Abstract
Changes in life expectancy (LE) across metropolitan areas in the United States have been associated with substantial differential reductions in fine particulate matter (aerodynamic diameter <2.5 μm; PM2.5) air pollution that occurred during the 1980s and 1990s. It has been suggested that a single influential observation was largely responsible for the statistically significant LE-PM2.5 associations. In this paper, the role of influential observations is further explored. Stable and statistically significant LE-PM2.5 associations are observed in analyses that control for available socioeconomic, demographic, and proxy smoking variables and that use robust regression procedures that are relatively resistant to influential observations. These associations are not dependent upon the inclusion or exclusion of any single observation.
These results contribute to the large and growing literature indicating that exposure to fine particulate matter air pollution has substantive adverse effects on human health. These results, however, also provide encouraging evidence that the improvements in air quality that occurred during the 1980s and 1990s contributed to measurable improvements in human health and life expectancy in the United States.
Introduction
In the accompanying “Notebook Paper,” CitationKrstić (2012) presents some limited reanalysis related to our 2009 New England Journal of Medicine (NEJM) findings that reductions of fine particulate matter (aerodynamic diameter <2.5 μm; PM2.5) air pollution were associated with improved life expectancy (LE) in the United States. It is asserted that the association between reductions in PM2.5 and life expectancy “is lost after removing one of the metropolitan areas from the regression analysis.” This assertion is a selective interpretation and not consistent with more comprehensive analyses of the data.
It is unusual for a paper to be published twice in the same journal. However, the Editor deems that the Notebook Paper by Goran Krstic´ (first published in September 2012) and the response by Pope et al. appear in the same issue because of their implications for public policy on health effects and control strategies on particulate matter in the atmosphere.
Details regarding data and methods are provided in the original paper (CitationPope et al., 2009). Succinctly, differential changes in LE and PM2.5 that occurred during the 1980s and 1990s in the United States were evaluated. First-difference regression models were used to estimate the LE-PM2.5 associations, adjusting for changes in socioeconomic, demographic, and proxy smoking variables. Results from various regression models and various sensitivity analyses are reported in the original paper. Primary findings were that when controlling for socioeconomic, demographic, and proxy smoking variables, a 10 µg/m3 decrease in PM2.5 was associated with an estimated increase in mean (± SE) LE of 0.61 ± 0.2 yr when all 211 counties were included in the analysis. When only the 51 largest counties in each metro area were included in the analysis, the effect estimate was larger at 0.95 ± 0.23 yr. This note provides additional sensitivity analysis regarding potentially influential data points (especially the Topeka observation) and statistical outliers.
Results and Discussion
reports expanded sensitivity analyses regarding influential data points. The regression coefficients (and SE, P values, and R 2 values) of change in LE on change in PM2.5 (10 µg/m3) are presented for models using the county-level data for all counties, all counties minus Topeka, the 51 largest counties, the 51 largest counties minus Topeka, and the 51 largest counties excluding outliers and influential observations based on common diagnostics statistics. Models with no covariates and models controlling for available covariates are estimated. Models with and without clustering and models estimated using standard robust regression procedures to provide results that are resistant to the presence of outliers and influential observations are presented. The standard robust regression procedures include M estimation, S estimation, and MM estimation (using PROC ROBUSTREG, SAS 9.3; SAS Institute Inc., Cary, NC, USA). In-depth treatments and discussions of these robust regression procedures are provided elsewhere (CitationAndersen, 2008; CitationChen, 2002).
Table 1. Expanded sensitivity analysis regarding potentially influential data points with estimated regression coefficients (and SE, P values, and R 2 values) of change in life expectancy on change in PM2. 5 (10 µg/m3) for selected data sets and regression models
For comparison purposes, four of the models presented in (identified by asterisks) are identical to representative models reported in the original paper (CitationPope et al., 2009). When these identical models are used, the results originally reported are exactly replicated. Change in LE is significantly associated with change in PM2.5 when data for all 211 counties are used or when the Topeka observation is dropped. As expected, clustering results in larger standard errors, but the associations remain statistically significant. Controlling for socioeconomic, demographic, and proxy smoking variables results in statistically more robust estimated associations between declines in PM2.5 and LE. Estimates of the LE-PM2.5 association are highly stable to the use of the various robust regression procedures.
If the analysis is restricted to data for only the 51 largest central counties in each metro area, if there is no control for any of the available socioeconomic, demographic, and proxy smoking variables, and especially if an influential observation (Topeka) is excluded, the LE-PM2.5 association is not statistically significant. From a study design perspective, the exclusion of Topeka seems arbitrary because it is just one of the 51 metro areas selected based on a priori data availability criteria and it is the only metro area without a significant reduction in pollution. Nevertheless, even with Topeka excluded, when the models control for socioeconomic, demographic, and proxy smoking variables, the estimates of the LE-PM2.5 association are statistically significant and relatively large.
A remarkable conclusion based on the results presented in is that some of largest and most statistically significant estimated LE-PM2.5 associations come from analyses that are restricted to the largest counties in the metropolitan areas, that control for socioeconomic, demographic, and proxy smoking variables, and that use robust regression procedures that are relatively stable to outliers—regardless of whether or not Topeka is included. In these analyses, the estimates range from approximately 0.86 to 1.00 yr of increased LE per 10 µg/m3 decrease in PM2.5 and were strongly statistically significant (P values <0.01).
The potential influence on the LE-PM2.5 association of various observations before and after controlling for socioeconomic, demographic, and proxy smoking variables is further illustrated in Panel A presents changes in LE for each of the 51 most populated central counties in each metro area with the regression line fit through the data. In this plot of unadjusted changes in LE, there is substantial scatter around the regression line, with various observations (including Topeka, number 46) that appear to be influential observations. Panel B of presents residual change in LE controlling for all of the available socioeconomic, demographic, and proxy smoking variables (using covariate coefficients as estimated using Model 6 presented in the original paper). Panel B illustrates that after adjusting for other available relevant variables, there are no dramatic outliers or obvious excessively influential observations and, from a statistical perspective, the residuals are reasonably well behaved.
Figure 1. Changes in life expectancy (A) and residual changes in life expectancy after controlling from available socioeconomic, demographic, and proxy smoking variables (B) for the 1980s–1990s, plotted against reductions in PM2.5 concentrations for 1980–2000 for the most populated central counties in each of the 51 metropolitan areas. The numbers indicate the metropolitan areas as defined in the original paper (CitationPope et al., 2009). R 2 values are the coefficients of determination for each of the fitted curves as illustrated.
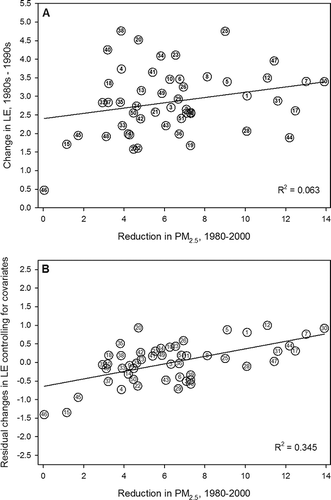
Diagnostics statistics for outliers and influential observations further suggest that the results are not dependent on any single observation. Based on the model controlling for all available socioeconomic, demographic, and proxy smoking variables, illustrated in , there was only one observation (20, Jersey City, NJ) that was a significant outlier (RSTUDENT value > 2) and only three observations (15 [Denver, CO]; 46 [Topeka, KS]; 47 [Washington, DC]) that were highly influential observations (DFBETA for reduction in PM2.5 > size-adjusted cutoff of 0.28). also presents results of this model without and with these exclusions, demonstrating consistently highly statistically significant and stable estimated LE-PM2.5 associations.
Conclusion
The assertion that the association between reductions in PM2.5 and life expectancy is lost after removing the Topeka observation from the regression analysis is a selective interpretation not consistent with more comprehensive analyses of the data. Statistically significant LE-PM2.5 associations are generally not dependent upon the inclusion of Topeka or any other single observation. Analyses that are restricted to the largest counties in the metropolitan areas, that control for available socioeconomic, demographic, and proxy smoking variables, and that use robust regression procedures that are relatively stable to outliers provide some of the largest and most statistically significant estimates of the LE-PM2.5 association—regardless of whether or not any single observation is included or excluded.
References
- Andersen , R. 2008 . Modern Methods for Robust Regression , Thousand Oaks , CA : Sage Publications .
- Chen , C. 2002 . Robust regression and outlier detection with the ROBUSTREG procedure , Cary , NC : SAS Institute . SUGI Paper 265–27
- Krstić , G. 2012 . A reanalysis of fine particulate matter air pollution versus life expectancy in the United States . J. Air Waste Manage. Assoc. , 62 : 989 – 991 . doi: 10.1080/10962247.2012.697445
- Pope , C.A. , Ezzati , M. and Dockery , D.W. 2009 . Fine particulate air pollution and life expectancy in the United States . N. Engl. J. Med. , 360 : 376 – 386 . doi: 10.1056/NEJMsa0805646