. Comparison of gaseous emission factors (g/km-veh) for the MMA obtained through two different techniques
Finally, a comparison of PM2.5 EFs with those of other tunnel studies is shown in , where it can be seen that the LLT EFs are the lowest, probably because the sampled fleet consists heavily of gasoline vehicles (diesel trucks tend to emit larger amounts of PM2.5 [CitationJamriska et al., 2004]). In addition, results from a parallel study indicate that the sampled fleet in this study appears to be rather well maintained and of a recent model year (CitationMendoza et al., 2012). It is also important to highlight the difference in times between studies (at least 4 years). Passenger cars of a recent model year would be expected to have lower EFs.
Table 9. Comparison of PM2. 5 emission factors in mg/km-veh with other tunnel studies
Conclusions
To create a confident emission inventory and air quality management strategies for any given region, it is important to use appropriate data for that particular region. As more experimental data are available, models' prediction capabilities can be improved. Based on this, a tunnel study was conducted during summer 2009 to derive gaseous and PM2.5 EFs for mobile sources for the MMA. The tunnel technique makes it possible to sample a large number of vehicles and has proven to be a robust method to obtain fleet-average EFs. Given the characteristics of the tunnel used here as the experimental setup, the results obtained are a good estimate for gasoline-powered light-duty vehicles of the MMA under nonnegligible road grades. This is the first study that reports this type of data for the region. The values obtained are consistent with those obtained in other similar studies and these can be complementary to those obtained by other techniques. Additional data are still necessary to have a better representation of vehicles, operating modes, and conditions not considered in this study for the MMA. Overall, EFs (mg/km-veh) for CO2 were in the range of values found in other studies, and CO and TNMHC tended to be higher than those reported in other tunnel studies, while NOx estimates were lower. Results for CO and NOx have to be used with caution due to the uncertainty associated with the estimation procedure used to derive them. For PM2.5 EFs, the values tended to be lower in comparison with those calculated in other tunnel studies. The effect of the tunnel slope can be relevant: Up-slope EFs for gaseous species were higher than down-slope, while for PM2.5 down-slope EFs were higher than up-slope, probably because of the additional contribution of brake wear to the total PM2.5 emissions. Average estimated fuel economy for the experiments corresponds well to what would be expected of the type of fleet sampled.
Acknowledgments
This work was supported by the Mexican National Council for Science and Technology (CONACYT) through grant 002024CONA and Tecnológico de Monterrey through grant CAT-186. Alejandro Araizaga received additional support (scholarship) through the Mexican Institute of Chemical Engineers (IMIQ) and Yasmany Mancilla through CONACYT. We appreciate the support of the municipality of Monterrey during the field campaign conducted in this study.
References
- Aguilar-Gómez , J.A. , Garibay-Bravo , V. , Tzintzun-Cervantes , G. , Cruz-Jimate , I. and Echániz-Pellicer , G. Mobile source emission estimates using remote sensing data from Mexican cities . Proceedings of EPA's 18th Annual International Emission Inventory Conference . April 14–17 , Baltimore , MD .
- Allen , J.O. , Mayo , P.R. , Hughes , L.S. , Salmon , L.G. and Cass , G.R. 2001 . Emissions of size-segregated aerosols from on-road vehicles in the Caldecott Tunnel . Environ. Sci. Technol , 35 : 4189 – 4197 . doi: 10.1021/es0015545
- Ban-Weiss , G. A. , McLaughlin , J. P. , Harley , R. A. , Kean , A. J. , Grosjean , E. and Grosjean , D. 2008 . Carbonyl and nitrogen dioxide emissions from gasoline- and diesel-powered motor vehicles . Environ. Sci. Technol , 42 : 3944 – 3950 . doi: 10.1021/es8002487
- Beevers , S.D. and Carslaw , D.C. 2005 . The impact of congestion charging on vehicle speed and its implications for assessing vehicle emissions . Atmos. Enviro , 39 : 6875 – 6884 . doi: 10.1016/j.atmosenv.2005.08.021
- Bukowiecki , N. , Lienemann , P. , Hill , M. , Figi , R. , Richard , A. , Furger , M. , Rickers , K. , Falkenberg , G. , Zhao , Y. , Cliff , S.S. , Prevot , A.S.H. , Baltensperger , U. , Buchmann , B. and Gehrig , R. 2009 . Real-world emission factors for antimony and other brake wear related trace elements: Size-segregated values for light and heavy duty vehicles . Environ. Sci. Technol , 43 : 8072 – 8078 . doi: 10.1021/es9006096
- Carson , D.J. 1973 . The development of a dry inversion-capped convectively unstable boundary layer . Q. J. R. Meteorol. Soc , 99 : 450 – 467 . doi: 10.1256/smsqj.42104
- Castillo-Hernández , P. , Mendoza-Domínguez , A. and Caballero-Mata , P. 2012 . Analysis of physicochemical properties of mexican gasoline and diesel reformulated with ethanol . Ing. Invest. Tecnol , XIII : 293 – 306 .
- Cheng , Y. , Lee , S. C. , Ho , K. F. , Chow , J. C. , Watson , J. G. , Louie , P. K. K. , Cao , J. J. and Hai , X. 2010 . Chemically-speciated on-road PM2.5 motor vehicle emission factors in Hong Kong . Sci. Total Environ , 408 : 1621 – 1627 . doi: 10.1016/j.scitotenv.2009.11.061
- Corsmeier , U. , Imhof , D. , Kohler , M. , ühlwein , J. K , Kurtenbach , R. , Petrea , M. , Rosenbohm , E. , Vogel , B. and Vogt , U. 2005 . Comparison of measured and model-calculated real-world traffic emissions . Atmos. Environ , 39 : 5760 – 5775 . doi: 10.1016/j.atmosenv.2005.06.048
- Dales , R. , Liu , L. , Wheeler , A. J. and Gilbert , N. L. 2008 . Quality of indoor residential air and health . Can. Med. Assoc. J , 179 : 147 – 152 . doi: 10.1503/cmaj.070359
- Díaz , R.V. and Domínguez , E.R. 2009 . Health risk by inhalation of PM2.5 in the Metropolitan Zone of the City of Mexico . Ecotox. Environ. Safe , 72 : 866 – 871 . doi: 10.1016/j.ecoenv.2008.09.014
- El-Fadel , M. and Hashisho , Z. 2001 . Vehicular emissions in roadway tunnels: A critical review . Crit. Rev. Environ. Sci. Technol , 31 : 125 – 174 . doi: 10.1080/20016491089190
- Garg , B.D. , Cadle , S.H. , Mulawa , P.A. , Groblicki , P.J. , Laroo , C. and Parr , G.A. 2000 . Brake wear particulate matter emissions . Environ. Sci. Technol , 34 : 4463 – 4469 . doi: 10.1021/es001108h
- Garrat , J.R. 1994 . Review: The atmospheric boundary layer . Earth-Sci. Rev , 37 : 89 – 134 . doi: 10.1016/0012-8252(94)90026-4
- Gertler , A.W. 2005 . Diesel vs. gasoline emissions: Does PM from diesel or gasoline vehicles dominate in the US? . Atmos. Environ , 39 : 2349 – 2355 . doi: 10.1016/j.atmosenv.2004.05.065
- GENL and SEMARNAT . 2008 . Programa de Gestión para Mejorar la Calidad del Aire del Área Metropolitana de Monterrey 2008–2012 , Mexico City, Mexico : Instituto Nacional de Ecología .
- Gertler , A.W. , Sagebiel , J.C. , Wittorff , D.N. , Pierson , W.R. , Dippel , W.A. , Freeman , D. and Sheetz , L. 1997 . Vehicle Emissions in Five Urban Tunnels , Atlanta, GA : Desert Research Institute, Reno, NV, Report to the Coordinating Research Council .
- Gillies , J.A. , Gertler , A.W. , Sagebiel , J.C. and Dippel , W.A. 2001 . On-road particulate matter (PM2.5 and PM10) emissions in the Sepulveda Tunnel, Los Angeles, California . Environ. Sci. Technol , 35 : 1054 – 1063 . doi: 10.1021/es991320p
- Guo , H. , Zhang , Q. , Shi , Y. and Wang , D. 2007 . On-road remote sensing measurements and fuel-based motor vehicle emission inventory in Hangzhou, China . Atmos. Environ , 41 : 3095 – 3107 . doi: 10.1016/j.atmosenv.2006.11.045.doi:10.1016/j.atmosenv.2006.11.045
- Handler , M. , Puls , C. , Zbiral , J. , Marr , I. , Puxbaum , H. and Limbeck , A. 2008 . Size and composition of particulate emissions from motor vehicles in the Kaisermühlen-Tunnel, Vienna . Atmos. Environ , 42 : 2173 – 2186 . doi: 10.1016/j.atmosenv.2007.11.054
- Hsu , Y.-C. , Tsai , J.-H. , Chen , H.-W. and Lin , W.-Y. 2001 . Tunnel study of on-road vehicle emissions and the photochemical potential in Taiwan . Chemosphere , 42 : 227 – 234 . doi: 10.1016/s0045-6535(00)00074-6
- Hung , W.-T. , Tam , K.-M. , Lee , C.-P. , Chan , L.-Y. and Cheung , C.-S. 2005 . Comparison of driving characteristics in cities of Pearl River Delta, China . Atmos. Environ , 39 : 615 – 625 . doi: 10.1016/j.atmosenv.2004.10.019
- Hung-Lung , C. and Yao-Sheng , H. 2009 . Particulate matter emissions from on-road vehicles in a freeway tunnel study . Atmos. Environ , 43 : 4014 – 4022 . doi: 10.1016/j.atmosenv.2009.05.015
- Hwa , M.-Y. , Hsieh , C.-C. , Wu , T.-C. and Chang , L.-F.W. 2002 . Real-world vehicle emissions and VOCs profile in the Taipei tunnel located at Taiwan Taipei area . Atmos. Environ , 36 : 1993 – 2002 . doi: 10.1016/S1352-2310(02)00148-6
- INE and SEMARNAT . 2009 . Cuarta comunicación nacional ante la convención marco de las naciones unidas sobre el cambio climático , México, D.F : Instituto Nacional de Ecología, Secretaría del Medio Ambiente y Recursos Naturales .
- Jamriska , M. , Morawska , L. , Thomas , S. and He , C. 2004 . Diesel bus emissions measured in a tunnel study . Environ. Sci. Technol , 38 : 6701 – 6709 . doi: 10.1021/es030662z
- Jimenez , J.L. , Koplow , M.D. , Nelson , D.D. , Zahniser , M.S. and Schmidt , S.E. 1999 . Characterization of on-road vehicle NO emissions by a TILDAS remote sensor . J. Air Waste Manage. Assoc , 49 : 463 – 470 . doi: 10.1080/10473289.1999.10463814
- John , C. , Friedrich , R. , Staehelin , J. , Schläpfer , K. and Stahel , W.A. 1999 . Comparison of emission factors for road traffic from a tunnel study (Gubrist tunnel, Switzerland) and from emission modeling . Atmos. Environ , 33 : 3367 – 3376 . doi: 10.1016/s1352-2310(98)00377-x
- Kean , A.J. , Harley , R.A. and Kendall , G.R. 2003 . Effects of vehicle speed and engine load on motor vehicle emissions . Environ. Sci. Technol , 37 : 3739 – 3746 . doi: 10.1021/es0263588
- Kristensson , A. , Johansson , C. , Westerholm , R. , Swietlicki , E. , Gidhagen , L. , Wideqvist , U. and Vesely , V. 2004 . Real-world traffic emission factors of gases and particles measured in a road tunnel in Stockholm, Sweden . Atmos. Environ , 38 : 657 – 673 . doi: 10.1016/j.atmosenv.2003.10.030
- Kuhns , H.D. , Mazzoleni , C. , Moosmüller , H. , Nikolic , D. , Keislar , R.E. , Barber , P.W. , Li , Z. , Etyemezian , V. and Watson , J.G. 2004 . Remote sensing of PM, NO, CO and HC emission factors for on-road gasoline and diesel engine vehicles in Las Vegas, NV . Sci. Total Environ , 322 : 123 – 137 . doi: 10.1016/j.scitotenv.2003.09.013
- Lau , J. , Hung , W.T. and Cheung , C.S. 2012 . Observation of increases in emission from modern vehicles over time in Hong Kong using remote sensing . Environ. Pollut , 163 : 14 – 23 . doi: 10.1016/j.envpol.2011.12.021
- Legreid , G. , Reimann , S. , Steinbacher , M. , Staehelin , J. , Young , D. and Stemmler , K. 2007 . Measurements of OVOCs and NMHCs in a Swiss highway tunnel for estimation of road transport emissions . Environ. Sci. Technol , 41 : 7060 – 7066 . doi: 10.1021/es062309+
- Lough , G.C. , Schauer , J.J. , Park , J.-S. , Shafer , M.M. , Deminter , J.T. and Weinstein , J.P. 2005 . Emissions of metals associated with motor vehicle roadways . Environ. Sci. Technol , 39 : 826 – 836 . doi: 10.1021/es048715f
- Mancilla , Y. and Mendoza , A. 2012 . A tunnel study to characterize PM2.5 emissions from gasoline-powered vehicles in Monterrey, Mexico . Atmos. Environ , doi: 10.1016/j.atmosenv.2012.05.025
- Mendoza , A. , Araizaga , A.E. and Mancilla , Y. 2012 . Volatile organic compound emissions from light-duty vehicles in Monterrey, Mexico: A tunnel study . Int. J. Environ. Res , in press
- Martins , L.D. , Andrade , M.F. , Freitas , E.D. , Pretto , A. , Gatti , L.V. , Albuquerque , E.L. , Tomaz , E. , Guardani , M.L. , Martins , M.H.R.B. and Junior , O.M.A. 2006 . Emission factors for gas-powered vehicles traveling through road tunnels in São Paulo, Brazil . Environ. Sci. Technol , 40 : 6722 – 6729 . doi: 10.1021/es052441u
- McGaughey , G.R. , Desai , N.R. , Allen , D.T. , Seila , R.L. , Lonneman , W.A. , Fraser , M.P. , Harley , R.A. , Pollack , A.K. , Ivy , J.M. and Price , J.H. 2004 . Analysis of motor vehicle emissions in a Houston tunnel during the Texas Air Quality Study 2000 . Atmos. Environ , 38 : 3363 – 3372 . doi: 10.1016/j.atmosenv.2004.03.006
- Ohura , T. , Amagai , T. , Shen , X. , Li , S. , Zhang , P. and Zhu , L. 2009 . Comparative study on indoor air quality in Japan and China: Characteristics of residential indoor and outdoor VOCs . Atmos. Environ , 43 : 6352 – 6359 . doi: 10.1016/j.atmosenv.2009.09.022
- Parrish , D.D. 2006 . Critical evaluation of US on-road vehicle emission inventories . Atmos. Environ , 40 : 2288 – 2300 . doi: 10.1016/j.atmosenv.2005.11.033
- Pierson , W.R. , Gertler , A.W. , Robinson , N.F. , Sagebiel , J.C. , Zielinska , B. , Bishop , G.A. , Stedman , D.H. , Zweidinger , R.B. and Ray , W.D. 1996 . Real-world automotive emissions—Summary of studies in the fort McHenry and Tuscarora mountain tunnels . Atmos. Environ , 30 : 2233 – 2256 . doi: 10.1016/1352-2310(95)00276-6
- Sagebiel , J. C. , Zielinska , B. , Pierson , W. R. and Gertler , A. W. 1996 . Real-world emissions and calculated reactivities of organic species from motor vehicles . Atmos. Environ , 30 : 2287 – 2296 . doi: 10.1016/1352-2310(95)00117-4
- Sawyer , R.F. , Harley , R.A. , Cadle , S.H. , Norbeck , J.M. , Slott , R. and Bravo , H.A. 2000 . Mobile sources critical review: 1998 NARSTO assessment . Atmos. Environ , 34 : 2161 – 2181 . doi: 10.1016/S1352-2310(99)00463-X
- Schifter , I. , Diaz , L. , Vera , M. , Castillo , M. , Ramos , F. , Avalos , S. and Lopez-Salinas , E. 2000 . Impact of engine technology on the vehicular emissions in Mexico City . Environ. Sci. Technol , 34 : 2663 – 2667 . doi: 10.1021/es991254r
- Valiulis , D. , Ceburnis , D. , Sakalys , J. and Kvietkus , K. 2002 . Estimation of atmospheric trace metal emissions in Vilnius City, Lithuania, using vertical concentration gradient and road tunnel measurement data . Atmos. Environ , 36 : 6001 – 6014 . doi: 10.1016/S1352-2310(02)00764-1
- Vega , E. , Mugica , V. , íaz , L. D and Ramos , F. 2000 . Comparación de perfiles de emisiones vehiculares en túnel y en dinamómetro . Rev. Int. Contam. Ambie , 16 : 55 – 60 .
- Velasco , E. , Lambi , B. , Westberg , H. , Allwine , E. , Sosa , G. , Arriaga-Colina , J. , Jobson , B. , Alexander , M. , Prazeller , P. , Knighton , W. , Rogers , T. , Grutter , M. , Herndon , S. , Kolb , E. , Zavala , M. , Foy , B. , Volkamer , R. , Molina , L. and Molina , M. 2007 . Distribution, magnitudes, reactivities, ratios and diurnal patterns of volatile organic compounds in the Valley of Mexico during the MCMA 2002 & 2003 field campaigns . Atmos. Chem. Phys , 7 : 329 – 353 . doi: 10.5194/acp-7-329-2007
- Venkatram , A. 1980 . Estimating the Monin-Obukhov length in the stable boundary layer for dispersion calculations . Bound.-Layer Meteorol , 19 : 482 – 485 . doi: 10.1007/BF00122347
- Watson , J.G. and Chow , J.C. 2001 . Source characterization of major emission sources in the Imperial and Mexicali Valleys along the US/Mexico border . Sci. Total Environ , 276 : 33 – 47 . doi: 10.1016/s0048-9697(01)00770-7
- Weil , J.C. and Brower , R.P. Estimating convective boundary layer parameters for diffusion applications . Report No. PPSP-MP-48 . 1983 . Maryland Power Plant Siting Program, Maryland Department of Natural Resources, Baltimore, MD
- Yli-Tuomi , T. , Aarnio , P. , Pirjola , L. , äjelä , T. M , Hillamo , R. and Jantunen , M. 2005 . Emissions of fine particles, NOx, and CO from on-road vehicles in Finland . Atmos. Environ , 39 : 6696 – 6706 . doi: 10.1016/j.atmosenv.2005.07.049
- Zavala , M. , Herndon , S.C. , Slott , R.S. , Dunlea , E.J. , Shorter , J.H. , Zahniser , M. , Knighton , W.B. , Rogers , T.M. , Kolb , C.E. , Molina , L.T. and Molina , M.J. 2006 . Characterization of on-road vehicle emissions in the Mexico City Metropolitan Area using a mobile laboratory in chase and fleet average measurement modes during the MCMA-2003 field campaign . Atmos. Chem. Phys , 6 : 5129 – 5142 . M.L.C. doi: 10.5194/acp-6-5129-2006
- Zilitinkevich , S.S. 1972 . On the determination of the height of the Ekman boundary layer . Bound.-Layer Meteorol , 3 : 141 – 145 . doi: 10.1007/BF02033914