Abstract
Primary fine particulate matters with a diameter of less than 10 µm (PM10) are important air emissions causing human health damage. PM10 concentration forecast is important and necessary to perform in order to assess the impact of air on the health of living beings. To better understand the PM10 pollution health risk in Taiyuan City, China, this paper forecasted the temporal and spatial distribution of PM10 yearly average concentration, using Back Propagation Artificial Neural Network (BPANN) model with various air quality parameters. The predicted results of the models were consistent with the observations with a correlation coefficient of 0.72. The PM10 yearly average concentrations combined with the population data from 2002 to 2008 were given into the Intake Fraction (IF) model to calculate the IFs, which are defined as the integrated incremental intake of a pollutant released from a source category or a region over all exposed individuals. The results in this study are only for main stationary sources of the research area, and the traffic sources have not been included. The computed IFs results are therefore under-estimations. The IFs of PM10 from Taiyuan with a mean of 8.5 per million were relatively high compared with other IFs of the United States, Northern Europe and other cities in China. The results of this study indicate that the artificial neural network is an effective method for PM10 pollution modeling, and the Intake Fraction model provides a rapid population risk estimate for pollutant emission reduction strategies and policies.
The PM10 (particulate matter with an aerodynamic diameter ≤10 μm) yearly average concentration of Taiyuan, with a mean of 0.176 mg/m3, was higher than the 65 μg/m3 recommended by the U.S. Environmental Protection Agency (EPA). The spatial distribution of PM10 yearly average concentrations showed that wind direction and wind speed played an important role, whereas temperature and humidity had a lower effect than expected. Intake fraction estimates of Taiyuan were relatively high compared with those observed in other cities. Population density was the major factor influencing PM10 spatial distribution. The results indicated that the artificial neural network was an effective method for PM10 pollution modeling.
Introduction
Particulate matter (PM) has several environmental impacts, including contamination of ambient air, acid rain, reduced visibility, modified cloud properties, and health-related problems (CitationCharlson and Rodhe, 1982; CitationLonghetto et al., 1992; CitationSanhueza, 2001; Intergovernmental Panel on Climate Change [CitationIPCC], 2007). PM10 refers to particulates with a mean aerodynamic diameter less than 10 µm. Recently, the dangers of PM10 have been largely demonstrated by toxicological studies for both short and long exposure times. Epidemiological studies suggest that respirable particulate matter is a risk factor for respiratory morbidity and cardiopulmonary mortality (CitationCarlton et al., 1999). Because of their relatively larger surface area, these small particles are also associated with a higher proportion of metals and persistent organic compounds than an equivalent mass of larger particles. PM10 has been shown to be significantly associated with the decline in lung function and with respiratory and cardiovascular disease-related deaths (CitationBerico et al., 1997). A study shows that PM10 can have adverse effects on the cardiovascular system (CitationDonaldson et al., 2005). Thus, PM air pollution control is necessary to prevent worsening of situation over the long run. However, air quality modeling is needed to take preventive and evasive action during episodes of airborne pollution (CitationCaselli et al., 2008).
It is not at all straightforward to build useful relationships between emission sources and the resulting impact on human health. The models still tend to be plagued by uncertainty in describing both the emission and meteorological fields, and the former requires a highly detailed description of anthropogenic activities in an urban area. These requirements make it rather difficult to use deterministic air quality models in the forecasting mode (Pérez-Roa et al., 2006).
From the last century, with the development of computer science, manifold mathematics models and computer technologies were proposed by many researchers to confirm the exposure concentration of pollutants. CitationMukerjee et al. (2001) and CitationKukkonen et al. (2001) developed a model based on the correlation between PMxx and NOx to predict yearly average PM10 concentrations, whereas Jorquera et al. (2001) used box models to analyze air pollution in Santiago, Chile, and developed a linear model for PM2.5, PM10, and coarse PM2.5-PM10 fractions. CitationTiittaa et al. (2002) developed a semiempirical model and applied multiple regression analysis to predict the PM concentration. However, these models are mostly based on semiempirical statistical relations among available data and measurements. They do not necessarily reveal any relation between cause and effect. Moreover, the complex and sometimes nonlinear relationships of multiple variables make statistical models awkward and complicated (CitationComrie, 1997).
Recently, the trend of using neural networks seems to be growing. The use of the artificial neural network (ANN) has been shown to give acceptable results for atmospheric pollution forecasting of air pollutants. CitationPérez and Trier (2001) have also succeeded in modeling and forecasting NOx by means of neural networks in the surrounding area of Santiago, Chile. CitationOrdieres et al. (2005) analyzed and benchmarked a neural network approach to the prediction of PM2.5 concentrations. CitationPapanastasiou et al. (2007) developed models using multiple regression and neural network methods that might produce accurate 24-hr predictions of daily average value of PM10 concentration.
In our study, we attempted to integrate the back-propagation artificial neural network (BPANN) and intake fraction (IF) into PM10 concentration modeling application. ANN is an effective method for atmospheric forecasting that has high precision and accuracy in environmental modeling applications. Intake fraction is an emission-intake relationship for primary pollutants, and it is estimated in order to make simple estimates of health damages from air pollution during a given exposure time.
The objectives of this study are (i) to calculate the temporal-spatial distribution of PM10 concentrations caused by three main emission sources in the research area during the period 2002 to 2008 based on an ANN model; (ii) to estimate the health effects caused by PM10 concentration levels based on an IF model; and (iii) to compare the PM10 exposure concentrations and IF estimates of Taiyuan City with common studies in other cities.
Such assessment is useful in further guiding the local government when it comes to maximizing the benefits of regulatory actions or polices in regard to energy use and air pollution control. More generally, our research enables us to evaluate what kind of actions and policies will be the most effective in terms of reducing air pollution concentrations and what the health benefits are from the air quality improvements that have taken place. By depicting a practical tool for policy appraisal, this paper should be of great interest to policy advisors and government bodies who deal with energy and environment planning in China.
Materials and Methods
Area description
Taiyuan City is the capital of Shanxi Province. It is located between latitudes 37˚N and 38˚N and between longitudes 112˚E and 113˚E. Taiyuan, with a population of 2.67 million and an area of 1500 km2, is one of the most important industry-based cities in China, and it supplies one-quarter of China's coal. The city is surrounded by mountains on three sides, which lead to poor atmospheric diffusion and severe air pollution. PM10 is the major pollutant of concern (CitationZhang et al., 2010). The main PM10 compositions in Taiyuan City are generally organic carbon (OC), water-soluble ions, and levoglucosan. And the major sources of PM10 are in the order of suspended dusts 30%, coal combustion 18%, motor vehicle exhausts 15%, sulfate 11%, soil wind sand dusts 9%, cement dusts 7%, nitrate 4%, and others 1% (CitationWu et al., 2008). It is estimated that the annual coal consumption of the city is approximately 25 million tons, of which, 9.6 million tons are for energy use. The magnitude of coal consumption within such a small area is the cause of these high pollutant concentrations. The situation in winter is even worse due to the city's heat-supply system.
The research area, urban core of Taiyuan City, is divided into six administrative regions, Yingze, Wanbolin, Xinghualing, Xiaodian, Jingyuan, and Jiancaoping, as shown in This area was compartmentalized into 33 × 16 grids; each of them was 500 × 500 m and was tagged by horizontal and vertical numbers. As PM monitoring stations were located within the grids, the monitoring PM10 concentration was considered as the concentration of the grid.
Data
The technical flowchart of the present study is presented in The input data for ANN model are meteorological data, topographic data, emission data, and grid distance data from emission sources to the monitoring stations. The output data for ANN model are PM10 monitoring data. These data are explained in more detail below.
PM10 monitoring data were collected from the urban air quality monitoring network of Taiyuan Environment Monitoring Central Station. The air quality monitoring network includes nine stations (S1, S2, …, S9), which are shown in PM10 concentration was determined by RP1400a type atmospheric automatic sampler, which is a fully automatic operation with automatic sampling, automatic analysis, and real-time data/data returned in real time. Quality control of PM10 concentration data means that data were regularly calibrated by zero gas and standard gas, and corrected by manual monitoring analysis method. The temporal resolution of PM measured data was daily average concentration. However, the yearly average concentration, calculated by daily average concentration, was used as ANN model input data for training and simulation. The amount of the data is 81 (N = 81, nine stations, from 2000 to 2008).
Meteorological data were collected from the weather monitoring network of Taiyuan Meteorological Monitoring Central Station. As shown in , the weather monitoring network in research area includes three automatic meteorological stations (W1, W2, W3). Meteorological data, obtained from automatic meteorological station of Taiyuan, include six variables, which are rainfall, temperature, pressure, stability class, wind speed, and wind direction from 2000 to 2008. Quality control of meteorological data mainly concentrated on eliminating missing data and obviously unreasonable data. Meteorological data of every grid of the research area was interpolated with nearest neighbor algorithm and the time resolution was yearly average.
Topographic data were latitude, longitude, and elevation of every grid of the research area (33 × 16 grids), they were interpreted by remotely sensed image and digital elevation model (DEM). The topographic data were also used to train the ANN model.
Emission data were collected from the local Environmental Protection Bureau. The data here include five variables, which are the yearly average volume of industrial soot emissions, locations, productions, speed, and the characteristics of the enterprises of the emission sources. The research area is the urban core of Taiyuan City, and the main function of the research area is administration and commerce. So there are not many emission sources there. According to the pollution sources survey in 2007 in Taiyuan, there are a dozen individual emission sources in the research area, and the three emission sources (E1, E2, E3) shown in are the main ones. However, these three sources are actually the three largest coal-consumption groups of the city, including a set of subsidiaries, and the soot emission is the total ratio of coal consumption of the three enterprises, which is more than 80% of that of the research area. Traffic is also a major contributor to PM10; however, as there's still a lack of relevant data to be evaluated at present in Taiyuan City, it was unable to estimate the influence of traffic to PM10.
The population data from 2000 to 2008 were obtained from the statistical data of Taiyuan. The temporal resolution of population density data was yearly average. Population density in each grid was approximately considered as the population density of the administrative regions and was used to calculate IFs and then to estimate the marginal health effects.
As shown in , the meteorological yearly average data (rainfall, temperature, wind speed and wind direction, atmospheric pressure), topographic data (latitude, longitude, elevation), the yearly average emission data (emission speed, emission volume), and grid distance data from emission sources to the monitoring stations are the input data, and the yearly average PM10 monitoring data are the output data to train the ANN network. The amount of the data is 81 (N = 81). We randomly selected 80% of the original data (n = 65) as the training sample and the other 20% (n = 16) as the verification sample. Once the ANN network was trained well, which was inflected by error between forecasting values and monitoring values, the PM10 exposure concentration of all the grids in the research area was calculated by the trained ANN net. Then, the PM10 exposure concentration data, population density, and breathing data were given into the IF model to calculate the IFs, which were the basis of assessing the health benefit of the research area.
ANN model
ANNs are powerful tools with a proven efficiency in dealing with complex, nonlinear problems, and particularly in the fields of association, classification, and prediction (CitationOrdieres et al., 2005). In this paper, we applied a BPANN to predict the PM10 exposure concentrations of urban area in Taiyuan.
Typically, forecasting with a BP machine involves two steps: training and simulation. Training of networks is normally performed in a supervised manner. With back propagation, the input data are repeatedly presented to the neural network. With each presentation, the output of the neural network is compared with the desired output and an error is computed. This error is then fed back (back propagated) to the neural network and is used to adjust the weights, so that the error decreases with each iteration and the neural model gets closer and closer to the desired output. This process is known as “training.” This kind of training entails an optimization process (CitationSharda, 1994).
At the end of the training period, the final configuration of the weights is crystallized and the network can be used in a simulation stage in order to perform forecast. The knowledge acquired from the neural network through the learning process is tested by applying new data it has never seen before, called the testing set. The network should be able to generalize and have an accurate output for this unseen data (CitationVahidinasab, 2008).
BPANN is a supervised learning algorithm that aims at reducing overall system error to a minimum (CitationBoznar et al., 1993). This algorithm has made multilayer neural networks suitable for various prediction problems. When designing a network, the chosen initial transfer function is applied to each layer of the network. A transfer function is typically a nonlinear function that transforms the weighted sum of the effective inputs to a potential output value. To provide basis for development of the procedure for prediction of PM10, the neural network used to find relation among PM10 and emission source data, meteorological variables, terrain complexity of the area, and grid distance from emission sources to the monitoring station. There are various types of learning rules and transfer functions, but for ANN network employed in this study, the transfer functions used were sigmoid.
The mean absolute error (MAE) and the correlation factor (R 2) were used to provide a numerical description of the accuracy of the estimates.
Intake fraction model
An assessment of human exposure to PM10 from emissions of research area is essential to identify and characterize the industry sources of health damages, which in turn are necessary for devising efficient control strategies.
Our aim here is to make such an assessment using an emission-to-exposure pathway called the intake fraction (IF), and the fraction of a pollutant released is inhaled by a receptor population. The intake fraction model was proposed to determine the prioritization of harmful chemicals. Smith developed the model, and it was first used in environmental assessment (CitationSmith and Ahuja, 1990; CitationSmith, 1993). In the early 1990s, Evan developed the concept of intake fraction and put forward that IF is the proportion of ingestion or inhalation emissions in total emissions from the source (CitationEvans et al., 2002). At present, IF is widely used in the assessment of health effects of atmospheric pollutants (CitationJolliet and Crettaz, 1997; CitationThompson and Evans, 1997; CitationMarshall and Behrentz, 2005; CitationMarshall et al., 2005; Wang et al., 2006; CitationZhou et al., 2006). Its basic formula can be summarized as follows:
where I is the total amount of human intake of the pollutants, g; E is the total emission of pollutants, g; Pj is the population at location j, which is collected from the local statistical department; Cj is the exposure concentration at location j caused by emissions; in this paper, Cj is the annual average PM10 concentration calculated by the trained ANN model, g/m3; Br is the breathing rate, m3/sec (Layton assumed 12 m3/day as a constant breathing rate equal to the estimated lifetime of the population [CitationLayton, 1993]. Here, we assume a constant breathing rate of 20 m3/day); and EM(t) is the source emission at time t (g/sec).
Results
Model testing and validation
The training set (n = 65) consisted of 80% of all sampling stations data were selected randomly for training the model. The remaining 20% (n = 16) were used to test the model's validation once the model was developed. The training process of ANN model was performed by using the software MATLAB, in particular the tool box Neural Networks, developed by the MathWorks (Natick, MA). The simulator iteratively adjusted the weights until the error between the output data and the measured data was minimized. There was one hidden layer with 27 neurons of the BP model. The model predictions (calculated value) were then compared with monitoring value, and statistical numerical measures were then calculated. The best results were obtained as shown in The dotted line in was the monitoring data of all 9-station 2000–2008 year average of PM10 concentration. The solid line was the data calculated by ANN of all 9-station 2000–2008 year average of PM10 concentration. We can see that there was a close fit between monitoring value and the model's calculated value.
Figure 3. Comparison of PM10 concentration between calculated value and monitoring value. PM10 monitoring concentration for all the stations from 2000 to 2008 (); PM10 calculated concentration by the ANN model for all the stations from 2000 to 2008 (——).
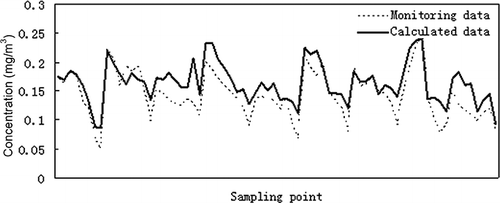
The R 2 values for the test set (n = 16) was 0.7203 (). This result indicated that approximately 72% of the variability in the PM10 concentration levels could be explained by the selected independent variables and by the data used for model development. The MAE between all of the input data and output data was 0.1%. The results showed that the neural network model was able to produce reasonably accurate predictions. Having trained the network successfully, the PM10 concentration of every grid based on the trained ANN model was simulated and the temporal-spatial distribution of the research area was estimated.
ANN-based predictions of PM10 concentrations
The annual average PM10 concentration of every grid from 2001 to 2008 was calculated on the basis of the trained ANN model.
Temporal trend of PM10 concentration of the research area from 2001 to 2008 was described in . The annual average PM10 concentration in Taiyuan fluctuated significantly from 0.048 (the minimum) to 0.299 (the maximum) mg/m3, with a mean of 0.176 mg/m3. It showed that the annual average PM10 concentrations of the research area decreased with time because of the rigorous environmental policy of the local government in recent years. However, the average was still higher than the average daily concentration of 65 μg/m3 recommended by U.S. Environmental Protection Agency (CitationEPA, 1997).
Table 1. Descriptive statistic of PM10 concentration in period of 2001 to 2008 (mg/m3)
Spatial distribution of PM10 concentrations of the research area from 2001 to 2008 was also described, and in , the case of 2002 was listed as an example. showed that the PM10 concentrations between administrative regions can be described as Jinyuan > Jiancaoping > Yingze > Xinghualing > Wanbolin > Xiaodian. The spatial distribution of PM10 concentrations was influenced by many factors, such as source emissions, meteorological conditions, and topographic factors. As there are many factors influencing the spatial distribution of PM10 in a regional scale, and the availability and accuracy of measured data of the influence factors are limited, it is difficult to model the spatial distribution exactly between emission sources and recipient area. Consequently, this paper just attempts to describe the relationship among the emission source of PM, meteorological conditions, terrain complexity, and PM10 spatial distribution. It can at least give us some useful information about where the key control areas are and which the important factors are, so as to provide some suggestion for PM reduction and control. shows that places near emission sources have a relatively high concentration of PM10 than other areas, decreasing with distance from the source. It was found that wind direction and wind speed played an important role in influencing the spatial distribution of PM10, whereas temperature and humidity had a lower effect than expected.
Figure 5. Spatial distribution of PM10 concentration of research area in 2002. Spatial distribution shown on the figure is produced by neural network model for each of the shown grid cells.
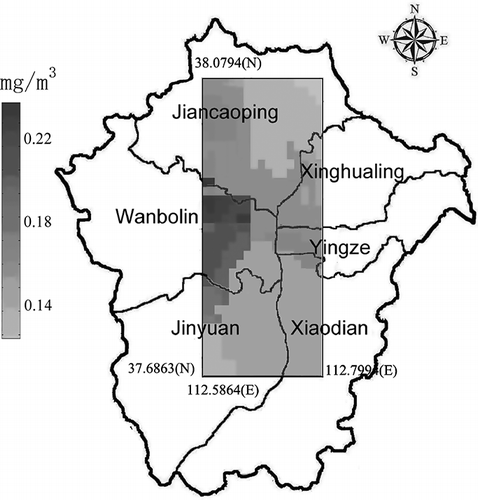
Comparing PM10 concentrations of the research area with that of other cities in China, it was found that the annual average PM10 concentration in Taiyuan with a mean of 0.202 mg/m3 in 2002 was lower than that in Nanjing with a mean of 0.280 mg/m3 in 2002, but it was higher than that in other cities in China, such as Beijing, with a mean of 0.175 mg/m3, the 0.141 mg/m3 of Urumqi, the 0.133 mg/m3 of Yinchuan, the 0.123 mg/m3 of Tianjin, and the 0.115 mg/m3 of Jinan in 2002 (CitationWang et al., 2002; CitationBi et al., 2007; CitationWang et al., 2008).
IF-based health estimates of PM10
With PM10 concentrations calculated and mapped into fine grid cells as described above, by integrating the population of the region, the breathing rate, and the source's emission, IF values were calculated according to Equationeq 1.
Temporal trend of IFs of the research area from 2001 to 2008 was described by high-low chart in The annual average IF estimates of Taiyuan fluctuated significantly from 2.28 per million in 2001 to 8.89 per million in 2007. The IF mean in urban area of Taiyuan was 8.5 per million and in suburb area 4.61 per million. In order to compare IF variation with PM10 concentration variation, we also listed the PM10 concentrations (enlarged 10 times) from 2001 to 2008 in It showed that there was a consistent fluctuation between the IFs and the PM10 concentrations. IFs increased when PM10 concentrations increased, and IFs decreased when PM10 concentrations decreased, especially from 2001 to 2004. However, this fluctuation was not very clear from 2005 to 2008, and the explaining factors for the fluctuation of IFs were both the population density and the PM10 concentrations.
Figure 6. Temporal trend of IF and PM10 of research area in the period of 2001 to 2008. PM10 yearly average concentration enlarged 10 times (mg/m3) from 2000 to 2008 (); IF value (per million) from 2000 to 2008 (——). Max = maximum yearly value of six administrative regions; Min = min yearly value of six administrative regions; Mean = average yearly value of six administrative regions.
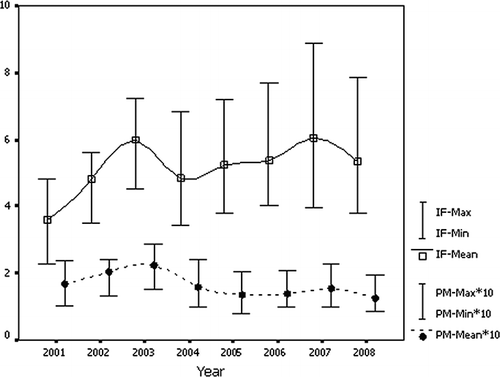
Spatial variations of IFs in the research area were simulated by a trend surface analysis method. The spatial distribution of IF in the research area in 2002 was listed in as an example. It showed that the IF value magnitude between administrative regions can be described as Yingze > Xinghualing > Wanbolin > Xiaodian > Jiancaoping > Jinyuan. By contrasting the distribution of IFs in 2002 () with the distribution of PM10 concentrations in 2002 (), which can be described as Jinyuan > Jiancaoping > Yingze > Xinghualing > Wanbolin > Xiaodian, we can see the difference in spatial distribution. An area with high PM10 exposure concentration does not necessarily have a high IF value. We concluded that this difference was caused by the distribution of population density in the research area, and the population density in different administrative regions varied significantly (). The Yingze region reached 4115 person/km2, the Xinhualing region reached 3063 person/km2 in 2002, and the other regions were under 1610 person/km2 at the time. IF, on the one hand, has a relation with the PM10 exposure concentration, but on the other hand, it depends more on the population density of research regions.
Table 2. Population density of research area in period of 2001 to 2008 (person/km2)
Intake fractions can be used to provide a rapid estimate of local human health damage due to PM10 emissions from industrial emissions. These simple estimates of damage should be useful for guiding pollution control priorities across different emission categories, and they can serve as a basis for the benefit-cost analysis of control strategies.
Comparison of intake fraction estimates with other researches
The IF of Taiyuan City calculated in this study was compared with the IFs of other cities to clarify the relative health risk of PM10 in different cities and different countries. The results were shown in . The results in this study are only for the main stationary sources of the research area, and the traffic sources have not been included. The computed IF results are therefore underestimations. We can see that the IF estimates of PM10 emissions in Taiyuan were relatively high compared with those observed in the United States, northern Europe, and other cities of China. The IFs of Taiyuan urban area, with a mean of 8.5 per million, were higher than those of five other cities in China, with a mean of 4.4 per million in Wang's results. Even in suburb area of Taiyuan, the IF value reached 4.61 per million. Population density is one of the most important factors for the difference of IFs among cities. The average population density of Yingze was more than 4800 person/km2; even in the suburbs, it was still more than 627 person/km2. The location of emission sources also influences IF. For example, three emission sources at the present study located in the urban area caused a high-level exposure concentration of PM10.
Table 3. Comparison of intake fraction estimates with other studies
Discussion and Conclusion
It is interesting to discuss to what extent the present methodology is capable of discriminating between different sources of uncertainty inherent in air quality modeling and health effect estimation. There are several influential factors contributing to the diversity of PM10 concentrations and IF estimates, including urban industrial distribution, urban landscape patterns, and meteorological parameters, of which the population density is probably the most important. The precision of all input parameters in computing may have an impact on model uncertainty. These uncertainties include the following: (i) The error caused by meteorological data. The precision of meteorological data is extremely important for determining the credibility of the distribution of PM10 exposure concentrations. Therefore, increasing the number of meteorological monitoring sites and collecting long-term, extensive monitoring data will reduce errors. (ii) The error caused by PM10 monitoring data. PM10 data are the key and kernel variables of the ANN model. Therefore, increasing atmosphere monitoring sites and distributing the samplings rationally will reduce the errors. (iii) The error caused by PM10 emission data. The PM10 emission data should include all the PM10 emission sources in the research area. We ignored the exposure concentration caused by minor PM10 emission sources and traffic emission, even through it does take a small proportion of IF. The computed IF results are therefore underestimations. Furthermore, release heights of emission sources are crucial for PM10 dispersion and health effects; however, it was not considered in PM10 forecasting because we couldn't collect the information of release heights. It should be considered in further study.
In this paper, ANN-based PM10 concentration forecasting and spatial variation are presented. The presented ANN model can produce reliable simulations of not only the PM10, but also other air pollutant concentration levels. Although the present ANN model is only valid for Taiyuan, the ANN-based approach can be applied to other urban areas as well. When correctly designed, it could describe the nonlinearities of the atmosphere to a specific meteorology and terrain spatial domain features. Therefore, a key factor in their application is the understanding of the domain and studied phenomena peculiarities. This is achieved by integrating domain knowledge in the definition of nonlinear model structure, such as properly selecting the input and output patterns to be used in the identification phase, and the temporal/spatial detail of the neural networks.
In this study, we calculated the temporal-spatial distribution of PM10 concentrations, and estimated the IFs of PM10 in Taiyuan, Shanxi, China. The results show the following: (i) ANN models constitute a useful and effective tool for modeling the complex pollutant dispersion processes, as they are able to extract functional relations between model inputs and outputs from data without requiring an explicit consideration of the measured data generating process. The ANN model used in this study provided a quite satisfactory accuracy, showing a correlation of 0.72 between the forecast values and the monitoring values, which could meet the requirements of the model. (ii) The annual average PM10 concentration of Taiyuan fluctuated significantly from 0.048 to 0.299 mg/m3, with a mean of 0.176 mg/m3, which is higher than that of the average daily concentration recommended by the EPA. (iii) The annual average IF estimates of Taiyuan fluctuated significantly from 2.28 per million in 2001 to 8.89 per million in 2007. The IF mean in urban areas of Taiyuan was 8.5 per million and in suburb area 4.61 per million. Population density is the major factor that influences the spatial distribution of IFs among different areas. Exposure concentration of PM10 is the major factor that influences the variation trend of IF in time. (iv) The IF estimates of Taiyuan are relatively high compared with other IFs of the United States, northern Europe, and other cities in China. Also, the IFs of power plant emissions in China are higher than that in the United States and in northern Europe.
Acknowledgment
This work was supported by the National Natural Science Foundation of China (grant nos. 41101558 and 41271513). The authors also acknowledge and appreciate the useful comments of reviewers, which helped us improve the paper.
References
- Berico , M. , Luciani , A. and Formignani , M. 1997 . Atmospheric aerosol in urban area—Measurements of TSP and PM10 standards and pulmonary deposition assessments . Atmos. Environ. , 31 : 3659 – 3665 . doi: 10.1016/S1352-2310(97)00204-5
- Bi , XH , Feng , YC. , Wu , JH. , Wang , YQ. and Zhu , T. 2007 . Source apportionment of PM10 in six cities of northern China . Atmos. Environ. , 41 : 903 – 912 . doi: 10.1016/j.atmosenv.2006.09.033
- Boznar , M. , Lesjak , M. and Mlakar , P. 1993 . A neural network-based method for short-term predictions of ambient SO2 concentrations in highly polluted industrial areas of complex terrain . Atmos. Environ. , 27B : 211 – 230 . doi: 10.1016/0957-1272(93)90007-S
- Carlton , AG , Turpin , JB. , Johnson , W. , Buckley , BT. , Simcik , M. , Eisenreich , SJ. and Porcja , RJ. 1999 . Methods for characterisation of personal aerosol exposures . Aerosol Sci. Technol. , 31 : 66 – 80 . doi: 10.1080/02786829909709777
- Caselli , M , Trizio , L. , de Gennaro , G. and Ielpo , P. 2008 . A simple feed forward neural network for the PM10 forecasting: Comparison with a radial basis function network and a multivariate linear regression model . Water Air Soil Pollut. , 201 : 365 – 377 . doi: 10.1007/s11270-008-9950-2
- Chaloulakou , A , Assimacopoulos , D. and Lekkas , T. 1999 . Forecasting daily maximum ozone concentrations in the Athens basin . Environ. Monit. Assess. , 59 : 97 – 112 . doi: 10.1023/A:1005943201063
- Charlson , RJ and Rodhe , H. 1982 . Factors controlling the acidity of natural rain water . Nature , 295 : 683 – 685 . doi: 10.1038/295683a0
- Comrie , A.C. 1997 . Comparing neural networks and regression models for ozone forecasting . J. Air Waste Manage. Assoc. , 47 : 653 – 663 . doi: 10.1080/10473289.1997.10463925
- Donaldson , K , Mills , N. , MacNee , W. , Robinson , S. and Newby , D. 2005 . Role of inflammation in cardiopulmonary health effects of PM . Toxicol. Appl. Pharmacol. , 207 : 483 – 488 . doi: 10.1016/j.taap.2005.02.020
- Evans , JS , Wolff , SK. and Phonboon , K. 2002 . Exposure efficiency: An idea whose time has come . Chemosphere , 49 : 1075 – 1091 . doi: 10.1016/S0045-6535(02)00242-4
- Greco , SL , Wilson , AM. , Spengler , JD. and Levy , JI. 2007 . Spatial patterns of mobile source particulate matter emissions-to-exposure relationships across the United States . Atmos. Environ. , 41 : 1011 – 1025 . doi: 10.1016/j.atmosenv.2006.09.025
- Grivas , G and Chaloulakou , A. 2006 . Artificial neural network models for prediction of PM10 hourly concentrations, in the greater area of Athens, Greece . Atmos. Environ. , 40 : 1216 – 1229 . doi: 10.1016/j.atmosenv.2005.10.036
- Intergovernmental Panel on Climate Change . 2007 . The Physical Science Basis: Contribution of Working Group I to the Fourth Assessment Report of the Intergovernmental Panel on Climate Change , Geneva : Intergovernmental Panel on Climate Change .
- Jolliet , O and Crettaz , P. 1997 . A life cycle impact assessment methodology including fate and exposure . Int. J. Life Cycle Assess. , 2 : 104 – l10 . doi: 10.1007/BF02978768
- Jorquera , H. , Palma , W. and Tapia , J. 2000 . An intervention analysis of air quality data at Santiago, Chile . Atmos. Environ. , 34 : 4073 – 4084 . doi: 10.1016/S1352-2310(00)00161-8
- Kukkonen , J. , Harkonen , J. , Karppinen , A. , Pohjola , M. , Pietarila , H. and Koskentalo , T. 2001 . A semi-empirical model for urban PM10 concentrations, and its evaluation against data from an urban measurement network . Atmos. Environ. , 35 : 4433 – 4442 . doi: 10.1016/S1352-2310(01)00254-0
- Layton , D.W. 1993 . Metabolically consistent breathing rates for use in dose assessments . Health Phys. , 64 : 23 – 36 . doi: 10.1097/00004032-199301000-00003
- Levy , JI , Wilson , AM. , Evans , JS. and Spengler , JD. 2003 . Estimation of primary and secondary particulate matter intake fractions for power plants in Georgia . Environ. Sci. Technol. , 37 : 5528 – 5536 . doi: 10.1021/es034484l
- Longhetto , A , Giacomelli , L. , Giraud , C. and Zaramella , G. 1992 . A study of correlation among solar energy, atmospheric turbidity and pollutants in urban area . Atmos. Environ. B Urban Atmos. , 26 : 29 – 43 . doi: 10.1016/0957-1272(92)90035-Q
- Marshall , JD and Behrentz , E. 2005 . Vehicle self-pollution intake fraction: Children's exposure to school bus emissions . Environ. Sci. Technol. , 39 : 2559 – 2563 . doi: 10.1021/es040377v
- Marshall , JD , Teoh , SK. and Nazaroff , WW. 2005 . Intake fraction of nonreactive vehicle emission in US urban areas . Atmos. Environ. , 39 : 1363 – 1371 . doi: 10.1016/j.atmosenv.2004.11.008
- Mukerjee , S. 2001 . Selected air quality trends and recent air pollution investigations in the US-Mexico border region . Sci. Total Environ. , 276 : 1 – 18 . doi: 10.1016/S0048-9697(01)00782-3
- Ordieres , J. B. , Vergara , E.P. , Capuz , R.S. and Salazar , R.E. 2005 . Neural network prediction model (PM2.5) for fine particulate matter on the US-Mexico border in E1 Paso (Texas) and Ciudad Juarez (Chihuahua) . Environ. Modell. Softw. , 20 : 547 – 559 . doi: 10.1016/j.envsoft.2004.03.010
- Papanastasiou , D. , Melas , D. and Kioutsioukis , I. 2007 . Development and assessment of neural network and multiple regression models in order to predict PM10 levels in a medium-sized Mediterranean city . Water Air Soil Pollut. , 182 : 325 – 334 . doi: 10.1007/s11270-007-9341-0
- Pérez , P. and Trier , A. 2001 . Prediction of NO and NO2 concentrations near a street with heavy traffic in Santiago, Chile . Atmos. Environ. , 35 : 1783 – 1789 . doi: 10.1016/S1352-2310(00)00288-0
- Pérez-Roa , R , Castroa , J. , Jorqueraa , H. , érez-Correaa , JR. P and Vesovic , V. 2006 . Air-pollution modeling in an urban area: Correlating turbulent diffusion coefficients by means of an artificial neural network approach . Atmos. Environ. , 40 : 109 – 125 . doi: 10.1016/j.atmosenv.2005.09.032
- Sanhueza , E. 2001 . Hydrochloric acid from chlorocarbons: A significant global source of background rain acidity . Tellus , 53B : 122 – 132 . doi: 10.1034/j.1600-0889.2001.d01-11.x
- Sharda , R. 1994 . Neural networks for the OR/MS analyst: An application bibliography . Interfaces , 24 : 116 – 130 . doi: 10.1287/inte.24.2.116
- Smith , KR and Ahuja , DR. 1990 . Toward a greenhouse equivalence index: The total exposure analogy . Climatic Change , 17 : 1 – 7 . doi: 10.1007/BF00148996
- Smith , KR. 1993 . Fuel combustion, air pollution exposure, and health: The situation in developing countries . Annu. Rev. Environ. Resour. , 18 : 529 – 566 . doi: 10.1146/annurev.energy.18.1.529
- 2009 . Evaluation of the European population intake fractions for European and Finnish anthropogenic primary fine particulate matter emissions . Atmos. Environ. , 43 : 3052 – 3059 . Tainio M., Sofiev M., Hujo M., Tuomisto JT., Loh M., Jantunen MJ., Karppinen A., Kangas L., Karvosenoja N., Kupiainen K., Porvari P., Kukkonen, J.doi:10.1016/j.atmosenv.2009.03.030
- Thompson , KM and Evans , JS. 1997 . The value of improved national explore information for perchloroethylene: A case study for dry cleaners . Risk Anal. , 17 : 253 – 271 . doi: 10.1111/j.1539-6924.1997.tb00864.x
- Tiittaa , P. , Raunemaa , T. , Tissari , J. , Yl-Tuomi , T. , Leskinen , A. , Kukkonen , J. , Harkonen , J. and Karppinen , A. 2002 . Measurements and modeling of PM2.5 concentrations near a major road in Kuopio, Finland . Atmos. Environ. , 36 : 4057 – 4068 . doi: 10.1016/S1352-2310(02)00309-6
- U.S. Environmental Protection Agency, Office of Air and Radiation, Office of Air Quality Planning and Standards . 1997 . Fact Sheet—EPA's Recommended Final Ozone and Particulate Matters Standards , Research Triangle Park , NC : U.S. Environmental Protection Agency .
- Vahidinasab V, Jadid S, Kazemi A . 2008 . Day-ahead price forecasting in restructured power systems using artificial neural networks . Electr. Pow. Syst Res. , 78 : 1332 – 1342 . doi: 10.1016/j.epsr.2007.12.001
- van Zelm , R. , Huijbregts , M.A.J. den Hollander , H.A. 2008 . European characterization factors for human health damage of PM10 and ozone in life cycle impact assessment . Atmos. Environ. , 42 : 441 – 453 . doi: 10.1016/j.atmosenv.2007.09.072
- Wang , GH , Huang , LM. and Gao , SX. 2002 . Measurements of PM10 and PM2.5 in urban area of Nanjing, China and the assessment of pulmonary deposition of particle mass . Chemosphere , 48 : 689 – 695 . doi: 10.1016/S0045-6535(02)00197-2
- Wang , HL , Zhuang , YH. and Wang , Y. 2008 . Long-term monitoring and source apportionment of PM2.5/PM10 in Beijing, China . J. Entomol. Sci. , 20 : 1323 – 1327 . doi: 10.1016/S1001-0742(08)62228-7
- Wang , SX , Hao , JM. , Ho , MS. , Li , J. and Lu , YQ. 2006 . Intake fractions of industrial air pollutants in China: Estimation and application . Sci. Total Environ. , 354 : 127 – 141 . doi: 10.1016/j.scitotenv.2005.01.045
- Wolff , SK. 2000 . Evaluation of fine particle exposures, health risks, and control options , Doctoral dissertation .
- Wu , JH , Zhu , T. , Feng , YC. , Bi , XH. and Li , JP. 2008 . Analytic studies on source of TSP and PM10 in environmental air of Taiyuan City [in Chinese] . Urban Environ. Urban Ecol. , 21 : 40 – 43 .
- Zhang , DS , Aunan , K. and Seip , HM. 2010 . The assessment of health damage caused by air pollution and its implication for policy making in Taiyuan, Shanxi, China . Energy Policy , 38 : 491 – 502 . doi: 10.1016/j.enpol.2009.09.039
- Zhou , Y , Levy , JI. , Evans , JS. and Hammitt , JK. 2006 . The influence of geographic location on population exposure to emissions from power plants throughout China . Environ. Int. , 32 : 365 – 373 . doi: 10.1016/j.envint.2005.08.028