ABSTRACT
Wireless communication systems have been broadly applied in various complicated traffic operations to improve mobility and safety on roads, which may raise a concern about the implication of the new technology on vehicle emissions. This paper explores how the wireless communication systems improve drivers’ driving behaviors and its contributions to the emission reduction, in terms of Operating Mode (OpMode) IDs distribution used in emission estimation. A simulated work zone with completed traffic operation was selected as a test bed. Sixty subjects were recruited for the tests, whose demographic distribution was based on the Census data in Houston, Texas. A scene of a pedestrian’s crossing in the work zone was designed for the driving test. Meanwhile, a wireless communication system called Drivers Smart Advisory System (DSAS) was proposed and introduced in the driving simulation, which provided drivers with warning messages in the work zone. Two scenarios were designed for a leading vehicle as well as for a following vehicle driving through the work zone, which included a base test without any wireless communication systems, and a driving test with the trigger of the DSAS. Subjects’ driving behaviors in the simulation were recorded to evaluate safety and estimate the vehicle emission using the Environmental Protection Agency (EPA) released emission model MOVES. The correlation between drivers’ driving behavior and the distribution of the OpMode ID during each scenario was investigated. Results show that the DSAS was able to induce drivers to accelerate smoothly, keep longer headway distance and stop earlier for a hazardous situation in the work zone, which driving behaviors result in statistically significant reduction in vehicle emissions for almost all studied air pollutants (p-values range from 4.10E-51 to 2.18E-03). The emission reduction was achieved by the switching the distribution of the OpMode IDs from higher emission zones to lower emission zones.
Implications: Transportation section is a significant source of greenhouse gas emissions. Many studies demonstrate that the wireless communication system dedicated for safety and mobility issues may contribute to the induction in vehicle emissions through changing driving behaviors. An insight into the correlation between the driving behaviors and the distribution of Operating Mode (OpMode) IDs is essential to enhance the emission reduction. The result of this study shows that with a Drivers Smart Advisory System (DSAS) drivers accelerated smoothly and stopped earlier for a hazardous situation, which induce the switch of the OpMode IDs from high emission zones to lower emission zones.
Introduction
A transportation system is indispensable to enhance the mobility of passengers and goods in an efficient way. However, there are two sides to every coin. According to the U.S. Environmental Protection Agency (EPA, Citation2015a), the transportation sector directly accounts for 27% of total greenhouse gas emissions in 2013. In particular, the burning fossil fuel for passenger cars contributes 34% of the total emission from the transportation sector (U.S. Department of Transportation [DOT], Citation2006). In the transportation sector, improper driving behaviors, such as excessive acceleration, rapid deceleration, or aggressive overtaking, could lead to statistically significant higher emission and fuel consumption (Li et al., Citation2014). These driving behaviors may raise a higher probability of non-recurring events, such as congestion and crash, particularly when merging to a work zone in heavier traffic volumes, consequently resulting in an increase in unexpected travel delays. More than 480 million vehicle hours of unexpected travel delay were recorded from roughly 7,200 work zones in the summer of 2003 (U.S. DOT, Citation2009). The area of work zones has been considered as one of the hotspots of traffic incidents and congestions.
CO2 is the major emission in the combustion process of a carbon-based fuel and has been identified as a greenhouse gas (Schmidt et al., Citation2010), but it is an essential chemical for plants as well. During an excessive acceleration more unexpected air pollutants, mainly carbon dioxide (CO), hydrocarbons (HC), and nitrogen oxides (NOx), may be produced. While CO and HC result from incomplete combustion (EPA, Citation1994), NOx is formed by the interaction between nitrogen and oxygen atoms in the air at a higher temperature and pressure in an engine. The NOx emissions are primarily in the form of NO, and less than 10% are NO2 (Longhurts, 1989). Though NOx is not a greenhouse gas, it can react with HC and still air under sunlight to form smog that is toxic to humans. What is more, the smog contains a harmful gas called ozone, which can cause coughing and throat/chest irritation, fatal asthma attacks, and difficulty breathing and lung damage (Healthline, Citation2013). Besides, NOx is associated with the formation of acid rain. Therefore, the NOx emission has been involved with the air quality assessment, which is one of criteria to measure the effectiveness of a transportation system (Roess, Citation2010).
The Environmental Protection Agency (EPA) model MOtor Vehicle Emission Simulator (MOVES) (EPA, Citation2012) is widely used to estimate vehicle emissions, and works at national, county, and project levels. The estimated emission includes criteria pollutants (e.g., NOx, HC, CO, and PM), greenhouse gases (e.g., CO2), and air toxics (EPA, Citation2015b). MOVES is a good tool to evaluate the air quality impacts of different traffic operation and environment protection strategies.
To improve the air quality in transportation systems, the U.S. Department of Transportation (U.S. DOT) proposed a program to develop the “Applications for the Environment: Real-Time Information Synthesis (AERIS),” aiming to “generate and acquire environmentally-relevant real-time transportation data, and use these data to create actionable information that support and facilitate ‘green’ transportation choices by transportation system users and operators” (U.S. DOT, Citation2015a). This includes the Eco-Signal Operations, Eco-Lanes, Low Emissions Zones, Eco-Traveler Information, and Eco-Integrated Corridor Management. The main focus was to characterize the correlation between transportation and the environment.
Mobility and safety are also the main concerns of designing a transportation system (Roess, Citation2010). The advent of new technologies in varied areas, such as wireless communication engineering, electronic engineering, computer engineering, computer science, and automatic control engineering, has promoted innovation in vehicle and infrastructure systems and traffic control systems for better mobility and safety on roads. Recently, the U.S. DOT launched a “Connected Vehicle” program, aiming to develop a fully connected transportation system for “safe, stable, interoperable, reliable system operations” that minimizes risk and maximizes opportunities (U.S. DOT, Citation2015b).
A Drivers Smart Advisory System (DSAS) is one of the newly developed systems, adapting innovative wireless technology to build up vehicle-to-infrastructure (V2I) communication. The DSAS is dedicated to enhance the safety on roads by providing real-time advance messages on upcoming traffic control information (Qiao et al., Citation2014; Li et al., Citation2015b). Such a DSAS employs radiofrequency identification (RFID) technology to place tags on roadside infrastructure, while deploying a reader (also called a receiver) inside the vehicle by connecting to a carry-on or embedded computer unit. Qiao et al. (Citation2014) briefly tested the impacts of the DSAS on drivers’ driving behaviors in two work zone areas. Although the results were promising, safety was not ensured in such an on-road test with a crossing worker. Also, it is still unclear how and to what extent a wireless communication system like the DSAS can enhance the safety and improve the air quality.
With regard to this, the objective of this paper is to explore the implications of a wireless communication system on vehicle emissions through changing drivers’ driving behaviors, in terms of the operating mode (OpMode) ID distributions on emission estimation and the NOx versus CO2 diagram. Meanwhile, the impact of DSAS on safety in a work zone is evaluated by drivers’ headway distance and stopping distance for a hazardous situation.
Methodology and data collection
Testing method
In this research, the impacts of a wireless communication system on vehicle emissions and safety on roads were tested in a simulated environment within a test bed of a typical rural work zone area. The wireless communication system in the test was a simulated DSAS, which could provide drivers with both work zone warning messages and safety-warning messages. A pedestrian was preset to cross the street within the activity area of the work zone. Drivers’ driving performances along the advance warning area and within the activity area were recorded for further statistical analysis. Vehicle emissions were then calculated based on operational activities using MOVES 2010b (EPA, Citation2011).
Scenarios design
The test track and three pairs of scenarios in a typical work zone were designed as shown in , incorporated with the illustration of the advance warning area and activity area. is for the tests of a leading vehicle entering the work zone and encountering a pedestrian crossing the street within the activity area. The entire path is split into two regions.
The first region in is within the advance warning area, which is for the first pair of scenarios, LW–D and LW+D. Here, letter “L” is for leading vehicle, “W” for advance warning area, and “D” for DSAS. The symbol “–” stands for “without,” while “+” stands for “with.” An advanced DSAS message “Work Zone Ahead” is provided at a location, 570 m toward the beginning of the activity area. Due to the perception–reaction time needed, the drivers would react starting from the location of 500 m, which is the beginning point for data processing later on.
The second region is within the activity area for the second pair of scenarios LA–D and LA+D. Letter “A” is for the activity area. The remaining letters and symbols have the same meaning as in the first pair of scenarios. A pedestrian walks out at the location of –400 m (the negative number means the location after the beginning of the activity area). Another warning message of “Pedestrian Crossing” is prompted at the location of 280 m to the leading vehicle.
shows the pair of scenarios FF–D and FF+D for a following vehicle positioned at the end of the advance warning area. The first letter “F” is for the following vehicle, and the second letter “F” for forward collision message (FCM). The rest of the letters and symbols are the same as for the previous scenarios in the simulation. The leading vehicle had to stop to prevent a crash with a pedestrian from occurring within the activity area. Unfortunately, a heavy truck blocked the vision of the following vehicle, which was going to change to the left lane to enter the work zone activity area. A smartphone-based DSAS warning message is provided to the following vehicle, with the intention that the vehicle could stop almost simultaneously with the leading truck.
Driving simulator and subject recruitment
A driving simulator has been extensively used to test drivers’ driving behaviors for various purposes, such as risk assessment (Ba et al., Citation2015; Li et al., Citation2014) and vehicle emissions (Li et al., Citation2015a). In this paper, a DriveSafety DS-600c driving simulator was employed as the test equipment, which can realistically simulate a driving environment and the vehicle’s dynamic motion. Forty subjects were recruited to be the driver in the leading vehicle on the test track in , while another 20 subjects were the driver in the following vehicle on the track in . The demographics of all subjects followed the real distribution of Houston, TX, based on the 2010 U.S. census database (U.S. Census Bureau, Citationn.d.).
Test procedure
Each subject was instructed to go through a training procedure for about 5 min to become familiar with the simulation environment. After that, each subject drove twice along one of the tracks illustrated in . The first round of driving was with no warning message, while the second round was with the DSAS messages.
Given that the vehicle’s engine was warmed up while driving through the work zone, catalyst temperature was not taken into account in the emission estimation using MOVES.
Data collection and processing
Vehicle geo-locations, speed, acceleration rates, braking, and other relevant information were recorded during the entire tests. The sampling rate of record was 60 Hz. The time-based data sets from the driving simulator were converted into space-based attributes, so that the driving activities (e.g., speed, acceleration rates, and brake information) could be aggregated among all subjects within the test group. This was achieved by an interpolation procedure, which was to convert the evenly distributed 1/60-s data sets along the time domain into new data sets with even 1-m intervals.
Emission estimation method
The estimation of vehicle emissions of the tests in this paper follows the EPA model MOVES, which is currently a standard tool for emission estimation from transportation sector. MOVES defines bins of vehicle specific power (VSP), which is the instantaneous tractive power per unit vehicle mass determined by aerodynamic drag, acceleration, rolling resistance, grade, and the mass of the vehicle (Jimenez-Palacios, Citation1999; Pease, Citation2009). The VSP for light-duty vehicles was calculated using eq 1:
where v is the vehicle speed (m/s), a is the vehicle acceleration (m/s2), and grade (%) is the vehicle vertical rise divided by slope length.
The data collection area was set as a plain, so the grade was 0 in eq 1. Therefore, eq 1 was simplified as eq 2:
The VSP distribution represents the driving characteristics, and is highly correlated with the vehicle emission characteristics (Jimenez-Palacios, Citation1999; Zhai and Frey, Citation2008; Lai et al., Citation2013; Hill et al., Citation2014). The accuracy of the VSP-based modeling approach relies on how VSP bins are defined. Like many of other studies related to vehicle emissions (Carrera, Citation2015; Zhou et al., Citation2015; Hoover et al., Citation2015; Li et al., Citation2015a), the cut points of the VSP by MOVES 2010b were adopted in this study. The operating mode ID was categorized to describe vehicle behaviors based on the instantaneous speed and VSP characteristics.
illustrates the detailed operating mode binning provided by MOVES 2010b (EPA, Citation2011). In , the operating mode ID refers to operating mode bins that represent braking, idling, and varying levels of the vehicle’s VSP and speed. The operating mode bins were broken down into 23 bins with the IDs of 0 to 40. Bins 0 and 1 represent braking and idling; bins 11 to 16 represent driving behavior with lower speeds; bins 21 to 30 represent driving behavior between 40 km/h (25 mph) and 80 km/h (50 mph); and bins 33 to 40 represent driving behavior with speed 80 km/h (50 mph) and greater.
Table 1. Definition of MOVES operating mode characteristics (EPA, Citation2011).
In this study, the VSP values were calculated using real-time speed and acceleration rates collected from the driving simulator tests. Combined with the calculated VSPs, the speeds, and acceleration rates in different operating mode bins, the OpMode IDs for this test were generated, and represent different emission rates for the studied air pollutants, namely, carbon monoxide (CO), carbon dioxide (CO2), nitrogen oxides (NOx), and hydrocarbons (HC). The emission rates used in this paper were assumed for a 5-year-old light duty gasoline passenger (2008 year), as shown in (Frey and Liu, Citation2013). The total vehicle emissions per trip were calculated using these emission rates.
Table 2. Default operating mode bin average emission rates (g/h) in the MOVES model for selected pollutants: passenger cars, gasoline, 5 years old (Frey and Liu, Citation2013).
Results and discussion
Stopping distance for safety
The DSAS is dedicated to enhance the safety on roadways, including in a work zone area. Subjects’ entering speeds to the work zone and stopping distance for a sudden crossing worker were measured. Based on the test results in a normal situation, without the DSAS, subjects’ driving speed decelerated from 72 ± 7.5 km/h to 62.3 ± 11.7 km/h, while passing the traffic sign “WORK ZONE AHEAD.” Then the speed further decreased to 55.5 ± 9.4 km/h by the traffic sign “RIGHT LANE CLOSED.” However, the speed limit in the work zone was 48 km/h (30 mph). When the DSAS was triggered, subjects’ driving speeds decreased from 72 ± 8.2 km/h to 52.8 ± 8.1 km/h, then to 50.7 ± 5.6 km/h by the aforementioned two traffic signs, which were very close to the speed limit (48 km/h). The DSAS was able to effectively instruct subjects to obey traffic regulations, which is a basic condition of defensive driving on roadways.
Further, the DSAS may improve subjects’ safety awareness on a hazardous situation to avoid a fatal crash. In a normal situation without the DSAS, 29% of the subjects did not stop for the sudden crossing of the worker. A fatal crash might take place, if the driving test was conducted on a real road. Subjects’ headway distances gradually decreased from 22 m, and then dropped to about 17 m. Though 71% of them stopped for the worker, their average stopping distance to the worker was within 14.1 ± 8.2 m. On the contrary, when the DSAS was applied, their stopping distance was extended to 28 ± 11.2 m, and all subjects stopped for the worker smoothly. In the forward collision area, subjects’ headway distances were kept more than 25 m from the leading vehicle, when the DSAS was provided, which was considerably longer than the distance in a normal situation by an average of 6 m. Thus, the proposed DSAS was able to improve drivers’ awareness of dynamic traffic situation to drive slowly and stop earlier for an obstacle.
Total emissions
There is a concern that the lower driving speeds affected by the DSAS may result in a longer duration and eventually lead to higher total emissions in a work zone. , , and list the total emissions and their relevant standard deviations in the advance area, in the activity area, and in the forward collision warning area of the work zone, respectively. In all the three cases, the total emissions of CO2, NOx, CO, and HC for normal situations (LW–D, LA–D, and FF–D) were higher than those in the situations with the DSAS (LW+D, LA+D, and FF+D) for the four air pollutants. In particular, the total emissions of CO in LW–D, LA–D, and FF–D were much higher than in those in LW+D, LA+D, and FF+D, respectively. In other words, the DSAS was able to cause a reduction in CO emission in the three studies areas.
Figure 2. (a) Total emissions recorded in the advance warning area (LW). (b) Total emissions recorded in the activity area (LA). (c) Total emissions recorded in the forward collision warning area (FF).
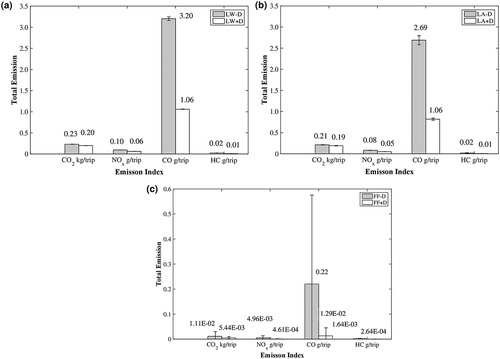
Further, the standard deviations were very small, except the CO emissions in FF–D. The higher variability of the incomplete combustion gas CO in FF–D may be caused by rapid deceleration and acceleration in stop-and-go activities. Using the DSAS, the stop-and-go activity could be avoided in the forward collision area.
In , the total emissions in the forward collision warning area (FF) were tremendously lower than the total emissions in LW and LA areas in and . This can be explained by the enormous difference in the duration of driving through study areas. An average of 3 and 5 s was needed to drive through the forward collision warning area without and with the DSAS, respectively, whereas the duration for the other two tests (LW and LA) were up to 54 s (on average 34 s for LW–D, 36 s for LW+D, 39 s for LA–D, and 54 s for LA+D).
More specifically, the total emissions of the four pollutants produced per trip are listed in . In , CO2 is the predominant vehicle emission and accounts for more than 97% of the total emissions per trip. CO emissions rank second, ranging from 0.24% for FF+D to 1.96% for FF–D, followed by NOx and HC.
Table 3. Total emissions estimated in the six scenarios.
A higher percentage of CO2 emissions is expected. There are slight differences in CO2 emissions found between with and without the DSAS, approximately 30 g/trip in LW, 20 g/trip in LA, and 5.6 g/trip in FF.
Without the DSAS, the CO emitted was notably higher than in the normal situation, which may be ascribable to high frequency of a stop-and-go activity and excessive acceleration. Nevertheless, the CO emissions remained extremely low for the scenarios with DSAS. With respect to the reduction in CO emission, the DSAS could promote fuel combustion efficiency.
The significant difference in the individual total vehicle emissions per trip caused by the DSAS was examined using a statistical t-test as listed in . In , there are significant differences in the total NOx, CO, and HC emissions in the three study areas, whereas the significant effect is not found in the activity area (LA) for the total CO2 emission.
Table 4. Significant test results for the four studied vehicle emissions.
Emission rates under each OpMode ID
The operating mode bins in MOVES represent various fleet average emission rates based on individual vehicle speed profile. To obtain an insight into the characteristics of vehicle emissions, which resulted from the application of the DSAS, the estimated emission rates represented by OpMode ID distribution in the LW and LA were investigated and are shown in .
Figure 3. (a) Emission rates of CO2 for scenario LW. (b) Emission rates of NOx for scenario LW. (c) Emission rates of CO for scenario LW. (d) Emission rates of HC for scenario LW. (e) Emission rates of CO2 for scenario LA. (f) Emission rates of NOx for scenario LA. (g) Emission rates of CO for scenario LA. (h) Emission rates of HC for scenario LA.
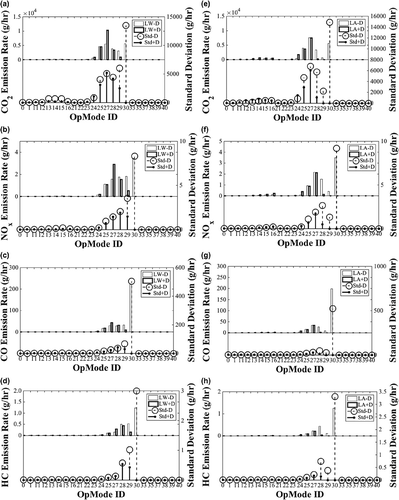
shows that there was little difference in emission rates in the LW and LA area for the two scenarios (–D and +D), but the common ground was that few emission rates were observed before OpMode ID 24, a distribution that represents the cruise mode or moderate speed with the VSP less than 6 kW/ton. In this driving simulator test, objective conditions in the test were the same, but subjects’ individual acceleration rates and speeds varied from others, which means lower emission rates may be credited to steady acceleration and lower driving speed.
In the normal situation (LW–D and LA–D), the OpMode IDs mainly distributed between 24 and 30, which represents the VSP range from 6 to 30 kW/ton and the speeds from 40 to 80 km/h (25 to 50 mph), respectively. When the DSAS was applied (LW+D and LA+D), their distribution became narrower, concentrating in the OpMode bins from 24 to 28. states that between the OpMode bins 24 and 30, the VSP increases along with the increase in OpMode bins in the speed range between 40 and 80 km/h. As subjects drove within a steady speed range, the decrease in acceleration rates may be a major factor, which led to the narrower distribution, switching from 24–30 to 24–28. In other words, the DSAS was able to affect subjects’ acceleration rates and resulted in lower emission rates.
Furthermore, the higher emission rates are shown in the OpMode ID 30 and 27 for LW–D and LA–D, and the ID 27 for LW+D and LA+D. Looking up the average emission rates for default OpMode Bins in the MOVES model (), the emission rates for the ID 30 is tremendously higher than those for the ID 27. It is striking that the emission rates of CO and HC for the ID 30 are more than 18 times and 19 times higher than for the ID 27, respectively. Meanwhile, in LW–D and LA–D, the emission rates categorized to ID 30 for the four air pollutants were substantially higher than those to other IDs. Thus, the incomplete combustion rates represented by the HC and CO emission in the normal situation were considerably higher than in the situation with the DSAS.
Also, illustrates that the variability of the emission rates in LW–D and LA–D was higher than in LW+D and LA+D, as a whole. In particular, without the DSAS, the variability of the emission rates represented by ID 30 was enormously higher. The ID 30 falls into the speed range of 40.2 km/h to 80.4 km/h and signifies the highest VSP in this range. In this range, higher acceleration rates may be responsible for the higher VSPs, leading to higher emission rates. Therefore, the noticeable higher emission rates were due to excessive acceleration rates in the situation without the DSAS.
On the other hand, in crowded traffic situations like a bottleneck caused by a work zone, vehicles often need to brake hard or rapidly for some unexpected situations, such as the front vehicle suddenly stopping, then re-accelerate to resume the trip. The specific OpMode ID distributions are shown in .
Figure 4. (a) Emission rates of CO2 for scenario FF. (b) Emission rates of NOx for scenario FF. (c) Emission rates of CO for scenario FF. (d) Emission rates of HC for scenario FF.
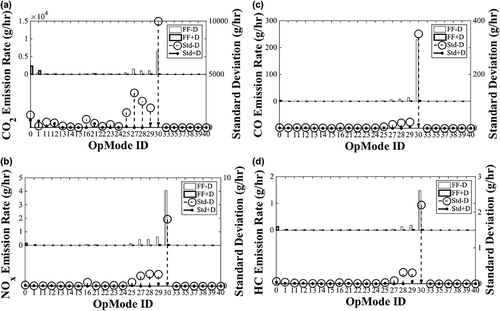
shows that the OpMode IDs were concentrated in the range from 27 to 30 for the four air pollutants in the normal situation (FF–D), whereas in the situation with the DSAS (FF+D) the distributions of the OpMode IDs moved to the bins from 0 to 1 represented the mode of idling and braking with lower VSP. Meanwhile, the standard deviations for FF–D were drastically higher than those in the DSAS scenario (FF+D). These distributions further demonstrate that the DSAS was able to instruct subjects to react to an unexpected situation properly, without excessive acceleration that is categorized in the higher emission zones.
CO2 versus NOx diagram
The relationships between the complete combustion gas CO2 and NOx emitted in the tests without and without the DSAS are shown in .
– illustrates that the emission rate of CO2 rises with the increase in the emission rate of NOx in moles per hour for the three cases, in which the slopes of trend lines without the DSAS (LA–D, LW–D, and FF–D) are greater than those with the DSAS (LA+D, LW+D, and FF+D). Producing the same amount of CO2 with the DSAS would be accompanied by lower NOx than without the DSAS. As NOx is the primary contributor in the formation of smog, the employment of the DSAS would possibly be associated with a positive impact on public health.
Conclusions
In this paper, the impact of DSAS on vehicle emissions, which resulted from the associated changes in driving behaviors, was investigated. Two driving simulator tests were conducted to examine the effect of the DSAS for leading vehicles in an advance warning area, activity area, and for the following vehicles in the forward collision warning area of a work zone. Subjects’ stopping distance, headway distance, vehicle total emissions, and the OpMode ID distribution for each vehicle air emission were studied. The emission model MOVES was used to calculate the vehicle emissions.
Results show that the DSAS was able to enhance the safety in work zones by improving drivers’ driving behaviors in terms of longer headway distance and stopping earlier for a sudden worker’s crossing. These changes in driving behaviors induced the switch of the OpMOde IDs distribution from higher to lower emission zones, thereby resulting in considerable emission reduction in the three studied areas. Furthermore, the DSAS was capable of reducing NOx emission per mole CO2 produced, and thus would potentially mitigate the adverse health impacts of traffic operations.
Funding
The authors acknowledge that this research is supported in part by the U.S. Tier 1 University Transportation Center TranLIVE number DTRT12GUTC17/ KLK900-SB-003, and by the National Science Foundation (NSF) under grant 1137732. The opinions, findings, and conclusions or recommendations expressed in this material are those of the authors and do not necessarily reflect the views of the funding agencies.
Additional information
Funding
Notes on contributors
Qing Li
Qing Li is a Ph.D. candidate in environmental toxicology at Texas Southern University (TSU).
Fengxiang Qiao
Fengxiang Qiao is an associate professor and co-director of the Innovative Transportation Research Institute (ITRI) at Texas Southern University (TSU).
Lei Yu
Lei Yu is a professor in the Department of Transportation and Dean of the College of Science, Engineering, and Technology at Texas Southern University (TSU).
References
- Ba, Y., W. Zhang, G. Salvendy, A.S.K. Cheng, and P. Ventsislavova. 2015. Assessments of risky driving: A go/no-go simulator driving task to evaluate risky decision-making and associated behavioral patterns. J. Appl. Ergonom. 52:265–274. doi:10.1016/j.apergo.2015.07.020
- Carrera, H.E., J. Portillo, G. Mejia, and A. Mendoza. 2015. Emissions of light-duty vehicles with respect to cruising speed under real-world driving conditions. J. Environ. Eng. 141:Paper # 04015004. doi:10.1061/(ASCE)EE.1943-7870.0000935
- Environmental Protection Agency. 1994. Automobile emission: An over view. EPA 400-F-92-007. http://www3.epa.gov/otaq/consumer/05-autos.txt (accessed November 8, 2015).
- Frey, H.C., and B. Liu. 2013. Development and evaluation of a simplified version of MOVES for coupling with a traffic simulation model. Transportation Research Board 91st Annual Meeting Compendium of Papers paper 13–1201.
- Healthline. 2013. The dangers of smog: What you need to know about air pollution. http://www.healthline.com/health/dangers-smog-what-you-need-know-about-air-pollution#2. (accessed November 4, 2015).
- Hill, K., F. Qiao, M. Azimi, and L. Yu. 2014. Impacts of restaurant drive-through configurations on vehicle emissions. Transportation Research Board 93rd Annual Meeting Compendium of Papers paper 14–4659.
- Hoover, C., L. Yu, F. Qiao, and M. Azimi. 2015. Emission implications of alternative origin–destination routes: Case study in Houston, Texas. Proc. Transportation Research Board 94th Annual Meeting, January 11 to 15, 2015, Washington, DC.
- James, W.S.L. 1989. Acid deposition. Origins, impacts and abatement strategies. Published jointly by the British Library and Technical Communication. Berlin Heidelberg: Springer-Verlag. ISBN-13:978-3642-76475-2, e-ISBN-13:978-3-642-76473-8, 978-3-642-76475-2. doi:10.1007/978-3-642-76473-8
- Jimenez-Palacios, J.L. 1999. Understanding and quantifying motor vehicle emissions with vehicle specific power and TILDAS remote sensing. Ph.D. dissertation, Massachusetts Institute of Technology, Cambridge, MA.
- Lai, J., L. Yu, G. Song, P. Guo, and X. Chen. 2013. Development of city-specific driving cycles for transit buses based on VSP distributions: Case of Beijing. J. Transport. Eng. 139(7):749–57. doi:10.1061/(ASCE)TE.1943-5436.0000547
- Li, Q., and F. Qiao. 2014. How Drivers’ Smart Advisory System Improves Driving Performance: A Simulator Imitation of Wireless Warning on Traffic Signal Under Sun Glare. Saarbrucken, Germany: Lambert Academic Publishing.
- Li, Q., F. Qiao, and L. Yu. 2015a. Will vehicle and roadside communications reduce emitted air pollution? Int. J. Sci. Technol. 5(1).
- Li, Q., F. Qiao, X. Wang, and L. Yu. 2015b. Measures of performance when drivers approaching STOP sign intersections with the presence of drivers smart advisory system. Proc. 28th Annual Conference of the International Chinese Transportation Professionals Association (ICTPA), May 14–16, 2015, Los Angeles, CA.
- Pease, K. 2009. Vehicle Range Finder. U.S. Patent US20100138142 A1, US 12/504,810. New World Encyclopedia. Combustion. http://www.newworldencyclopedia.org/entry/combustion (accessed April 3, 2015).
- Qiao, F., J. Jia, L. Yu, Q. Li, and D. Zhai. 2014. Drivers’ smart assistance system based on radio frequency identification: Enhanced safety and reduced emissions in work zones. Transport. Res. Rec. 2458:37–46.
- Roess, R.P., E.S. Prassas, and W.R. McShane. 2010. Traffic Engineering, 4th ed. India: Dorling Kindersley (India) Pvt. Ltd., Licensees of Pearson Education in South Asia.
- Schmidt, G.A., R. Ruedy, R.L. Miller, and A.A. Lacis. 2010. The attribution of the present-day total greenhouse effect (PDF). J. Geophys. Res. 115. doi:10.1029/2010JD014287, issue D20, http://onlinelibrary.wiley.com/doi/10.1029/2010JD014287/abstract. (accessed January 25, 2016).
- U.S. Census Bureau. n.d. http://www.census.gov/2010census/data (accessed April 3, 2015).
- U.S. Department of Transportation. 2006. http://climate.dot.gov/about/transportations-role/overview.html (accessed November 4, 2015).
- U.S. Department of Transportation. 2009. Coordinating, planning and managing the effects of roadway construction with technology. http://www.its.dot.gov/press/2009/WorkZone_QRv5%205.pdf (accessed November 10, 2015).
- U.S. Department of Transportation. 2015a. http://www.its.dot.gov/aeris (accessed February 20, 2015).
- U.S. Department of Transportation. 2015b. Connected vehicle technology. Intelligent Transportation Systems Joint Program Office. http://www.its.dot.gov/connected_vehicle/connected_vehicle_tech.htm#sthash.BhDY0BT8.dpuf (accessed February 20, 2015).
- U.S. Environmental Protection Agency. 2011. Development of emission rates for light-duty vehicles in the Motor Vehicle Emissions Simulator (MOVES2010). Final Report EPA-420-R-11-011. http://www3.epa.gov/otaq/models/moves/documents/420r11011.pdf (accessed January 25, 2016).
- U.S. Environmental Protection Agency. 2012. Motor Vehicle Emission Simulator (MOVES), User Guide for MOVES 2010b. EPA-420-B-12-001b. http://www3.epa.gov/otaq/models/moves/documents/420b12001b.pdf (accessed January 25, 2016).
- U.S. Environmental Protection Agency. 2015a. Sources of greenhouse gas emission. http://www3.epa.gov/climatechange/ghgemissions/sources/transportation.html (accessed November 4, 2015).
- U.S. Environmental Protection Agency. 2015b. EPA releases MOVES2014a Mobile Source Emissions Model: Questions and answers. Office of Transportation and Air Quality. EPA-420-F-15-046 November. http://www3.epa.gov/otaq/models/moves/documents/420f15046.pdf (accessed January 25, 2016).
- Zhai, H., H. C. Frey, and N. M. Rouphail. 2008. A vehicle-specific power approach to speed- and facility-specific emissions estimates for diesel transit buses. Environ. Sci. Technol. 42,(21): 7985–91. doi:10.1021/es800208d
- Zhou, X., S. Tanvir, H. Lei, J. Taylor, B. Liu, N. Rouphail, and H.C. Frey. 2015. Integrating a simplified emission estimation model and mesoscopic dynamic traffic simulator to efficiently evaluate emission impacts of traffic management strategies. J. Transport. Res. Part D Transport Environ. 37:123–136. doi:10.1016/j.trd.2015.04.013