Abstract
Dry fly ash disposal involves dropping ash from a truck and the movement of a heavy grader or similar vehicle across the ash surface. These operations are known to produce fugitive particulate emissions that are not readily quantifiable using standard emission measurement techniques. However, there are numerous situations--such as applying for a source air permit--that require these emissions be quantified. Engineers traditionally use emission factors (EFs) derived from measurements of related processes to estimate fly ash disposal emissions. This study near a dry fly ash disposal site using state-of-the-art particulate monitoring equipment examines for the first time fugitive emissions specific to fly ash handling at an active disposal site. The study measured hourly airborne mass concentrations for particles smaller than 2.5 μm (PM2.5) and 10 μm (PM10) along with meteorological conditions and atmospheric turbidity at high temporal resolution to characterize and quantify fugitive fly ash emissions. Fugitive fly ash transport and dispersion were computed using the on-site meteorological data and a regulatory air pollutant dispersion model (AERMOD). Model outputs coupled with ambient measurements yielded fugitive fly ash EFs that averaged 96 g Mg−1 (of ash processed) for the PMc fraction (=PM10 - PM2.5) and 18 g Mg−1 for PM2.5. Median EFs were much lower due to the strongly skewed shape of the derived EF distributions. Fugitive EFs from nearby unpaved roads were also characterized. Our primary finding is that EFs for dry fly ash disposal are considerably less than EFs derived using US Environmental Protection Agency AP-42 Emissions Handbook formulations for generic aggregate materials. This appears to be due to a large difference (a factor of 10+) between fugitive vehicular EFs estimated using the AP-42 formulation for vehicles driving on industrial roads (in this case, heavy slow-moving grading equipment) and EFs derived by the current study.
Fugitive fly ash emission factors (EFs) derived by this study contribute to the small existing knowledge base for a type of pollutant that will become increasingly important as ambient particulate standards become tighter. In areas that are not in attainment with standards, realistic EFs can be used for compliance modeling and can help identify which classes of sources are best targeted to achieve desired air quality levels. In addition, understanding the natural variability in fugitive fly ash emissions can suggest methods that are most likely to be successful in controlling fugitive emissions related to dry fly ash storage.
Introduction
The U.S. Environmental Protection Agency (EPA) defines fugitive emissions in Title V (parts 70 and 71) of the Clean Air Act as emissions that cannot “reasonably pass through a stack, chimney, vent, or other functionally-equivalent opening” (see Code of Federal Regulations, CFR, CFR title 40, part 70.2, 2011). This definition includes gases, liquid droplets, and solid particulate matter. Emissions streams that pass through a vent, stack, or chimney are confined, making them relatively easy to sample. By contrast, fugitive emissions are not confined and that makes quantifying them a challenge.
Despite the difficulties measuring them, fugitive emissions can often comprise a large portion of the total emissions associated with a source that is required to obtain an air permit. Fugitive particulate matter emissions (hereafter, “fugitive emissions”) can also be difficult to control. They must be considered when determining whether a source adversely impacts attainment of ambient air quality standards. In addition, fugitive emissions must be addressed for New Source Review and Prevention of Significant Deterioration impact analyses for electric generating units larger than 250 million BTU per hour heat input (CFR title 40, part 51, §166, 2008). Fugitive emissions modeling is generally required if annual emissions of a pollutant exceed certain thresholds. These thresholds are 10 English tons per year (tpy) for fine particle (PM2.5) mass and 15 tpy for particles <10 µm in size (PM10). Accurate emissions estimates may, in many situations, determine whether annual emission estimates fall above or below the threshold for modeling. Quantifying fugitive emissions is especially important to source operators that handle granular materials (e.g., coal, fly ash, limestone) or that operate vehicles on unpaved surfaces.
Quantifying fugitive emissions for air permitting purposes generally relies on published emission factors. These factors relate the amount of particulate material emitted into the air from a specific process to some more easily quantifiable aspect of the process. For example, a formula exists (U.S. CitationEPA, 1995, and subsequent updates) that estimates the amount of fugitive emissions associated with the dumping of a load of material from a dump truck or similar material conveyor. The value determined in this manner—expressed as mass of particulate matter emitted per mass of material deposited—is an emission factor (EF).
This paper describes a study designed to quantify fugitive EFs for particles smaller than 10 and 2.5 micrometers (PM10 and PM2.5, respectively) associated with dry fly ash disposal at a coal-fired power plant. The intent of this work was to develop EFs specific to fly ash storage activities and compare them with EFs derived using standard formulations in the U.S. EPA AP-42 emissions handbook (CitationU.S. EPA, 1995, and subsequent updates). The AP-42 handbook is the widely accepted authoritative source in the United States for estimating EFs for all types of sources and pollutants.
Previous Work
The dry disposal of fly ash requires that ash be dropped or dumped onto the ground from a haul truck and leveled by a grader. Dropping and grading operations produce fugitive dust, and the emissions caused by these types of actions have been parameterized to a limited extent by previous studies. In practice, parametric formulas are used to approximate emissions from a variety of activities even though not all such activities (such as grading fly ash) have been explicitly studied. Hence, there is a need to investigate the applicability of published fugitive emissions formulations. Our review of previous work focuses on fugitive emissions from the dropping of aggregate materials and from the movement of vehicles over surfaces composed of loose, granular materials.
Few emissions data can be found for dropping operations, and only those from CitationCowherd et al. (1974) are readily accessible. Cowherd et al. sampled dust from aggregate storage operations with high-volume filter samplers in the immediate downwind vicinity of the operations. The aggregate storage operations were associated with a sand and gravel pit. Sources of fugitive emissions were a combination of wind erosion from aggregate piles, aggregate dumping (both sand and gravel), and vehicles moving on the unpaved surfaces between piles and dump trucks. High-volume samplers were operated to measure airborne dust concentrations and an Anderson high-volume cascade impactor was used to determine particle size distributions. Five monitors were placed immediately downwind of five aggregate piles (one per pile). None of the monitors consistently recorded the highest concentrations, indicating that the location of the fugitive emission source was not stationary. This analysis was hampered by operations logs that contained only daily summary information and 24-hr particulate concentrations that included periods when no aggregate handling occurred. Data were available to evaluate fugitive emissions as a function of precipitation, wind speed, aggregate size, and activity levels.
Cowherd et al. concluded that wind speed was not a major factor (i.e., wind erosion of dust from storage piles was not important). This is not surprising because (1) the study occurred in Ohio during the summer when winds are generally not very strong and (2) the sand and gravel piles contained mostly larger particles not easily eroded by wind. Also, aggregate size was not found to be a predictor of fugitive emissions. Only wetness (“wet” days were defined as those receiving rainfall on the day before or the day of sampled operations) and activity level at the site were significant predictors of fugitive emissions. Estimated EFs across all particle sizes averaged 0.42 lb per ton of material handled (“ton” denotes the unit equal to 2000 pounds). Separate measurements were made by CitationCowherd et al. (1974) near a contrived aggregate offloading operation using a pile of crushed limestone and a front loader. Analysis produced an estimated EF of 0.11 lb/ton of limestone processed, and the airborne dust had a mass mean diameter of 1.4 µm.
As reported in the U.S. EPA AP-42 emissions handbook (CitationU.S. EPA, 1995), material dropping operations were quantified by a U.S. EPA-sponsored study in the 1980s. The AP-42 handbook assigns the EF formulation (factor Edrop ) an “A” rating for the highest level of certainty. Factor Edrop is represented by
where kdrop is a dimensionless particle size fraction multiplier, U is wind speed (m sec−1), and M is the material moisture content (in percent). Units of Edrop are kilograms of airborne particles emitted per megagram of material dropped, and AP-42 defines kdrop as 0.74 for particles <30 µm, 0.35 for particles <10 µm, and 0.053 for particles <2.5 µm. EquationEquation 1 is most applicable when dropping conditions fall within the range of conditions that occurred during the study on which it is based, as follows: material silt content S of between 0.44 and 19%, 0.25 ≤ M ≤ 4.8%, and 0.6 ≤ U ≤ 6.7 m sec−1. Silt content is defined as the fraction of particles <75 µm in size. Note that fly ash as handled at power plants is typically conditioned with moisture to suppress dust so that M > 10%. In addition, S in fly ash is near 100%, well above the range of the data behind Equationeq 1. Thus, if Equationeq 1 is applied to fly ash dropping it is used outside the recommended range of applicability.
One of the earliest (if not the first) comprehensive compilations of particulate fugitive EFs for unpaved roads was made by CitationCowherd et al. (1974). Most references in Cowherd et al. are obscure reports no longer available, and CitationCowherd et al. (1974) must be relied upon as the source of information on these studies. One exception is the work of CitationHoover (1973), who reported measurements of dust deposition near a gravel road. All approaches to quantifying fugitive emissions have used measurements of airborne particles, or particle deposition plus dispersion and deposition estimates, to link measured downwind concentrations or deposition back to emission rates. Some focused on suspended particles across a large size range (3–100 µm diameter), whereas others collected data exclusively on smaller particles (in the 1–10 µm range). The largest particles—especially those >30 µm—tended to deposit quickly and could be captured by simple “dustfall” collectors. Smaller particles were collected on filters from sampling air streams. CitationCowherd et al. (1974) mostly focused on fugitive emissions from paved and unpaved (dirt and gravel) roads. They reported unpaved road fugitive emissions of between 0.5 and 13.9 lb per vehicle mile traveled (lb/VMT) for total particle concentrations, between 0.4 and 5.2 lb/VMT for particles smaller than 10 µm, and between 0.11 and 0.43 lb/VMT for particles smaller than 2 µm.
Section 13.2 in AP-42 lists EFs for various fugitive dust sources, including vehicles traveling on unpaved surfaces. Fugitive emissions associated with wind erosion emissions are not considered by this study because measured wind speed never met the erosion threshold criteria in AP-42. The updated version of the EPA AP-42 handbook contains numerous references to studies of unpaved and paved road fugitive emissions. The only such reference from a peer-reviewed (and thus readily accessible) source is CitationDyck and Stuckel (1976), whose experimental results for unpaved industrial surfaces are described here and are incorporated into the handbook along with data from CitationCowherd et al. (1974) and others.
CitationDyck and Stuckel (1976) deployed a 4.5-ton flatbed truck carrying different weight loads driven along a dry unpaved dirt road while high-volume particulate samplers were operated at varying locations upwind (for background samples) and downwind (4 between 15 and 76 m). Truck speeds—held steady during each experiment—were varied between 4.5 and 11.2 m sec−1 (16–40 km hr−1). Truck weight was varied at 3900, 5700, and 7500 kg and three road types were tested. Experiments each lasted 1 hr and included multiple truck passes. Results computed from each of four downwind air samplers were averaged to yield one EF per experiment. Multivariate regressions were done to determine relationships between EF, truck weight, truck speed, road silt content, road surface moisture content, and wind speed. CitationDyck and Stuckel (1976) computed the fugitive emission rate using a dispersion equation for an infinite line source, measured 1-hr particle concentrations, the number of truck passes per hour, and meteorological data collected nearby.
Variations in road moisture content had no effect on computed EFs, but this is probably because the tests were only conducted during dry conditions. The results suggested a linear relationship between the fugitive EF for dust and the predictors of vehicle weight, speed, silt content, and road type, as follows:
where R is road type (= 0 or 1), V is vehicle speed (miles per hour), W is vehicle weight (tons), and S is road surface silt content (percent). At a speed of about 12 m sec−1, with road silt of 5–20% and for the vehicle weights used in the Dyck and Stuckel experiments, Equationeq 2 yields EFs of between 5 and 30 lb/VMT, similar to those reported by CitationCowherd et al. (1974).
Two AP-42 road dust EF formulations are given, one for traffic on public roads and one for vehicles driving at industrial sites. The latter is the EF formula typically used for estimating dust emitted from heavy machinery such as graders/bulldozers operating at a power plant (its application to grading fly ash is described later). Traffic on public roads is assumed to be primarily from automobiles and small trucks whose speeds vary over a larger range than that for heavy trucks at industrial sites. Thus, the public road formulation allows for vehicle speed but neglects vehicle weight. The opposite is true for the industrial site formulation. Both unpaved road formulations are assigned a quality rating of “B” (one level below the rating for the dropping formulation), but the designation degrades if conditions fall outside those used to derive the formulations.
For industrial unpaved roads or surfaces the AP-42 EF Eir is given as
with kir being a dimensionless particle size fraction parameter (different from kdrop in Equationeq 1), W is vehicle weight (in tons), and exponent a = 0.9 for particles smaller than 10 µm. Values for kir are 1.5 for the PM10 mass fraction and 0.15 for the PM2.5 fraction. Units of Eir are kilograms of airborne particles emitted per vehicle kilometer traveled. Likewise, The AP-42 formulation for Epr (public unpaved roads) is
with kpr = 1.8 for the PM10 mass fraction and 0.18 for the PM2.5 fraction. Exponents c and d are given as 0.2 and 0.5, respectively, for all particles <10 µm. Vehicle speed (V) is in units of miles per hour but the conversion factor has been included in Equationeq 4 to produce Epr in metric units. Parameter Cnf is included to remove the contributions of vehicle fleet exhaust and brake and tire wear that were combined with road dust in the field experiments performed to derive Equationeq 4. AP-42 gives Cnf as 0.00047 and 0.00036 lb/VMT for PM10 and PM2.5, respectively.
The primary applicability of Equationeq 3 is for 2 ≤ S ≤ 25%, 2 ≤ W ≤ 290 tons, V < 70 km hr−1, and M ≤ 13%. Likewise, the applicability of Equationeq 4 is primarily for 2 ≤ S ≤ 35%, 1.5 ≤ W ≤ 3 tons, 16 ≤ V ≤ 88 km hr−1, and M ≤ 13%. Thus, Equationeq 3 is applicable over a much greater vehicle weight range than Equationeq 4, whereas Equationeq 4 is applicable over a greater vehicle speed range than Equationeq 3. These limitations must be remembered when interpreting later comparisons between EFs. The sensitivities of Equationeqs 1, Equation3, and 4 to various physical parameters (e.g., M and S) are illustrated in Supplemental Information. Note that just because W does not appear in Equationeq 4 and V does not appear in Equationeq 3 does not mean that fugitive emissions under those conditions do not respond to W and V. Data scatter tends to be large with these kinds of relationships and it is likely that parameters exhibiting relatively small variations do not become significant predictors when multivariate statistical analyses are performed.
An alternate EF for fugitive dust from nonagricultural unpaved roads was developed by researchers at the University of California–Davis and the Desert Research Institute and adapted by the California Air Resources Board and the Western Regional Air Partnership (CitationWRAP, 2006). This EF is a constant value of 2.27 lb/VMT for PM10 and is scaled down to 0.227 lb/VMT for PM2.5. These values are slightly lower than those produced for California roads using the AP-42 methodology. More recently, CitationGillies et al. (2005) estimated fugitive road dust emitted by various sized vehicles traveling on unpaved roads at speeds as high as 80 km hr−1. Their estimates used light scattering and wind measurements at various heights to calculate total horizontal particle (PM10 mass) fluxes for each vehicle pass at three downwind distances. These experiments confirmed the sensitivity of particulate EFs to vehicle size (including four to eight wheels per vehicle) and speed. Gillies et al. suggested that unpaved road EFs were even greater than those estimated by AP-42 for high vehicle speeds. No data were collected for vehicles with treads (i.e., bulldozers) or for speeds <16 km hr−1.
Despite several studies of emissions from vehicles traversing unpaved surfaces, none duplicated the conditions relevant to fly ash handling, that is, the high moisture content of dropped loads, S ≈100%, and vehicles moving at extremely slow speeds on either treads or specially designed tires for use on loose surfaces. Vehicles with treads or special tires are used because they provide better traction on surfaces composed of loose materials. It is possible that the reduced surface slippage of these vehicles results in less dust emissions than from road tires. Therefore, a need exists to explicitly examine fly ash handling fugitive emissions.
Experimental Method
Approach
The method used to quantify fly ash disposal EFs was—like previous studies—inferential and based on ash handling information, meteorological data, and a transport/diffusion model to link source activity with measured downwind concentrations. An illustration of the monitoring approach is provided in . Air sampling downwind of the fly ash storage area collected data that included impacts from both fly ash and unpaved road dust. Light scattering (so-called “bscat ”) data detected the presence of particle plumes and airborne particle samplers provided information on particle mass concentrations for two particle size ranges. Digital photographs and statistical methods were used to remove the influence of road and construction dust, allowing quantification of the fly ash influence. Records and other observations of ash handling enabled a coupling of simulated (using the atmospheric dispersion model) dust emission estimates with on-site ash handling activity.
Figure 1. Schematic of sampling scheme designed to capture fugitive fly ash particles downwind of a fly ash disposal area. Unpaved roads between the disposal site and monitoring equipment were a major source of confounding emissions.
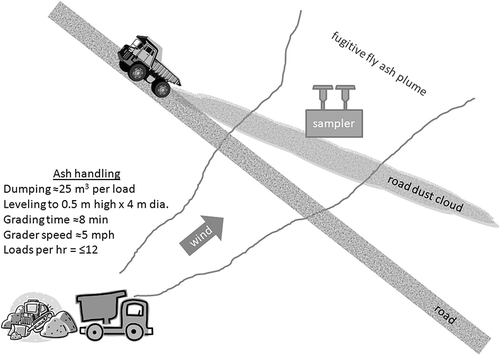
The field measurement campaign was conducted at the 1200-MW Colbert coal-fired electric generating plant operated by the Tennessee Valley Authority in northwest Alabama. Colbert does not operate SO2 removal technology and currently burns about 7000 tons of low-sulfur bituminous coal daily. Fly ash is conveyed pneumatically to hoppers that hold it for transport to the disposal area (the so-called “dry stack”). Fly ash is conditioned (moistened prior to transport) to around 15% moisture and is then transferred to ∼30-ton haul trucks that transport it about 1.5 km to the disposal site. At the dry stack (latitude: 34.731˚ N/longitude: 87.834˚ W) the ash is dumped onto the top of the stack and spread into a pile of uniform height. During the study the stack top was about 30 m above the surrounding ground level and the active disposal area covered roughly 1–2 ha. The terraced sides of the dry stack are covered with short grass. Occasionally, as the ash level rises the exposed outer edge of the ash is covered in clay, an activity that is very infrequent and did not occur during periods analyzed for fugitive emission rates.
Activity logs provide data on the daily amount of fly ash moved to the dry stack, the number of truck loads hauled, and whether other materials were handled at the site. The fly ash disposal foreman reported that each load of ash dumped is leveled by a grader (bulldozer) to a depth of 18–24 inches (0.46–0.61 m). Given the typical volume of ash per truck load, this is equivalent to a circular pile about 3.5 m in radius. Each load requires an average of 8 min for pile leveling work. The average speed of the grader is 5 miles per hour (2.2 m sec−1). This speed constrains the distance traveled by the grader while processing a load to about 1000 m. The processing time allows a maximum of about 7–8 ash loads deposited per hour. However, in some cases up to 12 loads were deposited per hour. We assumed that the grading activity was slightly more efficient during the peak periods (i.e., less than 8 min was needed to level a pile) but that some grading work continued into the next hour. The foreman also reports that piles are typically dumped in contiguous areas which allows for the most efficient means of leveling multiple piles and constrains the ash dumping/processing activities to only a small portion of the dry stack top on any given day. This information was used to model fugitive fly ash dispersion from the storage area and to compute EFs using AP-42 formulations.
Measurements
Detailed meteorological data were collected to characterize site weather (e.g., precipitation amount), airflow variations by direction, atmospheric turbulence, and turbidity. The latter data were critical in detecting particle plumes from nearby fugitive dust sources. Except for the nephelometer (for light scattering measurements) the meteorological instruments were deployed on or near a 10-m tower () in a grassy field located north of the fly ash dry stack. The field had a slight (∼4%) positive elevation gradient from southeast to northwest. This put the base of the tower (located about 60 m northwest of monitoring Site 2) at an elevation about 2–3 m above Site 2. Temperature, relative humidity, and three-dimensional airflow (using sonic anemometers) were measured at 2.3 and 9.6 m above the ground. A net radiometer was mounted at the lower tower level and a tipping bucket rain gauge was located nearby.
Figure 2. Aerial photograph of the area around the fly ash disposal site showing locations for various physical features and monitoring equipment. The photograph predates the study by a few years (all sides and most of the top of the fly ash dry stack were covered by vegetation during the study) and was taken when the grass was dormant. Monitoring sites 2 and 3 (triangles) are labeled. The meteorological tower is represented by a square. A cartoon camera illustrates the location of the video surveillance system. Four circles denote locations of potential ash disposal sites used in the dispersion modeling. The background monitoring site, not in this field of view, was about 1500 m northwest of the dry stack.
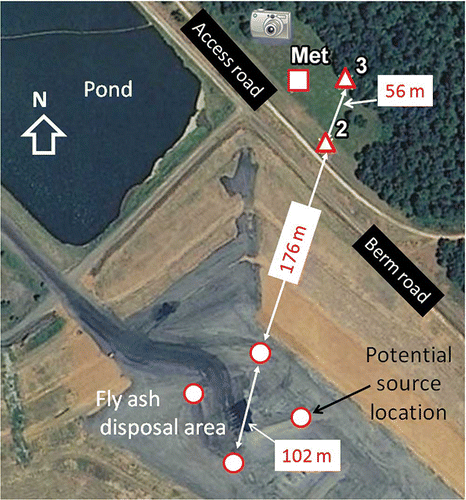
Particle concentration data were needed to calculate the contributions of fugitive sources to total concentrations of PM10 (CPM10 ) or PM2.5 (CPM2.5 ). These measurements were made hourly using Met One beta attenuation monitors (BAMs), a Federal Equivalence Method instrument, located at three sites (a list of measurements and instrumentation is provided in Supplemental Information). Measurements at Site 1 (not shown in ) approximated background conditions, and Sites 2 and 3 were downwind of the fly ash disposal area when winds had a southerly component. Similar instrumentation has been used in other studies to measure fugitive dust impacts (e.g., CitationWatson et al., 2011). Particle mass was also collected using BGI PQ200 Federal Reference Method high-volume filter samplers. Tandem samplers with PM10 inlets were used at both Site 1 and Site 2, with one collecting mass on a Teflon filter and the other collecting mass on a quartz filter. This enabled subsequent analysis for organic material (quartz) and silicates (Teflon) along with other elements. The filter sampling was done for 12 hr starting a 7:30 a.m. local time to characterize airborne particulate composition during the daytime when fugitive dust impacts were most likely to occur.
Sites 2 and 3 were located 227 m and 283 m at a compass direction of ∼17˚ from the center of a circle roughly encompassing the active fly ash disposal area. Site 2 was 23 m from the industrial unpaved (clay) road—called the “berm” road—that was the source of most of the road dust impacts recorded during the study. The base of the berm road was about 3.7 m above the elevation of Site 2, but the BAMs sampling inlets were 2.4 m above ground, placing the particulate measurements at just over 1 m from the vertical center height of road dust plumes. A seldom-used gravel “access” road was between the berm road and Site 2. The access road was 3.5 m from Site 2 and at the same elevation.
The digital camera used was a Mobotix M24M surveillance system. The primary benefit of this video system is its relatively high resolution (3 megapixels per image) and motion detection capability. The camera was configured to operate Monday through Friday, from 7:30 a.m. through 5:00 p.m. local time, and coincided with the schedule of the ash handling crews. The camera viewing angle covered the northwest part of the ash pile, while the image foreground included the access road, the berm road, and a perpendicular road that connects the berm and main roads. Six video motion windows (VMW) were defined to closely monitor activities occurring inside the camera field of view. When one or more VMWs detected movement the camera automatically stored images at a predetermined minimum time interval (to conserve camera memory we used 10 sec and, later, 5 sec). The images provided a vehicle census during the study, a record of vehicular activities (i.e., grading work), and a means of quantifying vehicle speed. The types of vehicles involved in fly ash hauling and dumping were heavy-duty haul trucks, water trucks, and excavators. Vehicles involved in road and drainage constructions included front loaders, graders, bulldozers, a watering truck, a school bus (for personnel transport), pickup trucks, and small utility vehicles of various types. The surveillance system helped determine the likely cause of light scattering spikes observed at Site 2.
Fly ash plume modeling
The U.S. EPA AERMOD atmospheric dispersion model (CitationU.S. EPA, 2004) is the tool that is recommended by the U.S. EPA for computing the dispersion of atmospheric pollutants within a few tens of kilometers from a regulated source. AERMOD is capable of simulating pollutants emitted from a nonbuoyant area source such as a fly ash disposal site. AERMOD is a Gaussian plume model with the highest simulated pollutant concentrations at plume centerline and decreasing concentrations—following a Gaussian distribution—toward a plume's lateral (cross-wind) boundaries. AERMOD is a spatially uniform steady-state model in that it only considers one set of meteorological conditions for representing the entire period of pollutant transport from source to downwind receptor. The model calculates a pollutant concentration C based on a user-supplied emission rate Q. If AERMOD is run with Q = 1 then each simulated concentration is mathematically equivalent to the rate-normalized concentration C/Q because in the model C is directly proportional to Q.
The Gaussian plume assumption represents an analytical challenge because it is a statistical approach to dispersion modeling that is most relevant when simulating a large number of plumes under similar conditions. In truth, no plume is “infinitely” wide as the Gaussian assumption implies. When used in the current analysis, an extremely wide plume can yield nonzero estimates of C/Q at downwind monitoring sites. These results would produce very high emission rates but with a corresponding very low probability of being real. To avoid this problem, all AERMOD model results were based on a 4σ y finite-width plume, rather than allowing AERMOD to assume a Gaussian plume of infinite width. Thus, the value of C/Q was set to zero for periods when a receptor (i.e., air monitoring station) was more than 2σ y from the plume centerline.
AERMOD is normally run using hourly meteorological data. This approach neglects sub-hourly meteorological variability that could be important in determining 1-hr average concentrations. Wind direction variations are especially problematic in summer when wind speeds are often light and direction variability is large. This problem was minimized by processing six individual 10-min meteorological averaging periods each hour and using them to model 10-min average concentrations that were subsequently combined to yield hourly averages.
The method used here was to provide AERMOD all meteorological parameters that were measured at the study site and allow the model to select the parameters using its built-in data preferences. Thus, AERMOD was given wind speed, direction (θ), air temperature, relative humidity, the standard deviation of the vertical wind component (σ w ) and the standard deviation of the horizontal wind direction (σθ) at both tower levels along with solar radiation, net radiation, precipitation amount, and surface roughness (z 0 ). Surface roughness, computed by wind direction sector for the study site using data for 10-min periods under neutral atmospheric stability, averaged 0.03 m for the important southerly sectors. This low value indicates a relatively smooth surface. Wind speed, wind direction, σ w , and σθ are the parameters most likely to be used by AERMOD when calculating C/Q. AERMOD also reads and uses other derived parameters (e.g., surface heat flux, Monin Obukhov length). These were computed following procedures outlined in AERMOD documentation (CitationU.S. EPA, 2004) but were unlikely to be used. The height of the mixing layer (z m ) was not measured on site. A constant 800 m was used as the convective z m for all events and a constant 200 m was input for the mechanically mixed z m . Tests conducted on sensitivity to zm in AERMOD found that the value did not affect C/Q over the short distances between the source (fly ash disposal site) and the monitoring sites. Thus, the parameters that controlled simulated C/Q in this study were measured U, θ, σθ, and σ w .
AERMOD was applied in two ways to simulate dispersion from an area source with a diameter of 7 m and a release height of zero. This approach assumes that airflow streamlines follow the contour of the fly ash dry stack. An elevated release could only occur with strong winds that force streamline separation in the lee of the dry stack. With nearly all 10-min wind speeds of 1 to 3 m sec−1, the likelihood of streamline separation was very low because the pressure perturbation that can create streamline separation in the lee of air flowing over a hill is a positive function of wind speed (CitationJackson and Hunt, 1975). An “elevated” plume would produce a negative association between concentration (at the monitoring sites) and speed because streamline separation increases with speed. Faster transport allows less time for particles to disperse from aloft to the surface, thereby reducing the downwind concentration of particles at ground level beneath an elevated plume (the U−1 dilution effect would further compound this negative association; see, for example, plume dispersion formulations for a Gaussian plume in CitationHanna et al., 1982). However, a comparison of plume concentration versus wind speed for hours with wind directions most closely aligned (±15˚) with the monitoring sites—implying that plumes were sampled near centerline—indicated that wind speed variance accounted for about one-third of the total variance in concentrations and the association was positive. This is the kind of association that would occur for a ground-level plume in which reduced transport time from higher winds means less dispersion and higher concentrations near the plume centerline (in this case the effect of reduced transport and diffusion time of the surface plume clearly overcomes and is a stronger effect than the opposing U−1 effect).
In one approach, AERMOD simulations were based on actual sub-hourly meteorological data and results combined to produce one hour average C/Q for four alternate source locations on the dry stack. These locations were at the downwind, upwind, westernmost and easternmost edges of the circle defining the active ash storage area (see ). This approach provided estimates of the uncertainty due to not knowing the exact location of ash deposits for each event hour. A second approach applied a Monte Carlo sampling procedure to estimate alternate sub-hourly meteorology based on measured variations in input parameters. This approach provided estimates of the uncertainty due to meteorological variability between the monitoring and ash disposal sites. In both approaches, the area emission rate for the ith particle size fraction was determined from
with units of emitted particle mass per unit area per unit time. The value Cxs (i) represents the “excess” concentration associated with the fly ash fugitive dust plume and derived from observations as described later. During each hour the mass of fly ash processed at the source was known (Mash ) in units of mass of ash per unit area per unit time. The equivalent particulate emission factor Eash (i)—with units of emitted particle mass per mass of processed ash—was computed as
Separating road dust and fly ash from background particulate levels
Individually quantifying road and fly ash disposal contributions to measured hourly CPM2.5 and CPMc (= CPM10 – CPM2.5 ) was difficult because there is no direct means of knowing the degree to which airborne particles were derived from soil or fly ash (soil and ash chemical signatures are too similar). We used an indirect phenomenological approach based on camera information, measured bscat , and derived statistical relationships between various measured parameters. This method was not perfect but it captured the majority of local sources and enabled us to isolate those events that were most likely associated only with fugitive fly ash emissions. If anything, the approach may have enabled some contributions from unknown sources to impact the fly ash calculations, thereby slightly overestimating fly ash fugitive emissions.
Particle concentrations for the two mass fractions at Sites 2 and 3 were impacted by background sources (i.e., upwind of the plant site), fly ash disposal activity and local sources (those between the fly ash site and the monitoring sites). Measured background levels of PM10 and PM2.5 when airflow was from the southerly directions were provided by Site 1 data. Subtracting background values from Site 2 and 3 values provided concentrations due to the combined effects from fly ash disposal and local sources. Fly ash contributions at Sites 2 and 3 were computed using measured bscat at Site 2 to remove local source impacts. The procedure was developed after analyzing 447 hr of data when wind directions were from the south-southeast through south-southwest sectors. Relationships were examined between bscat , CPM2.5 , CPMc , and associated meteorological parameters.
From scaling arguments it can be shown that, unless the number of particles in the PMc size fraction is a lot more abundant than those <2.5 µm, the measurement of bscat will be more sensitive to CPM2.5 than CPMc . Note that CPM2.5 is significantly correlated (>99% level of confidence) with CPMc but the associated variance (r2 ) is small, ∼10%, implying that only a small portion of the PM2.5 and PMc at Site 2 comes from the same source(s). Multivariate analysis for conditions when airflow was from the south-southeast through west southwest sectors also yielded the following:
• | bscat is significantly correlated with Site 2 CPM2.5 and CPMc but the association with CPMc is weak (i.e., they share little variance in common). The correlation of bscat with CPMc is due to the association between CPM2.5 and CPMc . | ||||
• | Seventy-six percent of the variance in CPM2.5 at Site 2 is associated with variance in bscat and a small additional amount of variance is associated with relative humidity at 2.3 m (f2 ) and the standard deviation of bscat (σ bscat ). Predictor variable confidence exceeds 99% for all predictors. | ||||
• | The multivariate regression between CPM2.5 and its predictors bscat , σ bscat and f2 (r2 = 0.81) is given by |
In Equationeq 7, cbscat = 0.201 Mm µg m−3, cf2 = 0.054 µg m−3 percent−1, c σ = 0.052 Mm µg m−3, and cint = 6.56 µg m−3. This equation provides a means for directly estimating CPM2.5 from other measured parameters. The association between CPM2.5 and σ bscat was not nearly as strong as the association between CPMc and σ bscat .
The larger particles in the coarse size fraction are not well represented by visual wavelength bscat primarily because of the lower light scattering efficiency and smaller number concentration of particles larger than a micrometer in diameter (CitationFriedlander, 2000). However, it is clear from examining bscat and CPMc time-series plots that fugitive sources produce coarse particles. One way to model CPMc is to find a surrogate for the various physical processes (vehicle passages, ash dumps, etc.) that generate fugitive emissions. We found the best surrogate to be the standard deviation of 1-min bscat . Peaks in bscat are an indication of physical activity that generates fugitive dust. As the activity increases and produces more PMc, 1-min bscat exhibits more peaks and these translate into larger variance in bscat .
A multivariate analysis of CPMc and various parameters yielded the following:
• | Hourly CPMc is highly correlated (r2 = 0.67) with σ bscat . | ||||
• | CPMc is significantly correlated with wind speed at 2.3 m (U2 ). | ||||
• | CPM2.5 provides some additional correlation with CPMc beyond what is captured by σ bscat and U2 . | ||||
• | Jointly, σ bscat , U2 and CPM2.5 are associated with 71% of the variance in CPMc . Although somewhat lower than the model of CPM2.5 , this is still a very high correlation. The resultant model is expressed as |
In Equationeq 8, c σ = 3.87 Mm µg m−3, cU2 = 7.85 µg s m-4, cPM25 = 1.57, and cint = −29.4 µg m−3. All predictors in Equationeq 8 are significant at greater than 99% confidence. Comparing Equationeqs 7 and Equation8 in terms of regression parameters reveals that CPMc is >10 times more sensitive to σ bscat than CPM2.5 is to bscat .
Given these relationships, it is possible to estimate CPM2.5 and CPMc from parameters that are continuously measured onsite. The procedure to determine fugitive dust plume hourly “excess” concentrations for the ith particle size fraction at Site 2 was to first compute an adjusted concentration that removed the influence of local sources from the measured value, Cobs (i):
where “obs” and “adj” superscripts refer to the hourly values measured, respectively, across all minutes and those minutes for which no local disturbances were identified. Minutes when bscat was impacted by a local source were those when bscat increased >20% above the baseline value. Note that cbscat = 0 for the PMc size fraction and cPM2.5 = 0 for the PM2.5 size fraction. In addition, CPM2.5 must be computed before CPMc because the former is needed to compute the latter.
Cxs (i) is computed from Cadj (i) as
with Cbck (i) representing the background concentration of particle size fraction i. Site 1 PM2.5 concentrations were strongly correlated with those at Site 2, and PM2.5 background at Sites 2 and 3 was determined from the linear regression between Sites 1 and 2. However, there was little association between PMc levels at Sites 1 and 2. For the >90% of hours when sufficient data were available, daily average background PMc concentrations at Sites 2 and 3 were computed using daytime PMc levels measured at Sites 2 and 3 during hours when no fly ash handling occurred and when the contribution of local fugitive sources to PMc were insignificant (i.e., <1% of the hourly particulate loading based on bscat data). The PMc level observed at Site 1 was used as a background estimate of last resort for only a few (<10%) of all hours analyzed.
Site 3 measurements were immediately downwind of Site 2 when winds were favorable for fugitive fly ash impacts. Adjusted Site 3 concentrations were computed assuming equal proportionality between Site 3 and Site 2 such that the ratio Cadj /Cobs was equal at the two sites.
Results
Fly ash emission factors
Hours were analyzed for fugitive fly ash emissions as long as they met a list of criteria that included wind directions between south-southeast and south-southwest (to ensure optimal alignment between the source area and downwind monitors), the availability of valid data, no precipitation, the absence of other dust source interferences whose influence could not be removed (including screening of events based on PM10 chemical signatures to remove periods clearly affected by biomass burning), and a reliable indication that fly ash disposal was actively occurring. A description of how fly ash disposal activity was determined is provided in the Supplemental Information.
After removing background and local source interferences, measured particulate concentrations were, in ∼10% of all cases, not significantly different from zero for PMc. The occurrence was more frequent for PM2.5 because of the relatively low fraction of particulate emissions in the smaller size fraction and the relatively large uncertainty in the measurement. Measurement sensitivity was determined prior to the start of ambient measurements following manufacturer guidelines that all instruments be initially operated for 3 days with special inlet filters that remove particles smaller than 10 µm in size. A subsequent comparison of the “zero” concentration data provides information on measurement sensitivity. Our zero-air comparisons yielded a mean sensitivity for the BAMs data of ±3.6 µg m−3 for both PM10 and PM2.5 measurements. illustrates the frequency distributions of Cobs
at each measurement site during the periods when ash was typically processed. Fine particle concentrations rarely exceeded 30 µg m−3 but PM10 values exceeded 60 µg m−3 a significant fraction of the time. Mean measured values listed in each plot, do not vary much by site for PM2.5 but exhibit a lot of differentiation for PM10. This shows that fugitive dust emissions are primarily in the coarse particle size fraction. illustrates the distributions of PM2.5 and PMc
Cxs
for the cases analyzed to determine EFs. About 60% of the PM2.5
Cxs
and 47% of the PMc
Cxs
was below 3.6 µg m−3, making the signal-to-noise ratio especially low for PM2.5 emissions.
Figure 3. Frequency distributions of measured PM2.5 and PM10 concentrations at the three monitoring sites for Monday–Friday, 07:00 a.m. through 3:00 p.m. local standard time, when fly ash was typically moved to the storage area. Mean values (µg m−3) are denoted . All meteorological conditions are represented.
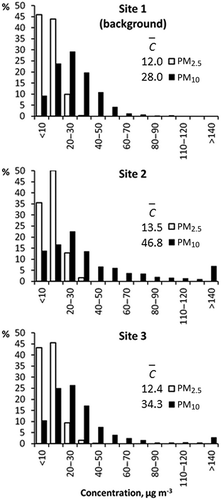
Figure 4. Distributions of “excess” fugitive fly ash plume concentrations (Cxs ) determined for fly ash plume events captured by the particulate monitors.
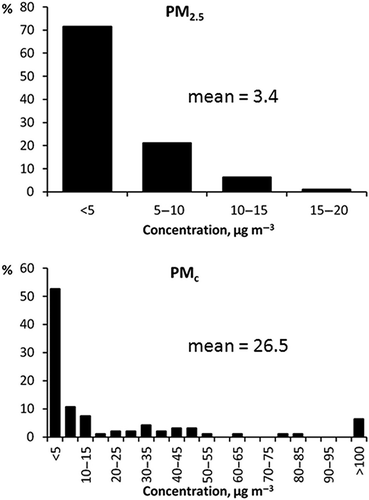
Although Cxs values were not always >3.6 µg m−3, they were usually >1 µg m−3 (BAM precision level). However, when Cxs was <1 µg m−3 it was usually <<1 µg m−3 (and even <0). For cases when 4 µg m−3 < Cxs < 0.5 µg m−3, Cxs was arbitrarily set to 3.6 µg m−3 to calculate an EF upper limit during extremely low and highly uncertain plume levels (cases were not analyzed if Cxs < -4 µg m−3). These were typically events when ash handling rates were very low at the disposal site. summarizes the EFs computed from field data and compares them with values derived using aggregate AP-42 EF formulations for ash handling processes (i.e., Equationeqs. 2 and Equation3). These results include only those hourly events when the derived number of ash truck loads was at least one and when EFs were computable using both the field study and AP-42 methods.
Table 1. Fly ash dry disposal fugitive emission factors derived from field data and using AP-42 formulations for dropping and grading operations
Emission factors based on field study data exhibited a much larger range than factors derived from AP-42 formulations. This is probably because natural variability in atmospheric conditions coupled with large variations in ash handling conditions conspired to produce large variations in downwind concentrations used to compute fugitive fly ash EFs. Factors derived using AP-42 formulations were based on a small range in input parameters and the EFs themselves do not rely on downwind measurements for verification. In addition to differences in range/variability, field study EFs were smaller in magnitude and more strongly skewed toward low values than AP-42 values (and this was despite the fact that EFs based on field study data included values representing an upper limit whenever extremely low concentrations were measured). Coarse mass EFs averaged 63% less for field study data compared to AP-42 values and PM2.5 EFs averaged 38% less. Median values showed an even greater disparity. This is especially noteworthy because of the conservative approach used to estimate EFs when Cxs was very low.
Fly ash emission factor uncertainties
Simulated emission rate uncertainty (which translates directly into EF uncertainty) was examined by computing the variability in AERMOD-derived Qa due to uncertainty in the exact location of fugitive emissions on the fly ash dry stack and uncertainty in the meteorological data input to AERMOD. The Qa uncertainty due to source location (“location” uncertainty) derives from the fact that the exact distance and direction from where ash was deposited relative to the downwind monitors were not known during any given hour. Source–receptor distances varied from 176 to 278 m for Site 2 and from 232 m to 334 for Site 3. Likewise, source–receptor directions varied ±12˚ relative to direct alignment between the assumed fly ash emission centroid at Sites 2 and 3. Meteorological uncertainty may exist (although we have no evidence that suggests it is important), because meteorological measurements critical to AERMOD dispersion calculations were co-located with the downwind monitors and not with the source. In a convective boundary layer, turbulence and its impact on winds can vary considerably over the few hundred meters separating the source and measurement locations, especially when using 10-min averaging periods for dispersion calculations.
Location uncertainty was determined by simulating downwind impacts for four separate locations representing extremes in source–receptor distance and direction (see locations in ). The resulting emission rates are denoted Qloc. Meteorological uncertainty was examined using a Monte Carlo resampling of sub-hourly meteorological parameters for each fugitive fly ash event. Rates generated from this exercise are denoted Qmet . The source position was set to the ash disposal centroid location (source–receptor distances of 227 and 283 m for Sites 2 and 3) for Qmet simulations. A thousand independent replications of 10-min meteorological parameters provided alternate realities of conditions driving transport and diffusion. Meteorological variances were taken directly from the observed variability of 10-min parameters during each event hour. Each replicated set of meteorology was modeled by AERMOD. The means and uncertainties associated with Qmet represent an independent calculation of the sensitivity of simulated emission rates to meteorological variability between the measurement location (tower) and the fly ash disposal area. The Monte Carlo replication methodology is described further in the Supplemental Information. The most variable input parameter was wind direction which is typical of weak airflow summertime conditions in Alabama. Wind direction variability can result in either direct plume hits on a receptor, a “glancing blow” by a plume, or a total miss. Impacts from plumes that passed a receptor at a distance >2σ y were assumed to make no contribution to measured particle concentrations. However, plumes that impacted a receptor “on edge” (near the 2σ y limit) can result in high emission rate estimates and contributed to the large upper tail of the Qmet (and Eash ) frequency distributions.
Simulated 90% confidence intervals for Qloc
and Qmet
are summarized in . Meteorological uncertainty effects were larger than location uncertainty effects. Source location uncertainty is more likely to produce emission rate estimates that are less than the average rate based on all four potential source locations. Meteorological uncertainty is more likely to produce overestimates compared to the average of Qa
based on all meteorological realizations. The upper distribution tail is so extreme that mean Qmet
values in are based only on the results that fall within the 90% confidence interval to avoid an otherwise absurdly large summary statistic. The mean for coarse mass Qmet
was 34% higher than the mean for Qloc
(denoted ), but the fine mass mean Qmet
was nearly double
.
Table 2. Average simulated fugitive fly ash emission rates (g ha−1 sec−1) for all event hours on days when the site material processing log indicated nonzero fly ash disposal activitya
These results should not be interpreted as indicating that the Qmet results are somehow more realistic than Qloc , because the former only represent hypothetical multiparameter variations in meteorology. Qmet results indicate the worst-case level of uncertainty that would exist if the observed meteorological data did not represent conditions over the ash disposal area. The dry stack top is 20–30 m above the elevation at Site 2 and 17–27 m above the top measurement level on the meteorological tower. There is a possibility that the elevation difference and source-receptor distance might conspire to introduce meteorological dissimilarities between the tower and the dry stack. Thus, the Monte Carlo test for meteorological uncertainty is an acknowledgment of its potential impact on emission rate estimates while recognizing that these test results likely overstate the influence of any differences on simulated dispersion results.
The PMc Eash values based on this study are so much lower than the AP-42 based values that the potential meteorological uncertainty does not alter the conclusion that the former are significantly lower than the latter. The difference between the mean study-derived and AP-42 PM2.5 Eash values is less than that for PMc Eash and the two sets of PM2.5 results may not be as different as implied, based on the potential for meteorological uncertainty. However, the median PM2.5 Eash values still exhibit a large difference that remains significant even if meteorological uncertainty was important.
Factors affecting differences between field-derived and AP-42 EFs
Differences between AP-42 and our estimates of Eash are due entirely to the AP-42 estimates of fugitive dust from graders driving over ash ( Equationeq 3). This is because the average contribution to total fugitive fly ash emissions of grading operations—using the AP-42 formulation for vehicles on unpaved industrial surfaces—is >99% of the total of estimated dumping ( Equationeq 1) and grading emissions. Uncertainty in applying AP-42 Equationeqs 1 and Equation3 was computed based on expected uncertainty in ash moisture content (M) of 10-20%, silt content (S) of fly ash (±10%), grader operating times (±20% error), and wind speed (based on two tower level options). The mean of the AP-42 PMc Eash values that included parameter uncertainty was nearly identical to that listed in , but the range expanded to 156–443 g Mg−1, the median increased to 242 g Mg−1, and the standard deviation increased to 62 g Mg−1. These results illustrate that uncertainty inherent in the AP-42 approach is capable of producing greater variability in Eash than is implied by using single values for input parameters, but the AP-42 method is unlikely to produce significantly lower values more in line with those computed using our field study approach.
The apparent overestimate of fugitive emissions from slow vehicles on unpaved industrial surfaces motivated us to investigate the relative merits of the two AP-42 EFs for unpaved surfaces (
Equationeqs 3 and Equation4) at an alternate location at the plant. The two unpaved roads near Site 2 offered that opportunity. Dust emission factors (Eroad
) for the two unpaved roads were determined based on data (particle concentration and nephelometer) from Site 2, meteorological measurements, line source dispersion calculations (conditions summarized in ), and various assumptions described in Supplemental Information. Also summarized there are the influences of these assumptions, the road characteristics and the behavior of the road dust plumes. Results of the Eroad
analysis based on observations are summarized in . Coarse mass Eroad
—denoted —was found to average 90 g per vehicle kilometer traveled (VKT−1) for both roads but with emissions from the seldom-traveled gravel/dirt access road averaging nearly 4 times greater than the more frequently used clay-surfaced berm road. Individual event values ranged from near zero to more than 600 g VKT−1. However, the distribution of
is skewed toward small values with a median emission factor of 44 g VKT−1. Values of
are much smaller, averaging 4 g VKT−1 with a median of around 2 g VKT−1.
Table 3. Average parameters used to compute dispersion of fugitive road dust at Site 2
Table 4. Data, road conditions, and mean fugitive road dust emission factorsa
Emission factors computed using AP-42 formulations (
Equationeqs 3 and Equation4 yielding Eir
and Epr
) are summarized in for the same events. Less traffic at industrial sites is expected to contribute to higher levels of surface silt. Also, industrial site traffic is expected to move more slowly, such that vehicle speed is unlikely to be important. Intermittent precipitation can be expected to condition public road surfaces in ways not expected for industrial roads (unless the latter are watered as is now standard practice at many sites, including the berm road). This requires using surface moisture content when estimating Epr
with Equationeq 4. Averages of road surface parameters and estimated EFs are listed in for the 42 road emission events analyzed. Field data indicated that the access road EFs were higher than those for the berm road, consistent with the public road formula (
Equationeq 4) but not the industrial road formula (
Equationeq 3). All methodologies agree that PM2.5 EFs are much lower than PMc EFs. Also, was less than Eir
and Epr
for both roads. Even though the berm and access roads were not composed of fly ash, the large differences between
and Eir
suggest an explanation for why field-derived values of Eash
were so much lower than their AP-42 counterparts using the industrial road formulation.
Conclusion
Five months of measurements at the TVA Colbert fossil plant captured a number of hours when meteorological conditions coincided with activities that produced fugitive particulate emissions. A methodology for removing local source effects on measured particle concentrations enabled an estimate of fly ash fugitive particulate emission rates and emission factors. A separate set of brief (∼1–3 min) periods was analyzed to independently estimate fugitive road dust emission factors. Results from both source types were compared with EFs derived using formulations in the EPA AP-42 emissions handbook.
The fly ash disposal process at Colbert requires that “dry” ash (although the ash is not totally free of water this process is distinctly different from the “wet” process in which ash is pumped in a water slurry to a wet ash disposal pond) be dumped from the bed of a haul truck and then immediately spread into a layer of uniform thickness before the next load arrives. Multiple ash loads are usually deposited during peak work hours. Downwind measurements of hourly particle concentrations appear to respond as expected to this activity. However, atmospheric variability drives plume dynamics in a way that makes it difficult to measure fugitive plumes on a consistent basis. Also, during the field study some events produced such low downwind concentrations that fugitive fly ash plumes could not be detected with high confidence above the background levels. This is due in part to atmospheric variability and in part to the measurement sensitivity of the monitoring equipment. Previous studies of this type relied on even coarser measurement methods (dustfall collectors and high-volume particle samples operated for extended periods of time very near the source). It is difficult to conduct close-proximity measurements in an operational setting such as the one at Colbert. To our knowledge this study represents the first attempt to conduct a fugitive dust measurement campaign at an operating fly ash disposal area. The study data integrate emissions over the multiple operations involved in fly ash disposal, rather than relying on parameterizations of individual processes (i.e., dumping and grading). In addition, this study measured both coarse and fine particles at 1 hr time resolution, thereby minimizing the uncertainties introduced when longer averaging times are involved and even more atmospheric variability comes into play. Data from this study represent a fresh examination of the fugitive emissions formulations that have been used for several decades without being reevaluated.
The picture that emerges is that AP-42 formulations produce EFs significantly higher than those derived from the current study. When applied to ash disposal the AP-42 formulations result in a narrow range of EFs, even when the uncertainty of factors like ash moisture content and the representativeness of the formulations for high silt content materials (such as fly ash) are considered. By contrast, the natural variability of the atmosphere produces EFs that cover a much wider range of values, with most clustered in the lower range of values but a few spread out to form a long upper tail to the distribution. The selection of a “best” metric is perhaps debatable under these circumstances, but given that EFs are typically applied to produce long-term (especially annual) estimates of total emission it seems that use of a mean or median value is appropriate. The mean is more conservative than the median due to the distribution skewness, but even so the EFs derived here remain quite low when compared to equivalent AP-42 EFs.
It is important to remember that EFs for fly ash disposal are strongly influenced by the AP-42 formulation ( Equationeq 3) for fugitive emissions from vehicles moving over unpaved surfaces. This formulation is distinctly different from the one recommended for use on unpaved public roads ( Equationeq 4) in which vehicle speed and surface moisture content are treated explicitly. Thus, the comparison of fugitive road EFs from both AP-42 and field data supports our belief that the AP-42 industrial unpaved road EF formulation is biased high for a surface composed mostly of silt-sized particles. Results from this study suggest that coarse particle EFs for unpaved surfaces are much less than those derived using either the industrial or public road formulation. Fine particle (PM2.5) EFs determined by the present study are also somewhat lower than those produced using either AP-42 formulation.
Reasons why EFs derived from AP-42 formulations are greater than those derived by this study are not obvious, but a number of reasons can be contemplated. The EF formulation for dropping operations is based on materials that were far drier than the moisture content of the fly ash. The EF for vehicles driving on unpaved surfaces at industrial sites was based on data for surfaces with silt content far below what is appropriate for fly ash, and most data were for vehicles traveling at speeds well above those involved in grading fly ash. Contemporary measurement technology is capable of providing hourly particle concentrations, whereas older measurements utilized high-volume filter measurements that required sampling periods longer than 1 hr and were incapable of detecting short-term emission rate variations. Long sampling periods necessarily include variable meteorology, and that implies the possibility of a highly variable relationship between emissions source and downwind measurement locations while measurements are made. It is not clear how this might affect derived emission factors, but it is not surprising that AP-42 formulations provide emission estimates that do not match closely with those measured using modern techniques.
It is interesting to compare the relationship between PM2.5 and PM10 for the different sources and emission factor derivations. The ratio of PM2.5 to PM10 in fugitive fly ash plumes was observed to be 9%, which compares very well with the ratio of 10% that is inherent in the AP-42 formulations. However, the fraction of PM10 that is PM2.5 in fugitive road dust was observed to be only 4%, which is considerably lower than the 10% ratio in the AP-42 results. The ratio of PM2.5/PM10 of 0.1 in the AP-42 EFs is consistent with the ratio reported by a fugitive dust study of western sources (CitationWRAP, 2005). That same report also mentioned that there was some evidence the ratio might be closer to 0.05 and that would be very similar to the 0.04 ratio reported here.
Finally, fly ash is the particulate matter collected by electrostatic precipitators (ESPs) similar in design to controls used on most large combustion sources. The small size of the particles (all being smaller than “silt” or 75 µm in diameter) is characteristic of ESPs. The AP-42 emissions handbook (CitationU.S. EPA, 1995, .-6 through .-8) differentiates the emitted size distributions of particles from coal-fired boilers based on boiler type and particulate control technology. Colbert has dry bottom pulverized coal boilers with ESP controls, and these are the primary determinants for fly ash size based on U.S. EPA guidance. Therefore, dry fly ash fugitive disposal EFs estimated from this study should be representative of conditions at other plants with dry bottom pulverized boilers and ESPs. Other boiler types (especially cyclone boilers) and plants with baghouse controls likely produce fly ash with somewhat different characteristics (and with potentially different fly ash EFs), but plants of this type are less common in the United States.
uawm_a_795201_sm7813.pdf
Download PDF (393.2 KB)Acknowledgment
The authors are grateful for the support provided by Colbert plant personnel in conducting the field measurements for this study. Funding for this work was provided by the Electric Power Research Institute and the Tennessee Valley Authority.
References
- Cimorelli , A.J. , Perry , S.G. , Venkatram , A. , Weil , J.C. , Paine , R.J. , Wilson , R.B. , Lee , R.F. , Peters , W.D. , Brode , R.W. and Paumier , J.O. 2004 . AERMOD: Description of Model Formulation. EPA-454/R-03-004 , Research Triangle Park , NC : U.S. Environmental Protection Agency, Office of Air Quality Planning and Standards .
- Code of Federal Regulations, 2011. Title 40, Sections 70.2. Washington, DC: Office of the Federal Register, Government Printing Office
- Code of Federal Regulations, 2008. Title 51, Section 166. Washington, DC: Office of the Federal Register, Government Printing Office
- Cowherd , C. , Axetell , K. , Guenther , C.M. and Jutze , G.A. 1974 . “ Development of Emission Factors for Fugitive Dust Sources ” . In Midwest Research Institute report to the EPA. EPA-450/3-74-037
- Dyck , R.J. and Stukel , J.J. 1976 . Fugitive dust emissions from trucks on unpaved roads . Environ. Sci. Technol. , 10 : 1046 – 48 . doi: 10.1021/es60121a015
- U.S. Environmental Protection Agency. 1995 [with subsequent updates]. Compilation of Air Pollutant Emission Factors—Volume I: Stationary Point and Area Sources, AP-42, Fifth Edition. Research Triangle Park, NC: Office of Air Quality Planning and Standards. (accessed 12 March 2013) http://www.epa.gov/ttn/chief/ap42 (http://www.epa.gov/ttn/chief/ap42)
- U.S. Environmental Protection Agency . 2004 . User's Guide for the AMS/EPA Regulatory Model—AERMOD, EPA-454/B-03-001 , Research Triangle Park , NC : Office of Air Quality Planning and Standards .
- Friedlander , S.K. 2000 . Smoke, Dust, and Haze , 130 – 139 . New York , NY : Oxford University Press .
- Gillies , J.A. , Etyemezian , V. , Kuhns , H. , Nikolic , D. and Gillette , D.A. 2005 . Effect of vehicle characteristics on unpaved road dust emissions . Atmos. Environ. , 39 : 2341 – 47 . doi: 10.1016/j.atmosenv.2004.05.064
- Hanna , S.R. , Briggs , G.A. and Hosker , R.P. 1982 . Handbook of Atmospheric Diffusion , 25 – 34 . Washington , DC : U.S. Department of Energy. DOE/TIC-11223 .
- Hoover , J.M. 1973 . Surface Improvement and Dust Palliation of Unpaved Secondary Roads and Streets , Report on ERI Project 856-S. Ames: Engineering Research Institute, Iowa State University .
- Jackson , P.S and Hunt , J.C.R. 1975 . Turbulent wind flow over a hill . Q. J. R. Meteorol. Soc. , 101 : 929 – 55 . doi: 10.1002/qj.49710143015
- Watson , J.G. , Chow , J.C. , Chen , L. , Wang , X. , Merrifield , T.M. , Fine , P.M. and Barker , K. 2011 . Measurement system evaluation for upwind/downwind sampling of fugitive dust emissions . Aerosol Air Qual. Res. , 11 doi: 10.4209/aaqr.2011.03.0028
- Western Regional Air Partnership . 2005 . Analysis of the Fine Fraction of Particulate Matter in Fugitive Dust—Final Report . Midwest Research Institute report for the Western Governors’ Association Western Regional Air Partnership. ,
- Western Regional Air Partnership . 2006 . WRAP Fugitive Dust Handbook , chap. 6. Western Governors’ Association, WGA contract 30204-111 .
Supplemental Materials
Supplemental materials are available for this paper. Go to the publisher's online edition of the Journal of the Air & Waste Management Association.