ABSTRACT
This study aims to examine the effect of short-term changes in the concentration of particulate matter of diameter ≤2.5 µm (PM2.5) and ≤10 µm (PM10) on pediatric hospital admissions for pneumonia in Jinan, China. It explores confoundings factors of weather, season, and chemical pollutants. Information on pediatric hospital admissions for pneumonia in 2014 was extracted from the database of Jinan Qilu Hospital. The relative risk of pediatric hospital admissions for pneumonia was assessed using a case-crossover approach, controlling weather variables, day of the week, and seasonality. The single-pollutant model demonstrated that increased risk of pediatric hospital admissions for pneumonia was significantly associated with elevated PM2.5 concentrations the day before hospital admission and elevated PM10 concentrations 2 days before hospital admission. An increment of 10 μg/m3 in PM2.5 and PM10 was correlated with a 6% (95% CI 1.02–-1.10) and 4% (95% CI 1.00–1.08) rise in number of admissions for pneumonia, respectively. In two pollutant models, PM2.5 and PM10 remained significant after inclusion of sulfur dioxide or nitrogen dioxide but not carbon monoxide. This study demonstrated that short-term exposure to atmospheric particulate matter (PM2.5/PM10) may be an important determinant of pediatric hospital admissions for pneumonia in Jinan, China.
Implications: This study demonstrated that short-term exposure to atmospheric particulate matter (PM2.5/PM10) may be an important determinant of pediatric hospital admissions for pneumonia in Jinan, China, and suggested the relevance of pollutant exposure levels and their effects. As a specific group, children are sensitive to airborne particulate matter. This study estimated the short-term effects attribute to other air pollutants to provide references for relevant studies.
Introduction
Pneumonia is an acute respiratory infection that affects the lungs. Globally, pneumonia accounts for 15% of all deaths in children less than 5 years of age and was the largest infectious cause of death in children in 2015 (Shibata et al., Citation2014). Many studies have demonstrated associations between selected air pollutants and adverse health effects in children in the aspects of childhood hospital admissions (Barnett et al., Citation2005), school absences (Hales et al., Citation2016), physician visits for upper and lower respiratory illness (Aguilera et al., 2005), and deficits in lung function growth rates (den Dekker et al., Citation2016). Infectious agents associated with pneumonia include bacteria, viruses, and fungi. Susceptibility to pneumonia may be increased by environmental factors, such as ambient air pollution (Darrow et al., Citation2014). Children are particularly vulnerable to the respiratory health effects of ambient air pollution because of their frequent exposure to outdoor air, high breathing rate, narrow airways, and developing lungs and immune systems (Lin et al., Citation2008).
Epidemiological studies in Europe, North America, and developing countries indicate that exposure to pollutants in ambient air has a detrimental effect on the health status of children (Darrow et al., Citation2014; Anenberg et al., Citation2012; Wang et al., Citation2013; Kesavachandran et al., Citation2013). These reports suggest that primary traffic pollutants, ozone, and fine and coarse particulate air pollution exacerbate upper and lower respiratory infections and the disease burden of respiratory infections such as pneumonia, bronchiolitis, bronchitis, and upper respiratory infection in early life (Darrow et al., Citation2014; Anenberg et al., Citation2012; Wang et al., Citation2013; Kesavachandran et al., Citation2013). Particulate matter originated from other sources, such as burning of fossil fuels and biomass, is also associated with increased incidence of pneumonia and increased symptoms and respiratory disease (Souza and Nascimento, Citation2016). Experimental and epidemiologic studies have demonstrated that the severity of pneumonia has positive associations with exposure of air pollutants (Harrod et al., Citation2003; Mikerov et al., Citation2008).
The World Health Organization includes the concentrations of particulate matter ≤2.5 µm (PM2.5) and ≤10 µm (PM10) in diameter as pollutant indicators in the 2014 ambient air pollution database (World Health Organization, Citation2014a). In spite of this, relatively few studies of ambient air pollution and respiratory infection have focused on early childhood, especially for young children in China (Qiu et al., Citation2014; Le et al., Citation2012).
The objective of this study was to examine the effect of short-term changes in airborne particulate matter (PM2.5/PM10) concentrations on pediatric hospital admissions for pneumonia in Jinan, China, and to explore confounding factors of weather, season, and chemical pollutants.
Materials and methods
Study population
Air quality in Jinan is of significant concern to public health. In fact, Jinan is among the 10 cities with the worst air quality in China. Jinan is a northern inland city with complex natural terrain and poor airflow and ventilation. Key environmental concerns include coal-based steel plants, cement plants, and thermal power plants, as well as other heavy industry in the urban and urban fringe areas. Data from the current study suggested smoke, dust, ground dust pollution, and SO2 caused by coal combustion are having a long-term impact on air quality and public health in Jinan.
The total population of Jinan has increased to 706.69 million at the end of 2014 and nine grade 3 and first-class pediatric hospitals located in downtown. Pediatric patients hospitalized for pneumonia in 2014 were eligible for this study. Patients had to be from the registered population of Jinan and got medical insurance, eliminating the floating population from the analyses.
Inclusion criteria were (1) ≤15 years of age; (2) receiving medication for the treatment of pneumonia; and (3) admission diagnosis of pneumonia from the emergency department (ED). Admission from the ED eliminated time bias. Pediatric patients diagnosed with pneumonia secondary to congenital heart disease and ventricular septal defect were excluded.
Information on pneumonia patients, including age, gender, date of admission, and date of discharge, was extracted from the Jinan Qilu Hospital database.
Airborne particulate matter and meteorology monitoring
Data describing the daily average concentrations of airborne particulate matter in Jinan in 2014 were provided by Quancheng Square monitoring station, which is managed by Jinan Environment Monitoring Center. Information on fine particles (PM2.5), inhalable particles (PM10), carbon monoxide (CO), nitrogen dioxide (NO2), and sulfur dioxide (SO2) was provided. Data describing daily average air temperatures and relative humidity in Jinan in 2014 were provided by the Jinan Bureau of Meteorology. To obtain a complete data set on daily average concentrations of airborne particulate matter, daily average air temperature, and relative humidity during the study period, the expectation–maximization (EM) method was used to impute missing data.
Statistical analyses
The impact of airborne particulate matter (PM2.5/PM10) on pediatric hospital admissions for pneumonia was evaluated using a case-crossover study design, as the environmental exposures responsible for pediatric hospital admissions for pneumonia are considered transient. Control days were selected using a time-stratified approach, as previously described (Santus et al., Citation2012). Briefly, every seventh day from the day of hospital admission within the same month and year of the hospital admission was considered a control. The day of hospital admission was termed lag 0, the day before hospital admission was lag 1, and the day before lag 1 was lag 2. The effects on the pediatric hospital admissions for pneumonia were investigated on the day of admission (lag 0), and also on the subsequent 5 days (lags 1–5).
To estimate the association between pediatric hospital admissions for pneumonia and concentrations of airborne particulate matter (PM2.5/PM10), conditional logistic regression models were fitted using odds ratios (OR) and corresponding 95% confidence intervals (95% CI), with weights equal to the number of hospital admissions on that day. Conditional logistic regression analysis was conducted using Cox regression models and the matched-paired method of 1:3 with SPSS Statistics version 19.0 software.
To assess the stability of the impact of airborne particulate matter (PM2.5/PM10) on health, single-pollutant models and two-pollutant models with different combinations of pollutants were used. Each pollutant model was adjusted for confounding factors, including average temperatures on the day of hospital admission and during lag intervals. An increment of 10 μg/m3 was measured for each pollutant to calculate an OR and determine the most appropriate lag period. The criterion for significance was set at p < 0.05.
Stratified analyses of exposure based on gender (male or female), age (<1 year; 1 to 4 years; 5 to 15 years) and season (warm: April to September; cold: October to March) were conducted to examine the impact of confounding factors on pediatric hospital admissions for pneumonia.
Results
Study subjects and pollutants
There were 379 admissions for pediatric pneumonia to the Jinan Qilu hospital in 2014. Of these, 253 were male (66.8%) and 126 were female (33.2%); 103 (27.2%) were infants aged <1 year, 144 (38.0%) were 1 to 4 years of age, and 132 (34.8%) were 5 to 15 years of age.
The average annual concentrations of PM2.5, PM10, SO2, and NO2 were 96 μg/m3, 175 μg/m3, 69 μg/m3, and 55 μg/m3, respectively, in Jinan in 2014. These concentrations were 3.2-, 4.1-, 1.2-, and 0.9-fold, respectively, greater than the Annual Secondary National Ambient Air Quality Standards (GB3095-2013). The distribution of daily concentrations of air pollutants and daily average temperatures is shown in .
Table 1. The distribution of daily ambient pollutant concentrations and daily temperature in Jinan, China, in 2014.
shows the Spearman correlation coefficients among daily concentrations of air pollutants, daily average temperature, and relative humidity. There was a positive correlation among several pollutants, in particular between PM10 and PM2.5 (r = 0.875), PM2.5 and CO (r = 0.745), SO2 and NO2 (r = 0.721), SO2 and CO (r = 0.760), and NO2 and CO (r = 0.741) (p < 0.01). Daily pollutant levels were significantly increased in association with a decrease in daily average temperature and relative humidity (p < 0.01).
Table 2. Spearman correlation coefficients among daily concentrations of air pollutants and daily average temperature in Jinan, China, in 2014.
Pediatric hospital admissions for pneumonia
The impacts of PM2.5/PM10 on pediatric hospital admissions for pneumonia were explored with single-pollutant and two-pollutant models.
In the single-pollutant models, the most appropriate lag period for PM2.5, PM10, NO2, and SO2 was lag 5, lag 2, lag 2, and lag 2, respectively. The single-pollutant model demonstrated a 6% (95% CI 1.02–1.10) increased risk of pediatric hospital admissions for pneumonia per 10-μg/m3 increase in PM2.5 concentration on lag 5; a 4% (95% CI 1.00–1.08) increased risk of pediatric hospital admissions for pneumonia per 10-μg/m3 increase in PM10 concentration on lag 2; and a 5% and 3% increased risk of pediatric hospital admissions for pneumonia per 10-μg/m3 increase in NO2 and SO2 concentrations on lag 2, and lag 2, respectively ( and ).
Table 3. The association between daily ambient pollutant concentrations and pediatric hospital admissions for pneumonia in Jinan, China, in 2014: single-pollutant model.
Figure 1. Lag structures of the odds ratios (OR) and 95% confidence intervals (95% CI) of daily ambient pollutant concentrations with pneumonia admissions for children for pneumonia in Jinan, China, in 2014. Adjusted for temperature and relative humidity; represented as per 10-μg/m3 increases in pollutant. *Significant at p < 0.05.
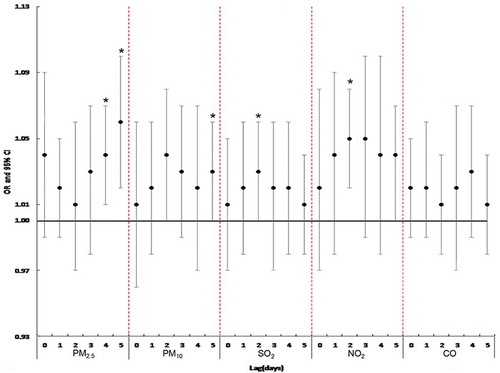
The two-pollutant models of PM2.5 and SO2 and PM10 and NO2 showed that PM2.5 and PM10 remained significantly associated with pediatric hospital admissions for pneumonia. Two-pollutant models of PM2.5 or PM10 and CO were not statistically significant for PM2.5 and PM10 ( and ).
Table 4. The association between daily ambient pollutant concentrations and pediatric hospital admissions for pneumonia in Jinan, China, in 2014: two-pollutant models.
Figure 2. Association between air pollutants concentration and pneumonia admissions for children in two-pollutants models. Adjusted for temperature and relative humidity; represented as per 10-μg/m3 increases of PM2.5/PM10. Significant at *p < 0.05.
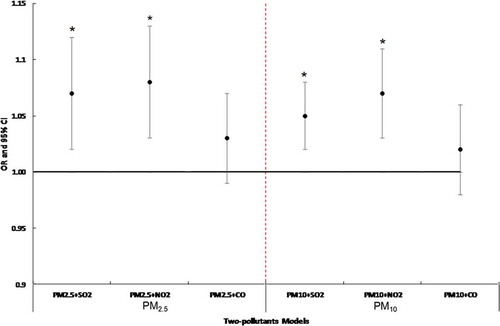
Stratifying data based on gender, age, and season showed that children of male gender and the youngest age group (<1 year) were at highest risk of hospital admission for pneumonia due to airborne particulate matter, particularly on warm days ().
Figure 3. Stratification analyses of gender, season, and age specific odds ratios (OR) and 95% confidence intervals (95% CI) of PM2.5/PM10 associated with pneumonia admissions for children in exposure to the most appropriate lag period. Adjusted for temperature and relative humidity; represented as per 10 μg/m3 increases of PM2.5/PM10. Significant at *p < 0.05.
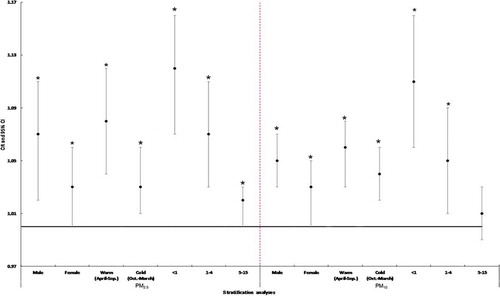
Discussion
This study showed pediatric hospital admissions for pneumonia increased to 1.06% (95% CI, 1.02–1.10) per 10-μg/m3 increase in previous-day PM2.5 concentrations, and to 1.04% (95% CI, 1.00–1.08) per 10-μg/m3 increase in PM10 concentrations 2 days prior to hospital admission, in Jinan, China, in 2014. The association between PM2.5 and PM10 concentrations and pediatric hospital admissions for pneumonia remained significant in two pollutant models that included SO2 or NO2, but not CO. It was difficult to obtain an accurate effect estimate in two-pollutant models including CO due to the high positive correlation between levels of PM10 and PM2.5 with CO. This could result in multicollinearity and lead to a distortion estimation, or make the effect difficult to assess accurately.
Our findings are in accordance with previous reports. Tsai et al. found increased numbers of admissions for pneumonia were significantly associated with higher PM2.5 levels both on warm days (>23°C) and on cool days (<23°C) in Taipei, Taiwan. This accompanied a 12% (95% CI = 13–10%) and 4% (95% CI = 3–6%) rise in the number of admissions for pneumonia per IQR elevation, respectively (Tsai and Yang, Citation2014). Negrisoli et al. revealed an acute effect of exposure to NO2 and a later effect of exposure to particulate matter on pediatric hospital admissions for pneumonia in Sorocaba, São Paulo, Brazil (Negrisoli and Nascimento, Citation2013). Souza et al. found a strong association between hospitalization and PM10 and NO2. The percentage increase in relative risk was 15% to PM10 in Lag 0 and 7% in Lag 1 for NO2 (Souza and Nascimento, Citation2016). Patto et al. reported that a rise of 10 µg/m3 in the concentration of PM2.5 leads to significant increase between 25 ppt and 28 ppt in the risk of hospitalization due to pneumonia among children 4 and 5 days after exposure (Patto et al., Citation2016). A Rome study conducted by Belleudi et al. demonstrated a 10-μg/m3 increment in the level of PM2.5 was correlated with a 2.82% (95% CI = 0.52–5.19%) increase in hospitalizations for lower-respiratory-tract infections, including acute bronchitis and pneumonia. César et al. showed that an increase of 5 µg/m3 in PM2.5 contributes to increasing the relative risk for hospitalization for pneumonia from 20.3 to 38.4 percentage points (César et al., Citation2016). Strickland et al. found a 10-µg/m3 increase in same-day PM2.5 concentrations was associated with ED visits for asthma or wheeze (OR = 1.013, 95% CI: 1.003, 1.023) and upper respiratory infections (OR = 1.015, 95% CI: 1.008, 1.022) in the U.S. state of Georgia (Strickland et al., Citation2015). Kan et al. demonstrated that an increase of 10 µg/m3 in 2-day moving average concentrations of PM10, SO2, NO2, and O3 corresponded to 0.26% (95% CI, 0.14–0.37), 0.95% (95% CI, 0.62–1.28), 0.97% (95% CI, 0.66–1.27), and 0.31% (95% CI, 0.04–0.58) increases, respectively, in daily mortality in Shanghai (Kan et al., Citation2010). Halonen et al. revealed associations between pneumonia over a 5-day mean (3.1%; 95% CI, 0.43–5.8), asthma–chronic obstructive pulmonary disease (COPD) at lag 0 (3.8%; 95% CI, 1.3–6.3), and respiratory mortality at lag 0 (5.1%; 95% CI, 1.2–9.0), with an interquartile range (IQR) increase in accumulation mode particles (0.1–0.29 µm) in Helsinki, Finland (Halonen et al., Citation2009). Tramuto et al. demonstrated a positive significant association between exposure to ambient levels of PM10 and ER visits for acute respiratory symptoms at lag 0 in Palermo, Sicily (Tramuto et al., Citation2011). It is important to note that, despite the similar findings, the hysteresis effect varied between our results and the aforementioned studies, as well as among those studies, possibly because of differences in the chemical components of the ambient pollution.
The current study revealed significant seasonality in the distributions of the concentrations of PM2.5, PM10, CO, NO2, and SO2 in Jinan, with pollutant concentrations increasing in the cold season and decreasing in the warm season. This is likely due to an increase in coal burning in the cold season in Jinan, and the development of a temperature inversion. In contrast to these findings, Jalaludin et al. found the concentrations of ambient air pollutants in Sydney, Australia, were greater in warm months (Jalaludin et al., Citation2008). These divergent findings may be explained by differences in geographic characteristics, human activities, and climatic conditions between cities.
Stratification analyses of our data showed that PM2.5/PM10 had adverse effects on children’s health in both cold and warm seasons but the effects were more pronounced in the warm season. Tramuto et al. reported similar findings, demonstrating that exposure to ambient levels of air pollution was a particularly important determinant of ER visits for acute respiratory symptoms during the warm season in Palermo, Sicily (Tramuto et al., Citation2011). Compared with cold seasons, in warmer weather children may be exposed to greater levels of ambient pollution as they spend more time exercising outdoors and rooms are ventilated more frequently. Our study also indicated that infants less than 1 year of age were most sensitive to the health effects of PM2.5/PM10. It is possible that the developing respiratory and immune system increase the risk of hospitalizations for pneumonia in infants. Children in the infancy stage inhale and retain larger amounts of atmospheric particulate matter per unit weight than at the preschool and post-preschool stage, and the air intake of a resting infant is twice or more that of an teenager. The organ systems are still growing with the growth of children, and the normal growth possible be affected by the exposure to atmospheric particulate matter in critical periods.
The analyses in this study used the case-crossover and general additive model approach, which are widely used for studying the impact of short-term exposures on acute disease and have provided similar data in previous studies (Maclure, Citation1991; Wang et al., Citation2015). The study design controlled for confounders such as weather and season, as all comparisons were made within the same month and on the same day of the week. The bidirectional case-crossover design in this study eliminated bias caused by temporal trends in exposures. However, this study had some limitations. First, we were unable to investigate the effects of potential confounding factors, such as the clinical characteristics and behaviors of the individual children, because this information was not readily available (Tramuto et al., Citation2011). Second, the results cannot be used as the basis of causal inference but only to suggest the relevance of pollutant exposure levels and their effects. Third, this study had a small sample size, short study duration, and few monitoring stations, and was conducted in a single hospital in Jinan city. At last, several studies have reported that indoor air pollution likely is a risk factor for childhood pneumonia; however, indoor air pollutants in the residences and in the kindergarten/school in this study also were not considered. Therefore, these data may not be generalizable to other health care settings in different regions.
In conclusion, short-term exposure to atmospheric particulate matter (PM2.5/PM10) may be a determinant of pediatric hospital admissions for pneumonia in Jinan, China, particularly in infant males during the warm season.
Funding
The authors gratefully acknowledge funding from the Science and Technology Planning Project of Shandong Province (No. 2015GSF118112).
Additional information
Funding
Notes on contributors
Chenguang Lv
Chenguang Lv is a member of the Biomedical Engineering Institute.
Xianfeng Wang
Xianfeng Wang is a research professor at the Department of Environment Protection of Shandong Province.
Na Pang
Na Pang is a research professor in the Department of Equipment in Jinan central hospital.
Lanzhong Wang
Lanzhong Wang is a research professor at PFLMET Experimental Center.
Yuping Wang
Yuping Wang, Tengfei Xu, Yu Zhang, and Tianran Zhou are members of Biomedical Engineering Institute.
Yu Zhang
Yuping Wang, Tengfei Xu, Yu Zhang, and Tianran Zhou are members of Biomedical Engineering Institute.
Tianran Zhou
Yuping Wang, Tengfei Xu, Yu Zhang, and Tianran Zhou are members of Biomedical Engineering Institute.
Wei Li
Wei Li is a research professor at the Biomedical Engineering Institute.
References
- Aguilera, I., Pedersen, M., Garcia-Esteban, R., Ballester, F., Basterrechea, M., Esplugues, A., et al. 2013. Early-life exposure to outdoor air pollution and respiratory health, ear infections, and eczema in infants from the INMA study.Environ. Health Perspect. 121:387–92.
- Anenberg, S.C., J. Schwartz, D. Shindell, M. Amann, G. Faluvegi, Z. Klimont, G. Janssens-Maenhout, L. Pozzoli, R. Van Dingenen, E. Vignati, et al. 2012. Global air quality and health co-benefits of mitigating near-term climate change through methane and black carbon emission controls. Environ. Health Perspect. 120(6):831–9. doi:10.1289/ehp.1104301
- Barnett, A.G., G.M. Williams, J. Schwartz, A.H. Neller, T.L. Best, A.L. Petroeschevsky, et al. 2005. Air pollution and child respiratory health: A case-crossover study in Australia and New Zealand. Am. J. Respir. Crit. Care Med. 171(11):1272–8. doi:10.1164/rccm.200411-1586OC
- Belleudi V., A. Faustini, M. Stafoggia, G. Cattani, A. Marconi, C.A. Perucci, et al. 2010. Impact of fine and ultrafine particles on emergency hospital admissions for cardiac and respiratory diseaes. Epidemiology 21:414–23. doi:10.1097/EDE.0b013e3181d5c021
- César, A.C., L.F. Nascimento, K.C. Mantovani, and L.C. Pompeo Vieira. 2016. Fine particulate matter estimated by mathematical model and hospitalizations for pneumonia and asthma in children. Rev. Paul Pediatr. 34(1): 18–23. doi:10.1016/j.rppede.2015.12.005
- Darrow, L.A., M. Klein, W.D. Flanders, J.A. Mulholland, P.E. Tolbert, and M.J. Strickland. 2014. Air pollution and acute respiratory infections among children 0–4 years of age: An 18-year time-series study. Am. J. Epidemiol. 180(10):968–77. doi:10.1093/aje/kwu234
- den Dekker, H.T., A.M. Sonnenschein-van der Voort, J.C. de Jongste, I. HAnessi-Maesano, S.H. Arshad, H. Barros, et al. 2016. Early growth characteristics and the risk of reduced lung function and asthma: A meta-analysis of 25,000 children. J. Allergy Clin. Immunol. 137(4):1026–35. doi:10.1016/j.jaci.2015.08.050
- Halonen, J.I., T. Lanki, T. Yli-Tuomi, P. Tiittanen, M. Kulmala, and J. Pekkanen. 2009. Particulate air pollution and acute cardiorespiratory hospital admissions and mortality among the elderly. Epidemiology 20:143–53. doi:10.1097/EDE.0b013e31818c7237
- Hales, N.M., C.C. Barton, M.R. Ransom, R.T. Allen, and C.A. Pope. 2016. A quasi-experimental analysis of elementary school absences and fine particulate air pollution. Medicine (Baltimore) 95(9):e2916. doi:10.1097/MD.0000000000002916
- Harrod, K.S., R.J. Jaramillo, C.L. Rosenberger, S.Z. Wang, J.A. Berger, J.D. McDonald, et al. 2003. Increased susceptibility to RSV infection by exposure to inhaled diesel engine emissions. Am. J. Respir. Cell Mol. Biol. 28(4):451–63. doi:10.1165/rcmb.2002-0100OC
- Jalaludin, B., B. Khalaj, V. Sheppeard, and G. Morgan. 2008. Air pollution and ED visits for asthma in Australian children: acase-crossover analysis. Int. Arch. Occup. Environ. Health 81(8):967–74. doi:10.1007/s00420-007-0290-0
- Kan, H., B. Chen, N. Zhao, S.J. London, G. Song, G. Chen, et al. 2010. A time-series study of ambient air pollution and daily mortality in Shanghai, China. Res. Rep. Health Effects Inst. 154:17–78.
- Kesavachandran, C., B.S, Pangtey, V. Bihari, M. Fareed, M.K. Pathak, A.K. Srivastava, et al. 2013. Particulate matter concentration in ambient air and its effects on lung functions among residents in the National Capital Region, India. Environ. Monit. Assess. 185(2):1265–72. doi:10.1007/s10661-012-2630-0
- Le, T.G., L. Ngo, S. Mehta, V.D. Do, T.Q. Thach, X.D. Vu, et al. 2012. Effects of short-term exposure to air pollution on hospital admissions of young children for acute lower respiratory infections in Ho Chi Minh City, Vietnam. Res. Rep. Health Effects Inst. 169:5–72.
- Liu, W., C. Huang, Y. Hu, Q. Fu, Z. Zou, C. Sun, et al. 2016. Associations of gestational and early life exposures to ambient air pollution with childhood respiratory diseases in Shanghai, China: A retrospective cohort study. Environ. Int. 92–93:284–93. doi:10.1016/j.envint.2016.04.019
- Lin, S., X. Liu, L.H. Le, and S.A. Hwang. 2008. Chronic exposure to ambient ozone and asthma hospital admissions among children. Environ. Health Perspect. 116(12):1725–30.
- Maclure, M. 1991. The case-crossover design: A method for studying transient effects on the risk of acute events. Am. J. Epidemiol. 133(2):144–53.
- Mikerov, A.N., R. Haque, X. Gan, X. Guo, D.S. Phelps, J. Floros, et al. 2008. Ablation of SP-A has a negative impact on the susceptibility of mice to Klebsiella pneumoniae infection after ozone exposure: Sex differences. Respir. Res. 9:77. doi: 10.1186/1465-9921-9-77
- Negrisoli, J., and L.F.C. Nascimento. 2013. Atmospheric pollutants and hospital admissions due to pneumonia in children. Rev. Paul. Pediatr. 31(4):501–6. doi:10.1590/S0103-05822013000400013
- Patto, N.V., L.F. Nascimento, K.C. Mantovani, L.C. Vieira, and D.S. Moreira. 2016. Exposure to fine particulate matter and hospital admissions due to pneumonia: Effects on the number of hospital admissions and its costs. Rev. Assoc. Med. Bras. (1992) 62(4):342–6. doi:10.1590/1806-9282.62.04.342
- Qiu, H., L.W. Tian, V.C. Pun, K.F. Ho, T.W. Wong, and I.T. Yu. 2014. Coarse particulate matter associated with increased risk of emergency hospital admissions for pneumonia in Hong Kong. Thorax 69(11):1027–33. doi:10.1136/thoraxjnl-2014-205429
- Santus, P., A. Russo, E. Madonini, L. Allegra, F. Blasi, S. Centanni, et al. 2012. How air pollution influences clinical management of respiratory diseases. A case-crossover study in Milan. Respir. Res. 13:95. doi:10.1186/1465-9921-13-95
- Shibata, T., J.L. Wilson, L.M. Watson, A. LeDuc, C. Meng, et al. 2014. Childhood acute respiratory infections and household environment in an eastern Indonesian urban setting. Int. J. Environ. Res. Public Health 11(12):12190–203. doi:10.3390/ijerph111212190
- Souza, LS., and L.F. Nascimento. 2016. Air pollutants and hospital admission due to pneumonia in children: A time series analysis. Rev. Assoc. Med. Bras. (1992) 62(2):151–6. doi:10.1590/1806-9282.62.02.151
- Strickland, M.J., H. Hao, X. Hu, H.H. Chang, L.A. Darrow, and Y. Liu. 2015. Pediatric emergency visits and short-term changes in PM2.5 Concentrations in the U.S. state of Georgia. Environ. Health Perspect. 124(5):690–6. doi:10.1289/ehp.1509856
- Tramuto, F., R. Cusimano, G. Cerame, M. Vultaggio, G.C.M. Maida, et al. 2011. Urban air pollution and emergency room admissions for respiratory symptoms: A case-crossover study in Palermo, Italy. Environ. Health 10:31. doi:10.1186/1476-069X-10-31
- Tsai, S.S., C.C. Chen, and C.Y. Yang. 2014. Short-term effect of fine particulate air pollution on daily mortality: A case-crossover study in a tropical city, Kaohsiung, Taiwan. J. Toxicol. Environ. Health A 77(8):467–77. doi:10.1080/15287394.2014.881247
- Tsai, S.S., and C.Y. Yang. 2014. Fine particulate air pollution and hospital admissions for pneumonia in a subtropical city: Taipei, Taiwan. J. Toxicol. Environ. Health A 77(4):192–201. doi:10.1080/15287394.2013.853337
- Wang, T., G.X. Li, J. Sun, N. Buys, H.M. Liu, M.F. Liu, et al. 2013. Association between ambient particulate matter and daily cause-specific mortality in Tanguu, Tianjin, Binhai New Area, China. Int. J. Environ. Health Res. 23(3):205–14. doi:10.1080/09603123.2012.713096
- Wang, X., W. Kindzierski, and P. Kaul. 2015. Comparison of transient associations of air pollution and AMI hospitalisation in two cities of Alberta, Canada, using a case-crossover design. BMJ Open 5(11):e009169. doi:10.1136/bmjopen-2015-009169
- World Health Organization. 2014a. Ambient (outdoor) air pollution database. http://www.who.int (accessed November 13, 2014).