ABSTRACT
This study used several real-time and filter-based aerosol instruments to measure PM2.5 levels in a high-rise residential green building in the Northeastern US and compared performance of those instruments. PM2.5 24-hr average concentrations were determined using a Personal Modular Impactor (PMI) with 2.5 µm cut (SKC Inc., Eighty Four, PA) and a direct reading pDR-1500 (Thermo Scientific, Franklin, MA) as well as its filter. 1-hr average PM2.5 concentrations were measured in the same apartments with an Aerotrak Optical Particle Counter (OPC) (model 8220, TSI, Inc., Shoreview, MN) and a DustTrak DRX mass monitor (model 8534, TSI, Inc., Shoreview, MN). OPC and DRX measurements were compared with concurrent 1-hr mass concentration from the pDR-1500. The pDR-1500 direct reading showed approximately 40% higher particle mass concentration compared to its own filter (n = 41), and 25% higher PM2.5 mass concentration compared to the PMI2.5 filter. The pDR-1500 direct reading and PMI2.5 in non-smoking homes (self-reported) were not significantly different (n = 10, R2 = 0.937), while the difference between measurements for smoking homes was 44% (n = 31, R2 = 0.773). Both OPC and DRX data had substantial and significant systematic and proportional biases compared with pDR-1500 readings. However, these methods were highly correlated: R2 = 0.936 for OPC versus pDR-1500 reading and R2 = 0.863 for DRX versus pDR-1500 reading. The data suggest that accuracy of aerosol mass concentrations from direct-reading instruments in indoor environments depends on the instrument, and that correction factors can be used to reduce biases of these real-time monitors in residential green buildings with similar aerosol properties.
Implications: This study used several real-time and filter-based aerosol instruments to measure PM2.5 levels in a high-rise residential green building in the northeastern United States and compared performance of those instruments. The data show that while the use of real-time monitors is convenient for measurement of airborne PM at short time scales, the accuracy of those monitors depends on a particular instrument. Bias correction factors identified in this paper could provide guidance for other studies using direct-reading instruments to measure PM concentrations.
Introduction
Over the past 15 years, studies have shown strong correlations between elevated particulate matter (PM) levels and a range of health effects, including early mortality (Schwartz et al., Citation1996; Klemm and Mason, Citation2000; U.S. EPA, Citation1996), exacerbation of respiratory-tract disease, reduced lung function (Hoek et al., Citation1998), and cardiovascular disease (Peters et al., Citation2000; Pope et al., Citation1999). According to the EPA, every 10-µg/m3 increase in fine particles (<2.5 μm in diameter) produces a 6% increase in the risk of death from cardiopulmonary disease and increased morbidity among all age groups (U.S. EPA, Citation1996). Among exposed populations, children are more susceptible than adults to many health effects caused by air pollution (Mathieu-Nolf, Citation2002). It was reported that every 10-µg/m3 increase in PM10 particles (<10 μm in aerodynamic diameter) is associated with a 10% decrease in children’s peak expiratory flow (Dockery and Pope, Citation1994). Such effects are especially severe when both indoor and outdoor concentrations of pollutants including PM10, NO2, SOX, and CO are elevated (Mathieu-Nolf, Citation2002).
Health effects of airborne particulate matter and other pollutants present indoors are important because people spend about 90% of their time inside buildings, and most of that time is in residences (Klepeis et al., Citation2001; Spengler et al., Citation1985; Spengler and Sexton, Citation1983). Green building practices are increasingly being used in residential buildings to reduce environmental burdens associated with building construction and operation (Eichholtz, Kok, and Quigley, Citation2013). Both the U.S. EPA and real-estate corporations have recognized the importance of indoor air quality in green buildings (Albanese Organization, Citation2004; U.S. EPA, Citation2009). A growing body of research suggests that green buildings have better indoor environmental (e.g., air quality, thermal comfort) quality and occupant satisfaction, productivity, and health than nongreen buildings (Abbaszadeh et al., Citation2006; Ahrentzen et al., Citation2012; Eichholtz, Kok, and Quigley, Citation2013). At the same time, data on PM in residential green buildings are only beginning to appear in the peer-reviewed literature and control of indoor PM concentrations is proposed in few green building certifications (Colton et al., Citation2014; Patton et al., Citation2016; Wei, Ramalho, and Mandin, Citation2015). The construction and operation of individual green buildings, as well as occupant behaviors, may strongly affect measured mass concentrations and indoor/outdoor ratios of PM (Patton et al., Citation2016).
Both filter-based gravimetric and real-time methods have been used to measure PM in buildings (e.g., Ramachandran et al., Citation2000; Wallace et al., Citation2006; Wallace et al., Citation2011; Wheeler et al., Citation2011; Yanosky, Williams, and MacIntosh, Citation2002; Van Ryswyk et al., Citation2014; Osman et al., Citation2007). Filter-based methods are robust and relatively easy to operate, but the accumulation of sufficient particle mass and filter weighing usually take a considerable amount of time (McMurry, Citation2000; Pui, Citation1996). In addition, conditioning of filters to remove moisture can also cause the loss of potentially health-related volatile compounds (Huang et al., Citation2013). Many real-time monitors for particulate mass concentrations sense light reflected by a particle stream onto a photodetector and convert the photometric voltage output into concentrations via a calibration curve (Wang et al., Citation2009). Photometers (e.g., DustTrak) measure light reflected at a specific angle, while nephelometers (e.g., pDR) measure light reflected over as wide a range of angles as possible (Hinds, Citation1999). Another monitor similar to a photometer, the optical particle counter, illuminates a single particle at a time to count the number of particles in defined size bins (Hinds, Citation1999). Real-time particle monitors can measure PM concentration spikes and peak exposures, which could have importance for health effects (McMurry, Citation2000; Jiang et al., Citation2011). However, the relationships of real-time measurements to the gravimetric measurements that are used to set air quality standards are often unknown because the relationship between light scattering intensity (and therefore instrument response of optical particle counters or photometers) and particle mass concentration determined gravimetrically depends on properties of the aerosols (e.g., density, reflectivity, size, shape, and composition) used for calibration and the aerosols in the environment (e.g., Kim et al., Citation2004; Yanosky, Williams, and MacIntosh, Citation2002; O’Shaughnessy and Slagley, Citation2002; Pui, Citation1996; Wang et al., Citation2009; Wallace et al., Citation2011; Sorensen et al., Citation2011; Liu and Daum, Citation2000; McMurry, Citation2000; Chang et al., Citation2001).
In the field, real-time monitors are typically calibrated by co-locating real-time and gravimetric instruments, and by using a scaling factor (i.e., calibration factor) or curve of best fit to adjust the measurements reported by the real-time monitors to match the gravimetric measurements (e.g., Sioutas et al., Citation2000; Wallace et al., Citation2011; Wang et al., Citation2009). For example, residential measurements of PM2.5 using a personal DataRAM sampling passively (pDR-1000, Thermo Scientific, Inc.) were 1.10–1.92 times higher than concurrent measurements with Harvard Impactors (HI) and Personal Environmental Monitors (PEM), two types of portable gravimetric instruments (Howard-Reed et al., Citation2000; Wallace et al., Citation2006; Wallace et al., Citation2011). Actively sampling DustTraks (a photometer; models 8520 and earlier) measured concentrations that were 1.94–2.57 times higher than filter-based and federal reference method measurements in occupied and test homes (Ramachandran et al., Citation2000; Wallace et al., Citation2011; Yanosky, Williams, and MacIntosh, Citation2002). All of these comparisons resulted in high correlations (i.e., >0.77) among measurements using different methods. Wheeler et al. (Citation2011) demonstrated that correcting different monitors for site-specific variation in instrument response could lead to high correlation (slope = 1.02; R2 = 0.712) and insignificant bias (intercept = –0.58 μg/m3) between different real-time monitors and filtration-based measurements (Wheeler et al., Citation2011), thus allowing for faster and more convenient estimation of indoor PM. However, the exact relationship between different methods depends on the particles and environments being sampled. Wallace et al. (Citation2006) found that the pDR-1000 had larger overestimates relative to HI and PEM filters for particles from cooking than for particles related to cleaning and personal care products. A study of another photometer (i.e., TSI SidePak) demonstrated that real-time monitors overestimated gravimetric measurements of PM2.5 by factors of 0.92–1.8 for outdoor sources, 1.3 for toasting bread, and 3.4 for cigarette smoke (Jiang et al., Citation2011). Therefore, factors specific to residential indoor air are needed for real-time monitors to approximate actual PM mass concentrations.
In our recent study investigating a green building, a multidisciplinary research team collaborated on a post-occupancy evaluation of the building to examine the advantages, synergies (positive and negative), and trade-offs for the environmental and social impact of a green multifamily dwelling on its residents. As part of the study, we used different impactors equipped with filters and real-time particle monitors to determine relationships in PM2.5 concentrations measured by those instruments. The objective of this paper is to describe the relationships among measurements by the various portable monitors.
Materials and methods
Study site and time frame
The study was performed in both wings of a two-wing multi-apartment (127) residential building in the northeastern United States. The six- and seven-story complex was the largest multifamily high rise building in the nation with Federal Energy Star certification at the time of the study. The building was naturally ventilated except for exhaust vents (designed for 50 m3/hr) in the kitchen and bathroom of each apartment. Smoking was allowed in apartments but not in the common spaces of the buildings, and occupants were allowed to cook, clean, and open windows. The building is located about 100 m from an elevated rail line and at the nexus of two side streets in an urban community known for a predominance of truck routes.
All building occupants were invited to participate in the study via communications by management and the research team using flyers and meetings. Forty households (mostly two-bedroom and one three-bedroom) volunteered for the study and were administered a baseline questionnaire examining their health, lifestyle, and satisfaction with the building. Twenty-one households (apartments) with presence of asthma, obesity, smoking, or a combination of these as per questionnaire were selected for and agreed to additionally participate in detailed indoor air quality (IAQ) measurements. On each sampling day, IAQ measurements were made in one to three apartments (usually in the sitting room). The IAQ measurements were performed in three phases, corresponding to the phases of a cleaning behavioral intervention: Phase I: July–September 2011, Phase II: October–December 2011, and Phase III: March–May 2012. In Phase I, 21 apartments were sampled, while in Phases II and III, 17 apartments were sampled due to participant attrition. These 17 apartments participated in Phase I sampling as well.
Equipment used
Twenty-four-hour PM2.5 samples were collected by a Personal Modular Impactor (PMI) and a personal DataRAM (pDR-1500) set up side-by-side on a tripod. The PMI (PMI2.5; SKC, Inc., Eighty Four, PA) is a single-stage impactor equipped with a filter that uses a 25-mm pre-oiled impaction disc to remove particles larger than 2.5 µm and reduce particle bounce. An AirChek XR5000 Pump (SKC, Inc.) provided the sampling airflow of 3 L/min through the PMI2.5. The SKC PM2.5 impactor, part of SKC’s modular impactor line, closely follows the EPA’s PM2.5 curve (Trakumas, Smith, and Nachreiner, Citation2010). Collocated runs of impactors from this series with MiniVol (Airmetrics, Inc.) and FH 62 C14 continuous ambient PM monitor (Thermo Andersen) samplers showed very good agreement (Trakumas and Smith, Citation2008; Trakumas et al., Citation2006).
The pDR-1500 (Thermo Scientific, Franklin, MA) is a sensitive nephelometric monitor with a cyclone inlet for measurement of the respirable fraction of the airborne particulate matter in different environments. The pDR-1500 reported the average PM2.5 concentration every 10 min and also collected particles that passed through the sensing zone on a 37-mm filter for gravimetric analysis. The pDR-1500 was operated at a flow rate of 1.52 L/min for a PM2.5 cut size. 37-mm Teflon filters with 2.0 μm pore size (SKC, Inc.) were used for both the PMI2.5 sampler and the pDR-1500. Sampling time was programmed into the sampling pump of the PMI2.5 and the pDR-1500 control software. Sampling flow rates were verified at the start and end of sampling using a mass flowmeter (model 4140, TSI, Inc., Shoreview, MN), and the average flow rate was used to calculate the amount of air sampled. All filters were allowed to equilibrate in a weighing room with controlled temperature (21°C) and relative humidity (30–40%) for a minimum of 72 hr prior to weighing before and after sampling. Filters were weighed using a Mettler Toledo MT5 Microbalance. All weighing sessions included weighing of 50- and 200-µg standards. In addition, three blank filters were always kept in the weighing room and weighed during each session as part of the quality assurance (QA) procedure. Their weight changes were within 2–3 µg and no drift was observed. All field sample masses were substantially above the detection limit.
In addition to 24-hr average PM2.5 concentration, various particle mass and size fractions were measured for 1 hr using two instruments: DustTrak DRX (TSI 8534, TSI, Inc.) and AeroTrak optical particle counter (OPC, model 8220, TSI, Inc.). The DustTrak DRX is a combined photometer and optical counter, where a laser diode illuminates a sample stream and reflected light is directed onto a photodetector by a mirror. When properly calibrated the DustTrak DRX has <10% error in comparison to TEOM measurements (Wang et al., Citation2009). The photometric voltage output from the DustTrak DRX was converted to real-time particulate matter (PM) mass concentrations corresponding to PM1, PM2.5, PM4, PM10, and total PM size fractions. The OPC provided particle number concentrations in the following diameter ranges: 0.3–0.5, 0.5–1.0, 1.0–2.5, 2.5–5.0, 5.0–10.0, and >10.0 μm. To compare the 1-hr average mass concentrations with other instruments, the number concentrations measured by OPC were converted into mass concentration using the following assumptions: (1) Particles were spherical, (2) particles in a particular size channel had a size equivalent to the arithmetic mean of that channel, and (3) indoor particles had density of 1.65 g/cm3 (Tittarelli et al., Citation2008). Except for at concentrations <0.5 μg/m3 (poor DustTrak signal) or > 100 μg/m3 (OPC coincidence error), the DustTrak DRX and OPC have good agreement in measurements of monodisperse aerosols (Wang et al., Citation2009). These two instruments were operated side-by-side on a table in the same sitting room. During this time, the PMI2.5 and pDR-1500 were also running.
All real-time monitors were manufacturer-calibrated for the study. As per manufacturers, the Aerotrak 8220 was calibrated using NIST traceable polystyrene latex (PSL) spheres; the DustTrak DRX was calibrated using emery oil and nominally adjusted to the respirable mass of standard ISO 12103-1, A1 test dust (Arizona Road Dust); and the pDR-1500 was calibrated using Arizona Road Dust. PSL has density ρp = 1.05 g/cm3 and refractive index m = 1.59, while Arizona Road Dust has density ρp = 2.65 g/cm3 and refractive index m = 1.54 (refractive index of Arizona Road Dust; Applied Physics, Inc., Citation2015; Wang et al., Citation2009).
Laboratory collocation tests were done where possible to assess the precision of the monitors. Two co-located PMI impactors were operated over 24 hr on 7 different days in a low-pollution environment (~0.06 mg collected on average per 24 hr); their results were not statistically different (p = 0.09 from a two-tailed t-test). The mass collected by two co-located SKC modular impactors in a high concentration environment (~0.8 mg on average in 24-hr samples) differed by less than 5% and were not statistically different (p = 0.49 from a two-tailed t-test). Two TSI OPCs were run side by side as part of this project, and particle counts within individual channels showed good agreement (average R2 of six size channels = 0.85). The two pDRs used in this study were run side-by-side indoors at 1.2 L/min and 3 L/min sampling flow rates, and the average ratios of their measured values were 1.02 ± 0.13 and 1.06 ± 0.09, respectively. The Pearson correlations for those two flow rates were 0.73 and 0.88, respectively. No collocation test was done for the DustTrak DRX because only one monitor was available; the manufacturer reported sampled airflow accuracy was ±5% (TSI, Incorporated, Citation2014).
Statistical analysis
STATISTICA 7 software package (StatSoft, Inc., Tulsa, OK) was used to evaluate both constant and proportional bias and to assess the correlation between any two sampling methods. A significance level of 0.05 was used in all tests. Ordinary least-squares linear regression was performed for the PM2.5 levels obtained by two sampling methods. The regression is presented by the following equation:
where β1 and β0 are regression coefficients, and SE is the standard error of β values. If two sampling methods match exactly, the regression intercept, β0, should be equal to 0 and the regression slope, β1, should be equal to 1. Thus, the following hypothesizes were developed and p-values for each hypothesis were calculated to compare sampling methods:
If the regression intercept, β0, was found to be significantly different from zero, it was considered to indicate the systematic (constant) bias of the PM2.5 levels between two samplers or methods. If the regression slope, β1, was found to be significantly different from unity (1), it was considered to indicate the proportional bias of PM2.5 levels between two samplers. The coefficient of determination (R2) was used to describe the correlation of measured levels between two sampling methods, and the root mean square error (RMSE) was calculated to compare the relative agreement of the sampling methods.
Results and discussion
The data from all three sampling phases were pooled together for the comparison analysis, for a total of 48 sets of measurements in 21 apartments. The 24-hr PMI2.5, OPC, and DustTrak DRX records were complete; only 40 apartment visits had pDR-1500 data because only two pDR-1500 units were available (). For 1-hr average PM2.5 levels, the OPC had only 34 valid data points from Phases II and III due to the malfunction (faulty data retrieval) of the unit in Phase I. Most measurement methods recorded mean PM2.5 mass concentrations between 22 μg/m3 and 35 μg/m3, although the mean OPC measurement was much lower (5.96 μg/m3). The highest recorded PM2.5 mass concentration was 156 μg/m3, and was obtained from a pDR-1500 direct reading. Indoor and outdoor measurements from the DustTrak DRX on the same day showed that concentrations of PM2.5 were typically higher indoors than outdoors; ratios of indoor concentrations to outdoor concentrations (both measured by DustTrak DRX) ranged from 0.53 to 9.26 (mean = 2.03, median = 1.41) (Patton et al., Citation2016). During measurements, investigators observed open windows in 25 apartments, closed windows in 16 apartments, and window air-conditioning units in 10 apartments. Investigators reported cooking in five nonsmoking apartments and smoke infiltration from a neighboring apartment in one nonsmoking apartment. One apartment with a smoker also had oil fragrance burning. No other sources of combustion were observed in the apartments.
Table 1. Summary of PM2.5 measurements (μg/m3) from the different real-time and filter-based metrics.
A comparison of the 24-hr PM2.5 average concentrations between pDR-1500 reading and pDR-1500 filter measurements is presented in . The intercept was not significantly different from zero (β0 = –3.30, p = 0.233), but the slope was significantly different from unity (β1 = 1.42, p < 0.001). Therefore, there was a significant proportional bias between these two methods using the same instrument, although the concentration values provided by the two methods were correlated (R2 = 0.804). The value β1 = 1.42 also indicates that direct reading showed concentrations on average 42% higher than filter weighing. One possible explanation for this result is that some PM was lost from the pDR filter during sampling, transport, or filter weighing (Alderman and Ingebrethsen, Citation2011; Scian et al., Citation2009). Alternatively, as mentioned on the calibration certificate, it is possible the pDR-1500 calibration factor determined by calibration with Arizona Road Dust was not the best fit for the environment investigated in this study. The pDR-1500 can save up to 50 different calibration factors, so saving a calibration factor in the instrument software based on preliminary data comparing the pDR-1500 direct reading to either the pDR-1500 filter or another gravimetric measurement can improve the accuracy of the direct readings from the pDR-1500 (Thermo Fisher Scientific, Inc., Citation2014).
Figure 1. Comparison of PM2.5 24 hr average concentrations between pDR-1500 direct reading and pDR-1500 filter-based measurements. The regression is presented by the following equation: y = β1(±SE)x + β0(±SE) where β1 and β0 are regression coefficients, and SE is the standard error of β values.
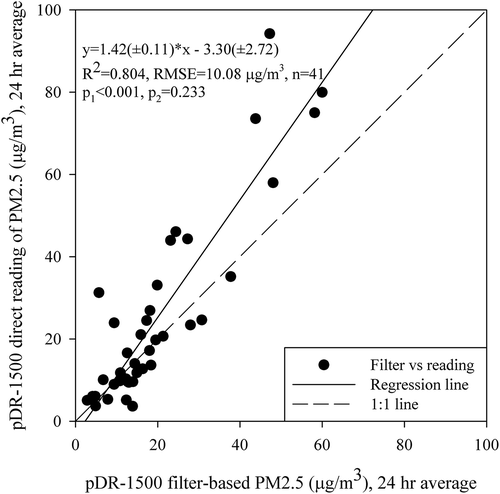
The PMI2.5 (filter) comparison with pDR-1500 filter measurements is shown in . The intercept was not significantly different from zero (β0 = 0.04, p = 0.982) but the slope was significantly smaller than unity (β1 = 0.79, p = 0.002); that is, on average pDR-1500 filter underestimated PM2.5 concentration by approximately 20% compared to the PMI2.5 filter. The comparison of the 24-hr average PM2.5 levels between PMI2.5 filter and pDR-1500 direct reading measurements is shown in . The intercept was significantly different from zero (β0 = –6.38, p = 0.042) and the slope was significantly different from one (β1 = 1.25, p = 0.018). Therefore, there were significant systematic (6.38 µg/m3) and proportional (25%) biases between these two methods, with pDR-1500 showing higher concentrations compared to PMI2.5 impactor.
Figure 2. Comparison of PM2.5 24-hr average concentrations determined by PMI PM2.5 filter-based measurements and pDR-1500 filter-based measurements. The regression is presented by the following equation: y = β1(±SE)x + β0(±SE) where β1 and β0 are regression coefficients, and SE is the standard error of β values.
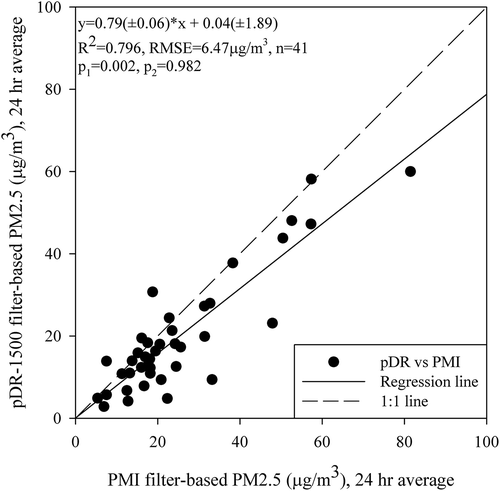
Figure 3. Comparison of PM2.5 24-hr average concentrations determined by PMI PM2.5 filter-based measurements and pDR-1500 direct reading measurements. The regression is presented by the following equation: y = β1(±SE)x + β0(±SE) where β1 and β0 are regression coefficients, and SE is the standard error of β values.
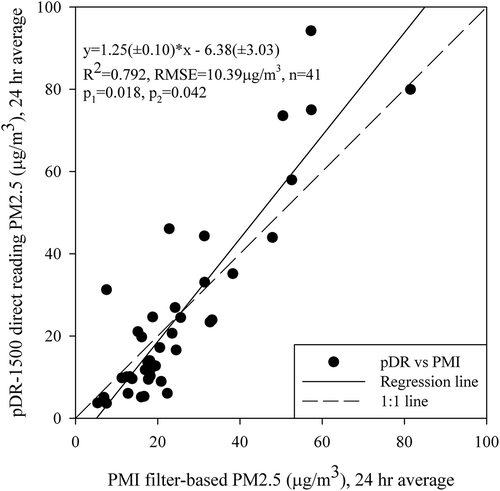
Since real-time monitors are known to overestimate concentrations of smoke particles more than other indoor aerosols relative to gravimetric measurements (Jiang et al., Citation2011), we conducted stratified analyses by household smoking status. According to the questionnaire data administered to the study participants, about 62% of apartments participating in this study self-reported having one or more smokers in the apartment. No smoking was observed during the time that investigators were in the apartments (except for one case of smoking in a neighboring apartment), and the questionnaire did not ask for the frequency or room of smoking. Thus, the data of the 24-hr PM2.5 average concentrations measured by PMI2.5 filter and pDR-1500 direct reading were stratified into two groups according to the self-reported smoking and nonsmoking status in investigated homes, and the results are presented in . It can be seen that for nonsmoking homes the correlation between these two methods has improved (R2 = 0.937) and that the biases were reduced to not statistically significant levels (β0 = –1.78, p = 0.604; β1 = 1.04, p = 0.718). On the other hand, for smoking homes, the differences between these two methods increased (R2 = 0.773; β0 = –10.29, p = 0.015; β1 = 1.44, p = 0.005). While direct comparison of the PMI or pDR-1500 filter concentrations to the OPC measurements was not possible because the monitors were not operating for the same amount of time, we would expect similarly improved relationships between these measurement techniques for subsets of homes with similar aerosol composition.
Figure 4. Comparison of PM2.5 24 hr average concentrations determined by PMI PM2.5 filter-based measurements and pDR-1500 direct reading measurements, with data stratified according to the self-reported smoking status in investigated apartments. The regression is presented by the following equation: y = β1(±SE)x + β0(±SE) where β1 and β0 are regression coefficients, and SE is the standard error of β values.
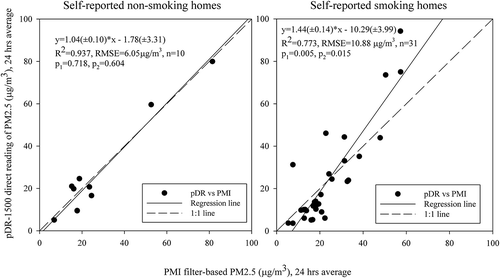
The differences in monitor comparisons between smoking and nonsmoking apartments may have been related to different factors affecting light-based and filter-based measurements, and are similar to differences previously reported in chamber studies of environmental tobacco smoke that compared responses of nephelometers to gravimetric measurements (Brauer et al., Citation2000; Jenkins et al., Citation2004). In general, filter-based readings are probably more accurate than light-based readings because the output of direct reading instruments depends on the reflective properties and size distribution of particles that were used for calibration (Liu and Daum, Citation2000; Sioutas et al., Citation2000; Kim et al., Citation2004; Yanosky, Williams, and MacIntosh, Citation2002; O’Shaughnessy and Slagley, Citation2002; Pui, Citation1996; Wang et al., Citation2009; Jiang et al., Citation2011). Although the mass median diameter of environmental tobacco smoke (ETS) is ~200 nm (Klepeis et al., Citation2003), larger than the lower limit of 100 nm for photometers that rely on Mie scattering, there may have been some undercounting of the smallest particles. Photometers calibrated with Arizona Road Dust are expected to read high for ETS because smoke particles are both smaller and less dense than Arizona Road Dust (Jiang et al., Citation2011). However, it has also been observed that filter-based techniques can have inaccuracies in both mass and composition due to the loss of volatile and semivolatile components of tobacco smoke when sampling smoking emissions (Thorne and Adamson, Citation2013; Adamson et al., Citation2012; Alderman and Ingebrethsen, Citation2011; Scian et al., Citation2009). Thus, it is possible that some particles originating from tobacco smoke lost mass due to evaporation during sampling, as well as during filter handling and weighing, and that this loss caused differences between gravimetric (PMI2.5 and pDR-1500 filters) and real-time measurements. It is also possible that the composition of particles in smoking and nonsmoking households had different light-scattering properties and thus affected pDR-1500 readings. Thus, according to our data, the discrepancy between pDR-1500 direct reading and the PMI2.5 filter method could be at least partially explained by the presence/absence of tobacco smoke and different properties of particles in those two household types. At the same time, due to the relatively low number of data points, especially for nonsmoking homes, the conclusion should be treated with caution and a more comprehensive investigation is warranted.
Another set of comparisons was carried out for 1-hr concentrations measured by direct reading instruments. The PM2.5 mass estimate by AeroTrak OPC was compared with a corresponding 1-hr average of pDR-1500 readings and the data are presented in . The correlation between these two methods was high with R2 = 0.936. However, the slope (β1 = 0.40) of the regression line was significantly lower than unity (p1 < 0.001), so the OPC PM2.5 mass estimate underestimated that based on pDR-1500 by a factor of 2.5. This underestimation is likely to be caused by the coincidence losses inside the OPC and/or violation of one or more of the assumptions used to calculate particles mass concentration based on their number distribution. Because OPCs measure optical equivalence size relative to the polystyrene latex spheres (PSL) used for calibration, which have a higher refractive index (1.59) than most atmospheric aerosols, the measured optical size would likely underestimate geometric size, causing the mass to be further underestimated (Binnig, Meyer, and Kasper, Citation2007; Liu and Daum, Citation2000). Another possible contribution to a lower mass measured by the OPC could be nonsphericity of particles or the presence of high concentrations of particles smaller than the OPC’s detection limit of 0.3 μm. Such particles were not measured in this study, but instances of their high concentrations indoors have been confirmed in an ongoing study (unpublished data). Systematic or constant bias was only ~1 µg/m3, but it was statistically significant (p = 0.012). On the other hand, a strong correlation between these two methods suggests that the biases can be corrected by using the slope and intercept from a regression line of the OPC estimation versus the pDR-1500 direct reading (considered a reference here). This is a reasonable assumption given the absence of filter data for 1-hr of sampling and a relatively good agreement between pDR-1500 direct reading and its filter data. Such regression equation would be as follows:
Figure 5. Comparison of PM2.5 1-hr average concentrations determined by pDR-1500 direct reading measurements and Aerotrak OPC estimate assuming particles are spherical and have a density of 1.68 g/cm3. The regression is presented by the following equation: y = β1(±SE)x + β0(±SE) where β1 and β0 are regression coefficients, and SE is the standard error of β values.
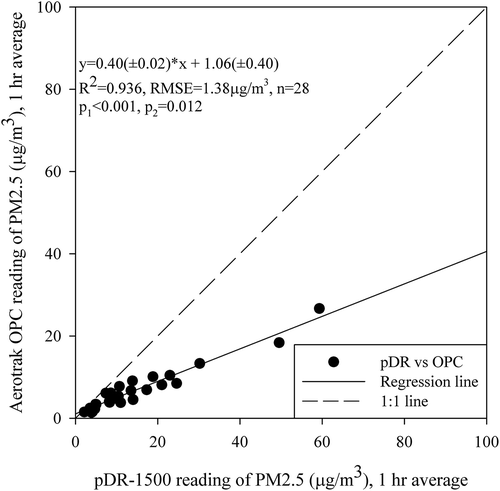
where y equals the corrected value for the OPC mass estimation and x equals the uncorrected OPC mass estimation using the three assumptions already described.
A comparison of 1-hr average levels between DustTrak DRX PM2.5 reading and pDR-1500 direct reading measurements is shown in . The intercept of the regression line was significantly higher than zero (β0 = 10.46, p < 0.001) and the slope was significantly higher than 1 (β1 = 1.94, p < 0.001), indicating significant systematic and proportional biases between these two methods. The DRX appears to overestimate the mass concentration by approximately a factor of 2 and has a systematic bias of approximately 10 µg/m3 compared to pDR-1500 direct reading. The bias observed in the intercept could have been related to nonlinearities in the relationship between measures at very low concentrations, or related to how particles of different composition affected the reading. Both monitors read zero during zero-filter checks prior to measurements. According to , the pDR-1500 direct reading overestimated mass concentration by 25% compared to PMI PM2.5 values; thus, one can infer that the DustTrak DRX PM2.5 reading overestimated PM2.5 mass by a factor of 2.4 compared to the PMI filter. Although we used a different DustTrak model (DRX vs. 8520) in our study, the slope of the regression in the present study was near the middle of the range of those for previous indoor air studies comparing DustTraks to filter-based methods: 1.94 observed by Ramachandran et al. (Citation2000) in the Minneapolis–St. Paul, MN, metropolitan area, 2.57 observed by Yanosky, Williams, and MacIntosh (Citation2002) in Athens, GA, 2.78 observed by Wallace et al. (Citation2011) in Windsor, ON, Canada, and 3 observed by Osman et al. (Citation2007) in northeast Scotland.
Figure 6. Comparison of PM2.5 1-hr average concentrations determined by pDR-1500 and DustTrak DRX direct reading measurements. The regression is presented by the following equation: y = β1(±SE)x + β0(±SE) where β1 and β0 are regression coefficients, and SE is the standard error of β values.
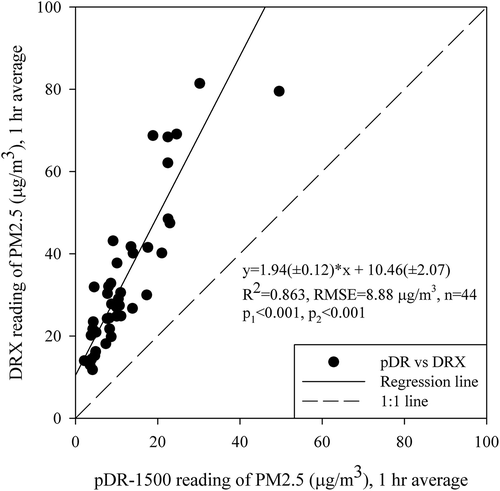
The high correlation (R2 = 0.863) between pDR-1500 and DustTrak DRX readings in our study indicates that their readings could be correlated by a linear regression function:
where y equals the corrected value for the DRX readings and x equals the uncorrected DRX readings. Therefore, our data support a calibration factor (inverse of overestimation factor) of 0.44 for the DustTrak DRX in the green building visited in this study, which is also similar to the manufacturer-recommended default of 0.38 (TSI, Citation2013). Using eq 5, one could quickly adjust DustTrak DRX readings to more accurately reflect PM2.5 concentration indoors, if pDR-1500 direct reading were used as a reference.
We have introduced additional relationships among real-time particle monitors that can be applied as correction factors to estimate airborne PM2.5 concentration. Overall, our data show that most of the compared instrument pairs had systematic and proportional biases relative to each other; however, in most cases they agreed within a factor of 2. The largest discrepancy was observed between the pDR-1500 direct reading and the Aerotrak OPC. In general, our comparisons of real-time and filter-based measurements are in agreement with results reported in other studies (e.g., Howard-Reed et al., Citation2000; Wallace et al., Citation2006; Chang et al., Citation2001; Ramachandran et al., Citation2000; Wallace et al., Citation2011). Our comparisons may have been marginally affected by particle size and number concentration, and were probably not affected by humidity. Concentrations reported by the pDR-1500 and DustTrak DRX were weakly negatively correlated with the volume mean diameter reported by the OPC (r = –0.3 and p = 0.06 for both). Similarly, the nephelometer and photometer reported slightly lower concentrations when the OPC number mean was larger (r = –0.3, p = 0.08 for pDR-1500 and r = –0.2, p = 0.18 for DustTrak DRX). Although the humidity inside the building was higher than outdoors during the summer and lower than outdoors during the heating season, we did not observe associations between humidity and real-time pDR or Dustrak DRX measurements (Patton et al., Citation2016). While readings of optical monitors are known to be substantially affected by relative humidity levels >80% (Wallace et al., Citation2011, Fischer and Koshland, Citation2006), the range of relative humidity experienced during measurements (13–68%) would be unlikely to affect readings by more than ~5% (Yu et al., Citation2015), a difference much smaller than other differences among apartments in the study.
Conclusions
For airborne particulate matter monitoring, researchers have been using gravimetric methods in both indoor and outdoor environments. With the advance of real-time measurement instruments, it is now possible to measure PM dynamics in indoor and outdoor environments at short time scales and thereby obtain a detailed picture of PM2.5 variability, especially in health effects studies. At the same time, our data suggest that caution is needed when real-time aerosol monitors are used for determining mass concentration.
We found that measurements by pDR-1500 direct readings matched fairly well with gravimetric measurements from its own filter and PMI2.5 sampler. The correlation between the two instruments especially improved when samples from nonsmoking households were considered (no significant bias and R2 = 0.937). We also found that 1-hr Aerotrak OPC and DustTrak DRX measurements had significant and substantial systematic and proportional biases compared to pDR-1500 1-hr readings. OPC underestimated mass concentration by a factor of 2.5, while DustTrak DRX overestimated mass concentration by a factor of 2 compared to pDR-1500. However, these biases could be reduced for aerosols with similar properties, for example, in residential green buildings, by using correction factors determined by preliminary filter-based sampling in the environment to be monitored with real-time monitors. Additional studies of indoor PM2.5 particle shape and density are needed to develop more universally applicable conversions among different types of measurements. While it is unlikely that the exact same factors presented in this paper could be applied in other indoor studies given site-to-site variability of particle composition, we hope that our data will provide guidance for other studies using direct-reading instruments to measure concentrations of PM fractions.
Funding
This study was supported by the U.S. Department of Housing and Urban Development (HUD) grant “Expanding the Definition of Green: Impacts of Green and Active Living Design on Health in Low Income Housing,” Healthy Homes Program, grant NJLHH-0202-09. APP was supported by a National Institute of Environmental Health Sciences (NIEHS) training grant in exposure science to Rutgers University (T32 ES198543). This work has not been reviewed by any governmental agency. The views expressed are solely those of the authors, and governmental agencies do not endorse any products or commercial services mentioned in this paper.
Additional information
Funding
Notes on contributors
Zuocheng Wang
Zuocheng Wang was at the time of the study a postdoctoral associate at the Department of Environmental Sciences of Rutgers University. Currently, he is a researcher with the IIT Research Institute, Chicago IL.
Leonardo Calderón
Leonardo Calderón is a graduate student at the Department of Environmental Sciences of Rutgers University, NJ.
Allison P. Patton
Allison P. Patton is a postdoctoral fellow at the Environmental and Occupational Health Sciences Institute of Rutgers University in Piscataway, NJ.
MaryAnn Sorensen Allacci
MaryAnn Sorensen Allacci is an Environmental Psychologist with the Center for Green Building at Edward J. Bloustein School of Planning and Public Policy, Rutgers University, NJ.
Jennifer Senick
Jennifer Senick is Executive Director of the Center for Green Building at Edward J. Bloustein School of Planning and Public Policy, Rutgers University, NJ.
Richard Wener
Richard Wener is a Professor of Environmental Psychology in the Department of Technology, Culture and Society at the Polytechnic Institute of New York University.
Clinton J. Andrews
Clinton J. Andrews is Director of the Center for Green Building and Professor at Edward J. Bloustein School of Planning and Public Policy, Rutgers University, NJ.
Gediminas Mainelis
Gediminas Mainelis is a Professor at the Department of Environmental Sciences of Rutgers University, NJ.
References
- Abbaszadeh, S., L. Zagreus, D. Lehrer, and C. Huizenga. 2006. Occupant satisfaction with indoor environmental quality in green buildings. Proceedings, Healthy Buildings 2006, Lisbon, Portugal.
- Adamson, J., S. Hughes, D. Azzopardi, J. McAughey, and M.D. Gaca. 2012. Real-time assessment of cigarette smoke particle deposition in vitro. Chem. Cent. J. 6(1):98. doi:10.1186/1752-153X-6-98
- Ahrentzen, S., J. Ball, H. Destaillets, E. Fonseca, M. Fraser, W. Johnson, M. Patel, and K. Shea. 2012. Is green housing healthy housing; A question for seniors. ENHR 2012 Conference (European Network for Housing Research), Workshop 19: Residential Context of Health, Lillihamer, Norway.
- Albanese Organization. 2004. The Solaire Residences. Marketing brochure. New York City, NY.
- Alderman, S.L., and B.J. Ingebrethsen. 2011. Characterization of mainstream cigarette smoke particle size distributions from commercial cigarettes using a DMS500 fast particulate spectrometer and smoking cycle simulator. Aerosol Sci. Technol. 45(12):1409–21. doi:10.1080/02786826.2011.596862
- Applied Physics, Inc. 2015. PSL Spheres 20 nm to 1 µm, NIST traceability size accuracy. http://www.appliedphysicsusa.com/pslspheres-20nm-900nm.html ( accessed May 9, 2016).
- Binnig, J., J. Meyer, and G. Kasper. 2007. Calibration of an optical particle counter to provide PM2.5 mass for well-defined particle materials. J. Aerosol Sci. 38(3):325–32. doi:10.1016/j.jaerosci.2006.12.001
- Brauer, M., R. Hirtle, B. Lang, and W. Ott. 2000. Assessment of indoor fine aerosol contributions from environmental tobacco smoke and cooking with a portable nephelometer. J. Expos. Anal. Environ. Epidemiol. 10(2):136–44. doi:10.1038/sj.jea.7500076
- Chang, L.-T., H.H. Suh, J.M. Wolfson, K. Misra, G.A. Allen, P.J. Catalano, and P. Koutrakis. 2001. Laboratory and field evaluation of measurement methods for one-hour exposures to O3, PM25, and CO. J. Air Waste Manage. Assoc. 51(10):1414–22. doi:10.1080/10473289.2001.10464375
- Colton, M.D., P. MacNaughton, J. Vallarino, J. Kane, M. Bennett-Fripp, J.D. Spengler, and G. Adamkiewicz. 2014. Indoor air quality in green vs conventional multifamily low-income housing. Environ. Sci. Technol. 48(14):7833–41. doi:10.1021/es501489u
- Dockery, D.W., and C.A. Pope 3rd. 1994. Acute respiratory effects of particulate air pollution. Annu. Rev. Public Health 15:107–32. doi:10.1146/annurev.pu.15.050194.000543
- Eichholtz, P., N. Kok, and J.M. Quigley. 2013. The economics of green building. Rev. Econ. Stat. 95(1):50–63. doi:10.1162/REST_a_00291
- Fischer, S.L., and C.P. Koshland. 2006. Field performance of a nephelometer in rural kitchens: Effects of high humidity excursions and correlations to gravimetric analyses. J. Expos. Sci. Environ. Epidemiol. 17(2):141–50. doi:10.1038/sj.jes.7500486
- Hinds, W.C. 1999. Aerosol technology: Properties, behavior, and measurement of airborne particles, 2nd ed. New York: Wiley.
- Hoek, G., D.W. Dockery, A. Pope, L. Neas, W. Roemer, and B. Brunekreef. 1998. Association between PM10 and decrements in peak expiratory flow rates in children: Reanalysis of data from five panel studies. Eur. Respir. J. 11(6):1307–11. doi:10.1183/09031936.98.11061307
- Howard-Reed, C., A.W. Rea, M.J. Zufall, J.M. Burke, R.W. Williams, J.C. Suggs, L.S. Sheldon, D. Walsh, and R. Kwok. 2000. Use of a continuous nephelometer to measure personal exposure to particles during the U.S. Environmental Protection Agency Baltimore and Fresno Panel Studies. J. Air Waste Manage. Assoc. 50(7):1125–32. doi:10.1080/10473289.2000.10464150
- Huang, L., S.V. Bohac, S.M. Chernyak, and S.A. Batterman. 2013. Composition and integrity of PAHs, nitro-PAHs, hopanes and steranes in diesel exhaust particulate matter. Water Air Soil Pollut. 224(8). doi:10.1007/s11270-013-1630-1. http://link.springer.com/article/10.1007%2Fs11270-013-1630-1
- Jenkins, R.A., R.H. Ilgner, B.A. Tomkins, and D.W. Peters. 2004. Development and application of protocols for the determination of response of real-time particle monitors to common indoor aerosols. J. Air Waste Manage. Assoc. 54(2):229–41. doi:10.1080/10473289.2004.10470892
- Jiang, R.T., V. Acevedo-Bolton, K.C. Cheng, N.E. Klepeis, W.R. Ott, and L.M. Hildemann. 2011. Determination of response of real-time SidePak AM510 monitor to secondhand smoke, other common indoor aerosols, and outdoor aerosol. J. Environ. Monit. 13(6):1695–702. doi:10.1039/c0em00732c
- Kim, J.Y., S.R. Magari, R.F. Herrick, T.J. Smith, and D.C. Christiani. 2004. Comparison of fine particle measurements from a direct-reading instrument and a gravimetric sampling method. J. Occup. Environ. Hyg. 1(11):707–15. doi:10.1080/15459620490515833
- Klemm, R.J., and R.M. Mason. 2000. Aerosol Research and Inhalation Epidemiological Study (ARIES): Air quality and daily mortality statistical modeling—Interim results. J. Air Waste Manage. Assoc. 50(8):1433–39. doi:10.1080/10473289.2000.10464188
- Klepeis, N.E., M.G. Apte, L.A. Gundel, R.G. Sextro, and W.W. Nazaroff. 2003. Determining size-specific emission factors for environmental tobacco smoke particles. Aerosol Sci. Technol. 37(10):780–90. doi:10.1080/02786820300914
- Klepeis, N.E., W.C. Nelson, W.R. Ott, J.P. Robinson, A.M. Tsang, P. Switzer, J.V. Behar, S.C. Hern, and W.H. Engelmann. 2001. The National Human Activity Pattern Survey (NHAPS): A resource for assessing exposure to environmental pollutants. J. Expos. Anal. Environ. Epidemiol. 11(3):231–52. doi:10.1038/sj.jea.7500165
- Liu, Y., and P.H. Daum. 2000. The effect of refractive index on size distributions and light scattering coefficients derived from optical particle counters. J. Aerosol Sci. 31(8):945–57. doi:http://dx.doi.org/10.1016/S0021-8502(99)00573-X
- Mathieu-Nolf, M. 2002. Poisons in the air: A cause of chronic disease in children. J. Toxicol. Clin. Toxicol. 40(4):483–91. doi:10.1081/CLT-120006751
- McMurry, P.H. 2000. A review of atmospheric aerosol measurements. Atmos. Environ. 34(12–14):1959–99. doi:10.1016/S1352-2310(99)00455-0
- O’Shaughnessy, P.T., and J.M. Slagley. 2002. Photometer response determination based on aerosol physical characteristics. AIHA J. (Fairfax, VA) 63(5):578–85. doi:10.1080/15428110208984743
- Osman, L.M., J.G. Douglas, C. Garden, K. Reglitz, J. Lyon, S. Gordon, and J.G. Ayres. 2007. Indoor air quality in homes of patients with chronic obstructive pulmonary disease. Am. J. Respir. Crit. Care Med. 176(5):465–72. doi:10.1164/rccm.200605-589OC
- Patton, A., L. Calderon, Y. Xiong, Z. Wang, J. Senick, M. Sorensen Allacci, D. Plotnik, R. Wener, C. Andrews, U. Krogmann, and G. Mainelis. 2016. Airborne particulate matter in two multi-family green buildings: Concentrations and effect of ventilation and occupant behavior. Int. J. Environ. Res. Public Health 13(1):144. doi:10.3390/ijerph13010144
- Peters, A., E. Liu, R.L. Verrier, J. Schwartz, D.R. Gold, M. Mittleman, J. Baliff, J.A. Oh, G. Allen, K. Monahan, and D.W. Dockery. 2000. Air pollution and incidence of cardiac arrhythmia. Epidemiology 11(1):11–17. doi:10.1097/00001648-200001000-00005
- Pope, C.A. 3rd, R.L. Verrier, E.G. Lovett, A.C. Larson, M.E. Raizenne, R.E. Kanner, J. Schwartz, G.M. Villegas, D.R. Gold, and D.W. Dockery. 1999. Heart rate variability associated with particulate air pollution. Am. Heart J. 138(5 Pt 1):890–99. doi:10.1016/S0002-8703(99)70014-1
- Pui, D.Y.H. 1996. Direct-reading instrumentation for workplace aerosol measurements. A review. Analyst 121(9):1215. doi:10.1039/an9962101215.doi: 10.1039/an9962101215
- Ramachandran, G., J.L. Adgate, N. Hill, K. Sexton, G.C. Pratt, and D. Bock. 2000. Comparison of short-term variations (15-minute averages) in outdoor and indoor PM2.5 concentrations. J. Air Waste Manage. Assoc. 50(7):1157–66. doi:10.1080/10473289.2000.10464160
- Schwartz, J., C. Spix, G. Touloumi, L. Bacharova, T. Barumamdzadeh, A. le Tertre, T. Piekarksi, A. Ponce de Leon, A. Ponka, G. Rossi, M. Saez, and J.P. Schouten. 1996. Methodological issues in studies of air pollution and daily counts of deaths or hospital admissions. J. Epidemiol. Commun. Health 50(Suppl. 1):S3–11. doi:10.1136/jech.50.Suppl_1.S3
- Scian, M.J., M.J. Oldham, J.H. Miller, D.B. Kane, J.S. Edmiston, and W.J. McKinney. 2009. Chemical analysis of cigarette smoke particulate generated in the MSB-01 in vitro whole smoke exposure system. Inhal. Toxicol. 21(12):1040–52. doi:10.1080/08958370802712705
- Sioutas, C., S. Kim, M.C. Chang, L.L. Terrell, and H. Gong. 2000. Field evaluation of a modified DataRAM MIE scattering monitor for real-time PM2.5 mass concentration measurements. Atmos. Environ. 34(28):4829–38. doi:10.1016/S1352-2310(00)00244-2
- Sorensen, C.M., J. Gebhart, T.J. O’Hern, and D.J. Rader. 2011. Optical measurement techniques: fundamentals and applications. In Aerosol Measurement, Principles, Techniques, and Applications, ed. P. Kulkarni, P.A. Baron and K. Willeke, 295–305. New York, NY: Wiley-Interscience.
- Spengler, J.D., and K. Sexton. 1983. Indoor air pollution: A public health perspective. Science 221(4605):9–17. doi:10.1126/science.6857273
- Spengler, J.D., R.D. Treitman, T.D. Tosteson, D.T. Mage, and M.L. Soczek. 1985. Personal exposures to respirable particulates and implications for air pollution epidemiology. Environ. Sci. Technol. 19(8):700–7. doi:10.1021/es00138a008
- Thermo Fisher Scientific, Inc. 2014. MIE pDR-1500 Instruction Manual, Franklin, MA, 356–370.
- Thorne, D., and J. Adamson. 2013. A review of in vitro cigarette smoke exposure systems. Exp. Toxicol. Pathol. 65(7–8):1183–93. doi:10.1016/j.etp.2013.06.001
- Tittarelli, A., A. Borgini, M. Bertoldi, E. De Saeger, A. Ruprecht, R. Stefanoni, G. Tagliabue, R. Contiero, and P. Crosignani. 2008. Estimation of particle mass concentration in ambient air using a particle counter. Atmos. Environ. 42(36):8543–48. doi:10.1016/j.atmosenv.2008.07.056
- Trakumas, S., and D.L. Smith. 2008. Modular impactors—Accurate, simple-to-operate, and cost-effective environmental PM samplers. American Industrial Hygiene Conference & Exposition (AIHce), Minneapolis, MN, May 31 -June 5.
- Trakumas, S., D.L. Smith, and C. Nachreiner. 2010. A cost-effective, simple-to-operate deployable PM sampling system. A&WMA Annual Conference, Calgary, Canada, 22–25 June.
- Trakumas, S., D.L. Smith, C. Nachreiner, and P.M. Hall. 2006. Deployable particulate sampler (DPS): A new cost-effective, simple-to-operate portable PM sampling system. Navy Occupational Health and Preventive Medicine Conference, Hampton, Virginia, 18–24 June.
- TSI. 2013. Rationale for programming a photometer calibration factor (PCF) of 0.38 for ambient monitoring. Application Note EXPMN-007. http://www.tsi.com/uploadedFiles/_Site_Root/Products/Literature/Application_Notes/EXPMN-007_Rationale_Programming_PCF_Ambient_Monitoring.pdf ( accessed August 26, 2013).
- TSI, Incorporated. 2014. DUSTTRAK™ DRX Aerosol Monitors Models 8533, 8533EP and 8534. http://www.tsi.com/uploadedFiles/_Site_Root/Products/Literature/Spec_Sheets/DustTrak-DRX-6001981_USA-web.pdf ( accessed April 26, 2016).
- U.S. EPA. 1996. Air Quality Criteria for Particulate Matter. Washington, DC: U.S. EPA.
- U.S. EPA. 2009. Indoor air quality website. http://www.epa.gov/iaq/greenbuilding ( accessed November 6, 2015).
- Van Ryswyk, K., A.J. Wheeler, L. Wallace, J. Kearney, H. You, R. Kulka, and X. Xu. 2014. Impact of microenvironments and personal activities on personal PM2.5 exposures among asthmatic children. J. Expos. Sci. Environ. Epidemiol. 24(3):260–68. doi:10.1038/jes.2013.20
- Wallace, L., R. Williams, A. Rea, and C. Croghan. 2006. Continuous weeklong measurements of personal exposures and indoor concentrations of fine particles for 37 health-impaired North Carolina residents for up to four seasons. Atmos. Environ. 40(3):399–414. doi:10.1016/j.atmosenv.2005.08.042
- Wallace, L.A., A.J. Wheeler, J. Kearney, K. Van Ryswyk, H. You, R.H. Kulka, P.E. Rasmussen, J. R. Brook, and X. Xu. 2011. Validation of continuous particle monitors for personal, indoor, and outdoor exposures. J. Expos. Sci. Environ. Epidemiol. 21(1):49–64. doi:10.1038/jes.2010.15
- Wang, X.L., G. Chancellor, J. Evenstad, J.E. Farnsworth, A. Hase, G.M. Olson, A. Sreenath, and J.K. Agarwal. 2009. A novel optical instrument for estimating size segregated aerosol mass concentration in real time. Aerosol Sci. Technol. 43(9):939–50. doi:10.1080/02786820903045141
- Wei, W.J., O. Ramalho, and C. Mandin. 2015. Indoor air quality requirements in green building certifications. Building Environ. 92:10–19. doi:10.1016/j.buildenv.2015.03.035
- Wheeler, A.J., L.A. Wallace, J. Kearney, K. Van Ryswyk, H.Y. You, R. Kulka, J.R. Brook, and X.H. Xu. 2011. Personal, indoor, and outdoor concentrations of fine and ultrafine particles using continuous monitors in multiple residences. Aerosol Sci. Technol. 45(9):1078–89. doi:10.1080/02786826.2011.580798
- Yanosky, J.D., P.L. Williams, and D.L. MacIntosh. 2002. A comparison of two direct-reading aerosol monitors with the federal reference method for PM2.5 in indoor air. Atmos. Environ. 36(1):107–13. doi:10.1016/S1352-2310(01)00422-8
- Yu, C.H., A.P. Patton, A. Zhang, Z.H. Fan, C.P. Weisel, and P.J. Lioy. 2015. Evaluation of diesel exhaust continuous monitors in controlled environmental conditions. J. Occup. Environ. Hyg. 12(9):577–87. doi:10.1080/15459624.2015.1022652