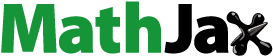
ABSTRACT
Road dust particles play an important role in atmospheric pollution and are associated with adverse human health effects. Traffic emissions are a major source of particles in road dust. However, there has been limited information about the relationship between distance from road and traffic-related elements levels in road dust. We investigated the relationships between proximity to the nearest major roadway and trace element mass fractions in PM10 and PM2.5 re-suspended from the road surface, based on measurements at three different distance ranges. We found that mass fractions of Ba, Cu, Zr, Zn, Cl, Co, Cr, Ca, Ti in PM10 road dust as well as Zr, Cu, Cl, Zn, Cr, Ti, Mn, Ca, Ni, and Fe in PM2.5 road dust, significantly decreased with distance from major road. Most of these elements are associated with road traffic emissions, including both tailpipe and non-tailpipe emissions. The decrease rates differed among elements due to differences in local traffic contributions. The decreases for elements which are mainly associated with non-tailpipe traffic emissions (e.g., Ba, Zr) were more dramatic. Our results indicate that traffic emissions, especially non-tailpipe emissions, contribute substantially to road dust, suggesting the need for control strategies for non-tailpipe emissions.
Implications: We investigated the relationships between road proximity with trace element mass fractions in PM10 and PM2.5 re-suspended from the road surface. We observed significant decrease of traffic-related elements in PM10 and PM2.5 road dust with log distance from major road. We also found that the mass fractions for elements, which mainly come from traffic decrease more sharply compared to elements which come from both traffic and other sources. Our results indicate that traffic emissions contribute substantially to road dust, and imply that the distance to major road can be used as a proxy for ambient exposure.
Introduction
Road dust is a complex mixture of particles that contain various chemical components such as organic, inorganic, and trace elements (Nakajima and Aryal Citation2018). Road dust commonly contains high concentrations of trace contaminants, which originate from road traffic (including tailpipe emissions from combustion of fuel and lubricating oil additives and non-tailpipe emissions from brake wear, tire wear, road surface wear), crustal material (such as soil and sand), road salt, vegetation debris, and so on. (Gunawardana et al. Citation2012; Hwang et al. Citation2018). For example, a considerable proportion of Zn, Cu, Pb, Ni, Cr, and Cd partly originate from road traffic; Si, Ca, and Al originate from soil; Na and Cl mainly originate from road salt (Gunawardana et al. Citation2012; Ho et al. Citation2003; Johansson, Norman, and Burman Citation2009).
Particulate matter (PM) exposure has been proved to be associated with increases in morbidity and mortality (Gilmour et al. Citation2007). Particulate matter (PM) is defined as an air-suspended mixture of a variety of chemical species (e.g., Water-Soluble Inorganic Ions [WSIIs], Organic Carbon [OC], Elemental Carbon [EC], crustal, and trace elements) (Samek et al. Citation2017). Hundreds of epidemiological studies have shown that exposure to PM is associated with increases in morbidity and mortality (Gilmour et al. Citation2007). The toxicity of PM is influenced by its sizes and compositions (Li et al. Citation2019). Road dust is typically re-suspended into the air, due to motion of vehicles and wind. As an important source of atmospheric PM (Li et al. Citation2018), road dust also has been proved to be significantly associated with adverse health effects (Khan and Strand Citation2018). Exposures to road dust particles have adverse effects on the respiratory system (Barrett et al. Citation2010; Maher Citation2010; Saini-Eidukat and Triplett Citation2014; Weissman and Kiefer Citation2011). PM2.5 in road dust have been found to be related to the increase of cardiovascular-related hospital admissions and mortalities (Kioumourtzoglou et al. Citation2014). Ba, Pb, and Cu in road dust are associated with non-carcinogenic health risks (Li et al. Citation2015). Ar in road dust has both carcinogenic and non-carcinogenic effects on humans (Tchounwou, Patlolla, and Centeno Citation2003).
According to existing studies, road traffic has significant influence on the resuspension of road dust, including PM2.5 and PM10 road dust (Amato et al. Citation2016; Birmili et al. Citation2006; Bukowiecki et al. Citation2010; Duong and Lee Citation2011; Harrison et al. Citation2012; Jeong et al. Citation2019; Ntziachristos et al. Citation2007). These studies indicated that traffic volume, vehicle speed, type of traffic emission (exhaust emission and non-exhaust emission) were influencing factors of contamination levels in road dust. Road proximity have also been recognized as an important predictor of ambient PM exposure (Dorans et al. Citation2016; Wilker et al. Citation2015). Studies also indicated that contaminants levels in road dust decreased with the increase of distances from major road (Hitchin et al. Citation2000; Pagotto et al. Citation2001).
In this study, our aim was to investigate the relationships between distance from the closest major road and the mass fractions of traffic-related trace elements (including Na, Mg, Al, Si, S, Cl, K, Ca, Ti, V, Cr, Mn, Fe, Co, Ni. Cu, Zn, Br, Sr, Zr, Sn, Sb, Ba, and Pb) in re-suspended road dust particles, including those with diameters that are below 10 µm (PM10) and 2.5 µm (PM2.5, fine particles). Our analysis was based on 78 PM10 and 79 PM2.5 re-suspended from the road surface, which were collected at different distance ranges from 20 major roads in the Greater Boston, Massachusetts area.
Materials and methods
We conducted a field study from June 2018 to January 2019 in the Greater Boston area to examine the relationships between road proximity and trace elements mass fractions in PM10 and PM2.5 road dust at different sites, including roadside (Road), near-road and background (Back) sites, with different distances from the closest major roads. The roadside site is at the side of the major road. The near-road and background sites are on small, low traffic roads, which were often residential but at different distance ranges from the major road. The distance ranges from the center of the nearest major road to the sampling sites were: ≤25 m for roadside site, 26–200 m for near-road site, and >300 m for background.
Road proximity
We put the coordinates in the “Google Earth Pro” software and measured the perpendicular distance (d in meters) from sampling site to center of the closest major road. The accuracy of this method is 1.7 m (Mohammed, Ghazi, and Mustafa Citation2013). We included three types of major roads from the U.S. Census Feature Class Codes: A1 road, a primary road with limited access via on ramps and exit ramps; A2 road, a primary road without limited access; and A3 road, a secondary or connecting road. For this study, we did not consider differences in elevation because they are small compared to distance variability (Huang et al. Citation2018).
Measurement of trace elements concentrations in PM10 and PM2.5 from road dust
We developed a novel Road Dust Aerosolizer (RDA) sampler to collect PM10 and PM2.5 re-suspended from surface road dust (PM10 and PM2.5 road dust). This sampler aerosolizes dust from the road surface, simulating ambient road dust resuspension, resulting in measured PM composition and size more appropriately (and less labor-intensive) than those obtained from studies using bulk road dust sieved and re-suspended in the laboratory. Our results showed that, for samples with elements that were well above the lower detection limit (LOD), the relative error for mass was 7% for PM10 and 3% for PM2.5 within pairs and ranged from 2 to 10% for elements, indicating that the RDA system has an excellent precision for mass and elements.
During this study, we collected samples of PM2.5 and PM10 dust re-suspended from the road surface at a series of three distances away from major road sites in the Greater Boston area: roadside, near-road, and local background (as described in the introduction). In order to avoid the impact of precipitation, we did not collect samples during rainy days. Major roadways sampled included multi-lane divided state and interstate highways (with<A1/> or without<A2/> limited access via onramps and exit ramps) and busy state secondary and connecting roads <A3/>. Near-road and background samples were collected at a location on an adjacent roadway within the target distance from the roadside site. Near-road samples were collected on roads in residential or commercial areas, while background samples were almost entirely on residential roads. Some locations were sampled more than once, during different seasons. The distribution of sampling sites shown in . Details of each sampling day including the road ID, date, and number of sampling sites are shown in Table S1 in Supplementary Material. We had repeated measurements for 6 major roads. Finally, we had 27 sampling days for 20 major roads at 62 sites, including 78 PM10 samples and 79 PM2.5 samples. We used energy dispersive X-ray fluorescence (EDXRF) spectrometry to analyze samples for 23 elements, including Na, Mg, Al, Si, S, Cl, K, Ca, Ti, V, Cr, Mn, Fe, Co, Ni. Cu, Zn, Br, Sr, Zr, Sn, Sb, Ba, and Pb. Uncertainty was determined by repeating a measurement to arrive at an estimate of the standard deviation of the values. LOD is 3 times of uncertainty (Kang et al. Citation2014). In our data analysis, we excluded elements for which the uncertainty was greater than the measurement in more than 20% of samples. The concentration of each element was expressed as mass fraction, that is, the mass of an element (μg) divided by PM10 or PM2.5 road dust mass (mg).
Other covariates
We examined the effects of season in our primary model. We considered June through September as the warm season and October through January as the cold season. In order to examine whether elemental fraction depends on road type, we examined the effect of road type as well as the interaction of road type and proximity. In order to increase statistical power, we combined A1 and A2 as primary roads (A12), as a result we developed statistical models for two road types (A12 and A3).
Statistical analysis
We used a mixed-effects regression model (package with “lme4” and “lmerTest” in R software, version 3.3.2, The R Project; https://www.r-project.org/) to examine the relationships between each of the trace element mass fractions in PM10 and PM2.5 with road proximity. We used linear mixed-effects models as we had repeated measurements for each major road (road, near-road, and background samples collected on the same day) on a given day defined as experiment i. We used the following model:
where Cij is the mass fraction of element j for experiment i; β0j is the fixed intercept; γij is the random intercept for experiment i; lndij is the log value of perpendicular distance of the sampling site to the nearest major road (meters); rt is the road type (rt = 1 for A12 roads, while rt = 0 for A3 roads); β1j is the slope of an element j for lndij; β2j is the slope for season (season = 1 for warm season, while season = 0 for cold season); β3j is the slope for road type; and eij is the error term.
Before we determined our final model, we also considered meteorology factors including wind direction (including upwind, downwind, and parallel wind), wind speed during sample period, and daily temperature. For each road, wind speed and direction were measured using a Davis Vantage Vue Weather Station at the roadside location. The daily temperature is from National Centers for Environmental Information (NOAA) (https://www.ncdc.noaa.gov/). However, we found no significant relationship between wind direction or wind speed with the mass fraction of elements. Therefore, we did not include these factors in our statistic model.
We considered a relationship as significant when p < .05.
Results
Mass fractions of trace elements
Table S2 shows the mass fractions of selected trace elements in 78 PM10 and 79 PM2.5 samples of re-suspended road dust, separated by columns representing different distance ranges from the nearest major road. We conducted paired sample t-tests to compare the differences of elements fraction masses in PM10 and PM2.5. The p-values of Welch’s t-tests for Na, Mg, Al, Si, K, Cr, Fe, Ni, Cu, Zn, and Br are less than 0.05 as shown in Table S3, indicating the mass fractions for these elements in PM2.5 were significantly higher than those in PM10. The main reason for this is that the PM10 has proportionally more soil particles (Hueglin et al. Citation2005), and therefore, the toxic elemental fractions are higher for the PM2.5 samples. The mass fractions of selected trace elements in cold and warm seasons are shown in Tables S4 and S5, respectively, in the Supplementary Material. shows the mass fractions of 6 elements (i.e., Ba, Ca, Cu, Fe, Zn, and Zr) at different distance ranges.
Figure 2. Mass fractions of Ba, Ca, Cu, Fe, Zn and Zr at different distance ranges
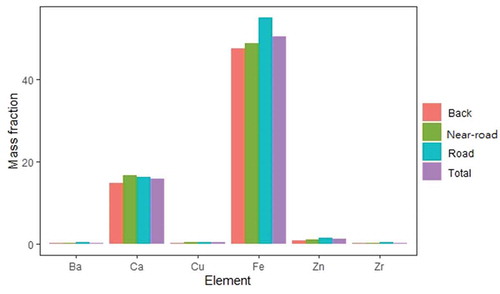
Percentages of the elements samples above the uncertainty are shown in Table S6 in the Supplementary Material. Our model only included elements for which the element measurement was greater than sample uncertainty for at least 80% of the samples. Based on these criteria, we excluded Br and Sn in the PM10 samples, and Br, Sn, and Ba in the PM2.5 samples.
Distribution of road proximity
The range of distances from the nearest major road ranged from 2 to 1,250 m. About 1/3 of the samples were collected at the roadside, within 25 m of the nearest major road. The average (SD, standard deviation) values from sites to major roads were 282 m (352 m). The average (SD) values for road, near-road, and background distances were 10.4 m (6.5 m), 113 m (51.0 m), and 745 m (237 m), respectively.
Associations between mass fractions of trace elements and road proximity
shows the regression coefficients of ln(road distance) for each of the elements in PM10 and PM2.5 road dust. Eleven elements (Cu, Zr, Zn, Cl, Co, Cr, Pb, Ca, Ti, Fe, and Ba) in PM10 road dust and 10 elements (Zr, Cu, Zn, Cl, Cr, Mn, Ti, Ca, Ni, and Fe) in the PM2.5 road dust have significantly negative associations with the log distance from major roads (trace elements are presented from the highest to lowest |β1i|). The decrease in mass fraction of these elements may reflect both a decreased contribution of re-suspended dust from the main roadway and the decreased traffic at the near-road and background sites compared to the major road.
Table 1. Regression coefficients of log road distance for each of the trace elements in PM10 and PM2.5 from road dust
Most of the above elements are mostly or partially originated from road traffic emissions: Ca originates from soil as well as the combustion of motor oil additives; Mn is mainly due to aged road dust and gasoline additive (MMT) (anti-knock agent) (Cadle et al. Citation1997; Dongarra, Manno, and Varrica Citation2009; Garg et al. Citation2000; Gertler et al. Citation2002; Hjortenkrans et al. Citation2003; Kam et al. Citation2012; Lough et al. Citation2005); Fe is associated with brake, tire, engine wear (Cadle et al. Citation1997; Garg et al. Citation2000; Harrison et al. Citation2012; Kam et al. Citation2012; Lough et al. Citation2005); Zn is associated with brake wear, tire wear, and tailpipe emissions of motor oil (specifically from zinc dithiophosphate, an anti-wear and antioxidant compound) (Cadle et al. Citation1997; Councell et al. Citation2004; Garg et al. Citation2000; Hjortenkrans, Bergback, and Haggerud Citation2003; Huang, Olmez, and Aras Citation1994; Lough et al. Citation2005); Cu is associated with brake wear, brake lining, vaporization from hot brake surfaces and wear of bearings or other components, and motor/lube oil combustion (antioxidant additive) (Cheung et al. Citation2010; Kaiser Citation2003; Lytle, Smith, and McKinnon Citation1995; Sternbeck, Sjodin, and Andreasson Citation2002); Zr comes primarily from brake dust (Adachi and Tainosho Citation2004; Garg et al. Citation2000), and can be effectively bound to humic substances in road dust with a preference for complexation with low molecular weight compounds; Ba is mostly due to brake wear and lining (Garg et al. Citation2000). Pb originates from motor vehicle wheel weights, motor oil combustion, brake wear, and vaporization from hot brake surfaces (Cadle et al. Citation1997; Garg et al. Citation2000; Gertler et al. Citation2002; Kam et al. Citation2012; Lough et al. Citation2005; Ning et al. Citation2008; Root Citation2000); Cl mostly originates from sea salt and road salt (deicing salt in winter season) (Ayers et al. Citation1999; Jang et al. Citation2007), and is also related to Motor vehicle emission (Bourotte et al. Citation2011). Ni comes mainly from oil combustion, and partially originates from automotive batteries (Jang et al. Citation2007; Kelly, Dai, and Wang Citation2020).
Different values of regression coefficients (β1j in EquationEquation (1)(1)
(1) ) as shown in indicate that the decreases of mass fractions for different elements in PM2.5 or PM10 with distance from major road are different. The mass fractions of elements which mainly come from traffic (e.g., Cu and Zn) decrease more sharply with distance from the major road compared to elements (e.g., Fe and Ti) which come from both traffic and additional source (i.e., soil), both in PM10 and PM2.5 road dust. show the relationship between log value of relative mass fraction decreases for Fe, Ti, Cu, Zn, Zr, and Ba in PM10 and Fe, Ti, Cu, Zn, Zr in PM2.5 road dust with the perpendicular distance from the nearest A12 road in warm season. show the relationship between log value of relative mass fraction decreases with the perpendicular distance from the nearest A12 road in cold season.
Figure 3. The relationship between log value of relative mass fraction decreases for elements in PM10from road dust and the perpendicular distance from the nearest A12 road in warm season. (a) Fe, (b) Ti, (c) Cu, (d) Zn, (e) Zr, (f) Ba
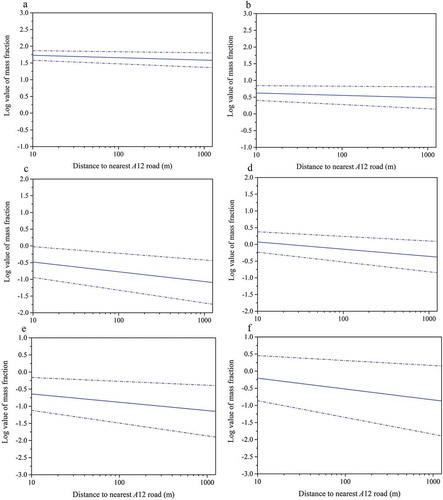
Figure 4. The relationship between log value of relative mass fraction decreases for elements in PM2.5from road dust and the perpendicular distance from the nearest A12 road in warm season. (a) Fe, (b) Ti, (c) Cu, (d) Zn, (e) Zr
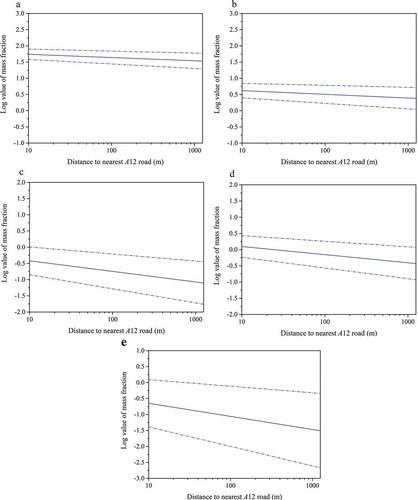
No significant relationships were found for elements mostly associated with other sources with distance from major road, in either PM10 or PM2.5. These elements include: Na (mostly from soil, and road and sea salt), Mg (from soil, ocean, and combustion of motor oil additives: detergent additives), Si (soil), K (from soil and wood burning), V (from oil combustion), and Sr (from crustal) (Cadle et al. Citation1997; Garg et al. Citation2000; Gertler et al. Citation2002; Huang et al. Citation2018; Kam et al. Citation2012; Lough et al. Citation2005; Ning et al. Citation2008; Root Citation2000).
Effect of season
The coefficients by season (β2j in EquationEquation (1)(1)
(1) ) are shown in Table S7 in the Supplementary Material. These results show that road dust mass fractions of Ti, Cr, Fe, Ni, Cu, Zn, Zr, and Pb in PM10 and Ti, Cr, Fe, Co, Ni, Cu, and Zr in PM2.5 were significantly higher in warm season than those in cold season. We found the same results for ambient PM elements and EC/OC. It rains less during warm season. During our experiment period, the average precipitation is 12.3 cm in cold season and 10.9 cm in warm season, according to data from National Centers for Environmental Information (NOAA) (https://www.ncdc.noaa.gov/). Therefore, the elements associated with traffic accumulate faster than during the cold season.
Effects of road type
The coefficients by road type (β3j in EquationEquation (1)(1)
(1) ) are summarized in Table S8 in the Supplementary Material. The coefficients of V, Fe, Co, Cu, and Zn in PM10 road dust and Cu and Zn in PM2.5 road dust are significant and positive, indicating that the mass fractions of these elements at the site close to A12 roads are higher than those close to A3 roads. The main reason is that these elements are associated with traffic, and A12 roads have larger traffic density compared to A3 roads. However, it shows no significant difference between A12 and A3 roads for ambient PM elements.
Discussion
In this study, we investigated the influence of road proximity on the mass fractions of 20 elements in 78 PM10 and 79 PM2.5 samples of road dust, which were collected at 62 sites with different distances from 20 major roadways in the greater Boston area. We found that mass fractions of Ba, Cu, Zr, Zn, Cl, Co, Cr, Pb, Ca, Ti, and Fe in the PM10 road dust; and, Zr, Cu, Cl, Zn, Cr, Ti, Mn, Ca, Ni, and Fe in the PM2.5 road dust decreased significantly with increasing distance from major road. Most of these elements are associated with road traffic emissions.
The mass fractions of elements decrease differently with distance from major road for elements from different sources. According to our results, the mass fractions of elements which mainly come from traffic (e.g., Cu and Zn), decrease more sharply with distance from the major road compared to elements which come from both traffic and soil (e.g., Fe and Ti). Elements mainly associated with other sources, such as Na, Mg, Si, K, V, and Sr are not significantly related to the distance from the major road. These results were similar to those reported for samples of ambient air coarse and fine PM collected during this study. Our results verify that, as expected, Cu and Zn are the most reliable elemental tracers of road traffic indirect emissions.
We also found that season affect the mass fractions of elements in PM10 and PM2.5 road dust. Ti, Cr, Fe, Ni, Cu, Zn, Zr, and Pb in PM10 and Ti, Cr, Fe, Co, Ni, Cu, and Zr in PM2.5 were significantly higher in warm season. The possible explanation is that the increased wintertime precipitation reduces the resuspension of the road dust (Amato et al. Citation2012), and the compressed atmospheric mixing height leads to increases in their concentrations (Wagner and Schäfer Citation2017). Opposite trends have been observed in other more arid areas like Los Angeles (Pakbin et al. Citation2009).
Furthermore, our results show that for V, Fe, Co, Cu, and Zn in PM10 road dust and Cu and Zn in PM2.5 road dust, the mass fractions at the site closer to A12 roads are significantly higher than for those closer to A3 roads. This is expected since the traffic emissions are generally higher on A12 roads than A3 roads, which typically feature higher traffic speed and density.
Elements primarily associated with soil and crustal materials (Al, Si, K) do not show a distance-related decrease in mass fraction in either the PM2.5 or PM10 road dust. In fact, they show a slight borderline-significant increase, suggesting an increased contribution of soil and a decreased contribution of traffic-derived road dust in the road surface dust sampled at the near-road and background sites compared to the major road. Furthermore, other elements mostly associated with non-traffic related sources (Na, Mg, Si, K, V, and Sr) were not significantly associated with distance from road in either PM2.5 or PM10 (Cadle et al. Citation1997; Garg et al. Citation2000; Gertler et al. Citation2002; Huang et al. Citation2018; Kam et al. Citation2012; Lough et al. Citation2005; Ning et al. Citation2008; Root Citation2000).
Our study has some limitations. Our sample size was smaller than desired due to several factors, including difficulty in scheduling use of road sites where permission was required for access (especially in commercial areas), weather conditions (the roads had to be dry to collect dust from the road surface), and costs. Some collected road dust samples were too heavily loaded to be analyzed by EDXRF, and thus were not included in the data analysis. The resulting small sample size limited statistical power to investigate other possible influencing factors, such as other traffic or roadway characteristics, land use, and meteorological variables. However, even with a small sample size, we were able to observe significant relationships between distance from major road and traffic-related element mass fractions in PM10 and PM2.5 road dust. In addition, our results were based on experiments in Boston area, which may not be generalizable to other geographical areas with different weather.
Our study also has several strengths. We have developed a new method to collect PM10 and PM2.5 re-suspended from surface road dust, which results in a more realistic measurement of PM composition and size. To the best of our knowledge, this is the first study to include a wide range of traffic-related elements in both PM10 and PM2.5 road dust collected at different distances from major roads. Even with a small dataset, we observed significantly negative associations between mass fractions of traffic-related elements in PM2.5 and PM10 road dust with the log distance from major road.
Conclusion
We collected 78 PM10 and 79 PM2.5 samples of road dust collected at 62 sites with different distances from 20 major roads in the Boston area. Using a linear mixed effects model, we observed significant decrease of traffic-related elements in PM10 and PM2.5 road dust with log distance from major road. We also found that the mass fraction for elements, which mainly come from traffic, including the tailpipe emission and non-tailpipe emission, decrease more sharply with distance from the major road compared to elements which come from both traffic and other sources. In addition, elements associated with soil and crustal material do not decrease with distance to major roads; in fact, the elements associated with crustal materials show a small and borderline significant increase in their contribution to PM2.5 and PM10 road dust. Our results indicate that traffic emissions contribute substantially to road dust. As road dust plays an important role in atmospheric pollution, our results imply that the distance to major road can be used as a proxy for ambient exposure, and therefore, it is a reasonable exposure metric in epidemiological studies. Over the last decades, strict tailpipe emission controls from governmental agencies and technological upgrades have been pushing the automotive industry to a significant reduction of exhaust emissions (Allen et al. Citation2006). Our results implicated that government and future research should also pay particular attention to non-tailpipe emissions.
Supplemental Material
Download MS Word (1.3 MB)Acknowledgment
Research described in this article was conducted under contract to the Health Effects Institute (HEI), an organization jointly funded by the United States Environmental Protection Agency (U.S. EPA) (Assistance Award No. CR-83467701) and certain motor vehicle and engine manufacturers. The contents of this article do not necessarily reflect the views of HEI, or its sponsors, nor do they necessarily reflect the views and policies of the U.S. EPA or motor vehicle and engine manufacturers. This publication was made possible by U.S. EPA grant RD-83587201. Its contents are solely the responsibility of the grantee and do not necessarily represent the official views of the U.S. EPA. Further, U.S. EPA does not endorse the purchase of any commercial products or services mentioned in the publication.
Disclosure statement
The authors do not have any competing financial interests, including relevant financial interests, activities, relationships, and affiliation.
Supplementary material
Supplemental data for this article can be accessed on the publisher’s website.
Additional information
Funding
Notes on contributors
Shaodan Huang
Shaodan Huang is Ph.D., Research fellow, Department of Environmental Health, Harvard T.H. Chan School of Public Health, Boston, MA, USA. Research area: Indoor Air Quality, Ambient Air Pollution, Health Effect of Air Pollution.
Pablo Taddei
Pablo Taddei is Master student, Department of Environmental Health, Harvard T.H. Chan School of Public Health, Boston, MA, USA. Research area: Population Health Science.
Joy Lawrence
Joy Lawrence is Research associate, Department of Environmental Health, Harvard T.H. Chan School of Public Health, Boston, MA, USA. Research area: Air Pollution, Ambient Environment.
Marco A.G. Martins
Marco A.G. Martins is Ph.D., Research associate, Department of Environmental Health, Harvard T.H. Chan School of Public Health, Boston, MA, USA. Research area: Air Pollution, Ambient Environment.
Jing Li
Jing Li is Ph.D., Research fellow, Department of Environmental Health, Harvard T.H. Chan School of Public Health, Boston, MA, USA. Research area: Ambient Air Pollution, Atmospheric Chemistry.
Petros Koutrakis
Petros Koutrakis is Ph.D., Professor, Department of Environmental Health, Harvard T.H. Chan School of Public Health, Boston, MA, USA. Research area: Air Pollution and its Health Effects.
References
- Adachi, K., and Y. Tainosho. 2004. Characterization of heavy metal particles embedded in tire dust. Environ. Int. 30:1009–17. doi:10.1016/j.envint.2004.04.004.
- Allen, J. O., O. Alexandrova, K. E. Kaloush, and I. A. Fulton. 2006. Tire wear emissions for asphalt rubber and Portland cement concrete pavement surfaces. Final Report, Arizona State University
- Amato, F., O. Favez, M. Pandolfi, A. Alastuey, X. Querol, S. Moukhtar, B. Bruge, S. Verlhac, J. A. G. Orza, N. Bonnaire, et al. 2016. Traffic induced particle resuspension in Paris: Emission factors and source contributions. Atmos. Environ. 129:114–24. doi:10.1016/j.atmosenv.2016.01.022.
- Amato, F., M. Schaap, H. A. C. Denier van der Gon, M. Pandolfi, A. Alastuey, M. Keuken, and X. Querol. 2012. Effect of rain events on the mobility of road dust load in two Dutch and Spanish roads. Atmos. Environ. 62:352–58. doi:10.1016/j.atmosenv.2012.08.042.
- Ayers, G. P., R. W. Gillett, J. M. Cainey, and A. L. Dick. 1999. Chloride and bromide loss from sea-salt particles in Southern Ocean Air. J. Atmos. Chem. 33:299–319. doi:10.1023/A:1006120205159.
- Barrett, J. E., K. G. Taylor, K. A. Hudson-Edwards, and J. M. Charnock. 2010. Solid-phase speciation of Pb in urban road dust sediment: A XANES and EXAFS study. Environ. Sci. Technol. 44:2940–46. doi:10.1021/es903737k.
- Birmili, W., A. Allen, F. Bary, and R. M. Harrison. 2006. Trace metal concentrations and water solubility in size-fractionated atmospheric particles and influence of road traffic. Environ. Sci. Technol. 40:1144–53.
- Bourotte, C. L. M., O. R. Sanchéz-Ccoyllo, M. C. Forti, and A. J. Melfi. 2011. Chemical composition of atmospheric particulate matter soluble fraction and meteorological variables in São Paulo state, Brazil. Rev. Bras. Meteorologia 26 (3):419–32. doi:10.1590/S0102-77862011000300008.
- Bukowiecki, N., P. Lienemann, M. Hill, M. Furger, A. Richard, F. Amato, A. S. H. Prévôt, U. Baltensperger, B. Buchmann, and R. Gehrig. 2010. PM10 emission factors for non-exhaust particles generated by road traffic in an urban street canyon and along a freeway in Switzerland. Atmos. Environ. 44 (19):2330e2340. doi:10.1016/j.atmosenv.2010.03.039.
- Cadle, S. H., P. A. Mulawa, J. Ball, C. Donase, A. Weibel, J. C. Sagebiel, K. T. Knapp, and R. Snow. 1997. Particulate emission rates from in-use high-emitting vehicles recruited in Orange County, California. Environ. Sci. Technol. 31:3405–12. doi:10.1021/es9700257.
- Cheung, K. L., L. Ntziachristos, T. Tzamakiozis, J. J. Schauer, Z. Samaras, K. F. Moore, and C. Sioutas. 2010. Emissions of particulate trace elements, metals and organic species from gasoline, diesel, and biodiesel passenger vehicles and their relation ot oxidative potential. Aerosol Sci. Technol. 44:500–13. doi:10.1080/02786821003758294.
- Councell, T. B., K. U. Duckenfield, R. R. Landa, and E. Callender. 2004. Tire-wear particles as a source of zinc to the environment. Environ. Sci. Technol. 38:4206–14. doi:10.1021/es034631f.
- Dongarra, G., E. Manno, and D. Varrica. 2009. Possible markers of traffic-related emissions. Environ. Monit. Assess. 154:117–25. doi:10.1007/s10661-008-0382-7.
- Dorans, K. S., E. H. Wilker, W. Y. Li, M. B. Rice, P. L. Ljungman, J. Schwarz, B. A. Coull, I. Kloog, P. Koutrakis, R. B. D’Agostino, et al. 2016. Residential proximity to major roads, exposure to fine particulate matter and coronary artery calcium: The framingham heart study. Arterioscler Thromb Vasc. Biol. 36 (8):1679–85. doi:10.1161/ATVBAHA.116.307141.
- Duong, T. T., and B. K. Lee. 2011. Determining contamination level of heavy metals in road dust from busy traffic areas with different characteristics. J. Environ. Manage. 92 (3):554–62. doi:10.1016/j.jenvman.2010.09.010.
- Garg, B., S. H. Cadle, P. A. Mulawa, P. J. Groblicki, C. Laroo, and G. A. Parr. 2000. Brake wear particulate matter emissions. Environ. Sci. Technol. 34:4463–69. doi:10.1021/es001108h.
- Gertler, A. W., J. A. Gillies, W. R. Pierson, C. F. Rogers, J. C. Sagebiel, M. Abu-Allaban, W. W. Coulombe, L. Tarnay, and T. A. Cahill. 2002. Emissions from diesel and gasoline engines measured in highway tunnels. Health Effects Institute Research Report 107. Montpelier VT: Capital City Press.
- Gilmour, M. I., J. McGee, R. M. Duvall, L. Dailey, M. Daniels, E. Boykin, S. H. Cho, D. Doerfler, T. Gordon, and R. B. Devlin. 2007. Comparative toxicity of size-fractionated airborne particulate matter obtained from different cities in the United States. Inhal. Toxicol. 19 (sup1):7–16. doi:10.1080/08958370701490379.
- Gunawardana, C., A. Goonetilleke, P. Egodawatta, L. Dawes, and S. Kokot. 2012. Source characterisation of road dust based on chemical and mineralogical composition. Chemosphere 87 (2):163–70. doi:10.1016/j.chemosphere.2011.12.012.
- Harrison, R. M., A. M. Jones, J. Geitl, J. Yin, and D. C. Green. 2012. Estimation of the contributions of brake dust, tire wear and resuspension to nonexhaust traffic particles derived from atmospheric measurements. Environ. Sci. Technol. 46:6523–29. doi:10.1021/es300894r.
- Hitchin, J., L. Morawska, R. Wolff, and D. Gilbert. 2000. Concentrations of submicrometre particles from vehicle emissions near a major road. Atmos. Environ. 34:51–59. doi:10.1016/S1352-2310(99)00304-0.
- Hjortenkrans, D. S. T., B. G. Bergback, and A. V. Haggerud. 2003. Metal emissions from brake linings and tires: Care studies of Stockholm, Sweden 1995/1998 and 2005. Environ. Sci. Technol. 41:5224–30. doi:10.1021/es070198o.
- Ho, K. F., S. C. Lee, J. C. Chow, and J. G. Watson. 2003. Characterization of PM10 and PM2.5 source profiles for fugitive dust in Hong Kong. Atoms. Environ. 37:1023–32.
- Huang, S., J. Lawrence, C. M. Kang, J. Li, M. Martins, P. Vokonas, D. R. Gold, J. Schwartz, B. A. Coull, and P. Koutrakis. 2018. Road proximity influences indoor exposures to ambient fine particle mass and components. Environ. Pollut. 243 (Pt B):978–87. doi:10.1016/j.envpol.2018.09.046.
- Huang, X., I. Olmez, and N. K. Aras. 1994. Emissions of trace elements from motor vehicles: Potential marker elements and source composition profile. Atmos. Environ. 28:1385–91. doi:10.1016/1352-2310(94)90201-1.
- Hueglin, C., R. Gehrig, U. Baltensperger, M. Gysel, C. Monn, and H. Vonmint. 2005. Chemical characterisation of PM2.5, PM10 and coarse particles at urban, near-city and rural sites in Switzerland. Atmos. Environ. 39:637–51. doi:10.1016/j.atmosenv.2004.10.027.
- Hwang, H. M., M. J. Fiala, T. L. Wade, and D. Park. 2018. Review of pollutants in urban road dust: Part II. Organic contaminants from vehicles and road management. Int. J. Urban Sci. 23 (4):445–63. doi:10.1080/12265934.2018.1538811.
- Jang, H. N., Y. C. Seo, J. H. Lee, K. W. Hwang, J. I. Yoo, C. H. Sok, and S. H. Kin. 2007. Formation of fine particles enriched by V and Ni from heavy oil combustion: Anthropogenic sources and drop-tube furnace experiments. Atmos. Environ. 41 (5):1053–63. doi:10.1016/j.atmosenv.2006.09.011.
- Jeong, C. H., J. M. Wang, N. Hilker, J. Debosz, U. Sofowote, Y. Su, M. Noble, R. M. Healy, T. Munoz, E. Dabek-Zlotorzynska, et al. 2019. Temporal and spatial variability of traffic-related PM2.5 sources: Comparison of exhaust and non-exhaust emissions. Atmos. Environ. 198:55–69. doi:10.1016/j.atmosenv.2018.10.038.
- Johansson, C., M. Norman, and L. Burman. 2009. Road traffic emission factors for heavy metals. Atoms. Environ. 43:4681–88. doi:10.1016/j.atmosenv.2008.10.024.
- Kaiser, J. 2003. A high-octane dispute. Science 300:926–28. doi:10.1126/science.300.5621.926.
- Kam, W., J. W. Liacos, J. J. Schauer, R. J. Delfino, and C. Sioutas. 2012. On-road emission factors of PM pollutants for light-duty vehicles (LDVs) based on urban street driving conditions. Atmos. Environ. 61:378–86. doi:10.1016/j.atmosenv.2012.07.072.
- Kang, C. M., S. Achilleos, J. Lawrence, J. M. Wolfson, and P. Koutrakis. 2014. Interlab comparison of elemental analysis for low ambient urban PM2.5 levels. Environ. Sci. Technol. 48:12150–56. doi:10.1021/es502989j.
- Kelly, J. C., Q. Dai, and M. Wang. 2020. Globally regional life cycle analysis of automotive lithium-ion nickel manganese cobalt batteries. Mitig. Adapt. Strat. Gl. 25:371–96. doi:10.1007/s11027-019-09869-2.
- Khan, R. K., and M. A. Strand. 2018. Road dust and its effect on human health: A literature review. Epidemiol. Health 40:e2018013. doi:10.4178/epih.e2018013.
- Kioumourtzoglou, M. A., B. A. Coull, F. Dominici, P. Koutrakis, J. Schwartz, and H. Suh. 2014. The impact of source contribution uncertainty on the effects of source-specific PM2.5 on hospital admissions: A case study in Boston, MA. J. Expo. Sci. Environ. Epidemiol. 24:365–71. doi:10.1038/jes.2014.7.
- Li, J., H. X. Chen, X. Y. Li, M. F. Wang, X. Y. Zhang, J. J. Cao, F. X. Shen, Y. Wu, S. Y. Xu, H. Q. Fan, et al. 2019. Differing toxicity of ambient particulate matter (PM) in global cities. Atmos. Environ. 202:305–15. doi:10.1016/j.atmosenv.2019.05.048.
- Li, J., T. Kandakji, J. A. Lee, J. Tatarko, J. Blackwell 3rd, T. E. Gill, and J. D. Collins. 2018. Blowing dust and highway safety in the southwestern United States: Characteristics of dust emission “hotspots” and management implications. Sci. Total Environ. 621:1023–32. doi:10.1016/j.scitotenv.2017.10.124.
- Li, P., J. Xin, Y. Wang, G. Li, X. Pan, S. Wang, and Z. Liu. 2015. Association be-tween particulate matter and its chemical constituents of urban air pollution and daily mortality or morbidity in Beijing City. Environ. Sci. Pollut. Res. Int. 22:358–68.
- Lough, G. C., J. J. Schauer, J.-S. Park, M. M. Shafer, J. T. Deminter, and J. P. Weinstein. 2005. Emissions of metals associated with motor vehicle roadways. Environ. Sci. Technol. 39:826–36. doi:10.1021/es048715f.
- Lytle, C. M., B. N. Smith, and C. Z. McKinnon. 1995. Manganese accumulation along Utah roadways: A possible indication of motor vehicle exhaust pollution. Sci. Total Environ. 162:105–09. doi:10.1016/0048-9697(95)04438-7.
- Maher, B. 2010. Epidemiology: Fear in the dust. Nature 468:884–85. doi:10.1038/468884a.
- Mohammed, N. Z., A. Ghazi, and H. E. Mustafa. 2013. Position accuracy testing of google earth. Int. J. Multidiscip. Sci. Eng. 4:6–9.
- Nakajima, F., and R. Aryal. 2018. Heavy metals in urban dust. Heavy Met. doi:10.5772/intechopen.74205.
- Ning, Z., A. Polidori, J. J. Schauer, and C. Sioutas. 2008. Emission factors of PM species based on freeway measurements and comparison with tunnel and dynamometer studies. Atmos. Environ. 42:3099–114. doi:10.1016/j.atmosenv.2007.12.039.
- Ntziachristos, L., Z. Ning, M. D. Geller, R. J. Sheesley, J. J. Schauer, and C. Sioutas. 2007. Fine, ultrafine and nanoparticle trace element compositions near a major freeway with a high heavy-duty diesel fraction. Atmos. Environ. 41:5684–96. doi:10.1016/j.atmosenv.2007.02.043.
- Pagotto, C., N. Rémy, M. Legret, and P. Le Cloirec. 2001. Heavy metal pollution of road dust and roadside soil near a major rural highway. Environ. Technol. 22 (3):307–19. doi:10.1080/09593332208618280.
- Pakbin, P., N. Hudda, K. L. Cheung, K. Moore, and C. Sioutas. 2009. Spatial and temporal variability of coarse (PM10−2.5) particulate matter concentrations in the Los Angeles area. Aerosol Sci. Technol. 44:514–25. doi:10.1080/02786821003749509.
- Root, R. A. 2000. Lead loading of urban streets by motor vehicle wheel weights. Environ. Health Persp. 108:937–40. doi:10.1289/ehp.00108937.
- Saini-Eidukat, B., and J. W. Triplett. 2014. Erionite and offretite from the Killdeer Mountains, Dunn County, North Dakota, USA. Am. Mineral. 99:8–15. doi:10.2138/am.2014.4567.
- Samek, L., Z. Stegowski, L. Furman, and J. Fiedor. 2017. Chemical content and estimated sources of fine fraction of particulate matter collected in Krakow. Air Quality, Atmosphere & Health 10 (1):47–52. doi:10.1007/s11869-016-0407-2.
- Sternbeck, J., A. Sjodin, and K. Andreasson. 2002. Metal emissions from road traffic and the influence of resuspension-results from two tunnel studies. Atmos. Environ. 36:4735–44. 10: 47–52. doi:10.1016/S1352-2310(02)00561-7.
- Tchounwou, P. B., A. K. Patlolla, and J. A. Centeno. 2003. Carcinogenic and systemic health effects associated with arsenic exposure–a critical review. Toxicol. Pathol. 31:575–88.
- Wagner, P., and K. Schäfer. 2017. Influence of mixing layer height on air pollutant concentrations in an urban street canyon. Urban Clim. 22:64–79. doi:10.1016/j.uclim.2015.11.001.
- Weissman, D., and M. Kiefer. 2011. Erionite: An emerging north american hazard. http://blogs.cdc.gov/niosh-science-blog/2011/11/22/erionite/
- Wilker, E. H., S. R. Preis, A. S. Beiser, P. A. Wolf, R. Au, I. Kloog, W. Y. Li, J. Schwartz, P. Koutrakis, C. DeCarli, et al. 2015. Long-term exposure to fine particulate matter, residential proximity to major roads and measures of brain structure. Stroke 46:1161–66. doi:10.1161/STROKEAHA.114.008348.