Abstract
A study of a Powder River Basin (PRB) coal pile found that fugitive emissions from natural and human activity each produced similar levels of downwind fine + coarse (i.e., smaller than 10 µm, or PM10) particle mass concentrations. Natural impacts were statistically removed from downwind measurements to estimate emission factor Ev for bulldozers working on the pile. The Ev determined here was similar in magnitude to emission factors (EFs) computed using a U.S. Environmental Protection Agency (EPA) formulation for unpaved surfaces at industrial sites, even though the latter was not based on data for coal piles. EF formulations from this study and those in the EPA guidance yield values of similar magnitude but differ in the variables used to compute Ev variations. EPA studies included effects of surface silt fraction and vehicle weight, while the present study captured the influence of coal moisture. Our data indicate that the relationship between PRB coal fugitive dust Ev (expressed as mass of PM10 emitted per minute of bulldozer operation) and coal moisture content Mc (in percent) at the study site is best expressed as Ev =10f(Mc) where f(Mc) is a function of moisture. This function was determined by statistical regression between log10(Ev) and Mc where both Ev and Mc are expressed as daily averages of observations based on 289 hours sampled during 44 days from late June through mid-November of 2012. A methodology is described that estimates Mc based on available meteorological data (precipitation amount and solar radiation flux). An example is given of computed variations in daily Ev for an entire year. This illustrates the sensitivity of the daily average particulate EF to meteorological variability at one location. Finally, a method is suggested for combining the moisture-sensitive formulation for Ev with the EPA formulation to accommodate a larger number of independent variables that influence fugitive emissions.
Implications: Fugitive coal dust emission factors (EFs) derived by this study contribute to the small existing knowledge base for a type of pollutant that will become increasingly important as ambient particulate standards become tighter. In areas that are not in attainment with standards, realistic EFs can be used for compliance modeling and can help identify which classes of sources are best targeted to achieve desired air quality levels. Reconciling emission factor formulations that are sensitive to different factors (i.e., those from the EPA and this study) produces a more powerful tool for estimating fugitive emissions at a broad range of sources.
Introduction
Fugitive emissions are emitted gaseous or aerosol materials that do not pass through vents, stacks, or other openings. These emissions can affect air quality near a source and are regulated by federal and state agencies. By their nature, fugitive emissions are difficult to quantify. Indirect techniques have been used to estimate emissions and relate them to some kind of human activity or physical process. For example, Cowherd et al. (Citation1974) employed a mass balance approach to derive fugitive dust emission rates from unpaved roads and other surfaces. The U.S. Environmental Protection Agency (EPA) has adopted emission factors (EFs) based on this method. Fugitive dust EFs for coal mining operations, developed using mass balance techniques, were reported by the EPA (Citation1998). These EFs have been adopted by states and in other countries (e.g., Ghose, Citation2004). Unfortunately, there has been little original research published on fugitive coal dust EF development (other than the work for EPA) and there is a paucity of literature on the topic.
The mass balance method requires extensive monitoring upwind and downwind of a source to ensure that the majority of source emissions are detected. Successful application of this method requires that source attributes be reasonably constant during each monitoring period, that other sources not be present, and that all emitted mass be accurately detected. The method is not without its problems, though. In the case of particulate matter, the variable nature (and in some cases the size) of sources and the size distribution of emitted particles are factors that make it difficult to meet all these requirements. An additional problem occurs when the source is co-located at a site in close proximity to other sources whose emissions may confound measurements. In a summary of fugitive emissions data from construction activities, Muleski et al. (2005) note that vehicle exhaust is often a major contributor to PM2.5 (i.e., airborne mass concentrations of particles with aerodynamic diameters <2.5 µm) emissions when measurements are made in close proximity to moving vehicles. The presence of stationary engines (such as cranes, power shovels, generators, etc.) or burning debris can complicate efforts to assign emissions to vehicles in motion. These issues often require that measurements be made at locations where activity is staged to eliminate undesirable interferences. Venkatram (Citation2000) analyzed limitations associated with the empirical methods used to derive EF formulations like those used by the EPA, including the lack of a mechanistic basis and (as just mentioned) a dependence on the unique characteristics of the data sets from which they are derived. Given these limitations, there is adequate motivation to supplement purely empirical methods with alternate approaches to estimating EFs.
An alternate method for quantifying fugitive emissions is one in which ambient monitoring is combined with dispersion modeling. In this approach, ambient data are combined with detailed meteorological measurements and a model of pollutant transport and dispersion to compute downwind patterns of fugitive pollutants and back-calculate (i.e., inverse model) the source strength. This method was previously applied to estimate particulate EFs for roadways (Venkatram et al., Citation1999) and for a dry fly ash disposal operation (Mueller et al., Citation2013). In the latter study, the source area of fugitive particles covered roughly 8200 m2 (0.8 ha) and the sampling was performed sufficiently far downwind that the fugitive dust plume had adequate time to grow large enough to frequently impact downwind monitors. This method eliminated anything artificial about sampling emissions from a staged activity and allowed study of a source that is rarely found anywhere except at an active coal-burning power plant. Even so, care was needed to identify confounding sources and to remove their contributions to ambient measurements.
Recently, a study was undertaken of fugitive dust from a pile of Powder River Basin (PRB) coal at an active power plant site following methods previously used by Mueller et al. (Citation2013). The pile covered about 6.6 ha and its dimension perpendicular to the predominant wind directions (southwest and south-southwest) was about 380 m (see the next section for details). Monitors were located within 200 m downwind of this pile. Measurements collected thousands of hours of particulate and meteorological data near the pile plus video imagery in an effort to quantify fugitive dust emissions. In the present context, “dust” refers to particles smaller than 10 µm in size (PM10). Previous studies have included particles larger than 10 µm but those particles are unlikely to be transported off-site and have little bearing on air regulatory compliance because current standards focus only on inhalable particulate matter (i.e., PM10 and PM2.5).
Enhanced particulate levels were measured downwind of the pile both with and without human activity on the pile. The primary human activity that produced fugitive dust was the movement of bulldozers across the coal surface, although coal was periodically dropped onto the pile from an overhead conveyor system. The latter activity was infrequent and hours when it occurred were not analyzed. Bulldozers worked to move coal between points on the pile and to scrape the coal surface. The latter activity was needed to suppress the spontaneous combustion of coal dust and is referred to here as “grooming.”
Quantifying coal dust fugitive emissions from bulldozer activity required that natural dust contributions to downwind concentrations be removed from the total observed concentrations. Natural dust emissions are described at length by Mueller et al. (Citation2015) for this site. Their primary finding was that natural processes at the study site produced significant downwind particulate concentrations that could not be ignored when using ambient measurements to determine fugitive emissions from anthropogenic activity. This finding depended in part on knowledge of hourly bulldozer activity, which was documented using a camera. Dust transport and dispersion from the coal pile were subsequently simulated to identify the human-made dust impact. Estimated PM10 emission rates and EFs due to bulldozer activity were then derived based on measured concentrations and observed coal pile activity. An upper limit was estimated for PM2.5 emissions relative to PM10. This paper summarizes the analysis used to estimate the EFs, compares results with those from previous work and provides an example of how the new EF formulation can be applied to a site using representative meteorological data.
Emissions Factor as Engineering Tool
The emissions factor E is an engineering tool to facilitate fugitive emissions estimates in terms of a more easily derived quantity. For example, fugitive dust emissions can be estimated for an unpaved road using a dust emissions factor expressed as mass of dust emitted per vehicle distance traveled. When multiplied by vehicle travel distance per unit time (such as an annual value) this factor yields a mass emission rate.
Emission factors are typically used when emission estimates are needed for air permit applications or when there is a requirement to explicitly model the downwind air quality impacts of a fugitive dust source. In many situations formulas for E do not exist for the type of source in question, requiring use of E formulations for other source types. The primary source of these formulations is the U.S. Environmental Protection Agency (EPA) AP-42 Emissions Handbook (EPA, Citation1995). AP-42 contains fugitive dust EF formulations for aggregate dropping operations and for vehicles driving on unpaved surfaces. In the case of bulldozers driving across a coal pile, the formulation for vehicles driving on unpaved roads at an industrial facility is the one most closely related:
Coal piles exposed to varying weather conditions can have moderate or even large variations in surface moisture content in all but the driest climates. In addition, water spraying is one method used to control wind erosion from coal piles. Therefore, there is a need to derive a dust EF formulation for coal piles that considers the influence of moisture.
Emission Derivation
Measurements
A study of fugitive dust emissions from a PRB coal pile was conducted at the Gallatin (Tennessee) electric power generating station during the summer and autumn of 2012. The area around the coal pile is shown in . Beta attenuation monitors (BAMs) measured hourly PM10 and PM2.5 concentrations at sites labeled 1, 2, and 3 in the figure. The BAM measurement accuracy was ±4 µg m−3 (Mueller et al., Citation2013), whereas the instrumental precision was 1 µg m−3. Frequent southerly winds made site 1 the prevailing upwind site, while sites 2 and 3 were most often downwind. A 10-m meteorological tower near site 2 collected data on winds (three-dimensional sonic anemometers), temperature, and humidity at two levels (2.3 and 9.6 m) and net radiation. A nearby rain gauge, buried resistance sensor, and nephelometer collected data on precipitation, soil moisture content (at 5 cm below the surface), and light scattering at 525 nm. The latter measurement was useful in identifying at high-time-resolution (i.e., 1 min) plumes of particles smaller than about 1 µm. At sites 1 and 2, low-volume Federal Reference Method particle filter samplers collected PM10 mass on Teflon and quartz filters for subsequent chemical analysis. The instrumentation used in this study was the same as that described by Mueller et al. (Citation2013) for a previous study.
Figure 1. Environs surrounding the coal pile (oval black area) at the Tennessee study site. The photograph is oriented north-south from top to bottom. Monitoring sites are denoted by green circles.
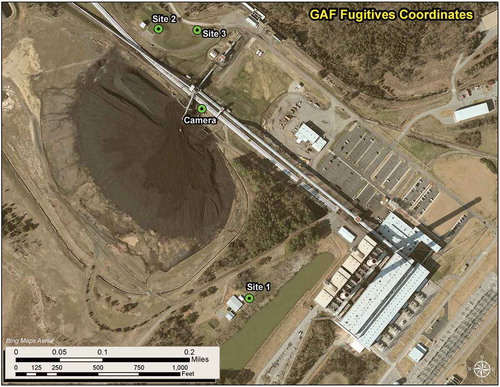
A time-lapse camera (see ) with a 90-degree field of view lens imaged the coal pile and provided information on both human (e.g., bulldozers, other vehicles) and natural (e.g., dust devils, fires) activity at the pile. Site logs provided information on quantities of coal processed, along with the movement of coal through the handling system. On four separate occasions during the study coal samples were collected on the pile. These samples were later analyzed to determine silt and moisture content.
Separating contributions from different dust sources
The excess particulate concentration, Cxs, is defined in terms of the upwind and downwind concentrations:
Average values of Cxs* for hours with and without human activity on the coal pile are summarized in . Concentrations in the presence of human activity (i.e., active bulldozers) were greater than concentrations without activity. The preponderance of nonzero Cxs* for hours without activity implies a “natural” source of particle emissions.
Table 1. Average adjusted PM10 concentration Cxs* for hours without and with human activity on the coal pile
Mueller et al. (Citation2015) identified wind erosion and the action of microscale turbulence (a smaller scale form of wind erosion) on the coal pile as the most probable sources of natural fugitive emissions. AP-42 lists three different values for friction velocity (u*) erosion thresholds associated with coal: 0.55 m s−1 for ground coal, 0.62 m s−1 for scraper tracks on a coal pile, and 1.12 m s−1 for an uncrusted coal pile. The present study used 0.55 m s−1 as the lower limit (ut*) for determining coal dust wind erosion at the study site. AP-42 indicates that wind is nearly 7 times more efficient at lofting particles in the PM10 mass fraction compared to the PM2.5 mass fraction for a given value of u*. Assuming a logarithmic wind speed profile, AP-42 suggests estimating the lowest short-term (i.e., ~1-min) surface wind speed us+ associated with particle erosion as
For hours when wind speed exceeded ut, a statistical model provided a reliable method for calculating the natural fugitive dust concentration excess because it accounted for 70% of the variance in concentration. A model was also derived for early morning and evening hours when wind was below the erosion threshold.
Statistical models linking observed excess particulate concentration due to natural sources, , with site characteristics took the general form
Statistically estimated values were constrained to be no more than the 95th percentile value observed during nonactivity hours to minimize the risk of overestimating natural dust contributions. During midday hours with low wind speeds (i.e., below the wind erosion threshold) for which no statistical model was available, median hourly values of
for each hour from 9:00 a.m. through 6:00 p.m. were applied to hours with pile activity (values ranged from 25 to 34 µg m−3). All estimates of
were subtracted from Cxs* to estimate PM10 concentrations attributable to human activity,
:
Table 2. Comparison of fugitive PM10 concentrationsa associated with natural and human activity on a coal pile (data from both downwind sites combined)
Frequency distributions of Cxs*, and
are illustrated in for hours when winds transported dust from the pile toward downwind monitors. Concentration values from sites 2 and 3 were so similar that data for each variable were combined into joint distribution plots. Values of Cxs* were very skewed, ranging up to 1196 µg m−3 with a mean value of 60 µg m−3 and a median value of 33 µg m−3. By comparison, estimated values of
ranged as high as 130 µg m−3 with mean and median values of 41 and 36 µg m−3, respectively. The distribution of
is not nearly as skewed as that of Cxs*, in part because of the artificial 95th percentile constraint. However, the cap on
is justified because of uncertainty in estimating it and the desire to not underestimate
. Subtracting the two distributions produces a strongly skewed distribution for
with maximum, mean, and median values of 1066, 31, and 3 µg m−3, respectively. This distribution () appears lognormal and is similar to that derived by Mueller et al. (Citation2013) downwind of a fly ash disposal site. Thus, the geometric mean of
—probably the most appropriate metric for describing these data—was 5.3 µg m−3. Note that constraints placed on hourly estimates of
—as derived from eq 8—influenced values at the low end of the distribution. These factors are described in the next section.
Inverse modeling to derive fugitive emission rates
Average concentration (mass per unit volume) of material emitted from a source at rate Q (mass per unit time) is related to the rate by
Coal dust transport and dispersion was simulated using the EPA AERMOD model (EPA, Citation2004) and dividing the pile into three direction sectors, each with two subsectors “a” and “b” (). The direction sectors were each about 30° wide and aligned approximately with the downwind monitoring sites. lists the frequencies associated with airflow across each sector toward sites 2 and 3 along with the observed frequency of bulldozer activity inside each subsector for the hours modeled. Airflow and bulldozer activity were most frequent in sector 1 and least frequent for sector 3. Thus, most dust impacting the monitoring sites originated in sector 1.
Figure 3. Coal pile direction sectors (numbered 1 through 3) and subsectors (labeled “a” and “b” within each direction sector) for which hourly bulldozer activity was quantified by use of camera imagery. The camera was initially oriented to acquire images within the yellow-delineated field of view but was adjusted (red delineation) to capture a more central field of view—and more of sector 3—in September.
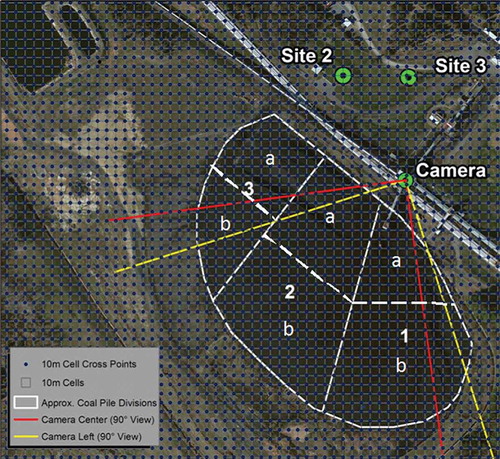
Table 3. Average observed frequencies (weights) used to allocate simulated fugitive dust emissions to each coal pile sector
summarizes sector-averaged meteorological parameters that controlled simulated coal dust transport and diffusion. The parameters (all measured at 10 m) are wind speed and direction (u10 and θ10), the standard deviation of θ10 (σθ), the standard deviation of vertical wind speed w (σw), and turbulent kinetic energy (TKE). Parameter averages were determined only for those 1-min periods during an hour when sector and monitor were aligned (to within ±15°) as determined by the 10-m wind direction. Wind speed in the sectors having the highest values averaged 4–8% more than speed in the sectors having the lowest. Vertical turbulence, represented by σw, averaged 13% higher for the middle sectors than for the lateral sectors. Overall, sector differences translated into values of TKE that averaged 15% more in the middle sectors compared with the flanking sectors. Higher TKE implies more dispersion for dust emitted from the middle sectors.
Table 4. Sector-specific meteorological conditions at 10 m averaged for periods when airflow over the coal pile aligned with downwind monitoring sites
The sector-specific (i.e., based on sector-specific meteorology) dispersion function is denoted and when combined with the adjusted concentration yields
The largest potential for modeling error was when wind speed was intermittent, allowing airborne dust to accumulate over the pile during wind lulls and then transporting dust downwind when winds increased and aligned with the monitors. However, this nonsteady condition violates the steady-state assumption in AERMOD, so there is little expectation that averaging out these periods over an hour would do a better job of representing the dispersion function than limiting the modeling to those subhourly periods when the airflow aligned the source and monitors. This reasoning was the basis for modeling the dispersion function using subhourly meteorological data for minutes when source and monitor were aligned.
The six coal-pile subsectors were each assigned a weight ωj based on the combined frequency of airflow and human activity. Polygonal areas representing each subsector were input to AERMOD and dust emissions were modeled as an area source. This approach facilitated computing Q because it allowed us to weight for the jth subsector according to the frequency with which airflow was aligned with a sector and the frequency with which human activity occurred within each source region during each hour. The particulate emission rate was computed from model results as
Table 5. Source characteristics used to model area emissions from the coal pile
Given the variability in both natural and human dust emissions, it was not surprising that hours occurred when ≥ Cxs*. In these cases,
Computing PM10 emission factors
The PM10 fugitive particulate emission flux (mass per unit area per unit time, Qf10 = Q/A where A is the source area) of coal dust produced by bulldozers operating on the coal pile was calculated from and the total simulated emission rate attributable to all six pile sectors weighted using the joint wind direction and activity frequencies determined for each sector. summarizes Qf10 and several other related particulate fluxes and emission rates. The mean values were skewed because of a small number of high values, so the median values are also provided because they better represent the central tendency of the derived emission flux distributions. The mean values are more than a factor of 10 greater than the medians.
Table 6. Calculated nonzero PM10 coal dust emission rates due to bulldozer activity
The mass emission rate Qe represents the emitted mass per hour computed as
One way to evaluate Ev is to compare its geometric mean with equivalent values computed using the AP-42 formula for the PM10 fugitive EF (mass per distance traveled) for unpaved surfaces at an industrial site (i.e., eq 1). The mean observed s = 5% for the PRB coal with a range of 3.2–9.7%. A geometric mean of AP-42 Ev (note: Ev = VEir) of about 0.13 kg per minute (0.29 pounds min−1) for bulldozer operations was computed using the observed distribution of s and assuming a mean vehicle speed V = 8 km hr−1 (5 miles hr−1). The EF based on the mean s was slightly lower at about 0.12 kg min−1 (0.26 pounds min−1). This compares () to the geometric mean Ev from field data analysis of about 0.03 kg min−1 (0.07 pounds min−1).
Hourly 10-m wind direction indicated airflow across the coal pile (i.e., f > 0) in the absence of precipitation during 177 hours for site 2 and 178 hours for site 3 without human activity seen on the pile and with valid concentration data. Dust dispersion was simulated for all hours when subhourly (1-min) 10-m wind direction aligned a portion of the pile with the downwind monitors. For some hours there were no subhourly winds that aligned the pile and the monitors. This happened because the hourly wind direction was a resultant vector that was occasionally derived from winds blowing from directions on either side of the coal pile but not over the pile. No basis existed for computing dust plumes moving toward sites 2 and 3 without observing subhourly airflow across the pile. A small number of hours that were modeled produced no nonzero dust concentrations at sites 2 or 3. This was likely caused by winds that blew across the edge of the pile and, under stable conditions with little dispersion, did not produce plumes that were sufficiently wide to impact sites 2 or 3. Consequently, there were 38 hours at site 2 and 40 hours at site 3 when no plume impacts at the monitors were computed. The remaining joint (sites 2 and 3) data set contained 277 hours with valid, nonzero dust EFs.
Regarding a PM2.5 Ev
The database was examined for evidence that human activity on the coal pile generated PM2.5 emissions along with PM10. The ratio of [PM2.5]/
[PM10] was 0.14 when bulldozers operated, compared to 0.33 in the absence of human activity. The large decrease when human actions and natural erosion are mixed implies that the ratio due only to human activity is <0.14. Analysis showed that
[PM10] and
[PM10] were roughly equal for hours when human activity was present based on statistical estimates of the natural component. Observed natural PM10 levels were only one-third of PM10 measured in the presence of human activity (), although these data represent different sets of hours. It is likely that the actual ratio of natural to human-derived PM10 was between one-third and one-half. Combining natural particulate levels (having a PM2.5 mass fraction of 0.33) with human-influenced levels (having an unknown fraction of PM2.5) must produce a net ratio
:
This demonstrates that human activity on the pile produces less PM2.5 than natural processes. Comparing the two measured mass size fractions for the same hours revealed a small (r2 < 0.2) linear correlation with confidence exceeding 99% that the slope m (as in the equation PM2.5 = m PM10 + b) was not zero (i.e., m = 0.06). Because natural processes did not show a significant relationship between the two size fractions, this result suggests that some small amount (~6%) of PM2.5 is emitted from human-made actions on the pile. Given that this comparison uses data that include both natural and anthropogenic contributions to airborne particles, it is possible that a greater correlation exists between the two size fractions than is indicated from analysis.
These estimates of (from 0 to 0.06) are smaller than the ratio of 0.10 cited in AP-42 for fugitive particulate emissions associated with vehicle movement on unpaved industrial surfaces. The AP-42 value was not derived from tests of vehicles driving on crushed coal so its applicability is questionable. An upper limit of 0.06 is a conservative value to use based on the data presented here and would be consistent with results described for some western U.S. fugitive dust sources (Western Regional Air Partnership [WRAP], Citation2005).
Meteorological Variability
Derivation of PM10 Ev formula
It is important to know what drives the variation in Ev so that these results can be applied for maximum accuracy. The first step is computing daily averages of log10(Ev), denoted Evτ. The logarithmic transformation is needed to prevent extremely high values from controlling the results. Several potential predictors of Evτ were explored (e.g., temperature, wind speed, solar radiation flux, turbulence intensity, time spent by machinery driving on pile, bulldozer behaviors), but the strongest candidate was Mc. Coal moisture content can be measured from coal samples by comparing sample weights before and after oven drying. The loss in weight is attributable to water and is used to calculate Mc, usually expressed as a percent. This sampling could not be done all the time so a surrogate method was needed to determine Mc. It is likely that soil moisture content, Ms, is a suitable surrogate for Mc because both soil and crushed PRB coal contain particles of similar sizes and share some similarities in hygroscopic chemical constituents (e.g., calcium and other salts and metal oxides). Soil moisture content was measured adjacent to the meteorological tower using an electronic sensor buried roughly 5 cm below the soil surface. Twenty-four coal samples collected on four separate days were analyzed for Mc. Daily average standard deviations of measured Mc were only 2.4%, similar to that for Ms during corresponding rain-free periods. Daily average Mc and Ms were compared and found to share 85% of their variance. The resulting regression equation, (both Ms and Mc expressed as percent), was the basis for values of Mc used in subsequent analyses.
An investigation of soil moisture Ms and environmental factors was made to identify a means of estimating coal moisture content Mc using readily available data. Surface moisture levels result from a balance between water inputs (primarily precipitation) and losses (runoff and evapotranspiration). Runoff is highly variable, is greatest during and immediately following precipitation, and essentially falls to zero after prolonged periods without precipitation. Our soil monitoring site was flat (as was the sampling location for obtaining coal for water content analysis), implying that runoff toward and away from the measurement location was usually slow. Runoff can be neglected because it is likely to be infrequently affected by factors that control evapotranspiration on a 24-hr time scale. Therefore, to statistically analyze daily Ms variability, it is useful to assume that
This is exactly what was found when daily average Ms () was treated as the dependent variable in a multivariate linear statistical regression against the suite of observed meteorological variables. The only meteorological variables found to be significantly correlated with
were daily average 2-m T (
), daily average hourly Rsol (
), and P24 (24-hr precipitation amount). A similar statistical analysis of hourly Ms also indicated a dependence on wind speed but this dependence was not found for daily data.
During the 5-month (June–November) Gallatin coal pile study, more than half the variance in was associated with variance in
and P24, with only a minor amount tied to
. However, to derive a more generalized statistical model of
, a year of data collected previously at a different site in Alabama was included in our analysis. Inclusion of winter months increased the statistical importance of
(presumably because days were included with very low solar input) but still resulted in a regression model that included the same three predictors identified from the Gallatin data set. This model of the combined data set explained about half the total variance in the daily average
. However, an even better model was derived when
from the previous day was included as a predictor. This is because of the high autocorrelation of
with time-lagged values of itself. In such a model, 1-day lagged
accounts for more than 70% of the variance in today’s
, while
and P24 combine to account for an additional 14%. In this model solar radiation and precipitation serve as surrogates for soil (and coal) drying or moistening over the most recent 24-hr period while day-lagged
adds a time-integrated influence (including the effect of past temperature) to the prediction equation that is not included in the previous model based on same-day data. The result is a formulation for
that, when applied to an entire year of data, better replicates the observed daily variation in moisture content. Application of this method requires an initial guess of
but the influence of the initial guess disappears after only a couple days of
calculations. Initial values of
should be in the range typical for PRB coal: between 15 and 35%.
The following formulation is based on data from a wide range of meteorological and seasonal conditions encompassing 361 days at two sites (total r2 = 0.84):
Analysis of Evτ was based on daily average values (to reduce data noise) compared with corresponding averages of other variables, including Mc and Rsol. Vehicle weight and speed were constant or nearly so and did not provide good predictors. The resulting data set contained 44 sets of values. Multivariate modeling of Evτ identified only one predictor, Mc, with statistical confidence at >99%.
The study was divided into two periods distinguished by whether routine coal removal (reclamation) from the pile was occurring. Exclusive reclamation began October 15 and continued through the end of the study. Ninety-five percent of the coal added to the pile during the study was added before August 9 and no coal additions were made after October 7. Of the coal removed from the pile, 70% occurred after October 15. Thus, before October 15, work on the pile was mostly focused on suppressing coal dust combustion (coal removal occurred sporadically on 30 of 107 days during this period). Coal surface grooming, occurring less than 8 hr/day, involved a bulldozer scraping its blade across the top of the coal to mix the top layer with underlying coal containing higher moisture content. Beginning October 15, bulldozers worked daily (12+ hours on some days) pushing coal toward an underground hopper for conveyance to the plant. During reclamation, bulldozers also continually reshaped the pile as coal was removed and this involved more pushing of surface coal.
A statistical comparison was made of Evτ with Mc for the two different periods. Results are illustrated in . There is a clear negative association between Evτ and Mc that is different for each period. The regression for each period captures about half (46–52%) of the variance in Evτ. The remaining variability is due to measurement and modeling error, uncertainty in the correction to remove the natural dust component, and unknown variations in Mc across different portions of the pile. Regression slopes for the two periods are different, with less sensitivity to Mc and much higher values of Ev during the drier period. This difference could be influenced by the nature of the bulldozer work as previously described but the operational differences are subtle at best. The difference is more likely attributed to a seasonal effect driven by the drying capacity of the atmosphere. The cooler autumn period means less drying of the coal surface and higher Mc. The tendency for Evτ to decline more quickly as Mc increases is probably independent of season and bulldozer mode of action. Subbituminous coal becomes “sticky” (i.e., poses handling problems) with high moisture content. This change in physical characteristic—observed firsthand when taking coal samples for analysis—is likely the reason for the faster decline in Ev with increasing Mc because “sticky” coal has higher cohesive forces binding particles together. Note that the crushed coal has the consistency of wet concrete for Mc > 30%.
Figure 5. Plot compares daily average log10(Ev) versus Mc for separate periods either ending before or beginning on October 15. Separate linear regression lines (and coefficients of determination) are shown for each period. The two lines intersect at roughly Mc = 29.5%.
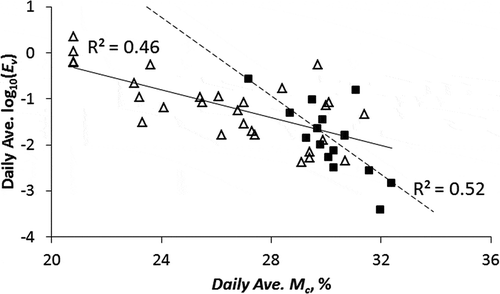
Daily average values of Evτ versus Mc were alternately fitted with different regression curves (i.e., linear and polynomial formulations for Mc), but none provided a more satisfactory fit than those illustrated in . Given the differences in the two data subsets previously described, it is recommended that either one or two linear regression formulas be used depending on circumstances. There is little data to support the Evτ extrapolated behavior at the extremes of Mc so caution is advised. A single formulation would be most appropriate in situations where Mc is always <30% (i.e., the “Before” data set regression in ). This is because the two linear equations shown in intersect at Mc ≈ 29.5%. For Mc > 30% the second formulation (the “After” data set regression in ) would be better because it reflects what is expected to be a rapid decline in Ev as the coal pile takes on a change in physical attribute. Thus, for Mc <29.5%,
compares Ev (using eqs 23 and 24) with Ev derived from V∙Eir (denoted Ev(AP)) based on typical conditions for bulldozers operating on the PRB coal pile (s = 5%; W = 66 tons; V = 8 km hr−1) and eq 1. The formula for Eir does not include a variation with surface moisture so it is a constant 104 g min−1 in the plot (the likely range in Ev(AP) due to s and V variability is also illustrated). On average, Ev > Ev(AP) for Mc < 25%. This comparison suggests that a very dry surface of PRB coal is capable of emitting PM10 at a rate above that indicated by Ev(AP) for dry surfaces. However, the emission rate drops quickly and becomes negligible for moist surfaces. For reference, also shows the AP-42 moisture-dependent EF for unpaved public roads, assuming the same bulldozer speed and surface conditions as the coal pile. This formulation is not applicable to bulldozer work on coal piles but is shown here as a point of reference to illustrate the expected lower limit of fugitive emissions from surfaces that emit a lot less dust because of frequent use and compacted surfaces. The next section describes an example of how to apply this method for estimating and daily average Ev (
) from standard meteorological data.
Figure 6. Comparison of different values of Ev versus Mc using emission factors from AP-42 for unpaved public roads, unpaved industrial surfaces, and from this study. The potential variability of the Ev(AP) for industrial sites—assuming 25% variability in vehicle speed and surface silt content—is illustrated using dashed lines above and below the parallel solid line.
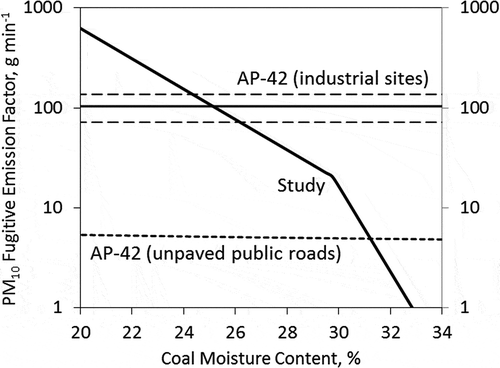
Application of Ev formula
Application of the coal dust EF Ev formulation is illustrated here using data collected by the National Weather Service (NWS) in Nashville, TN (temperature and precipitation), and in Bowling Green, KY (solar radiation). Nashville measurements are made a little more than 30 km southwest of the study site, whereas solar radiation data were collected about 65 km north of the site.
A time-series plot of daily average based on actual meteorological data is presented in . The pattern that emerges is one in which
is highest (29–33%) in winter and autumn and lowest in the middle of summer (<27%). Daily variations in moisture content can be large because of precipitation variability. plots the time series of
derived using estimated
. Emission factors tended to be lowest in winter and autumn—coinciding with higher
–and highest in summer when moisture content was lowest. The AP-42 value for Ev is plotted for comparison. A value of 104 g min−1 (229 lb min−1) was computed for the coal pile activity at the study site. Moisture content is not a factor in the AP-42-derived Ev so no daily variations exist. However, AP-42 allows assuming
= 0 on days when precipitation ≥0.01 inches is observed. On many of those days, our moisture-sensitive Ev produces such low emissions that they are essentially zero anyway. The AP-42 Ev is twice the annual average Ev (53 g min−1) derived from field data. In itself this difference is not too remarkable, given the uncertainty in the Ev estimates. What makes the AP-42 formulation inadequate is its inability to account for the effect of coal moisture on Ev, and this can produce large annual overestimates of fugitive emissions in regions where precipitation is plentiful for at least a portion of the year. Also, in situations where air quality modeling of fugitive emissions is required, a method for computing variations in Ev should produce more realistic results than assuming constant Ev.
Figure 7. Daily average coal moisture content for 2012 computed from hourly meteorological data measured near Nashville, TN, and eq 22. The period of the field study is denoted by the solid horizontal line in the upper part of the graph.
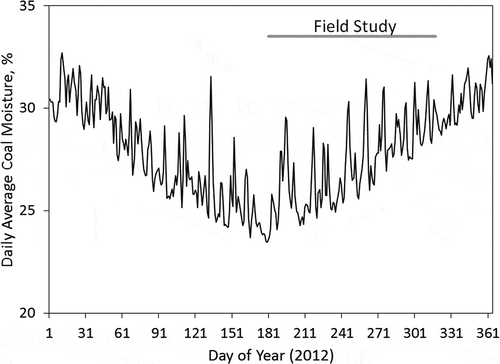
Figure 8. Daily emission factors for fugitive PM10 coal dust calculated using the formulations for Ev versus Mc (eqs 23 and 24) and Mc plotted in . The period of the field study is denoted by the solid horizontal line in the upper part of the graph. The Ev(AP) (= VEir) is represented by the horizontal dashed line near 100 g min−1, and the annual average of Ev based on the study formulation is represented by the dashed line parallel to and below the line for Ev(AP).
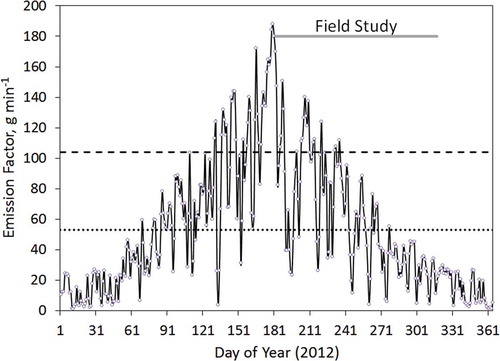
Ev Sensitivity
Although these results are specific to PRB coal, there is some question as to how to apply the derived EF formulation to other sites. First, note that vehicle speed is currently not a parameter used to estimate Eir using the AP-42 formulation (eq 1) and may not vary sufficiently to enable quantification for work on a coal pile. Given that our Ev formulation produces values that are greater than, equivalent to, and less than those from AP-42 (i.e., Ev(AP)) depending on Mc, it is not unreasonable to assume that the two EF approaches provide insight into a common phenomenon that has been measured using two different methods. This is likely true within the accuracy of either methodology for determining E. Therefore, it is appropriate to combine the Ev formulation derived here with the sensitivity of Eir to vehicle weight W and surface silt content s because the data on which Ev is based represent values of W and s that were within the range of values tested to develop the AP-42 Eir formulation. Put another way, how could assuming similar sensitivities to W and s result in greater uncertainty than using a formulation not based on PRB coal that ignores Mc?
Let Eir = f(s,W) represent eq 1. Assuming it is better to consider Mc than to apply a formulation that ignores it, it is suggested that
Figure 9. Sensitivity of Ev to various independent variables as determined from eq 27.
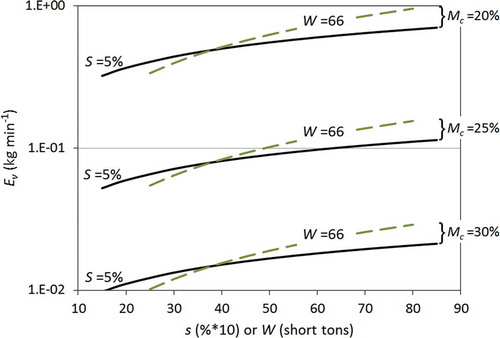
Conclusion
This study quantified the primary source of fugitive dust emissions from human activity on a PRB coal pile. Emissions from bulldozers working on the pile were estimated after removing the contribution from natural dust emissions. Bulldozer activity is needed to either groom or scrape the coal to minimize dust combustion or push coal around the pile (either toward an underground bunker for reclamation to the plant or to redistribute coal dumped onto the pile by the conveyor system). Coal dumping—another source of fugitive dust—occurred too infrequently to quantify its impact on dust levels.
It appears that downwind fugitive dust levels (as PM10) from natural processes can occasionally be as high as or higher than levels from human activity. This makes it imperative that natural impacts on downwind concentrations be considered when using monitored concentrations to estimate fugitive dust impacts from human activity. Natural and human-made fugitive dust emissions produce similar downwind impacts, in part because both processes tend to remove similar-size particles by mechanical means from the same surface. Also, the lengths of time involved in natural and anthropogenic particle-emitting actions are similar in that both are transient processes that do not stay in one location for very long. Thus, both types of dust-emitting events share similar origins and similar downwind signatures.
The movement of bulldozers across the coal pile was associated with an increase in downwind concentrations of PM10. Emissions of PM2.5 were ≤6% of PM10 emissions. Dispersion modeling of dust transported from the pile coupled with estimated anthropogenic levels of PM10 derived EFs that were smaller than those computed using an AP-42 formulation, which was not developed using coal pile data and does not consider moisture effects. Uncertainty in the EFs was large because of the uncertainty in estimated levels of natural particles. However, daily averaging of EFs and coal moisture content revealed that the latter is an important predictor of emissions. In fact, the data indicate that the EF for fugitive coal dust decreases by two orders of magnitude as coal moisture content increases from 21 to 33% and essentially vanishes for Mc >33%. This is a valuable finding because the applicable AP-42 formulation does not allow for variation with coal moisture content.
Daily average coal moisture content was found to be strongly associated with precipitation, air temperature, and solar radiation, parameters that serve as surrogates for water input and evaporation processes. Time-lagged surface moisture is an even better predictor of daily surface moisture because it provides a superior representation of time-integrated factors controlling the surface water balance (i.e., it eliminates the need for temperature or rainfall history in the form of previous-day temperature and precipitation data). A time series of surface moisture can be built from available data on precipitation and solar radiation. Potential meteorological predictors are available from on line National Oceanic and Atmospheric Administration (NOAA) data records and can be used to estimate fugitive coal dust emissions for periods when it is not raining and temperatures average above freezing. An alternate estimation methodology would be needed for wintertime in cold climates. The value of this approach is evident for plants located where frequent rainfall tends to add moisture to the coal, with the resulting emissions being lower than those otherwise derived using AP-42.
All of the preceding conclusions are for a PRB coal pile. The characteristics of PRB coal (average silt content of 5%) are such that the EFs derived in this study may overstate emissions from work on piles composed of different coal types. However, the moisture effect may also be unique to PRB coal. Additional research is needed to determine whether the EFs derived here are applicable to other coals.
Funding
The authors are grateful to the Gallatin plant personnel who supported the field measurement phase of this effort. Funding for this work was provided by the Electric Power Research Institute and the Tennessee Valley Authority.
Additional information
Notes on contributors
Stephen F. Mueller
Stephen F. Mueller, Jonathan W. Mallard, and Qi Mao perform environmental studies for the Tennessee Valley Authority.
Jonathan W. Mallard
Stephen F. Mueller, Jonathan W. Mallard, and Qi Mao perform environmental studies for the Tennessee Valley Authority.
Qi Mao
Stephen F. Mueller, Jonathan W. Mallard, and Qi Mao perform environmental studies for the Tennessee Valley Authority.
Stephanie L. Shaw
Stephanie L. Shaw is a senior project manager for air quality studies at Electric Power Research Institute.
References
- Cowherd, C., Jr., K. Axetell, Jr., C.M. Guenther, and G.A. Jutze. 1974. Development of Emission Factors for Fugitive Dust Sources, Midwest Research Institute report to the EPA. EPA-450/3-74-037. http://nepis.epa.gov/Adobe/PDF/2000MC6B.PDF.
- Ghose, M.K. 2004. Emission factors for the quantification of dust in Indian coal mines. J. Sci. & Ind. Res. 63, 763–768.
- Mueller, S.F., J.W. Mallard, Q. Mao, and S.L. Shaw, 2013. Fugitive particulate emission factors for dry fly ash disposal. J. Air Waste Manage. Assoc. 63. doi:10.1080/10962247.2013.795201
- Mueller, S.F., J.W. Mallard, and S.L. Shaw. 2015. Variability of natural dust erosion from a coal pile. J. Appl. Meteor. Climatol. doi:10.1175/JAMC-D-14-0126.1
- U.S. Environmental Protection Agency. 1995 with subsequent updates. Compilation of Air Pollutant Emission Factors—Volume I: Stationary Point and Area Sources, AP-42 Fifth Edition. Research Triangle Park, NC: Office of Air Quality Planning and Standards.
- U.S Environmental Protection Agency. 1998. Revision of emission factors for AP-42 Section 11.9—Western Surface Coal Mining. Prepared by the Midwest Research Institute for Office of Air Quality Planning and Standards. Research Triangle Park, NC. http://pubs.usgs.gov/pp
- U.S. Environmental Protection Agency. 2004. User’s Guide for the AMS/EPA Regulatory Model—AERMOD. EPA-454/B-03-001. Research Triangle Park, NC: Office of Air Quality Planning and Standards.
- U.S. Geological Survey. 1999. Coal quality and geochemistry, Powder River Basin, Wyoming and Montana. In 1999 Resource Assessment of Selected Tertiary Coal Beds and Zones in the Northern Rocky Mountains and Great Plains Region, chapter PQ. Report 1625-A, p. 27. http://pubs.usgs.gov/pp/p1625a/Chapters/PQ.pdf
- Venkatram, A. 2000. A critique of empirical emission factor models: A case study of the AP-42 model for estimating PM10 emissions from paved roads. Atmos. Environ. 34:1–11. doi:10.1016/S1352-2310(99)00330-1
- Venkatram, A., D. Fitz, K. Bumiller, S. Du, M. Boeck, and C. Ganguly, 1999. Using a dispersion model to estimate emission rates of particulate matter from paved roads. Atmos. Environ. 33:1093–1102. doi:10.1016/S1352-2310(98)00316-1
- Western Regional Air Partnership. 2005. Analysis of the fine fraction of particulate matter in fugitive dust—Final report, Midwest Research Institute report for the Western Governors’ Association Western Regional Air Partnership. http://www.epa.gov/ttnchie1/ap42/ch13/related/mri_final_fine_fraction_dust_report.pdf.