Abstract
The U.S. Environmental Protection Agency (EPA) short-distance dispersion model, AERMOD, has been shown to overpredict by a factor of as much as 10 when compared with observed concentrations from continuous releases at the Oak Ridge, TN (OR), and Idaho Falls, ID (IF), field experiments during stable periods when wind speeds often dropped below 1 m/sec. Some of this overprediction tendency can be reduced by revising AERMOD's meteorological preprocessor's parameterizations of the friction velocity, u * , during low-wind stable conditions, thus increasing the calculated σ v and σ w and hence the lateral and vertical dispersion rates. Observations show that as the mean wind speed approaches zero at night, there is always significant σ v and σ w over time periods of 15 to 60 min, while standard Monin–Obukhov Similarity Theory (MOST) predicts that σ v and σ w will approach zero. This paper focuses on the u * estimation methods and the minimum turbulence (σ v and σ w ) assumptions in AERMOD (beta option 4) and two widely used U.S. operational dispersion models, AERMOD (v12345) and SCICHEM. The U.S. EPA has provided results of its tests with the OR and IF data, with its base AERMOD version and its December 2012 modified versions, which assume adjustments to the low-wind u * and increases in the minimum σ v parameterization. SCICHEM has relatively small mean bias for both data sets. The revised AERMOD shows much less mean bias, agreeing more with SCICHEM.
Implications:
Suggestions are made for improvements to dispersion models such as AERMOD to correct overpredictions during light-wind stable conditions. Methods for estimating u*, L, and the minimum turbulence parameters (σv and σw) are reviewed and compared. SCICHEM and the current operational version and an optional beta version (December 2012) of AERMOD are evaluated with tracer data from low-wind stable field experiments in Idaho Falls and Oak Ridge. It is seen that the operational version of AERMOD overpredicts by a factor of 2 to 10, while the optional beta version of AERMOD and SCICHEM have much less bias.
Introduction and Background
Since the 1970s, dispersion modelers have recognized that when plumes are released continuously near the ground in low-wind (less than 1 or 2 m/sec) stable conditions, the resulting hourly-averaged concentration distributions are spread laterally over a much broader range than suggested by the standard (at the time) Pasquill–Gifford (PG) dispersion (as indicated by σy) model. The empirical PG dispersion curves are mostly based on field experiments where averaging times ranged from about 3 to 10 min. The PG curves are intended to be used in applications of operational models to scenarios where only routine weather observations are available (e.g., wind speed and direction, temperature, and cloudiness). The source scenarios of most interest are routine releases of pollutants from continuous point sources such as industrial stacks. Although the source emission rate may vary somewhat from hour to hour or from day to day, hourly averages are justified because emissions are usually relatively steady over a 1-hr period, and the U.S. National Ambient Air Quality Standards (NAAQS) are defined for averaging times that are an integer multiple of hours. Obviously there can be subhourly variations in emissions and meteorology, but these are ignored in U.S. Environmental Protection Agency (EPA) analyses of pollutants for which an NAAQS applies. Papers by CitationVan der Hoven (1976) and by CitationMitchell and Timbre (1979) suggest empirical corrections to the PG σy curves for low-wind stable conditions. They define multiplication factors for the σy values, where the multiplication factors steadily increase (greater than unity and as large as six) as wind speed decreases and as stability class becomes more stable.
The U.S. EPA straight-line Gaussian plume models, such as the Industrial Source Complex (ISC) model (CitationEPA, 1995), did not formally adopt the corrections for low-wind stable conditions, as described above. However, a few years ago, when AERMOD (CitationEPA, 2004; CitationCimorelli et al., 2005) replaced ISC, an empirical interpolation method was incorporated to account for the increased spread during low winds. The empirical interpolation used a “coherent” or standard deterministic plume solution as wind speeds became very large and a “random” or “pancake” solution (concentrations distributed uniformly around the circle) as wind speed approached zero (CitationEPA, 2004). The “coherent plume” solution, Ccoherent , is based on the primary AERMOD dispersion formulation and does not account for meandering during low-wind stable conditions. The “random plume” is the zero-wind concentration limit, Crandom , where the plume material is assumed to be distributed uniformly about the 360-degree circle surrounding the source. The interpolation procedure assumes that the concentration, C, equals WCrandom + (1 – W)Ccoherent , where the weighting factor, W, is assumed equal to σ r 2/σ h 2. σ r 2 is a measure of the random component of the wind energy and σ h 2 is a measure of the total wind energy. σ h 2 is assumed equal to 2σ v 2 + u2 , where σ v is assumed equal to σ u and u is the mean wind speed. The random component σ r 2 is assumed equal to 2σ v 2+ u2 (1 – exp(–x/uTr )), where Tr is a time scale at which mean wind information at the source is no longer correlated with the location of plume material at distance x. As Tr decreases, there is more weighting of the random/meandering component and less weighting of the coherent component. Based on CitationBrett and Tuller (1991), the CitationEPA (2004) assumes that Tr = 24 hr. In reality, Tr would depend on the surroundings well upwind, weather, and other conditions, but a single value is assumed until more detailed formulations are available. The random-coherent interpolation is thus a combination of suggestions found in the literature and empirical assumptions. The EPA changed Tr to 12 hr in its recent beta AERMOD options 2 and 4, which also increased the minimum σv from 0.2 to 0.3 m/sec. This is justified by R. Brode, U.S. EPA (private communication, July 31, 2013), who points out that of the CitationBrett and Tuller (1991) paper shows that most of the autocorrelation is lost after about 12 hr. The change from 24 to 12 hr will increase the influence of the random (meandering) component slightly.
Figure 1. Scatter plot for Oak Ridge observed and predicted hourly-averaged arc-maximum concentrations for (a) AERMOD (Base) operational model version 12345 and (b) SCICHEM and AERMOD (Beta 4), which is option 4 with adjusted u* and σ v = 0.3 m/sec.
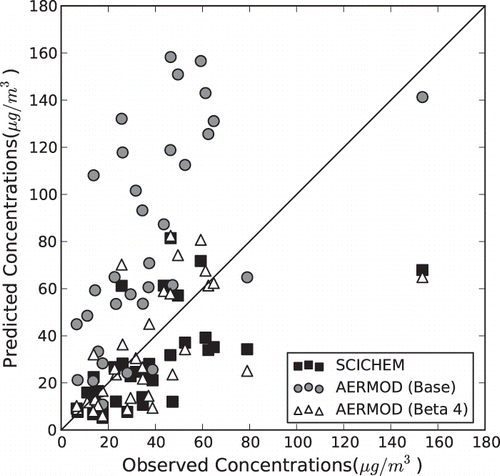
The U.S. CitationEPA (2004) coherent-random interpolation method was not adequately evaluated with the sets of field data used by the EPA to evaluate AERMOD (CitationPerry et al., 2005), since very few of those data involved low-wind stable conditions. However, there are nearly always several low-wind stable hours in the 5 years of meteorological observations used in routine AERMOD modeling for EPA regulatory purposes, and often relatively high concentrations are predicted by the model for those hours. One goal of the current paper is to test this method with appropriate stable low-wind field data.
While the AERMOD development was underway by the U.S. EPA, several research groups were making use of field observations of winds and turbulence and of dispersion from tracer studies during low-wind stable conditions to suggest some practical alternatives. Two field experiments carried out in the 1970s by the National Oceanic and Atmospheric Administration/ Environmental Research Laboratory/Air Resources Laboratory (NOAA/ERL/ARL) group in Idaho Falls, ID, have been very useful: the Idaho Falls (IF) experiment (CitationSagendorf and Dickson, 1974) and the Oak Ridge, TN (OR), experiment (CitationWilson et al., 1976). CitationVan der Hoven (1976) used these data in the development and testing of his corrections to the stable dispersion curves. Both field experiments involved continuous point sources of tracer gas near the ground (1.5 m at IF and 1.0 m at OR), stable conditions with low wind speeds (all less than 2 m/sec), and sampling arcs at distances of 100, 200, and 300 m. The IF field experiment took place over relatively flat desert terrain with scattered small vegetation and bushes, while the OR field experiment took place at a hilly site with terrain variations of about 30 m and with scattered trees and open cleared areas. The OR release and sampler arcs were mostly in the cleared areas, although there were scattered bushes. The wind speeds at OR were smaller than those at IF by a factor of 3 or 4, on average. CitationHanna (1990) published an analysis of the IF and OR data showing (1) that the observed standard angular deviation of the concentration distribution averaged over one hour, σθc , is much larger for smaller wind speeds, (2) that the observed standard deviation of the wind direction fluctuations, σθ, could be parameterized by tan−1((minimum σ v )/u), with σ v equal to 0.5 m/sec, and (3) that the parameterized σθ provided unbiased estimates of the observed σθc .
As an example of a recent related study, CitationTrini-Castelli and Falabino (2013) analyzed several field data sets with turbulence observations during low-wind conditions, for both stable and unstable conditions. They fitted the parameters of a simple empirical formula for σ u, σ v , and σ w based on MOST scaling variables, u*, L, and z. Histograms of relative frequencies of σ u, σ v , and σ w observed at four field sites showed a peak occurrence of σ u and σ v at values of 0.2 to 0.4 m/sec, with very few values less than 0.2 m/sec. The “proportionality factor” (leading constant) in their empirical formula had to be increased to fit the observed σ u values at wind speeds less than about 1 m/sec.
It is important to note that for standard U.S. EPA regulatory applications, it is required that a model such as AERMOD be run for at least 1 yr of hourly input data (meteorology and emissions). There is an incentive to speed up the model runs by using simplified formulas wherever possible. Thus minimum σ v and σ w approximations have been used in several U.S. operational dispersion models, such as AERMOD (CitationEPA 2004) and SCIPUFF (CitationSykes et al., 1986, Citation2011). SCICHEM is the version of SCIPUFF that focuses on plume chemistry (CitationKaramchandani et al., 2000; CitationChowdhury et al., 2012) and is being proposed for consideration by the EPA. SCICHEM (i.e., SCIPUFF) assumes larger minimum turbulence than assumed by AERMOD for both the lateral and vertical components (minimum σ v = 0.2 m/sec for AERMOD and 0.5 m/sec for SCICHEM; and minimum σ w = 0.02 m/sec for AERMOD and 0.1 m/sec for SCICHEM). These minimum σ assumptions are based on different interpretations of observed σ v data sets, including stable periods with light winds. For example, CitationHanna et al. (1985) plotted σ v for several field experiments, and concluded that σ v = 0.5 m/sec went through the middle of the data. However, when developing AERMOD, the EPA looked at the same data and preferred the more conservative value (σ v = 0.2 m/sec) that defined the lower envelope of the cloud of data points.
The details of the basis for the minimum values of σv and σw used in SCICHEM/SCIPUFF are provided in a series of journal articles on various aspects of SCIPUFF (CitationSykes et al., 1986, Citation1993, Citation1999; CitationSykes and Gabruk, 1997; CitationSykes and Henn, 1995) and in the detailed SCIPUFF Technical Document (CitationSykes et al., 2011). In short, the σv value was chosen for SCIPUFF to match the minimum r.m.s. lateral velocity fluctuation values from CitationHanna (1983) and CitationEtling (1990) under very light wind conditions, and the σw value was chosen to match the results of equilibrium vertical diffusivity provided by CitationRosenberg and Dewan (1974) and Lilly et al. (1973). It should be stressed that SCIPUFF and SCICHEM have been evaluated with various field data sets in several journal articles (e.g., CitationSykes et al. 1993; Warner et al., 2007).
In the past 5 years, in an attempt to improve AERMOD's predictions during low-wind stable conditions, there has been increased study of the meteorological preprocessor (AERMET) used in AERMOD. This preprocessor provides outputs of u* and L, which are subsequently used to estimate wind profiles, turbulence, and mixing depths. CitationLuhar et al. (2009) compared AERMOD/AERMET and other models’ u* estimates with boundary-layer observations during field experiments and found that at very low wind speeds, u* was being underestimated by AERMOD/AERMET by as much as a factor of about 2. Thus, the turbulent speeds, σ v and σ w , and mixing depths (which are proportional to u* during neutral and stable conditions) were also underestimated and hence the dispersion model calculations of concentration would be overestimated. CitationPaine et al. (2010) and CitationQian and Venkatram (2011) have addressed ways to modify the AERMOD meteorological preprocessor to improve its u* estimates. Of course, uncertainties in AERMOD's estimates of u* will always exist, due to the inherent natural variability of the atmosphere, and also due to its basic assumption of horizontal homogeneity. Terrain is nearly always heterogeneous, and advection of turbulence will occur from upwind areas.
Empirical corrections to the parameterizations associated with u* and minimum σ v are being tested by the CitationEPA (2012a) in updated beta versions of AERMOD, and indicate that the large overpredictions of concentrations at IF and OR can be substantially reduced. The EPA (2012b) has also made other updates to AERMOD in its operational version 12345 released in December 2012. The combination of an increase in AERMOD's minimum σ v and an adjustment to the u* parameterization in the beta versions is found to remove much of the mean bias. R. Brode (private communication, December 2012) of the U.S. EPA made available the quantitative outputs resulting from the CitationEPA (2012a) testing of AERMOD base version 12345 and the modified versions of AERMOD. The CitationEPA (2012a) evaluations include five AERMOD options:
Option 1. Base AERMOD (December 2012, version 12345). | |||||
Option 2. Option 1 but with no adjusted u*/u, with minimum σ v = 0.3 m/sec (increased from 0.2 m/sec), and with the random-coherent plume interpolation method (but with the time scale Tr reduced from 24 to 12 hr). The adjusted u*/u method is described in the next section, and an explanation of the random-coherent plume interpolation method was provided earlier in this section. | |||||
Option 3. Option 1 but with no adjusted u*/u, with minimum σ v = 0.5 m/sec (increased from 0.2 m/sec), and with no random-coherent plume interpolation method. | |||||
Option 4. Option 2 but with adjusted u*/u (and still with minimum σ v = 0.3 m/sec), and with the random-coherent plume interpolation method (but with the time scale Tr reduced from 24 to 12 hr). | |||||
Option 5. Option 3 but with adjusted u*/u (and still with minimum σ v = 0.5 m/sec and no random-coherent plume interpolation method). |
Note that the CitationEPA (2012a) memo explains that, if the minimum σ v is increased to 0.5 m/sec, there is no longer a need for the term with the time scale Tr , which is another parameterization intended to account for the random/meandering motions that occur under very stable conditions. The two empirical parameterizations (minimum σv term and time scale Tr term) are both intended to account for this enhanced plume spread.
The CitationEPA (2012a) sensitivity study found that AERMOD beta options 4 and 5 provided approximately similar improvements in model performance for the IF and OR field data sets. However, the EPA refrained from recommending any one option, and asked stakeholders to carry out sensitivity runs and report the results to them. Our response was to carry out side-by-side evaluations of the SCICHEM model and the base and optional AERMOD models for the IF and OR field experiment data that were the focus of the CitationEPA (2012a) report.
Anonymous reviewers of this paper have pointed out that many of our references, such as the two low-wind field experiment study reports from the 1970s and the recent AERMOD revisions and evaluations, are available only in “gray literature” agency and contractor reports. Thus, the scientific bases of several of the model assumptions have not been subjected to peer review. Yet these models are being used to make policy decisions. This issue has been the subject of much discussion in the scientific journals, including two editorials by the chief editors of Science magazine (CitationAlberts, 2010; CitationMcNutt, 2013), entitled “Policy-making needs science” and “Improving scientific communication,” respectively. The journal Environmental Science and Policy contains many relevant papers on this topic (e.g., CitationHolmes and Clark, 2008). CitationFrancis et al. (2005) describe a case study (for the state of Washington) of how science in incorporated in environmental policy decisions at various levels of local agencies. In the following sections in the current paper, we insert caveats at a few key steps where the updated science assumptions have not been subjected to adequate peer review.
Low-Wind Stable u* and L Formulas used in Meteorological Processors for Dispersion Models
This section reviews and compares methods for estimating the friction velocity, u* , during low-wind stable conditions, using routine inputs of wind speed and cloudiness from airport weather stations and using parameterizations of the components of the surface sensible heat flux, Hs . Hourly averages are used. The resulting u* (and inferred temperature scale, θ * , and Monin–Obukhov length, L) are used in U.S. EPA applied dispersion models such as AERMOD (CitationEPA, 2004; CitationCimorelli et al., 2005) and SCICHEM (CitationKaramchandani et al., 2000; CitationSykes et al., 1986, Citation2011) to calculate vertical profiles of wind speed, turbulence, and temperature, and to estimate mixing depth. These methods are based on the analysis by CitationVanUlden and Holtslag (1985).
There are many possible sources of meteorological “data” for input to the preprocessor, including single anemometers at a reference height zref , several anemometers at various levels on a meteorological tower, diagnostic mass-consistent wind model outputs based on a network of meteorological sites, and mesoscale meteorological model outputs. Temperature observations can be taken at one or more heights and/or can be provided by meteorological models, too. If fast-response observations of vertical velocity, w, and temperature, T, fluctuations are available (e.g., from sonic anemometers), the sensible heat flux, Hs , can be directly calculated. However, for most routine air permit application projects in the United States, the necessary fast-response data are not available. In fact, for most cases, the source of relevant measurements is not even on site; it may be located at an airport 50 km away.
MOST surface-layer modeling
For the brief discussion in this subsection, it is assumed that the standard (routine) surface weather inputs are available, with one level, zref , of wind observation. In the United States, the standard height, zref , is 10 m for the wind observation. The basic averaging time is 1 hr. Also, routine weather station observations of cloudiness (e.g., fractional cloud cover N) are available, and land-use information (e.g., surface roughness zo , displacement length d, albedo, Bowen ratio BR) is available from tables provided in AERMOD's and SCICHEM's meteorological preprocessors. These tables may appear to be oversimplified, but the data that they summarize are necessary for models that must be applied to areas with minimal information on local conditions (i.e., with no nearby weather station and with only approximate estimates of land use).
In the schemes now being considered, the preceding information on land use is combined with a standard surface energy balance methodology to estimate the sensible heat flux, Hs , for each hour, using methods described by CitationVanUlden and Holtslag (1985). AERMOD and SCICHEM will produce slightly different estimates of Hs , but we will be concerned about those differences only for very light wind limits during stable conditions.
Thus inputs of u(zref ),z o, d, and Hs are available for a given hour. AERMOD and SCICHEM call this the “one-level” type of input. If more than one level of input data is available, gradients can be calculated by the model and used to assist in estimating u* (see CitationEPA, 2004, CitationSykes et al., 2011, and CitationLuhar et al., 2009, for more details of these methods). The multiple-level input scenarios are not considered here, since that type of input is available only in special cases where an on-site meteorological tower has been installed.
Monin–Obukhov similarity theory (MOST) is valid in the surface layer, where the local u* and θ * are within about 10% of their surface value. The surface layer extends to a height zs , using the SCICHEM terminology (CitationSykes et al., 2011). The MO scaling length, L, is defined as –u* 3Tρcp /(0.4gHs ), where ρ is the air density, cp is the specific heat at constant pressure, and g is the acceleration of gravity. The sensible heat flux Hs (positive upward) can be expressed as - ρcp u* θ * . Note that the scaling temperature, θ * , is defined so that it is positive during stable conditions, when Hs is negative. L can also be written as u* 2T/(0.4g θ * ). z/L is a dimensionless stability parameter used in many MOST analyses:
AERMOD and SCICHEM use the well-known MOST wind profile formula:
The “constant” β m has a value of 5 in AERMOD (CitationCimorelli et al., 2005).
SCICHEM (CitationSykes et al., 1986 and Citation2011 and CitationKaramchandani et al., 2000) makes use of the suggestion in CitationVanUlden and Holtslag (1985) that Equationeq 3 is valid only for z/L < 1, and that observations lead to a different parameterization to treat z/L < 5:
This equation implies a β m value of 17 times 0.29 equals 4.93 as z/L approaches zero. At z/L = 5 (the top of the surface layer, zs , defined in SCICHEM), ψ m (z/L) equals 24.65 with Equationeq 3 and equals 13.0 with Equationeq 4. Thus, for a given u* and L, Equationeq 4 gives as much as a factor of 1.9 decrease compared to Equationeq 3 in calculated wind speed at height 5L. For a given wind speed at a height near 5L, Equationeq 4 would yield a u* value that is as much as 1.9 times larger than that from Equationeq 3. A larger u* leads to more turbulent dispersion and smaller concentrations predicted by AERMOD and SCICHEM for near-surface sources.
The meteorological preprocessors for the two models assume that the scaling temperature, θ * , is constant at night for moderate wind speeds and a given fractional cloud cover, N, following a suggestion by CitationVenkatram (1980), who analyzed observations from several field experiments (most at temperate mid-latitude locations):
Estimation of u* using wind speed observations at one level
The term u* and L, zref, zo , and d are required parameters in the wind profile formula ( Equationeq 2) for the stable surface layer listed in the previous subsection. EquationEquation 3 shows that L is a function of u* . AERMOD and SCICHEM also need u* to calculate σ v and σ w . As shown by CitationWeil and Brower (1984) and used in the current AERMOD preprocessor (CitationEPA, 2004), if Equationeq 3 is used in Equationeq 2, and a constant θ * ( Equationeq 5) is assumed during stable conditions, then a quadratic equation in u* results. The following analytical solution has been proposed as a method for estimating u* during stable conditions:
The drag coefficient for neutral conditions, CDN , equals (0.4/ln ((zref – d)/zo )))2 and the speed scale uo = (β mgzref θ * /T)1/2. Note that the ± sign indicates that there are two solutions. However, the positive sign is reported by CitationWeil and Brower (1984) to give the appropriate solution.
If Equationeq 4 is used for ψ m (z/L) instead of Equationeq 3, as done by SCICHEM, then there is no longer a quadratic equation with a simple analytical solution to Equationeq 2. Instead, iteration must be used to solve for u* as is done for unstable conditions by the meteorological preprocessor.
During stable conditions with low observed reference wind speeds, uref , a problem can occur with Equationeq 6, which is used in the AERMOD meteorological preprocessor. The term in the square brackets can become negative. Therefore, there is no solution for low-wind scenarios (i.e., with uref less than about 1 or 2 m/sec for zref equal to 10 m). The term equals zero at a “critical wind speed,” uc , of
For given uref, zref, zo, and d during stable conditions, the critical wind speed, uc , can be thought of as the wind speed below which MOST is not applicable.
At very large wind speeds, uref , the u* solution in Equationeq 6 approaches the solution for neutral conditions:
At small wind speeds, uref , approaching uc from above, u* approaches 0.5 times the above solution for neutral conditions (CitationEPA, 2004).
Thus, the fundamental scientific problem is that if the wind speed observation is from a height above the surface layer, then use of standard MOST surface layer formulas is not justified. This may seem to be a truism or circular reasoning, but it can lead to problems with operational models such as AERMOD, which must be applied to all hours of the year.
SCICHEM explicitly recognizes this fundamental scientific issue and incorporates the simple assumption that at zs < z < zref the wind speed is constant at its value at zref . The MOST formulas apply below zs . It is obvious that the constant wind speed assumption at z > zs is not often valid, as the wind speed can increase or decrease with height above zs . But in the absence of observations at those heights, the constant assumption is useful.
In AERMOD applications, there are likely to be a few hours in a year with very light observed winds at night, when uref < uc. In the technical documentation of the official version of AERMOD (CitationEPA, 2004), the solution in Equationeq 6 is used during stable conditions for uref > uc . However, for uref < uc , where the quadratic equation has no solution, the following simple linear empirical equations are used for stable conditions in AERMOD:
Note that this method gives u* and θ * proportional to uref for stable hours when uref < uc . It is still assumed that, for any given hour, u* and θ * are constant in the surface layer. We stress that the linear formulations in Equationeqs 9 and Equation10 are empirical assumptions that have not been satisfactorily peer reviewed.
SCICHEM imposes an additional condition on θ * so that L is not allowed to drop below an arbitrary minimum value of 10zo . Since a rule of thumb is that zo/ho = 0.1, where ho is the mean height of the roughness obstacles (e.g., grass, bushes), the SCICHEM condition is based on the scientific argument that L marks the depth of the layer where mechanical turbulence dominates, coupled with the fact that mechanical turbulence will dominate at z < ho , where mixing is primarily due to turbulence generated by the flow around the roughness obstacles. Both models’ meteorological preprocessors for the scenario being studied impose a requirement during higher wind stable conditions that the magnitude of the sensible heat flux, Hs , must not exceed a constant of 50 W/m2 (EPA, 2005; CitationSykes et al., 2011). Thus, θ * is not allowed to exceed (50 W/m2)/ρcp u* . Otherwise, the magnitude of the implied Hs would unrealistically increase continually as wind speed uref increases, since the base model assumes that θ * is a constant specified by Equationeq 5. Of course, there are special situations when the magnitude of the sensible heat flux during stable conditions will differ from 50 W/m2 (e.g., advection may be a contributing factor), but the 50-W/m2 limit is intended for general use in routine air quality modeling applications where Hs observations are unavailable.
SCICHEM's meteorological preprocessor recalculates the magnitude of Hs at each iteration as the minimum of four estimates, based on u* θ * from Equationeq 5, u* θ * from Equationeq 11 (shown next), the assumed maximum magnitude of 50 W/m2, and assuming Lmin = 10 zo . With this method, θ * calculated by SCICHEM will decrease as wind speed decreases during stable low-wind periods.
AERMOD revised u* for low-wind stable cases
In the recent update to AERMOD (CitationEPA, 2012a), the U.S. EPA attempts to improve the model's u* estimates during low-wind stable conditions. It adopts CitationQian and Venkatram's (2011) empirical suggestion for eliminating the critical wind speed, uc , issue by approximating the square root of the term in square brackets in Equationeq 6 with an exponential term,
Since the exponential term is never less than zero, an analytical solution is assured for all uref . Neither CitationQian and Venkatram (2011) nor the CitationEPA (2012a) update to AERMOD addresses the fact that θ * also might need to be adjusted for low wind speeds, as accounted for by Equationeq 10 in the operational “base” version of AERMOD. EquationEquation 12 is used in options 4 and 5 of the CitationEPA (2012a) beta versions of AERMOD, briefly described near the end of the Introduction and Background section. Beta option 4 is included in the evaluations in a later section.
Minimum σV and σW Assumptions in Aermod and Scichem
Like many other state-of-the-art short distance plume models, AERMOD and SCICHEM calculate the plume dispersion parameters σ y and σ z by assuming that they are proportional to σ v or σ w , respectively. One-hour averages are used for U.S. EPA regulatory runs. The models’ meteorological preprocessors estimate σ v and σ w from the u* and L estimates described in the previous section. According to MOST, as wind speed approaches zero during stable conditions, so also do u* , σ v , and σ w . Note that MOST only refers to the deterministic part of the relationship. Field observations of turbulent velocity fluctuations do not agree with this zero-wind limit and indicate significant σ v and σ w at night even during very light winds. These fluctuations are attributed to random or uncorrelated fluctuations, which include “meanders” with periods ranging from 20 sec to 1 hr. CitationHanna et al. (1985) and CitationHanna (1990) analyzed several sets of turbulence observations and suggested minimum σ v ranging from about 0.2 to 0.5 m/sec and minimum σ w ranging from about 0.01 to 0.1 m/sec for 1-hr averaging times. The field experiments used in this analysis included open flat terrain (e.g., deserts and oceans), rural terrain with typical patchy fields and woods, and hilly rural terrain.
The base operational version of AERMOD assumes a minimum σ v of 0.2 m/sec and a minimum σ w of 0.02 m/sec (CitationEPA, 2004), while SCICHEM assumes a minimum σ v of 0.5 m/sec and a minimum σ w of 0.1 m/sec (CitationSykes et al., 1986, Citation2011). These differences alone could result in a factor of as much as (0.5/0.2)(0.1/0.02) = 12.5 difference in AERMOD and SCICHEM concentration predictions, with AERMOD predictions being larger. This minimum σ assumption, in addition to the u* calculation methods discussed in the previous section, could account for most of the current AERMOD overpredictions of concentrations at the Idaho Falls and Oak Ridge field sites.
Comparisons of Scichem and Two Aermod Versions for the Idaho Falls and Oak Ridge Field Data Sets
The SCICHEM runs for the IF and OR field data sets are operational runs. They are what a routine user would produce.
The AERMOD 12345 base operational version and beta option 4 version runs for IF and OR were made by the CitationEPA (2012a). They presented quantile–quantile and scatter plots, and not tables of quantitative outputs. Consequently we obtained the tables of AERMOD predicted concentrations from R. Brode (private communication, December 2012). The comparisons in the following use the so-called AERMOD version 12345 base run (the current operational version), and the low-wind beta option 4 run (with adjusted u*/u , with minimum σ v = 0.3 m/sec and with the random-coherent plume interpolation method but with the time scale Tr reduced from 24 to 12 hr). We are not including the other three AERMOD beta options listed in the Introduction and Background section since they tend to clutter up the scatter plots. AERMOD beta option 4 was chosen because it includes revisions to all three methodologies (u*/u, minimum σ v and time scale Tr ).
The primary field data from the Idaho Falls and Oak Ridge tracer release trials used in this comparison are listed in the first six columns and the last column in and , respectively. The continuous source emission rate is listed for each of the 11 IF trials and 6 OR trials. The release height was 1.5 m at IF and 1.0 m at OR. Wind speeds and σθ from an anemometer on the 4-m level of a five-level meteorological tower at IF are listed. However, at OR the standard anemometers had threshold wind speeds above the actual values, so instead, the listed OR wind speeds are based on two orthogonal laser anemometers, which measure line-averaged wind speeds over an approximate 350-m path. The ground elevation under this path was variable and the CitationEPA (2012a) estimates that the effective elevation of the wind observations is 10 m. However, the actual effective height might be anywhere from about 4 m to 10 m, as indicated by the question mark in the column heading for the laser anemometer in At OR, no σθ observations were available.
Table 1. Idaho Falls field experiment observations (from CitationSagendorf and Dickson, 1974) and model predictions (1-hr average) for 11 trials and 3 downwind arcs, where tracer was released from a height of 1.5 m
Table 2. Oak Ridge field experiment observations (from CitationWilson et al., 1976) and model predictions (1-hr average) for 6 trials and 6 downwind arcs, where tracer was released at a height of 1.0 m
For IF, SCICHEM was run using inputs from the five levels of the meteorological tower. The standard procedure with SCICHEM is to use all available observed winds and temperatures if there are multiple levels available on a meteorological tower. SCICHEM was run for OR using a 10-m height for the wind observation, which is the same as the CitationEPA's (2012a) assumption for its AERMOD runs. and 2 also include the arc maximum hourly-averaged observed concentration for each of the three arc distances (100, 200, and 300 m) as well as the SCICHEM and two AERMOD versions-predicted concentrations. For the 100-m arcs, the observed σθc values for the concentration distribution on the 100-m arc are listed, as estimated by CitationHanna (1990).
For the model outputs listed in and , the mean source emission rate, Q, was 0.0320 g/sec at IF and 0.0817 g/sec at OR. The mean wind speed, u, averaged 1.23 m/sec at IF and 0.28 m/sec at OR. The averaged arc-maximum concentration C observed on the 100 m arc was 59.1 μg/m3 at IF and 40.4 μg/m3 at OR. Thus, despite the fact that Q/u was about an order of magnitude larger at OR than at IF (0.292 g/m vs 0.026 g/m), the observed average C (100 m) was about 30% smaller at OR than at IF. Much of the difference can be explained by the fact that the observed lateral spread (σθc ) is a factor of 2.6 larger at OR than at IF. With much smaller (more than a factor of 4) wind speeds at OR, σθ was larger and thus the plume material was spread around most of the 360º circle. The observed spreads agree fairly well with the parameterization σθc = tan−1((0.5 m/sec)/u), where 0.5 m/sec is the “minimum σ v ” (CitationHanna, 1990). The differences in underlying terrain (flat desert at IF and hilly with vegetation at OR) could also contribute to the differences in Q/u at the two sites.
contains quantitative comparisons of the median concentrations and maximum concentrations, predicted by AERMOD and SCICHEM and observed for the three distance arcs at OR and IF. Several statistical model performance measures were calculated using our BOOT model evaluation software (CitationChang and Hanna, 2004), but only a few are included in the table. The fraction of predictions within a factor of 2 of observations, FAC2, is listed as a single number for each field experiment. FAC2 is one measure of the relative mean bias and scatter. The base (operational) version of AERMOD is seen to overpredict the median and the maximum concentrations by about a factor of about 2 at IF and by a much larger factor (about 20) at OR. The difference in the amount of overprediction may be partially due to the much lower (by about a factor of 4) wind speeds at OR, where the base AERMOD's problems with u* and minimum σ v are accentuated. The hillier terrain at OR with clearings and forest interspersed may also contribute to the difference, as well as the uncertainty in the effective height of the laser anemometer measurements above local ground level.
Table 3. Listing of performance measures (median, FAC2, and maximum) for SCICHEM and for two AERMOD options, where the listed FAC2 values apply to all arcs for that field experiment
It is seen in that the predictions of the revised AERMOD beta option 4 have a much smaller mean bias (usually less than a factor of 2) at both IF and OR. The table also shows that the current operational version of SCICHEM performs much better than the base AERMOD and about the same as the revised AERMOD option at both IF and OR. The FAC2 values for SCICHEM and for the revised AERMOD average about 0.65 in the table.
Scatter plots containing the arc maximum concentration predictions and observations listed in and are presented in and , for IF and OR, respectively. (for OR) is broken into two parts, because the AERMOD base version predictions are so much larger (factor of 20) than the observations or the other model predictions. contains the scatter plot for the AERMOD base version, and contains the plot for AERMOD beta option 4 and for SCICHEM. These provide visual confirmation of the quantitative model performance measures listed in . It is clearly seen that performance is much better for IF than OR, and that the performance of SCICHEM and the AERMOD beta option is better than the performance of the base AERMOD. However, the scatter plots allow better understanding of some aspects of model performance. For example, it is seen in (for IF) that the single maximum observed concentration is an outlier, almost 2 times larger than the second highest. The base AERMOD prediction is close to this maximum observation, while the other two models predictions are more than a factor of 2 low. But for most of the observations on the figure, the base AERMOD predictions are too large and the other two models perform better (as seen in the comparisons of medians and FAC2 in ). The scatter plot () for OR for the base AERMOD shows that the model overpredicts at every point by a significant amount. , for OR for the AERMOD beta option and for SCICHEM, shows that the AERMOD beta option does not underpredict at any point whereas SCICHEM underpredicts at 5 of 18 points. Both models in are seen to overpredict by a significant amount (about a factor of 4 for the AERMOD beta option and about a factor of 8 for SCICHEM) at the smallest observed concentrations, which tend to be at the farthest (300 m) arc. These factors of overprediction are still much smaller than the factor of about 67 overprediction by the base AERMOD at that distance.
Effects of use of Aermod Minimum σV and Minimum σW Assumptions in Scichem
Both models use estimates of u* , described earlier, to calculate σ v and σ w based on MOST. The calculated dispersion parameters σ y and σ z are proportional to σ v and σ w , respectively. However, recognizing that observations show that significant random uncorrelated motions (e.g., meandering) occur as wind speed approaches zero in stable conditions, both AERMOD and SCICHEM prescribe a minimum σ v and minimum σ w (CitationEPA, 2004; CitationSykes et al., 1986, Citation2011). If the MOST-calculated σ v and σ w are less than the minimum values, the model uses the minimum value. As mentioned earlier, in reality the minimum value will vary due to differences in upwind terrain and land use and other factors, but these are not well known at routine sites where the model is being used to fulfill a requirement to estimate air quality impacts.
In an attempt to better understand how minimum σ v and minimum σ w are implemented in SCICHEM and the magnitudes of the differences in predicted hourly-averaged arc max concentrations, C, resulting from changes in the minimum σ v and minimum σ w values, we reran SCICHEM for three options:
1. | Use the AERMOD base version minimum σ v of 0.2 m/sec in place of the SCICHEM minimum σ v of 0.5 m/sec. Keep minimum σ w at its value of 0.1 m/sec. | ||||
2. | Use the AERMOD base version minimum σ w of 0.02 m/sec in place of the SCICHEM minimum σ w of 0.1 m/sec. Keep minimum σ v at its value of 0.5 m/sec. | ||||
3. | Change both minimum σ v and minimum σ w to the AERMOD base version values. |
To obtain an initial estimate of the expected effects of these changes in minimum σ v and minimum σ w , the basic Gaussian plume model formulation can be used, which suggests that the arc maximum C is proportional to 1/(σ y σ z ). Next assume that σ y is proportional to σ v and σ z is proportional to σ w . When only the minimum σ v is “triggered,” the simple equation yields the result that the maximum increase in arc maximum C for SCICHEM versus the AERMOD base version is (0.5 m/sec)/(0.2 m/sec) = 2.5. When only minimum σ w is triggered, the maximum increase in arc max C is (0.1 m/sec)/(0.02 m/sec) = 5.0. When both minimum σ v and minimum σ w are triggered, the maximum increase in arc max C is 12.5.
The minimum σ is triggered only if the model's MOST-estimated σ is less than the specified minimum σ. As an example, suppose that the AERMOD and SCICHEM MOST-estimated σ w is 0.05 m/sec. Then AERMOD's minimum σ w of 0.02 m/sec is less than the modeled value, and SCICHEM's minimum σ w of 0.10 m/sec is greater than the modeled value. In this example, AERMOD would use maximum (0.05 m/sec, 0.02 m/sec) = 0.05 m/sec, whereas SCICHEM would use maximum (0.05 m/sec, 0.10 m/sec) = 0.10 m/sec in its dispersion modeling. This can complicate our analysis of changes in modeled C. With this in mind, the following conclusions are found, for SCICHEM runs for the IF and OR trials with the three alternate minimum σ v and minimum σ w assumptions already listed:
1. | Change only to minimum σ v (0.5 to 0.2 m/sec): The simple Gaussian model approach already described leads to the expectation that the predicted arc maximum C (three arcs at each site, with 11 trials at IF and 6 at OR) will increase by about (0.5 m/sec)/(0.2 m/sec) = 2.5, and this is found in the SCICHEM outputs approximately on average (2.78). All field trials and arcs show about the same relative increase (ranging only from 1.90 to 3.70), indicating that the SCICHEM-calculated σv (from MOST) is smaller than both 0.2 and 0.5 m/sec for all trials. | ||||
2. | Change only to minimum σ w (0.1 m/sec to 0.02 m/sec): The simple Gaussian model approach leads to the expectation that the predicted arc maximum C will increase by about (0.1 m/sec)/(0.02 m/sec) = 5, and this is found for 1 (out of 11) of the IF trials and 4 (out of 6) of the OR trials. This means that for those trials with about a factor of 5 increase in C, the SCICHEM MOST-calculated σ w is smaller than 0.02 m/sec. On 8 of the IF trials, there is no change or minimal change in predicted arc maximum C, indicating that the MOST-calculated σ w is larger than both 0.1 m/sec. On 2 of the IF trials and 2 of the OR trials, the predicted concentrations increase by about a factor of about 2, suggesting that the calculated σ w is between 0.02 and 0.1 m/sec. | ||||
3. | Changes to both minimum σ v and minimum σ w : The resulting changes in arc maximum C are “in between” as suggested by the individual results for only minimum σ v and for only minimum σ w . The minimum change (increase) in SCICHEM's arc maximum C is a factor of 2.5, the average is 6.62, and the maximum is 15.1. As shown earlier, from the basic Gaussian plume model formulation, the maximum change is (0.5 m/sec)/(0.2 m/sec)(0.1 m/sec)/(0.02 m/sec) = 12.5. The change in arc maximum C only due to the minimum σ v change would be 2.5, which is the minimum found. The average ratio of 6.62 reflects the full change in minimum σ v and about half of the change in min σ w . |
The resulting changes in SCICHEM-predicted arc max C due to variations in minimum σ v and minimum σ w make sense from a basic science viewpoint. However, since the models use the logic of σ = maximum(MOST σ, minimum σ), then clearly the assumption of minimum σ has no effect on the predicted concentration if minimum σ < MOST σ (as happened for σ w for several of the IF and OR field trials).
Conclusion
This study was initiated after the U.S. CitationEPA (2012a, Citation2012b) released revised versions of its short-distance dispersion model, AERMOD. The AERMOD base version (used for regulatory applications) had been shown to overpredict by a factor of as much as 10 when compared with observed concentrations from the Oak Ridge (OR) and Idaho Falls (IF) field experiments, which were carried out during stable periods when wind speeds often dropped below 1 m/sec. The U.S. CitationEPA (2012a) described the results of its evaluations of the concentration predictions of four revised AERMOD versions (beta options released for sensitivity studies by stakeholders) using the OR and IF data, showing marked improvements in the predictions. We had already carried out some preliminary evaluations of the SCICHEM model (CitationSykes et al., 2011; CitationChowdhury et al., 2012) with these field data sets and believed that its performance was equivalent to that of the new AERMOD beta options. Consequently, we conducted this more extensive comparison of the performance of the AERMOD options with SCICHEM and concluded that, indeed, the performance measures for the AERMOD beta options and SCICHEM were similar.
The four revised AERMOD options involve various combinations of revisions to the u*/u estimation method, the minimum σ v assumption, and the prescribed time scale, Tr , which is an approximation of the time when the mean wind information at the source is no longer correlated with the location of plume material at distance x. A minimum σ v assumption is needed because observations show that as the mean wind speed approaches zero at night, there is always significant σ v . The standard MOST predicts that σ v will approach zero during these conditions. For our comparisons, we chose the predictions of AERMOD beta option 4, with the revised u*/u method, an assumed minimum σ v of 0.3 m/sec instead of 0.2 m/sec, and Tr reduced from 24 to 12 hr. The revised u*/u method intends to correct the base AERMOD's tendency to underpredict u* during light-wind stable conditions, when using a quadratic equation for u* . SCICHEM does not have this tendency to underpredict u* since it uses a different analytical equation from CitationVan Ulden and Holtslag (1985). Furthermore the base version of SCICHEM has an assumed minimum σ v of 0.5 m/sec.
Despite the fact that Q/u was about an order of magnitude larger at OR than at IF (0.292 g/m vs 0.026 g/m), the observed average C (100 m) was about 30% smaller. Much of the difference can be explained by the fact that the observed lateral spread (σθc ) is a factor of 2.6 larger at OR than at IF. With much smaller (more than a factor of 4) wind speeds at OR, σθ was larger and thus the plume material was spread around most of the 360º circle. The differences in underlying terrain (flat desert at IF and hilly with vegetation at OR) could also contribute to the differences in Q/u at the two sites.
The base (operational) version of AERMOD is seen to overpredict the observed median and maximum concentrations by about a factor of about 2 at IF and by a much larger factor (about 20) at OR. As mentioned in the previous paragraph, the difference in the amount of overprediction may be partially due to the much smaller wind speeds at OR, and partially due to the hillier terrain at OR. The predictions of the revised AERMOD beta option 4 have a much smaller mean bias (usually less than a factor of 2) at both IF and OR. SCICHEM performs much better than the base AERMOD and about the same as the revised AERMOD beta option at both IF and OR. The FAC2 values for SCICHEM and for AERMOD beta option 4 average about 0.65.
Scatter plots allow additional insights into model performance. For example, the IF scatter plot shows that the single maximum observed concentration is an outlier, almost two times larger than the second highest. The base AERMOD prediction is close to this maximum observation, while the other two models’ predictions are more than a factor of 2 low. But for most of the observations on the figure, the base AERMOD predictions are too large and the other two models perform better.
Sensitivity runs were carried out with SCICHEM to see the effects of using the AERMOD minimum σ v and/or σ w values in place of the SCICHEM values. The basic Gaussian plume model is used to derive the result that concentration is inversely proportional to σ v σ w . Thus, the replacement of the SCICHEM minimum σ v (0.5 m/sec) with the AERMOD base model value (0.2 m/sec) is expected to lead to an increase in predicted concentrations by a factor of (0.5 m/sec)/(0.2 m/sec) = 2.5. This is found approximately on average (2.78), and all field trials and arcs show about the same relative increase (ranging only from 1.90 to 3.70).
It is concluded that SCICHEM and the revised AERMOD beta option 4 have similar performance when compared to the IF and OR observations. Both are significant improvements over the base AERMOD version (removing most of the large overpredictions). However, it is important to note that the formulations in the operational version of SCICHEM for u*/u and minimum σ v during light-wind stable conditions have been present in the code for at least 10 years. That u*/u methodology is based on a formula suggested by CitationVanUlden and Holtslag (1985) for light-wind stable conditions and does not rely on the types of empirical approximations found in the revised AERMOD beta options.
These conclusions are tempered by the need to have the various empirical modifications to the models adequately peer-reviewed from a scientific perspective before they are used to set agency policy.
Acknowledgment
SCICHEM is used with permission of EPRI. The authors thank Roger Brode of the U.S. EPA for providing the AERMOD outputs used in these comparisons and for providing additional explanations of several of the assumptions in the AERMOD beta versions. They appreciate discussions with Robert Paine of AECOM, Dr. Akula Venkatram of the University of Riverside, and Dr. Ashok Luhar of CSIRO (Australia).
References
- Alperts , B. 2010 . Policy-making needs science . Science , 330 ( 6009 ) : 1287 doi: 10.1126/science.1200613
- Brett , A.C. and Tuller , S. E. 1991 . Autocorrelation of hourly wind speed observations . J. Appl. Meteorol , 30 : 823 – 33 . doi:10.1175/1520-0450(1991)030<0823: TAOHWS>2.0.CO;2
- Brode, R.W. 2012. Appendix W: Clarification memoranda. Presentation at 10th EPA Air Modeling Conference http://www.epa.gov/scram001/10thmodconf.htm (http://www.epa.gov/scram001/10thmodconf.htm)
- Chang , J.C. and Hanna , S.R. 2004 . Air quality model performance . Meteorol. Atmos. Phys , 87 : 167 – 96 . doi: 10.1007/s00703-003-0070-7
- Cheng , Y.G. and Brutsaert , W. 2005 . Flux-profile relationships for wind speed and temperature in the stable boundary layer . Bound. Layer Meteorol , 114 : 519 – 38 . doi: 10.1007/s10546-004-1425-4
- Chowdhury, B., I. Sykes, D. Henn, E. KnippingP. Karamchandani. 2012. Summary of updates to SCICHEM-2012 model and comparison of results with observations and previous version results. 11th Annual CMAS Conference, October 15–17, Chapel Hill, NC http://www.cmascenter.org/conference/2012 (http://www.cmascenter.org/conference/2012)
- Cimorelli , A. , Perry , S. , Venkatram , A. , Weil , J. , Paine , R. , Wilson , R. , Lee , R. , Peters , W. and Brode , R. 2005 . AERMOD: A dispersion model for industrial source applications. Part I: General model formulation and boundary layer characterization . J. Appl. Meteorol , 44 : 682 – 93 . doi: 10.1175/JAM2227.1
- Connors , J.A. , Szembek , C.D. and Paine , R.J. 2010 . AERMOD low wind speed evaluation study results , Westford , MA : Prepared for API by AECOM .
- Etling , D. 1990 . On plume meandering under stable stratification . Atmos. Environ , 24A : 1979 – 85 . doi: 10.1016/0960-1686(90)90232-C
- Francis, T.B., K.A. Whittaker, V. Shandas, A.V. MillsJ.K. Graybill. 2005. Incorporating science into the environmental policy process: a case study from Washington State. Ecol. Society 10:35. (online at http://www.ecologyandsociety.org/vol10/iss1/art35) (http://www.ecologyandsociety.org/vol10/iss1/art35))
- Hanna , S.R. 1983 . Lateral turbulence intensity and plume meandering during stable conditions . J. Climate Appl. Meteorol , 22 : 1424 – 31 . doi:10.1175/1520-0450(1983)022<1424: LTIAPM>2.0.CO;2
- Hanna , S.R. 1990 . Lateral dispersion in light wind stable conditions . Il Nuovo Cimento , 13 : 889 – 94 .
- Hanna , S.R. and Chang , J.C. 1993 . Boundary-layer parameterizations for applied dispersion modeling over urban areas . Bound. Layer Meteorol , 38 : 229 – 59 .
- Hanna , S.R. , Schulman , L. , Paine , R. , Pleim , J. and Baer , M. 1985 . Development and evaluation of the Offshore and Coastal Diffusion Model . J. Air Pollut. Control Assoc , 35 : 1039 – 47 . doi: 10.1080/00022470.1985.10466003
- Holmes , J. and Clark , R. 2008 . Enhancing the use of science in environmental policy-making and regulation . Environ. Sci. Policy , 11 : 702 – 11 . doi: 10.1016/j.envsci.2008.08.004
- Karamchandani , P. , Santos , L. , Sykes , I. , Zhang , Y. , Tonne , C. and Seigneur , C. 2000 . Development and evaluation of a state-of-the-science reactive plume model . Environ. Sci. Technol , 34 ( 5 ) : 870 – 80 . doi: 10.1021/es990611v
- Lilly , D.K. , Waco , D.E. and Adelfang , S.I. 1974 . Stratospheric mixing estimated from high altitude turbulence measurement . J. Appl. Meteorol , 13 : 488 – 93 . doi:10.1175/1520-0450(1974)013<0488: SMEFHA>2.0.CO;2
- Luhar , A.K., and Rayner , K.N. 2009 . Bound.-Lay. Meteorol , and Methods to estimate surface fluxes of momentum and heat from routine weather observations for dispersion applications under stable stratification. 132: 437–454.
- McNutt , M. 2013 . Improving scientific communication . Science , 342 ( 6154 ) : 13 doi: 10.1126/science.1246449
- Mitchell , A.E. and Timbre , K.O. 1979 . Atmospheric stability class from horizontal wind fluctuation . Proceedings of the LXXII Annual Meeting of the Air Pollution Control Association , : 79 – 29.2 . Paper
- Paine , R. , Connors , J. and Szembek , C. 2010 . AERMOD low wind speed evaluation study: Results and implementation , Calgary, Alberta , Canada. Paper 2010-A-631-AWMA : Presented at the 103rd Annual Conference and Exhibition of the Air & Waste Management Association .
- Perry , S. , Cimorelli , A. , Weil , J. , Venkatram , A. , Paine , R. , Wilson , R. , Lee , R. and Peters , W. 2005 . AERMOD: A dispersion model for industrial source applications Part II: Model performance against seventeen field-study databases . J. Appl. Meteorol , 44 : 694 – 708 . doi: 10.1175/JAM2228.1
- Qian , W. and Venkatram , A. 2011 . Performance of steady-state dispersion models under low wind speed conditions . Bound. Layer Meteorol , 138 : 475 – 91 . doi: 10.1007/s10546-010-9565-1
- Rosenberg, N.W.E.M. Dewan. 1974. Stratospheric turbulence and vertical effective diffusion coefficients. 3rd Conference on the Climate Impact Assessment Program, U.S. Department of Transportation, Cambridge, MA, pp. 91–101 http://www.dtic.mil/dtic/tr/fulltext/u2/a019708.pdf (http://www.dtic.mil/dtic/tr/fulltext/u2/a019708.pdf)
- Sagendorf , J.F. and Dickson , C.R. 1974 . Diffusion under low wind speed, inversion conditions , NOAA technical memorandum ERL ARL-52 . http://search.library.wisc.edu/catalog/ocm01707678
- Sykes , R.I. , Cerasoli , C.P. and Henn , D.S. 1999 . The representation of dynamic flow effects in a Lagrangian puff dispersion model . J. Hazard. Mater , 64 : 223 – 47 . doi: 10.1016/S0304-3894(98)00271-4
- Sykes , R.I. and Gabruk , R.S. 1997 . A second-order closure model for the effect of averaging time on turbulent plume dispersion . J. Appl. Meteorol , 36 : 1038 – 45 . doi:10.1175/1520-0450(1997)036<1038: ASOCMF>2.0.CO;2
- Sykes , R.I. and Henn , D. S. 1995 . Representation of velocity gradient effects in a Gaussian puff model . J. Appl. Meteorol , 34 : 2715 – 23 . doi:0.1175/1520-0450(1995)034<2715: ROVGEI>2.0.CO;2
- Sykes , R.I. , Henn , D.S. and Lewellen , W.S. 1993 . Surface-layer description under free-convection conditions . Q. J. R. Meteorol. Soc , 119 : 409 – 21 . doi: 10.1002/qj.49711951103
- Sykes , R.I. , Lewellen , W.S. and Parker , S.F. 1986 . A Gaussian plume model of atmospheric dispersion based on second-order closure . J. Climate Appl. Meteorol , 25 : 322 – 31 . doi:10.1175/1520-0450(1986)025<0322: AGPMOA>2.0.CO;2
- Sykes , R.I. , Parker , S. , Henn , D. and Chowdhury , B. 2011 . SCIPUFF version 2.7 technical documentation , Princeton , NJ : Sage Management .
- Sykes , R.I. , Parker , S.F. , Henn , D.S. and Lewellen , W.S. 1993 . Numerical simulation of ANATEX tracer data using a turbulence closure model for long-range dispersion . J. Appl. Meteorol , 32 : 929 – 47 . doi:
- Trini Castelli , S. and Falabino , S. 2013 . Analysis of the parameterization for the wind-velocity fluctuation standard deviations in the surface layer in low-wind conditions . Meteorol. Atmos. Phys , 119 : 91 – 107 . doi:10.1175/1520-0450(1993)032<0929: NSOATD>2.0.CO;2
- Environmental Protection , U.S. and Agency . 1995 . Users guide for the industrial source complex (ISC) dispersion models, Vol II—Description of model algorithms , Research Triangle Park , NC: U.S : EPA . EPA-454/B-95-003b
- U.S. Environmental Protection Agency. 2004. AERMOD: Description of model formulation. Publication no. EPA-454/R-03-004. Research Triangle Park, NC: U.S. EPA. http://www.epa.gov/scram001/7thconf/aermod/aermod_mfd.pdf (http://www.epa.gov/scram001/7thconf/aermod/aermod_mfd.pdf)
- U.S. Environmental Protection Agency. 2012a. Appendix F. Preliminary Evaluation of Low Wind Beta Options in AERMOD. December 10see Appendix F in the userguide addendum http://www.epa.gov/ttn/scram/models/aermod/aermod_userguide.zip (http://www.epa.gov/ttn/scram/models/aermod/aermod_userguide.zip)
- U.S. Environmental Protection Agency. 2012b. Model change bulletin # 8 (AERMOD, December 10; Julian day 345 in 2012) http://www.epa.gov/ttn/scram/models/aermod/aermod_mcb8.txt (http://www.epa.gov/ttn/scram/models/aermod/aermod_mcb8.txt)
- Van der Hoven , I. 1976 . A survey of field measurements of atmospheric diffusion under low-wind-speed inversion conditions . Nuclear Safety , 17 : 223 – 30 .
- VanUlden , A.P. and Holtslag , A.A.M. 1985 . Estimation of atmospheric boundary layer parameters for diffusion applications . J. Clim. Appl. Meteorol , 24 : 1196 – 207 . doi:10.1175/1520-0450(1985)024<1196: EOABLP>2.0.CO;2
- Venkatram , A. 1980 . Estimating the Monin–Obukhov length in the stable boundary layer for dispersion calculations . Bound.-Lay. Meteorol , 19 : 481 – 85 . doi: 10.1007/BF00122347
- Warner , T.T. , Sheu , R.-S. , Bowers , J.F. , Sykes , R.I. , Dodd , G.C. and Henn , D.S. 2002 . Ensemble simulations with coupled atmospheric dynamic and dispersion models: Illustrating uncertainties in dosage simulations . J. Appl. Meteorol , 41 : 488 – 504 . doi:10.1175/1520-0450(2002)041<0488: ESWCAD>2.0.CO;2
- Weil , J.C. and Brower , R.P. 1984 . An updated Gaussian plume model for tall stacks . J. Air Pollut. Control Assoc , 34 : 818 – 27 . doi: 10.1080/00022470.1984.10465816
- Wilson , R.B. , Start , G.E. , Dickson , C.R. and Ricks , N.R. 1976 . Diffusion under low wind speed conditions near Oak Ridge, Tennessee , NOAA technical memorandum ERL ARL-61 . http://search.library.wisc.edu/catalog/ocm02820503