ABSTRACT
Mumbai, a highly populated city in India, has been selected for air quality mapping and assessment of health impact using monitored air quality data. Air quality monitoring networks in Mumbai are operated by National Environment Engineering Research Institute (NEERI), Maharashtra Pollution Control Board (MPCB), and Brihanmumbai Municipal Corporation (BMC). A monitoring station represents air quality at a particular location, while we need spatial variation for air quality management. Here, air quality monitored data of NEERI and BMC were spatially interpolated using various inbuilt interpolation techniques of ArcGIS. Inverse distance weighting (IDW), Kriging (spherical and Gaussian), and spline techniques have been applied for spatial interpolation for this study. The interpolated results of air pollutants sulfur dioxide (SO2), nitrogen dioxide (NO2) and suspended particulate matter (SPM) were compared with air quality data of MPCB in the same region. Comparison of results showed good agreement for predicted values using IDW and Kriging with observed data. Subsequently, health impact assessment of a ward was carried out based on total population of the ward and air quality monitored data within the ward. Finally, health cost within a ward was estimated on the basis of exposed population. This study helps to estimate the valuation of health damage due to air pollution.
Implications: Operating more air quality monitoring stations for measurement of air quality is highly resource intensive in terms of time and cost. The appropriate spatial interpolation techniques can be used to estimate concentration where air quality monitoring stations are not available. Further, health impact assessment for the population of the city and estimation of economic cost of health damage due to ambient air quality can help to make rational control strategies for environmental management. The total health cost for Mumbai city for the year 2012, with a population of 12.4 million, was estimated as USD8000 million.
Introduction
The rapid growth of cities like Mumbai and Delhi, India, in the 21st century can be attributed largely to rural–urban migration (Patel and Burkle, Citation2012). Urbanization or population growth has large negative impact on quality of air, water, and soil (Huff and Angeles, Citation2011; Duh et al., Citation2008; Defries and Pandey, Citation2010; Cassidy et al., Citation2014). Air pollution is one of the most serious problems in the world. Regulatory and research institutes have installed air quality monitoring networks in urban regions to assess deteriorating air quality levels. Air quality shows very high temporal and spatial variations (Gerdol et al., Citation2014). Monitoring concentrations at several locations in a study area is usually resource intensive, difficult or expensive. Monitoring represents air pollution level for a particular point. Thus, spatial interpolation techniques are used to create a surface grid or contour map. Interpolation processes estimate concentrations in the study area using known concentrations at fewer points. A geographic information system (GIS) is a software tool to store, analyze, manage, and present all types of spatial or geographical data, which, along with the expert judgment of the GIS user or analyst, produces solutions to spatial problems. GIS techniques have been applied by various researchers primarily to analyze the spatial and temporal distribution of pollutants (Maantay, Citation2007; Sohrabinia and Khorshiddoust, Citation2007; van Westen, Citation2004; Jensen et al., Citation2001; Marquez and Smith, Citation1999). Health impact assessment due to air pollution has been done for many regions (Pope and Dockery, Citation2006; Krewski and Rainham, Citation2007; Lai et al., Citation2012). Whitworth et al. (Citation2011) and Kim et al. (Citation2014) have carried out ordinary Kriging spatial interpolation with monitored air quality data. Wong et al. (Citation2004) have carried out a study of comparing various spatial interpolation methods like spatial averaging, nearest neighbor, inverse distance weighting (IDW), and Kriging to estimate air pollution concentration. However, they did not include spline and Kriging techniques with spherical and Gaussian semi-variograms in their study. Also, the spatial interpolation techniques have not been used for mapping of air quality in Mumbai city before this. Even epidemiological studies have been conducted for exposure assessment due to air pollution by Ryan and Lemasters (Citation2008) but air quality mapping was not included. Furthermore, the economic cost of health impact assessment was not estimated due to the prevalence of different concentrations of air pollutants.
Various researchers have used air quality models for mapping of air quality and assessing the health impacts. In most of the cases it has been observed that interpolated concentrations by air quality dispersion models underestimate particulate matter (PM) concentrations (Mohan et al., Citation2011; Kumar et al., Citation2015; Rood, Citation2014). Air quality modeling requires emission data for all known sources of air pollution. However, some unidentified sources are missed, especially area sources such as emission of secondary aerosol, resuspended dust, and so on. Dispersion models are also known to give high uncertainty for the prediction of ground-level concentrations due to multiple assumptions as well as input data quality of the model. In addition to this, onsite hourly meteorological data for various parameters are required for modeling and are not available in all the cases. Hence, observed air quality has been used for this study.
A series of control measures with sustained efforts was undertaken by authorities due to public pressure. As a result, improvement in air quality was witnessed for 1991–2003 (Gupta and Kumar, Citation2006). In this period, many air polluting industries moved out of the city, better technology was adopted in vehicles, and the use of low emission fuel was imposed on vehicles. However, it has been observed that the air quality of Mumbai city is once again deteriorating (National Environment Engineering Research Institute [NEERI], Citation2004–2013). The National Environment Engineering Research Institute (NEERI) has reported that the levels of suspended particulate matter (SPM) and PM10 are much higher than the standards provided by the Central Pollution Control Board (CPCB). The standards for SPM and PM10 are 140 and 60 µg/m3, respectively, for residential area. n investigation of air pollution in megacities by Mage et al. (Citation1996) also showed that the major problem affecting these cities is their high levels of total suspended particles (TSP). It is well established that high levels of particulate matter (PM) are significantly associated with adverse health effects and ecosystem damage (Kristiansson et al., Citation2015; Srivastava and Kumar, Citation2002; Zhou et al., Citation2014; Chhabra et al., Citation2010; Kampa and Castanas, Citation2008).
In this study, the data for seasonal and annual variation of air quality are studied and several interpolation techniques are applied over the study region. The interpolated values of concentration are compared with observed data to evaluate the interpolation technique. Further, air quality data within a ward (an administrative subunit of city) are used to estimate the economic cost of morbidity due to air pollution based on total population of the ward.
Study area
Mumbai is the capital of the state of Maharashtra in India and is also the entertainment capital of the country. With 12.7 million people (Census of India Organisation, Citation2011), this is the most densely populated city in India and the fifth most populated city in the world. Mumbai city has an area of 438 km2 and is divided into 24 wards for the purpose of administration. shows a ward map of the city (Chakraborty et al., Citation2015). As some wards are large in terms of area and population, it has been subdivided into North, South, Central, and so on. Labels such as A, B, and C are administrative ward names and K/E, K/W, F/N, F/S, and RC+N are ward name with Eastern, Western, Northern, Southern, and Central.
The total number of vehicles in the city is now 2.33 million, a 55% increase in 7 years. Mumbai is one of the largest centers of commerce in terms of financial flow, generating 6.16% of India’s gross domestic product (GDP) and accounting for 25% of industrial output, 70% of maritime trade in India, and 70% of capital transactions to India’s economy. Mumbai city is now one of the most polluted cities in India (https://en.wikipedia.org/wiki/Mumbai, accessed November 10, 2015).
Methodology
One of the objectives of this work was to estimate temporal and spatial variation of air pollution over the region. Air pollution concentration data on SO2, NO2, and SPM were collected from an air quality monitoring network system. These monitoring stations are operated continuously by Brihanmumbai Municipal Corporation (BMC), NEERI and Maharashtra Pollution Control Board (MPCB) in Mumbai city. BMC monitors air quality data at seven locations (Worli, Khar, Andheri, Bhandup, Borivali, Maravali, and Deonar), NEERI monitors at three locations (Parel, Kalbadevi, and Worli), and MPCB monitors at two locations (Bandra and Sion) in the city. Bandra and Sion are 3.5 km from each other. Air quality sampling was done at 3 to 8 m height on all these locations throughout the year 2012. Maravali and Andheri represent traffic sites, Khar, Bhandup, and Worli_BMC represent both traffic and residential sites and Kalbadevi, Parel, Worli_NEERI, and Deonar represent residential and commercial sites. Many years back, the Borivali monitoring station was installed to represent the urban background but now it only represents a typical residential region. Location Worli (NEERI) is a residential area measurement and also its height of measurement is maximum (8 m). This location was compared with location Maravali, which provides traffic site measurements at 3 m height. We found 20, 21, and 24% ratios of residential and traffic site for SO2, NO2, and SPM, respectively. NEERI and BMC collect samples twice a week, while MPCB collects samples every day for 24 hr. The data from NEERI and BMC were used to interpolate and compare with the data from MPCB, as the temporal resolution of sample collection of MPCB is different from that of NEERI and BMC. Annual averages of air pollution data were calculated for all the locations. IDW, Kriging, and spline techniques were used. IDW has been used with power (p) value 2, the ordinary Kriging method has been used with spherical and Gaussian semi-variograms, and the regularized spline technique has been used with 0.1 weights. The interpolated concentrations were compared with the data from MPCB. The interpolated values that matched best with the observed concentrations were further used in cost estimation of health for each ward.
Ward population data for Mumbai city were used based on the census of 2011. Epidemiological studies of the relationship between concentration levels of pollutants and adverse health effects provide an estimate of the relationship of air quality changes to the relative risk of health effect. The number of cases of the health effect per year is then calculated by multiplying the incidence rate with the corresponding population of the ward. Finally, cost was estimated for health damage due to air pollution for the city. Economic valuations have been carried out by using the values from Patankar and Trivedi (Citation2011). However, the dynamic population exposure was not carried out in this study.
A geo-referenced shape file for Mumbai city was prepared to associate with physical earth space in ArcGIS. The shape file of Mumbai city includes 24 wards of BMC. The point shape files have also been created for air quality monitoring stations of Mumbai region. Air quality concentration data for SO2, NO2, and SPM were attributed to respective point shape file at seasonal and annual time scales. Annual average concentration data measured by BMC and NEERI were interpolated and the interpolated concentrations were compared with MPCB monitoring data. In order to assess health impact due to air pollution, annual average concentrations were used with concentration-response (CR) coefficients for respective pollutants and population of particular ward. Cost was estimated for the total exposed population for damaged health. The health impact assessment was carried out using the following equation:
Interpolation techniques of ArcGIS
There are many kinds of interpolation techniques inbuilt in GIS, and include IDW, Kriging, and spline (Colin, Citation2004). These techniques are mostly used in air pollution studies (Jha et al., Citation2011; Wong et al. Citation2004). When the set of points is dense enough to capture the extent of local surface variation needed for the analysis, IDW is used. It estimates grid values using a linear-weighted combination of a set of data and it is a function of two fields, namely, the distance between sampling sites and the site at which the interpolation has to be made (Gunnink and Burrough, Citation1996). Points will have higher weight if they are nearer to the point where SPM is to be interpolated. Weight is an inverse function of the distance according to the equation
Spline estimates values for unknown points using a mathematical function that minimizes the overall surface curvature (Greiner, Citation1991). This results in a smooth surface that passes exactly through the points. This method is a special type of piecewise polynomial interpolation and best for gently varying surfaces. It is often preferred with small interpolation error even when using low degree polynomials for the spline. It can predict ridges and valleys in the data and is the best method for representing smoothly varying surfaces of phenomenon.
Kriging is a powerful statistical method of interpolation for which the interpolated values are modeled by a Gaussian process governed by prior covariances (Bailey and Gatrell, Citation1995). It is a stochastic method that is used for diverse applications such as health science, geochemistry, and pollution modeling (Griffith, Citation1988). It assumes that the distance or direction between sample points reflects a spatial correlation that represents variation in surface. It produces better estimates to take explicit account of the effect of random noise. Also, it is less susceptible to use in arbitrary decision and indication of the estimates. It predicts the values for all locations within a specified radius as a function of specified number of points or all points. It uses a sophisticated weighted average technique to predict values from observed samples. There are many types of Kriging, such as ordinary, spherical, and Gaussian. The mathematical formulation is been given in eq 3:
Results and discussion
The variations of pollutants were seen on seasonal and annual basis. shows annual average concentrations for SO2, NO2, and SPM at 10 locations monitored by BMC and NEERI. The collected air quality data set was fed in an attribute of points shape files at monthly, seasonal, and annual temporal scales. SPM concentrations were always higher while SO2 and NO2 concentrations were less compared to the national ambient air quality standards (CPCB, Citation2009). The values of the standards of national ambient air quality are 60, 60, and 140 µg/m3 for SO2, NO2, and SPM, respectively. The maximum annual concentrations were 20 µg/m3, 67 µg/m3, and 683 µg/m3 at Maravali (in M ward) for SO2, NO2, and SPM, respectively. The minimum annual concentrations of SO2, NO2, and SPM were 4 µg/m3, 13 µg/m3, and 152 µg/m3 at Worli, Parel, and Kalbedevi, respectively. The annual average concentrations of monitored data were 10 µg/m3, 35 µg/m3, and 260 µg/m3 for SO2, NO2, and SPM, respectively. Also, spatial standard deviations calculated for annual averages of all monitoring locations were 4, 18, and 150 µg/m3 for SO2, NO2, and SPM, respectively.
Seasonal variations were also analyzed in this study. December, January, and February were considered the winter season; March, April, and May the premonsoon season; June, July, August, and September were the monsoon season; and the postmonsoon season comprised October and November. The maximum concentrations of all pollutants were found in the winter season, while the minimum concentrations were found in the monsoon season, which is as expected. The average concentration of each season was calculated for all locations. Then the average of all locations for each season was calculated for all pollutants. As expected, the winter season had a maximum concentration of 15.7, 52.3, and 379.3 µg/m3 for SO2, NO2, and SPM, respectively, as presented in . Low temperature and mixing height and greater calm conditions cause higher concentration levels in winter. The minimum concentrations are 7.5, 26.8, and 191.7 µg/m3 for SO2, NO2, and SPM in the monsoon season, respectively. The premonsoon season has lower concentration than the postmonsoon season for SO2 and NO2, while the premonsoon has higher concentration than the postmonsoon season for SPM. The standard deviations of seasonal averages were 4, 11, and 73 µg/m3 for SO2, NO2, and SPM. In both annual and seasonal averages, high spatial variation in SPM is observed, but it has less spatial variation in case of SO2 and NO2. SPM undergoes precipitation and scavenging and gives high variation in pollution levels.
Table 1. Seasonal average concentration and standard deviation in the seasons.
Further, GIS mapping was done in this study using spatial interpolation techniques. ArcGIS provides spatial analyst the extension tools for spatial data analysis (i.e., modeling suitability, distance). The interpolation study showed IDW, Kriging spherical, and Kriging Gaussian techniques gave better interpolation as compared to spline, as shown in to for SO2, NO2, and SPM, respectively. The interpolated values by Kriging spherical and Kriging Gaussian were very close to observed data, and they were equal at two locations for SO2 and at Sion for NO2. The percentage differences were calculated using the difference between interpolated and observed values, divided by observed values, and multiplied by 100. In SO2 interpolation, IDW and Kriging methods underestimated the values by 32% while the spline method overestimated them by 32% with observed data at the Bandra location. At Sion, IDW, Kriging, and spline methods overestimated by 24%, 18%, and 63%, respectively. In NO2 interpolation, IDW and Kriging underestimated by only 4% while the spline method overestimated by 41% at the Bandra location. At Sion, Spline has performed (35% underestimation) better than IDW and Kriging (around 46% underestimation). This might be due to uncertainty in meteorology, emissions, and a sparse monitoring network. In SPM interpolation, IDW and Kriging performed well for both locations of Bandra and Sion and it overestimated by 20% and 28%, respectively. The spline method has underestimated by 49% at Bandra and overestimated by 51% at Sion. In most of the cases, IDW and Kriging performed better than the spline method. Kriging performed best among all these interpolation techniques.
Figure 3. Comparison of interpolated SO2 concentration with observed concentration at two locations.
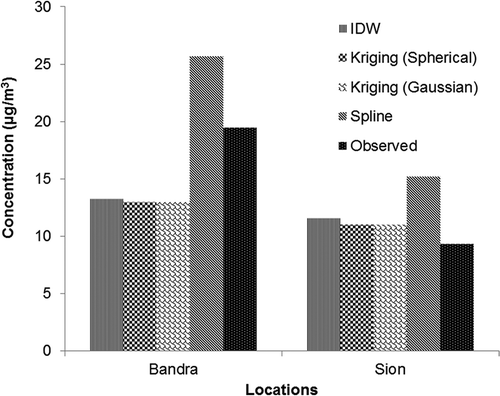
Health impact assessment was carried out using ward population, concentration data, and concentration-response coefficients. Population data of Census 2011 presented in were used in a health impact assessment for the year 2012. The maximum population is at location Maravali (M Ward) and P Ward. Concentration-response coefficients were taken from Patankar and Trivedi (Citation2011) for PM10 and are presented in . BMC monitors SPM at seven locations and MPCB monitors SPM and PM10 at two locations in the city. There were very low differences in ratios of SPM and PM10 at various locations. Thus, a factor of 0.59 was used to estimate PM10 concentrations from SPM concentrations based on available PM10 and SPM measurements (MPCB, Citation2012). Two health impacts (cardiac ailments and other chest illness) of SO2 pollutants have been considered that were not caused by NO2 and PM10. The upper respiratory health impacts of pollutants NO2 and PM10 have been considered, which covers morbidity like cough, breathlessness, wheezing, cold, allergic rhinitis, and chronic obstructive pulmonary disease (COPD). Equal health impacts are shown for allergic rhinitis and COPD by PM10.
Table 2. Concentration-response coefficients of pollutants for health impact.
Figure 4. Comparison of interpolated NO2 concentration with observed concentration at two locations.
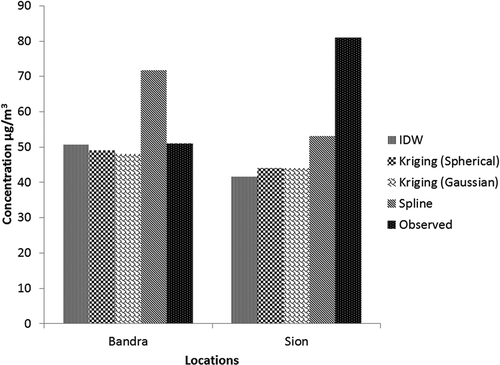
Figure 5. Comparison of interpolated SPM concentration with observed concentration at two locations.
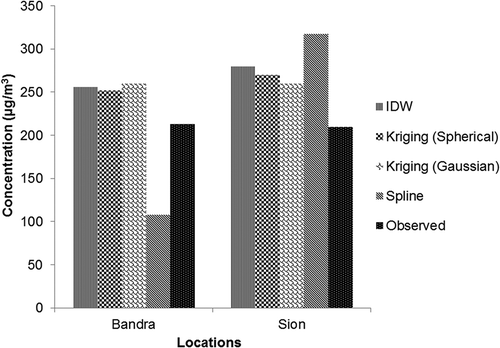
Baseline concentrations for SO2, NO2, and PM10 were taken from the output of a hemispheric model that gives background concentration in the urban or local region. It was simulated for the year 2012 at 150 km × 150 km resolution using the Danish Eulerian Hemisphere Model (DEHM) developed in Aarhus University, Aarhus, Denmark. It is a three-dimensional, offline, large-scale, Eulerian, atmospheric chemistry transport model developed to study long-range transport of air pollution in the Northern Hemisphere. The model domain used in previous studies covers most of the Northern Hemisphere, discretized on a polar stereographic projection, and includes a two-way nesting procedure with several nests with higher resolution over Europe, Northern Europe, and Denmark (Frohn et al., Citation2002). Several emission inventories such as the Emission Database for Global Atmospheric Research (EDGAR) Fast track, Global Emission Inventory Activity (GEIA), and Representative Concentration Pathways (RCP4.5) were used in this study. The emissions inventories are combined for the year 2012 so that all the relevant species are available for the year studied. More details of this model and emission inventories are given in Brandt et al. (Citation2012). The model has also been applied in many studies of air quality for different perspectives like international transportation of air pollutant and health impact and their cost evaluation (Hedegaard, Citation2012; Brandt et al., Citation2012, Citation2013a, Citation2013b; Geels et al., Citation2012a, Citation2012b; Geels et al., 2004).
The annual averages of background concentration of SO2, NO2, and PM10 were 2, 2, and 17 µg/m3, respectively, for the Mumbai region. SO2 and NO2 are mainly from anthropogenic emission so the baseline concentration (2 µg/m3) is taken to be the same as the background concentration of DEHM. Shah et al. (Citation1997) estimated baseline concentration for PM10 to be 41 µg/m3 for health impact assessment for Mumbai city under urban air quality management strategy in Asia. The value of 41 µg/m3 was also taken as baseline for health impact assessment for Mumbai city by Joseph et al. (Citation2003). DEHM estimated 17 µg/m3 as background concentration for PM10, and DEHM includes sea salt but presently it does not include resuspended dust and wind-blown dust from crustal sources. Globally, anthropogenic emission contributes to 25% of all dust emissions (Ginoux et al., Citation2012). The contribution of crustal source was reported as 25% of the total PM10 concentration by Pandit and Puranik (Citation2008). Hence, the baseline concentration was taken as 40 µg/m3.
shows annual baseline and measured concentrations of SO2, NO2, and PM10 at various locations of Mumbai city. The difference between baseline concentration and actual pollution level can be seen in .
, , and show health impact due to the air pollutants SO2, NO2, and PM10 at various locations. The locations Andheri, Deonar, and Maravali are highly affected by air pollution. Maximum population is exposed at Deonar, Andheri, and Maravali. The lowest population exposed for all the air pollutants is at Parel, Worli, and Kalbadevi.
Andheri, Maravali, Bhandup, and Deonar have maximum exposure due to pollutant PM10 as shown in . Chronic obstructive pulmonary disease (COPD) and allergic rhinitis have equal impact on population at each location of Mumbai city due to PM10 because it has the same concentration-response coefficient factor. shows that the minimum and maximum population is susceptible to cold and allergic rhinitis, respectively, due to NO2. Exposure to SO2 causes lower respiratory diseases like cardiac ailments and other chest illness. The population affected by other chest illnesses is higher than for cardiac ailments at all locations, as shown in . The minimum and maximum population is exposed at Kalbadevi and Deonar, respectively, for SO2.
Further, the cost of health impact was estimated using available costs for diseases. The average cost was taken for each disease and it was assumed that 25% of city residents use public health facilities and have a daily wage of approximately USD3.14 (INR200). Also, 20% of the population chooses to go to hospitals for breathlessness and wheezing and 50% of the population with COPD is hospitalized. The details are given in Patankar and Trivedi (Citation2011). Due to air pollution, among the various diseases, the cost for treatment for COPD and breathlessness is maximum because it has maximum exposure, as shown in and . Since both the ambient pollution level and the population density are higher at Andheri and Maravali, the total health cost at these sites is also high.
The total cost on health impact due to NO2 and PM10 was estimated at all locations as shown in . The costs of diseases caused by SO2 pollutant such as other chest illnesses and cardiac disease were not available in the literature. Since the SO2 levels were also low, SO2 was not included in this study. shows the cost of health estimated by spatial interpolation. It is observed that Andheri and Maravali (M Ward) incurred the maximum cost to health impact. Cost of health effect due to both PM10 and NO2 was maximum at M ward (Maravali). Total cost of health effect for NO2 and PM10 was estimated as USD8000 million (509 billion rupees) for Mumbai city.
Table 3. Total health impact and cost of health impact for the disease and pollutants.
Conclusion
Air quality monitoring is carried out to find out the status of air pollution levels of the city. BMC, NEERI, and MPCB monitor air quality at various locations of Mumbai city for SO2, NO2, and SPM. In this study, various spatial interpolations of ArcGIS, such as IDW, Kriging (spherical and Gaussian), and spline, were applied for spatial mapping over the study region. Most of the time, the performance of IDW and Kriging technique was found to be better than the spline technique when compared with observed concentrations. Interpolations with Kriging spherical and Gaussian were close to each other. Kriging performed best among all interpolation techniques.
Health benefit estimates are being looked at as an integral method of policy intervention for air pollution reduction leading to improved health. The results indicate enormous health benefits if air pollution levels, particularly of PM10, are brought down to the background level. Health impact was assessed based on air quality change from baseline concentration, population of the location, and concentration-response coefficients. Exposed population was estimated for all diseases and their cost was estimated. Maximum population is exposed at Deonar, Andheri, and Maravali for SO2, NO2, and PM10, respectively. Parel and Kalbedevi have low exposed population for all air pollutants. Andheri, Maravali, Bhandup, and Deonar have maximum exposure due to pollutant PM10. COPD and allergic rhinitis have equal impact on population at each location of Mumbai city due to PM10. However, the dynamic population exposure was not carried out in this study. The total health cost was estimated as USD8000 million (509 billion rupees) due to NO2 and PM10 pollution in Mumbai city for the year 2012, which is 4.24% of Maharashtra state GDP (Directorate of Economics and Statistics [DES], Citation2013).
Funding
The authors thank the China Section of the Air & Waste Management Association for the generous scholarship they received to cover the cost of page charges and to make the publication of this paper possible.
Supplemental Material
Download Zip (1.2 MB)Acknowledgments
The authors express their sincere thanks to Maharashtra Pollution Control Board, Air Quality Monitoring and Research Laboratory of Brihanmumbai Municipal Corporation for providing relevant data for this study.
Additional information
Notes on contributors
Awkash Kumar
Awkash Kumar, PhD, is a Scholar at IIT Bombay.
Indrani Gupta
Indrani Gupta is a Senior Scientist at CSIR-NEERI.
Jørgen Brandt
Jørgen Brandt is Senior Scientist at Aarhus University.
Rakesh Kumar
Rakesh Kumar is Chief Scientist and Head at CSIR-NEERI.
Anil Kumar Dikshit
Anil Kumar Dikshit is a Professor at IIT Bombay.
Rashmi S. Patil
Rashmi S. Patil is Emeritus Fellow at IIT Bombay.
References
- Bailey T.C., and A.C. Gatrell. 1995. Interactive Spatial Data Analysis. London, UK: Lomgman.
- Hedegaard, G.B. 2012. Effects of changed climate conditions on tropospheric ozone over three centuries. Atmos. Clim. Sci. 2(4): 546–61. doi:10.4236/acs.2012.24050
- Brandt, J., J.D. Silver, J.H. Christensen, M.S. Andersen, J. H. Bønløkke, T. Sigsgaard, C. Geels, et al. 2013a. Assessment of past, present and future health-cost externalities of air pollution in Europe and the contribution from international ship traffic using the EVA model system. Atmos. Chem. Phys. 13(15): 7747–64. doi:10.5194/acp-13-7747-2013
- Brandt, J., J.D. Silver, J.H. Christensen, M.S. Andersen, J.H. Bønløkke, T. Sigsgaard, C. Geels, et al. 2013b. Contribution from the ten major emission sectors in Europe and Denmark to the health-cost externalities of air pollution using the EVA model system—An integrated modelling approach. Atmos. Chem. Phys. 13(15): 7725–46. doi:10.5194/acp-13-7725-2013
- Brandt, J., J.D. Silver, L.M. Frohn, C. Geels, A. Gross, A.B. Hansen, K.M. Hansen, et al. 2012. An integrated model study for Europe and North America using the Danish Eulerian hemispheric model with focus on intercontinental transport of air pollution. Atmos. Environ. 53(June): 156–76. doi:10.1016/j.atmosenv.2012.01.011
- Cassidy, T., G. Inglis, C. Wiysonge, and R. Matzopoulos. 2014. A systematic review of the effects of poverty deconcentration and urban upgrading on youth violence. Health Place 26:78–87.doi:10.1016/j.healthplace.2013.12.009
- Census of India Organisation. 2011. Census, 2011. Census of India Organisation, Ministry of Home Affairs, Government of India. http://www.censusindia.gov.in
- Central Pollution Control Board. 2009. National Ambient Air Quality Monitoring 2008. Series: Naaqms//2009-10. http://cpcb.nic.in/upload/NewItems/NewItem_147_report-2008.pdf
- Chakraborty, A., B. Wilson, and S. Kashem. 2015. The pitfalls of regional delineations in land use modeling: Implications for Mumbai region and its planners. Cities 45:91–103. doi:10.1016/j.cities.2015.03.008.
- Chhabra, S.K., P. Chhabra, S. Rajpal, and R.K. Gupta. 2010. Ambient air pollution and chronic respiratory morbidity in Delhi. Arch. Environ. Health 56(1): 58–64. doi:10.1080/00039890109604055
- Colin, C., 2004. Interpolating surfaces in ArcGIS Spatial Analyst. ArcUser, ESRI Education Services. www.esri.com
- Duh, J.-D., V. Shandas, H. Chang, and L.A. George. 2008. Rates of urbanisation and the resiliency of air and water quality. Sci. Total Environ. 400(1–3): 238–56. doi:10.1016/j.scitotenv.2008.05.002
- DeFries, R., and D. Pandey. 2010. Urbanization, the energy ladder and forest transitions in India’s emerging economy: Trade-offs and synergies for ecosystem services and human well-being. Land Use Policy. 27:130–38.doi:10.1016/j.landusepol.2009.07.003
- Directorate of Economics and Statistics. 2013. Economic survey of Maharashtra 2012–13. Directorate of Economics and Statistics, Planning Development, Government of Maharashtra, Mumbai, India.
- Frohn, L.M., J.H. Christensen, and J. Brandt. 2002. Development of a high-resolution nested air pollution model. J. Comput. Phys. 179:68–94. doi:10.1006/jcph.2002.7036
- Geels, C., H.V. Andersen, C. Ambelas Skjøth, J.H. Christensen, T. Ellermann, P. Løfstrøm, S. Gyldenkærne, et al. 2012a. Improved modelling of atmospheric ammonia over Denmark using the coupled modelling system DAMOS. Biogeosciences 9(7): 2625–47. doi:10.5194/bg-9-2625-2012
- Geels, C., K.M. Hansen, J.H. Christensen, C. Ambelas Skjøth, T. Ellermann, G.B. Hedegaard, O. Hertel, L.M. Frohn, A. Gross, and J. Brandt. 2012b. Projected change in atmospheric nitrogen deposition to the Baltic Sea towards 2020. Atmos. Chem. Phys. 12(5): 2615–29. doi:10.5194/acp-12-2615-2012
- Gerdol, R., R. Marchesini, P. Iacumin, and L. Brancaleoni. 2014. Chemosphere monitoring temporal trends of air pollution in an urban area using mosses and lichens as biomonitors. Chemosphere 108:388–95. doi:10.1016/j.chemosphere.2014.02.035
- Ginoux, P., J.M. Prospero, T.E. Gill, N.C. Hsu, and M. Zhao 2012. Global-scale attribution of anthropogenic and natural dust sources and their emission rates based on MODIS Deep Blue aerosol products. Rev. Geophys. 50:RG3005, doi:10.1029/2012RG000388
- Greiner, H. 1991. A survey on univariate data interpolation and approximation by splines of given shape. Math. Comput. Model. 15(10): 97–108.doi:10.1016/0895-7177(91)90094-N
- Griffith, D.A. 1988. Advanced Spatial Statistics. Dordrecht, The Netherlands: Kluwer Academic.
- Gunnink, J.L., and P.A. Burrough. 1996. Interactive spatial analysis of soil attribute patterns using exploratory data analysis (EDA) and GIS. In Spatial Analytical Perspectives on GIS, ed. I. Masser and F. Salge, 87–99. New York, NY: Taylor & Francis.
- Gupta, I., and R. Kumar. 2006. Trends of particulate matter in four cities in India. Atmos. Environ. 40:2552–66. doi:10.1016/j.atmosenv.2005.12.021
- Huff, G., and L. Angeles. 2011. Globalization, industrialization and urbanization in pre-World War II Southeast Asia. Explor. Econ. Hist. 48(1): 20–36. doi:10.1016/j.eeh.2010.08.001
- Jensen, S.S., R. Berkowicz, H. Sten Hansen, and O. Hertel. 2001. A Danish decision-support GIS tool for management of urban air quality and human exposures. Transp. Res. Part D Transp. Environ. 6(4): 229–41. doi:10.1016/S1361-9209(00)00026-2
- Jha, D.K., M. Sabesan, A. Das, N.V. Vinithkumar, and R. Kirubagaran. 2011. Evaluation of interpolation technique for air quality parameters in Port Blair, India. Univ. J. Environ. Res. Technol. 1:301–10.
- Joseph, A.E., A.D. Sawant, and A. Srivastava. 2003. PM 10 and its impacts on health—A case study in Mumbai PM 10 and its impacts on health. Int. J. Environ. Health Res. 13:207–14. doi:10.1080/0960312031000098107
- Kampa, M., and E. Castanas. 2008. Human health effects of air pollution. Environ. Pollut. 151(2): 362–67. doi:10.1016/j.envpol.2007.06.012
- Kim, S., S. Yi, Y.S. Eum, H. Choi, H. Shin, H.G. Ryou, and H. Kim. 2014. Ordinary Kriging approach to predicting long-term particulate matter concentrations in seven major Korean cities. Environ. Health Toxicol. 29:1–8. doi:10.5620/eht.e2014012
- Krewski, D., and D. Rainham. 2007. Ambient air pollution and population health: Overview. J. Toxicol. Environ. Health Part A 70:275–83. doi:10.1080/15287390600884859
- Kristiansson, M., K. Sörman, C. Tekwe, and L. Calderón-Garcidueñas. 2015. Urban air pollution, poverty, violence and health—Neurological and immunological aspects as mediating factors. Environ. Res. 140:511–13. doi:10.1016/j.envres.2015.05.013
- Kumar, A., A.K. Dikshit, S. Fatima, and R.S. Patil. 2015. Application of WRF model for vehicular pollution modelling using AERMOD. Atmos. Clim. Sci. 5(April): 57–62.
- Lai, A.C.K., T.L. Thatcher, and W.W. Nazaroff. 2012. Inhalation transfer factors for air pollution health risk assessment. J. Air Waste Manage. Assoc. 50:1688–99. doi:10.1080/10473289.2000.10464196.
- Maantay, J. 2007. Asthma and air pollution in the Bronx: Methodological and data considerations in using GIS for environmental justice and health research. Health Place 13(1): 32–56. doi:10.1016/j.healthplace.2005.09.009
- Mage, D., G. Ozolins, P. Peterson, A. Webster, R. Orthofer, V. Vandeweerd, and M. Gwynne. 1996. Urban air pollution in megacities of the world. Atmos. Environ. 30(5): 681–86. doi:10.1016/1352-2310(95)00219-7
- Maharashtra Pollution Control Board,.2012. Ambient Air Quality Monitoring Network in Maharashtra. http://mpcb.gov.in/envtdata/envtair.php.
- Marquez, L.O., and N.C. Smith. 1999. A framework for linking urban form and air quality. Environ. Model. Software 14(6): 541–48. doi:10.1016/S1364-8152(99)00018-3
- Mohan, M., S. Bhari, A. Sreenivas, and P. Marrapu. 2011. Performance evaluation of AERMOD and ADMS-Urban for total suspended particulate matter concentrations in megacity Delhi. Aerosol Air Qual. Res. 11:883–94. doi:10.4209/aaqr.2011.05.0065
- National Environmental Engineering Research Institute. 2004 –2013. Ambient air quality status for six cities of India. Mumbai, India: National Environmental Engineering Research Institute.
- Pandit, G.G., and V.D. Puranik. 2008. Source apportionment of coarse and fine particulate matter at Navi Mumbai, India. Aerosol Air Qual. Res. 8(4): 423–36.
- Patankar, A.M., and P.L. Trivedi. 2011. Monetary burden of health impacts of air pollution in Mumbai, India: Implications for public health policy. Public Health 125(3): 157–64. doi:10.1016/j.puhe.2010.11.009
- Patel, R.B., and F.M. Burkle. 2012. Rapid urbanization and the growing threat of violence and conflict: A 21st century crisis. Prehosp. Disaster Med. 27(2): 194–97.doi:10.1017/S1049023X12000568
- Pope, C.A. III, and D.W. Dockery. 2006. Health effects of fine particulate air pollution: Lines that connect. J. Air Waste Manage. Assoc. 56:709–42. doi:10.1080/10473289.2006.10464485.
- Rood, A. S. 2014. Performance evaluation of AERMOD, CALPUFF, and Legacy Air Dispersion Models using the winter validation tracer study dataset. Atmos. Environ. 89: 707–20. doi:10.1016/j.atmosenv.2014.02.054
- Ryan, P.H., and G.K. Lemasters. 2008. A review of land-use regression models for characterizing intraurban air pollution exposure. Natl. Inst. Health Public Access 19(2): 127–33.
- Shah, J., T. Nagpal, and C. Brandon. 1997. Urban air quality management strategy in Asia: Greater Mumbai report. Guide book. World Bank Technical Paper 381. http://documents.worldbank.org/curated/en/1997/12/693857/urban-air-quality-management-strategy-asia-greater-mumbai-report (accessed February 2015).
- Sohrabinia, M., and A.M. Khorshiddoust. 2007. Application of satellite data and GIS in studying air pollutants in Tehran. Habitat Int. 31(2): 268–75. doi:10.1016/j.habitatint.2007.02.003
- Srivastava, A., and R. Kumar. 2002. Economic valuation of health impacts of air pollution in Mumbai. Environ. Monit. Assess. 75:135–43.doi:10.1023/A:1014431729649
- van Westen, C.J. 2004. Remote sensing and GIS for natural hazards assessment and disaster risk management, 1–61. Enschede, The Netherlands: University of Twente.
- Whitworth, K.W., E. Symanski, D. Lai, and A.L. Coker. 2011. Kriged and modeled ambient air levels of benzene in an urban environment: An exposure assessment study. Environ. Health 10(1): 21. doi:10.1186/1476-069X-10-21.
- Wong, D.W., L. Yuan, and S.A. Perlin. 2004. Comparison of spatial interpolation methods for the estimation of air quality data. J. Expos. Anal. Environ. Epidemiol. 14(5): 404–15. doi:10.1038/sj.jea.7500338
- Zhou, N., Z. Cui, S. Yang, X. Han, G. Chen, Z. Zhou, C. Zhai, et al. 2014. Air pollution and decreased semen quality: A comparative study of Chongqing urban and rural areas. Environ. Pollut. 187(2): 145–52. doi:10.1016/j.envpol.2013.12.030