Abstract
Tea is one of the most valuable crops in many tea-producing countries. However, tea plants are vulnerable to various diseases, which reduce tea production. Early diagnosis of diseases is crucial to averting their detrimental effects on the growth and quality of tea. Conventional disease identification methods depend on the manual analysis of disease features by experts, which is time-consuming and resource-intensive. Moreover, published approaches based on computer vision left a broad scope for improving accuracy and reducing computational costs. This work attempts to design an automated learning-based model by leveraging the power of deep learning methods with reduced computational costs for accurately identifying tea diseases. The proposed work uses a Convolutional Neural Network architecture based on depthwise separable convolutions and residual networks integrated with a Support Vector Machine. Additionally, an attention module is added to the model for precise extraction of disease features. An image dataset is constructed comprising the images of healthy and diseased tea leaves infected with blister blight, grey blight, and red rust. The performance of the proposed model is evaluated on the self-generated tea dataset and compared with eight other state-of-the-art deep-learning models to establish its significance. The model achieves an overall accuracy of 99.28%.
Acknowledgments
The authors thankfully acknowledge Dr. Pradip Baruah, Chief Advisory Officer, Tocklai Tea Research Institute, Assam, India, and other associated officials of the Institute for their continuous support and encouragement.
Data Availability
The data used to support the findings of this study can be obtained from the corresponding author upon request.
Disclosure statement
No potential conflict of interest was reported by the author(s).
Additional information
Notes on contributors
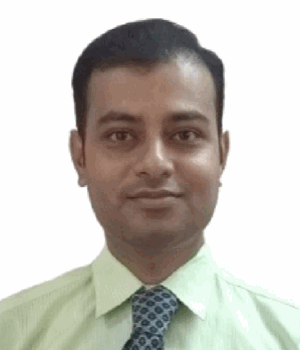
Manabendra Nath
Manabendra Nath is currently an Assistant Professor at NERIM, Guwahati, India and a PhD Scholar at National Institute of Technology, Meghalaya, India. He received his MCA degree from Jorhat Engineering College, Jorhat, India in 2008. His research interest includes Deep Learning and Image Processing.
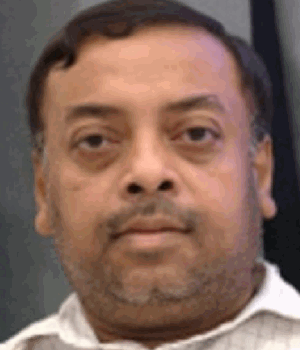
Pinaki Mitra
Pinaki Mitra is currently an Associate Professor at Department of Computer Science & Engineering, IIT Guwahati. He obtained his B.Tech in Computer Science and Engineering from Jadavpur University, Kolkata in 1987, India and his M. Tech in Computer Science and Engineering from Indian Institute of Science Bangalore, India in 1989. Finally, he obtained his Ph.D from Simon Fraser University, Canada in 1994. He worked on a project at Jadavpur University, department of Computer Science and Engineering. Subsequently he joined National Institute of Management, Kolkata, and served as an Assistant Professor. He joined IIT Guwahati in December, 2004. His research interest includes Cryptography, Network Security, Computer Graphics, Multimedia and Machine Learning.
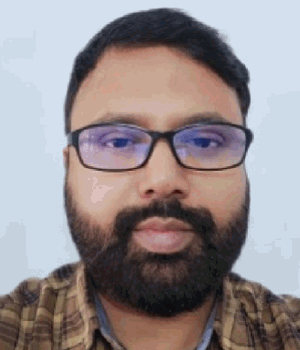
Deepak Kumar
Deepak Kumar is working as an assistant professor in National Institute of Technology Meghalaya, India since 2013. He received his B.E. degree in Electronics and communication engineering from VTU, Belgaum in 2007, and M.E. degree in computer science and technology from Bengal Engineering and Science University, Shibpur, India in year 2009. He completed his PhD from NIT Meghalaya in 2019. His research interest includes Computational Mathematics, Digital Signal Processing and Machine learning.