Abstract
A signal transformation system of posture movement for the stable state was designed in order to measure the bio-physical signal. To estimate the subject in a stable state on the basis of the bio-physical signal in the posture movement, the conditions of vision, vestibular, somatosensory apparatus and the central nervous system (CNS) were detected. Based on the vision condition, there was a greater average variation (Vi-αAVG-MAX and Vi-αAVG-MIN) in position with eyes closed and eyes opened (PC and PO), which was 27.11 ± 6.36 units. The vestibular condition showed a slightly larger average variation (Ve-αAVG-MAX and Ve-αAVG-MIN) in the body position with PC and PO, which was 6.60 ± 1.65 units. The somatosensory condition demonstrated a lower average variation (So-αAVG-MAX and So-αAVG-MIN) in position with PC and PO that amounted to 3.653 ± 2.424 units. The CNS condition was confirmed to indicate very little average variation (C-αAVG-MAX and C-αAVG-MIN) in body position with PC and PO that was at 0.401 ± 0.56 units. As the model depends on the bio-physical transformation system of posture movement, the average values of these perturbations were computed (0.01–2 Hz, range of Fourier frequency). The system consists of a data algorithm, an acquisition system, a data signal processing unit and a network system for the evaluated stability.
Introduction
The effect of bio-physical signals for human posture is enormous, both for individuals suffering from balance impairment and for the society at large. One major concern includes postural instability, which is associated with considerable attack rate and frequency of falls. As a consequence, the quality of life of patients with balance impairment and falls is remarkably low.[Citation1] The management of postural instability in patients is hindered by the intricate estimation of balance. The current tendency in practice is a combination of history taking and physical exercise, but also needs other variable conditions.[Citation2]
Posture reflex techniques are used to search the active and passive rule of balance under a diversity of conditions. The fundamental elements of most posture reflex techniques include the ability for posture or balance to be unaffected, and evaluate the response of the subject to such interactions. Available posture reflex techniques have recently been widely reviewed.[Citation3] Balance can be disturbed when the support surface makes an up-and-down motion, horizontal and vertical displacements.[Citation3] Most techniques use rapid and brief perturbations in order to study the immediate postural reactions, but slow and fast movements have been used to investigate the adaptation to movement and postural control mechanisms.[Citation4]
A network access protocol has been proposed for real-time transformation systems. The message in a real-time transformation system is associated with a timing restriction, generally in the form of a mode.[Citation5] The most commonly used network in distributed real-time systems is the network access protocol.[Citation6]
In this study, a bio-physical signal transformation system was developed to estimate the physical signal in order to detect posture movement, especially the parameters of human condition included in the opening and closing of an eye. This system was used to prove the transformation capability through the acquisition system to obtain local data in the near area.
Materials and methods
Subjects
The experiments were conducted using the database of Eulji University (Seoul, Korea), which is collected from 19 individuals (including 15 males and 4 females, Asian race). The age of the participants was between 19 and 30 years. In terms of occupation, they were all university students at our school. Informed consent for participation in the study was obtained from all participants.
Components of the bio-physical signal system
Bio-physical compound data are collected from signals for posture movement based on the studied conditions (). The bio-physical parameter was a posture movement for balance during visual condition of the ‘view open’ and ‘view close’ condition. Data analysis gave an account of a physical condition signal for system condition (). Data analysis was achieved through frequency.[Citation7]
As the systems are extremely complicated to be understood in detail and to be tested, simplified models are constructed.[Citation8] The system for signal component achieves complete automation of the testing process.[Citation9]
Since the time-labelled bio-physical signal system is infinite due to the infinitely large number of visual condition transitions, we can deal with it to generate view open/close cases. The challenge is therefore to considerably reduce the states of body movement in the system. To achieve this, a body movement of equivalent fluctuation is defined on the basis of the physical signal in order to acquire physical states equivalent to the body condition. The resulting time-labelled bio-physical signal system is called confined spaces.[Citation10,11]
Results and discussion
The proposed bio-physical signal system aims to check the movement of the posture for monitoring a subject in the health care service centre, i.e. the body movement (). This system can function normally both when the body condition is stable and restful and when it is abnormal and unstable.[Citation12–15]
Background
Many posturography studies investigated the effects of specific diagnosis modes on balance and postural control. A mode that received considerable attention is the possible effect of posture movement on signal control in different types of posturography.[Citation16] The effect of exercise has to be investigated with the posture movement in posturography. In posturography, balance exercise improved the performance on the posture movement.[Citation17] Posture sway during normal conditions was correlated with the frequency of perturbations while doing exercise. Moreover, self-reported movement was consistently correlated with the swaying frequency in more parameter.[Citation18] Even healthy subjects show substantial variability in their postural responses upon postural movement.[Citation19]
Algorithm of the bio-physical signal system
The posture movement aspects to be specified for a signal process are similar to those in the specification of the rectified posture ().[Citation20–22] The specification of a signal process included the definition of the inputs to be applied to the posture with eyes open and eyes closed (PO_PC) signal possibly in response to particular outputs received from the PO_PC value.
Figure 3. Block diagram of the proposed bio-physical signal transformation system for postural movement.
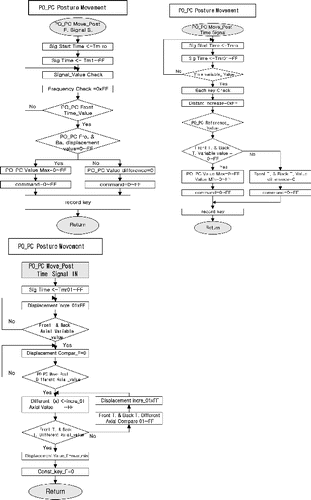
Several software algorithms are used in modules communicating PO_PC posture movement. We addressed the item of bio-physical signal systems in several time models. The following is a list of issues related to distributed testing of communication algorithms: (1) first, the frequency of the front and back signal for posture movement is defined. (2) There follows the time variable of the front and back time point. (3) Then, the axial sway displacement of front and back time is presented by the reference for PC and PO.
The frequency of the front and back signal in a transition from Front_time_value to Fro-Ba displacement gives ‘Yes’ as an output action, and a value ‘Max’ condition, while the transition from Fro-Ba displacement to Val-difference can execute on input ‘No’ at any time, and resets to zero command value. A distinction between a posture movement signal case and a front-back displacement case is made. The most important aspect is the form of the connection through which the frequency case interacts with the PO_PC value.
The time variable of the front and back time point in a transition from Reference_value to Front-Back_T variable value has ‘Yes’ as an output condition, and a value ‘Max–Min’ condition, while the transition from Front-Back_T variable value to T-variable difference can execute on input ‘No’ at any time, and resets to zero command value. A clear distinction between a posture movement time signal case and a reference displacement case is made. The most important aspect is the form of the connection through which the frequency case interacts with the PO_PC value of posture movement.
The axial sway displacement of front and back time by the reference in a transition from Front-Back Axial variable value to Move-Post Diff-Axial variable value has ‘Yes’ as a flow action; a transition from Move-Post Diff-Axial variable value to Front-Back_T_different Axial value has ‘Yes’ as a flow action, and a value ‘Displacement’ condition; while the transition from Front-Back_T_different Axial value to T_different Axial comparison can execute on input ‘No’ at any time, and reconstruct to zero Const-key value. A sharp distinction between a front_back signal case and an axial displacement case is made. The most important aspect is the form of the connection through which the axial displacement case interacts with the PO_PC value of sway.
Experimental results and analysis
The average results from the analysis of the studied parameters and conditions in the 19 participants are shown in .
Table 1. Average of posture movement difference measures to the various Vision (Vi-αAvg), Vestibular (Ve-αAvg), Somatosensory (So-αAvg) and CNS (C-αAvg) conditions.
Comparison of αMAX-POPC, αMIN-POPC and αAVG-POPC
The vision condition was verified as a variation for the Vi-αAVG-MAX and Vi-αAVG-MIN (). The greater average difference between Vi-αAVG-MAX and Vi-αAVG-MIN was with PC and PO of body. The greater average difference between Vi-αAVG-MAX and Vi-αAVG-MIN was at 27.11 ± 6.36 units. Vi-αAVG-AVG was presented slightly greater at 12.47 ± 4.23 units. Vi-αMAX-POPC was at 30.63 ± 7.78 units, which varied to a greater extent than Vi-αAVG-AVG. Vi-αMIN-POPC was 3.52 ± 1.42 units, which varied a more than Vi-αAVG-AVG.
The vision condition was observed to vary more with posture movement of PO and PC. The vision average showed larger fluctuation at 7.17 ± 3.55 units with Vi-αAVG-POPC. Therefore, the parameter that the body sway influences is the vision. This indicated that the vision condition was more important for postural control when the visual characteristics showed the largest variance.
The vestibular condition was determined based on the variation of Ve-αAVG-MAX and Ve-αAVG-MIN (). A little larger average difference between Ve-αAVG-MAX and Ve-αAVG-MIN was observed with PC and PO of body. The larger average difference between Ve-αAVG-MAX and Ve-αAVG-MIN was at 6.60 ± 1.65 units. Ve-αAVG-AVG was slightly larger at 6.78 ± 1.69 units. Ve-αMAX-POPC was at 10.88 ± 3.36 units, which varied slightly more than Ve-αAVG-AVG. Ve-αMIN-POPC was at 4.28 ± 1.71 units, which varied a little more than Ve-αAVG-AVG.
The vestibular condition showed a slightly larger variation with posture movement of PO and PC. The vestibular averages showed slightly larger variation at 4.10 ± 1.67 units with Ve-αAVG-POPC. The vestibular condition was less influenced by the posture movement during body sway. This showed that the vestibular condition was more important for postural control when the vestibular characteristic presented the largest variance.
The somatosensory condition showed a variation for the So-αAVG-MAX and So-αAVG-MIN (). The smaller average difference between So-αAVG-MAX and So-αAVG-MIN was with PC and PO of body. The smallest average difference between So-αAVG-MAX and So-αAVG-MIN was at 3.653 ± 2.424 units. So-αAVG-AVG was observed to be slightly smaller at 2.32±0.93 units. So-αMAX-POPC was at 4.86 ± 2.438 units, which varied slightly less than So-αAVG-AVG. So-αMIN-POPC was at 1.217 ± 0.214 units, which varied a little less than So-αAVG-AVG.
The somatosensory condition showed smaller variation with posture movement of PO and PC. The somatosensory average was shown to vary only a little at 2.54 ± 1.50 units with So-αAVG-POPC. Therefore, the somatosensory condition was less influenced by posture movement. This showed that the somatosensory condition was more important for postural control when the somatosensory characteristic demonstrated greater variation.
The central nervous system (CNS) condition was confirmed to vary for the C-αAVG-MAX and C-αAVG-MIN (). The smallest average difference between C-αAVG-MAX and C-αAVG-MIN was with PC and PO. The smallest average difference between C-αAVG-MAX and C-αAVG-MIN was at 0.401 ± 0.56 units. C-αAVG-AVG was slightly larger at 0.386 ± 0.141 units. C-αMAX-POPC was at 0.688 ± 0.57 units and varied a little more than C-αAVG-AVG. C-αMIN-POPC was at 0.287 ± 0.0004 units and varied a little less than C-αAVG-AVG.
The CNS condition was observed to vary less with posture movement of PO and PC. The CNS average was shown to be a little less at 0.302 ± 0.43 units with C-αAVG-POPC. Thus, CNS condition was little affected by posture movement during body sway. This showed that the CNS condition was more effective for postural control when the CNS characteristic presented a smaller variance.
Performance evaluations
The signal data to estimate bio-physical signal results of the stable state detected on the basis of signal in the posture movement are shown in . This condition was verified with a little greater average variation (AvgAVG-POPC) with a stability of body that was at 20.63 ± 12.42 units.
Figure 8. Signal data of stability by the normal postural movement in PO and PC (Stab-APM, AvgMAX-POPC, AvgMIN-POPC, AvgAVG-POPC).
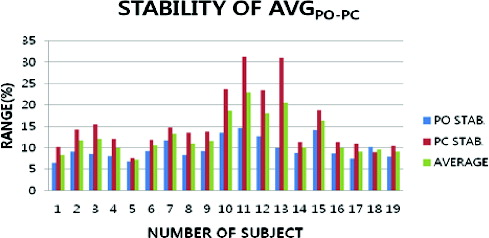
The average difference of postural movement was illustrated by the values of Stab-APM (including AvgAVG-POPC). The results are shown in . To present a perturbation, the average difference was larger in the PC condition. The stability part showed a little more stable state in the PO condition.
Table 2. Average of posture movement difference measures to the various Stab-APM values.
Conclusions
In this paper, we propose a model design of a signal transformation system of postural movement for the stable state. The bio-physical signals for the subject at the stable state were estimated in order to achieve detection of the postural movement on the basis of the signal. The detected conditions were Vision, Vestibular, Somatosensory and CNS. Further studies will focus on the possibility for the proposed system to be used for correction of posture.
Acknowledgements
This article is a revised and expanded version of a paper entitled ‘Estimation of the posture signal acquiring system’ presented at International Symposium on Advanced and Applied Convergence held on 14–16 November 2013, in Seoul, Korea.
References
- King MB, Tinetti ME. Falls in community-dwelling older persons. J Am Geriatr Soc. 1995;43(10):1146–1154.
- Cummings SR, Nevitt MC, Kidd S. Forgetting falls: the limited accuracy of recall of falls in the elderly. J Am Geriatr Soc. 1988;36(7):613–616.
- Bloem BR, Visser JE, Allum JH. Posturography. In: Hallett M, editor. Movement disorders – handbook of clinical neurophysiology. Elsevier; 2003. p. 295–336.
- Henry SM, Fung J, Horak FB. EMG responses to maintain stance during multidirectional surface translations. J Neurophysiol. 1998;80(4):1939–1950.
- Ulusoy Ö. A network access protocol for hard real-time communication systems. Comput Commun. 1995;18(12):943–948.
- Zhao W, Ramamritham K. Virtual time CSMA protocols for hard real-time communication. IEEE Trans Software Eng. 1987;13(8):938–952.
- Lee KJ, Park SW. Patient classification scheme for patient information management in hospital U-healthcare system. JIIBC. 2010;10(3):131–137.
- Beizer B. Software testing techniques (Van Nostrand Reinhold electrical computer science and engineering series). Van Nostrand Reinhold; 1983. p. 101–127.
- Groz R, Phalippou M. La génération automatique de tests est-elle possible. In: Jard C, Rolin P, editors. Colloque Francophone sur l’Ingenierie des Protocols CFIP’95. Boston (MA); 1995. p. 121–137.
- Alur R, Dill D. Automata for modeling real-time systems. In: Paterson M, editor. 17th ICALP International Colloquium on Automata, Languages, and Programming. UK: Springer; 1990. p. 322–335.
- Alur R, Dill D. A theory of timed automata. Theor Comput Sci. 1994;126:183–235.
- Verissimo P, Rodrigues L. A posteriori agreement for fault-tolerant clock synchronization on broadcast networks. In: Storms P, editor. Digest of Papers, The 22nd International Symposium on Fault-Tolerant Computing (IEEE). Boston (MA); 1992. p. 101–128.
- Broster I, Burns A. Timely use of the can protocol in critical hard real-time systems with faults, In: Williams D, editor. Proceedings of the 13th Euromicro Conference on Real-Time Systems 2001 (ECRTS’01). Boston (MA); 2001. p. 95–102.
- LabVIEW 7.1 [Internet]. Available from: http://www.ni.com/labview/upgrade.htm [accessed July 2006].
- Kim JL, Kim KD. Estimation of the posture signal acquiring system. Adv Appl Convergence Lett. 2013;1:24–26.
- Rocchi L, Chiari L, Horak FB. Effects of deep brain stimulation and levodopa on postural sway in Parkinson's disease. J Neurol Neurosurg Psychiatry. 2002;73(3):267–274.
- Hirsch MA, Toole T, Maitland CG, Rider RA. The effects of balance training and high intensity resistance training on persons with idiopathic Parkinson's disease. Arch Phys Med Rehabil. 2003;84(8):1109–1117.
- Baloh RW, Fife TD, Zwerling L, Socotch T, Jacobson K, Bell T, Beykirch K. Comparison of static and dynamic posturography in young and older normal people. J Am Geriatr Soc. 1994;42(4):405–412.
- Geurts AC, Nienhuis B, Mulder TW. Intrasubject variability of selected forceplatform parameters in the quantification of postural control. Arch Phys Med Rehabil. 1993;74(11):1144–1150.
- Cottet F, David L. A solution to the time jitter removal in deadline based scheduling of real-time applications. In: Werner B, editor. Fifth IEEE Real-Time Technology and Applications Symposium – WIP. Vancouver (Canada); 1999. p. 33–38.
- Jerri AJ. The Shannon sampling theorem – its various extensions and applications: a tutorial review. Proc IEEE. 1977;65(11):1565–1596.
- Kim JL, Yeom HJ, Lee KY. Physical signal control system for preservation of posture balance. Int J Multimed Ubiquitous Eng. 2012;7(2):247–252.