Abstract
Cyclotides are cysteine-rich cyclic peptides that have various activities and applications. Mining at the whole-genome scale facilitates the profiling and development of cyclotide-based markers. However, the characterization of cyclotide subfamilies at a genome-scale in plants is an untapped area that needs more studies. Here we developed four degenerate cyclotide subfamily-specific (CSS) markers based on their subfamily’s semi-conserved sequence for identifying each subfamily separately. Initially, an in silico cyclotide mining analysis was performed on 15 plant genomes/transcriptomes. The in silico PCR produced 476 amplicons in the Bracelet, Moebius, Hybrid and Trypsin Inhibitor (TI) subfamilies. In silico profiling results identified 903 cyclotide mature domains (CMDs) in the analyzed genomes/transcriptomes for the four subfamilies. In addition, the prevalence of each subfamily correlated positively with the genome size, particularly in Hybrid, followed by TI and Bracelet subfamilies. The CSS markers were validated in vitro on 19 genotypes/cultivars. The CSS markers generated a total of 175 bands, with a polymorphic percentage of 98%–100%. Cluster analysis showed distinct clusters for cultivars/genotypes of each plant species. The marker efficiency parameters demonstrated the capability of CSS markers to determine the genetic diversity among the studied plant species. This study represents the first report to perform genome/transcriptome-wide mining to characterize the Cyclotide subfamilies in plants. This study proposed potential applications of the novel CSS markers as an effective system for screening cyclotide subfamilies across multiple plant species with no prior sequencing knowledge requirement. Additionally, it proposes an innovative approach for identifying the appropriate cyclotide subfamily used in drug design.
Introduction
Cyclotides are a family of plant peptides with a short length of 28 to 37 amino acid residues [Citation1]. Lorents Gran discovered the cyclotide in the 1970s from a plant called Kalata-Kalata (Oldenlandia affinis) in northern Congo, Africa [Citation2]. Later in 1999, the term ‘cyclotide’ was formally coined by Craik and his colleagues [Citation3].
Cyclotides have a cyclic cysteine knot (CCK) motif as a key feature. This motif results from a combination of six conserved cysteine residues that form three disulphide bonds in a cysteine-knot arrangement (cysteine I–IV, II–V, and III–VI) [Citation4]. In addition to the CCK, the six backbone segments between the successive cysteines form loops, with loop 1 spanning from Cys I to Cys II, loop 2 from Cys II to Cys III, and so on until loop 6 spans from Cys VI back to Cys I to close the circular backbone [Citation5]. Loops 1 and 4 are highly conserved across subfamilies and are considered to be part of the cysteine knot motif, whereas the other loops are only conserved within the individuals of each subfamily [Citation4].
Cyclotides are multidomain peptides resulting from a protein’s precursors with a distinct genetic structure [Citation6]. The size of cyclotide precursors ranges from 100 to 200 amino acids [Citation7]. Both the basic and highly conserved layouts of precursor proteins are mostly identical. The precursor organization consists of five units: (i) endoplasmic reticulum signal domain (18–30 residues); (ii) N-terminal prodomain, (22–55 residues); (iii) N-terminal repeat domain (16–20 residues); (iv) mature cyclotide domain (28–37 residues); and (v) hydrophobic C-terminal tail region (3–11 residues) [Citation8].
The classification of cyclotides initially grouped them into Moebius and the bracelet as the main subfamilies (based on the presence or absence of a cis-Pro peptide bond in loop 5) [Citation9]. The conserved cis-Pro residue in loop 5 of the Moebius subfamily twists the loop at 180°, which does not occur in the bracelet subfamily [Citation10]. Later, the discovery of new cyclotides gave rise to three new subfamilies: Hybrid, Trypsin Inhibitors (TI) and Linear cyclotides [Citation11–13]. Hybrid cyclotides combine the sequence characteristics of both the Moebius and Bracelet subfamilies [Citation14]. TI cyclotides originated from gourd plants and are considered a cyclotide subfamily due to the presence of the CCK motif; otherwise, they have no sequence similarity with the other cyclotide subfamilies [Citation15]. Furthermore, the Linear cyclotide subfamily has sequence similarities with other cyclotide subfamilies except for their cyclic backbone [Citation11,Citation12].
Cyclotides have unique activities due to their unique CCK motif which offers structural stability and rigidity, making them resistant to chemical and thermal denaturation and proteolytic degradation [Citation16]. Moreover, naturally occurring cyclotides play different roles in plant defence and response due to their metal-binding [Citation17], cytotoxic [Citation18], anti-viral [Citation19] and antimicrobial [Citation20] properties. Besides, native cyclotides have various bioactivities such as uterotonic [Citation21], anticancer [Citation22], anti-HIV [Citation23] and immunosuppressive [Citation24], which make them an ideal substrate for molecular engineering to produce novel peptide-based diagnostic, therapeutic and research tools [Citation25].
Various cyclotides have been identified in different plant species of the Violaceae, Rubiaceae, Solanaceae, Fabaceae and Cucurbitaceae [Citation26–30]. The two main approaches for screening and identifying cyclotide sequences in plant species are the phytochemistry-based approach (amino acid sequencing of isolated cyclotides) and the gene-based strategy (sequencing cyclotide precursor gene sequence) [Citation22,Citation31]. Meanwhile, the first approach is responsible for establishing a foundation for discovering cyclotides and their applications by using mass spectrometry and various chromatographic techniques [Citation32–34]. The second approach, on the other hand, uses blast tools to screen cyclotide-encoding gene sequences from plant species’ RNA or DNA [Citation35].
Owing to the development of high-throughput sequencing technologies such as next-generation sequencing, hundreds of drafted or completed plant genomes have been decoded and released. With the help of current powerful bioinformatics tools, these published genomes offer scientists an opportunity for mining and identifying different types of cyclotide motifs that could be used to develop useful cyclotide-based markers [Citation36].
To date, there are almost no reports focusing on the characterization or mining of cyclotide subfamilies at the genome level in various plant species. Therefore, this study represents the first report aimed at the genome/transcriptome-wide cyclotide profiling and characterization of their subfamily’s frequencies across several plant genomes through the development of a cyclotide subfamily-specific (CSS) marker system. Additionally, the validation of its cyclotide-screening ability and efficiency in genetic diversity analysis makes it an ideal alternative to the sequencing approach.
Materials and methods
All experiments for this study were carried out at the Molecular Genetics and Genome Mapping Laboratory, Agricultural Genetic Engineering Research Institute (AGERI), Agricultural Research Centre (ARC).
Genome-wide in silico mining of cyclotides
Collection of cyclotide sequences
To identify conserved or semi-conserved sequences of each cyclotide subfamily, all available cyclotide nucleotide and amino acid sequences were retrieved from the NCBI and UniProt databases in both FASTA and Gen-Bank formats (Supplemental Table S1). After that, these sequences were classified based on their subfamily type.
Collection of plant genomes and transcriptomes
To validate all cyclotide primers developed at the genome/transcriptome level and perform cyclotide genome-wide profiling, the genomes and transcriptomes of 15 plant species were downloaded in FASTA format from the NCBI database (NCBI’s FTP site: ftp://ftp.ncbi.nlm.nih.gov/). All retrieved genomes/transcriptomes and their information are listed in Supplemental Table S2.
As the primers are universal within the plant kingdom, they will be used to screen the cyclotide subfamily plant peptides. We selected different plant genomes to represent different classes of plants/crops such as plant models, oil crops, cereal crops, horticultural crops, sugar crops, vegetable crops and ornamental plants. Additionally, two other factors affected our selection; not being studied before and complete genome/transcriptome availability.
Alignment of sequences and primer design
The nucleotide and amino acid sequences of each cyclotide subfamily were aligned separately using the Clustal-W algorithm in MegAlign 7.1.0 software (DNAStar Inc., Madison, WI, USA). Due to the limited number of nucleotide sequences of each cyclotide subfamily, the amino acid sequences were ‘reverse translated’ to nucleotide sequences and subsequently aligned. After the alignment of amino acid sequences for each cyclotide subfamily, only the most semi-conserved sequences located at the borders of each cyclotide mature domain (CMD) were used to design degenerate cyclotide subfamily-specific (CSS) markers.
In silico PCR analysis of cyclotides
To computationally determine the expected number of 1) amplifiable cyclotide mature domain (CMD) products, and 2) amplifiable regions representing the distance between two adjacent cyclotide domains within a certain plant genome, in silico PCR analysis was performed using the EMBOSS software (particularly, the primersearch tool) [Citation37]. To mimic the PCR reality, we tested different mismatch percentages for each primer pair (5%, 10% and 15%). Finally, the results of the primersearch output file were filtered to keep only the amplicons of less than 4 Kb.
Cyclotide profiling
Degenerate primers targeting the cyclotide mature domain were used to scan the prevalence of cyclotide subfamilies within the retrieved plant genomes and transcriptomes. To accomplish this goal, every single primer out of all pairs was used in cyclotide profiling analysis using FastPCR software [Citation38]. The FastPCR analysis tool was run according to the default parameters (maximum 1 mismatch per 3′ end of each primer).
To ensure the presence of cyclotides in the FastPCR output results, initially, only matches over 70% were extracted through in-house Python scripts. After that, the obtained results were screened for the loop features of cyclotides using the CyPerl in silico tool [Citation39].
Cyclotide in vitro analysis
Plant material and DNA isolation
Six species of plants representing different economically valuable cereal, vegetable and oil crops were selected to be used in the cyclotides in vitro analysis as follows: tomato (Solanum lycopersicum L.), cucumber (Cucumis sativus), rice (Oryza sativa), sesame (Sesamum indicum L.), wheat (Triticum aestivum), faba bean (Vicia faba) and wild pansy (Viola tricolor). Three cultivars/genotypes of each of the six plant species were used in the study, as presented in Supplemental Table S3. The seeds were kindly provided by the Field Crop Research Institute at the Agricultural Research Center, Egypt. Genomic DNA was extracted from fresh tissue of the cultivars of all plant species via the DNeasy Plant DNA Extraction Kit (Qiagen, Germany), according to the manufacturer’s instructions. Then, the isolated DNA was measured using the Nanodrop spectrophotometer (Thermo Fisher Scientific Inc., Wilmington, DE).
PCR amplification
For each plant species, the designed degenerate CSS primers were used to detect the presence of each cyclotide subfamily within the selected cultivars. The polymerase chain reaction was performed in a total volume of 25 μL per reaction containing: 30 ng of genomic DNA, 10 pmol of each primer pair, 5x PCR buffer, 25 mmol/L of MgCl2, 2 mmol/L of dNTPs and 0.1 U of Go-Taq Flexi polymerase (Promega). Amplifications were performed using the following program: initial denaturation at 94 °C for 4 min, 40 cycles (denaturation at 94 °C for 30s; the annealing temperature was adjusted depending on the primers for 30s; extension at 72 °C for 30s); and final extension at 72 °C for 7 min. The thermocycler 2720 (Applied Biosystems) was used for PCR product amplification.
The amplification products were initially resolved by 1.5% agarose gel electrophoresis and polyacrylamide non-denaturing gel electrophoresis with ethidium bromide staining. Finally, all gels were photographed using the Gel Doc XR + Gel Documentation Framework (Bio-Rad, USA).
Data analysis and visualization
For the in silico PCR analysis, the obtained results were visualized in an alluvial diagram using the SRplot online toolbox (http://www.bioinformatics.com.cn). Additionally, cyclotide profiling data were plotted as a Beesworm plot using the RAWGraphs online tool [Citation40]. Finally, a correlation coefficient between the genome size and the number of cyclotides of different subfamilies was calculated using the Pearson method and visualized using the SRplot online toolbox (http://www.bioinformatics.com.cn). Differences were considered statistically significant at the level of p < 0.05.
For the in vitro PCR data analysis, the amplified amplicons were visually scored in a binary form. Only the distinct and recognizable bands were scored to minimize errors. A dendrogram was constructed using the unweighted pair group method of the arithmetic averages (UPGMA) for all developed cyclotide subfamily markers using PAST Software [Citation41]. A similarity matrix was calculated based on the Jaccard similarity coefficient [Citation42]. Seven informative indices were calculated to evaluate the efficiency of the developed cyclotide subfamily markers, as described in Ref. [Citation43].
Results
This study initially aimed to develop as well as validate cyclotide-subfamily specific markers and perform genome/transcriptome-wide profiling and characterization of cyclotide subfamilies in several plant species. These goals were accomplished through the implementation of two approaches (in silico and in vitro approaches). These approaches are summarized and presented in the workflow diagram ().
Figure 1. Workflow for genome/transcriptome-mining of cyclotides. This workflow shows the main steps which were divided into two main approaches as follows: 1) In silico approach (involving retrieval of genomes/transcriptomes and cyclotide sequences, cyclotide sequence alignment, primer design, in silico mining analysis, data visualization and statistical analysis), 2) In vitro approach (involving DNA isolation, PCR amplification and data analysis).
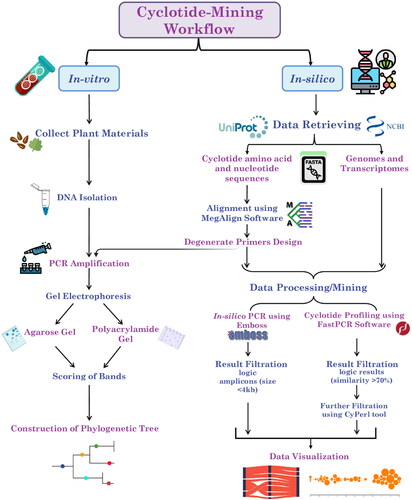
In silico approach
Cyclotide subfamily specific primers
The reproducibility and reliability of any PCR-based markers has been reported to be affected by various factors [Citation44]. In this study, cyclotide subfamily-specific (CSS) primers were designed based on the semi-conserved sequences located at the borders of each cyclotide mature domain (CMD). The average primer length was 20 mer, with an average degeneracy percentage of 48 and an average GC% of 52%. Specific nucleotides in all the primer pairs’ sequences were fixed (the TGY codon, which represents the cysteine amino acid residues) (Supplemental Table S4 and Figure S1).
In silico PCR analysis of cyclotides
An in silico PCR analysis of 15 plant genomes and transcriptomes was performed to determine each cyclotide subfamily’s amplifiable products/patterns. For the in silico PCR analysis results, the 10% mismatch showed the most reasonable/logical number of products compared to the 5% and 15% mismatches, which generated mostly no products or a massive number of products, respectively. At 10% mismatch, the Bracelet in silico PCR results ranged from one amplicon (in Cucumis sativus) to 53 amplicons (in Brassica napus). Meanwhile, Oryza sativa and Vicia faba plants showed no amplifiable products ().
Figure 2. Alluvial diagrams showing the in silico PCR results (logic results < 4 kb) of Bracelet, Moebius, Trypsin Inhibitors, and Hybrid primers against different plant types at a 10% mismatch percentage. Diagram (a) shows the number of amplicons/subfamily in each plant genome, and Diagram (b) shows the number of amplicons/subfamily in each plant transcriptome.
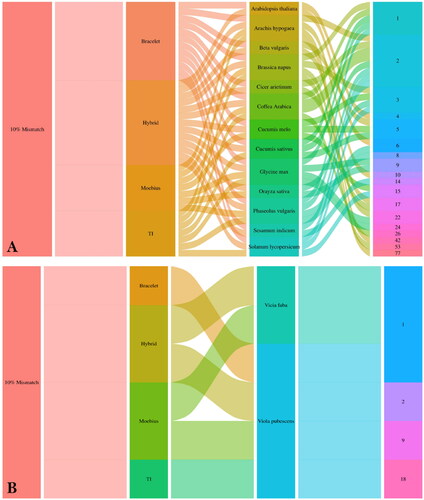
The amplifiable products generated from the Moebius in silico PCR analysis ranged from one amplicon (in Brassica napus, Coffea arabica, Phaseolus vulgaris, Glycine max and Vicia faba plants) to 18 amplicons (in Viola pubescens). Meanwhile, Arabidopsis thaliana, Beta vulgaris, Cicer arietinum, Cucumis sativus, Sesamum indicum and Solanum lycopersicum plants showed no amplifiable products ().
For the Hybrid in silico PCR analysis, the generated amplifiable products ranged from one amplicon (in Vicia faba) to 42 amplicons (in Arachis hypogaea) (). The amplifiable products generated from the Trypsin Inhibitor in silico PCR analysis ranged from one amplicon (in Viola pubescens) to 77 amplicons (in Arachis hypogaea). Arabidopsis thaliana, Beta vulgaris, Cicer arietinum, Cucumis sativus, Cucumis melo, Oryza sativa, Sesamum indicum, and Vicia faba plants showed no amplifiable products ().
The correlation analysis between the prevalence of the cyclotide subfamilies for each plant and its genome size revealed that the Hybrid subfamily showed a high positive correlation with genome size, followed by the Trypsin Inhibitors subfamily. Meanwhile, the Bracelet subfamily had the lowest positive correlation. In contrast, only the Moebius subfamily had a negative correlation with the genome size ().
In silico profiling analysis of cyclotides
An in silico mining search was performed to determine the prevalence of cyclotide subfamilies within the above-mentioned plant genomes and transcriptomes. The results revealed that the Trypsin Inhibitors were the most prevalent subfamily within the analyzed plant genomes and transcriptomes, except in Vicia faba, with a wide range of their records/hits (from 3 hits in Brassica napus, to 153 hits in Arachis hypogaea) (). Furthermore, the Moebius subfamily was the second most prevalent in all plant genomes/transcriptomes, with hits ranging from 2 (in Vicia faba) to 35 (in Brassica napus) (). The Hybrid was the 3rd most prevalent subfamily, followed by the Bracelet subfamily, which revealed the lowest number of records or hits compared to all other subfamilies ().
In vitro approach
The Cyclotide Subfamily-Specific (CSS) technique is based on primer pair amplified cyclotide mature domain (CMD) regions, unlike the Random Amplified Polymorphic DNA (RAPD) or Inter Simple Sequence Repeat (ISSR) techniques, which use a single primer as a forward and reverse. These CSS primers can either target one CMD or amplify the inter-spaced regions between two or more adjacent CMDs (with lengths of less than 4-5 kb) for each cyclotide subfamily (). An important factor that determines the number of amplified regions is the distance in base pairs between the primer pair binding sites. Each CSS primer pair is designed to anneal with about 66% of cysteine residues located within each CMD for each cyclotide subfamily (). However, PCR amplification uses CSS primers localized within genic regions on both DNA strands. Therefore, some CSS amplicons are expected to act as codominant markers due to insertion-deletion mutations.
Figure 5. Diagram showing the principle of Cyclotide Subfamily-Specific (CSS) PCR-based markers and their capability to differentiate between close genotypes based on the prevalence of cyclotide mature domains (CMDs) for a certain subfamily, and distances between two adjacent CMDs.
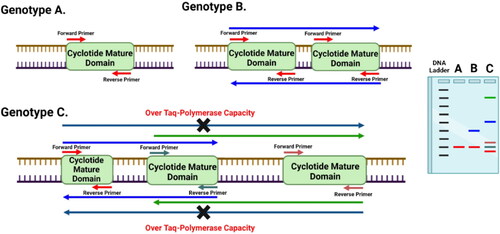
The developed CSS marker system was validated using three degenerate primer pairs representing the Bracelet, Hybrid and Trypsin Inhibitor cyclotide subfamilies. In addition, they were evaluated for their ability to investigate the genetic diversity levels of 19 genotypes and cultivars representing seven plant species. Although agarose gels demonstrated a reliable pattern (data not shown), only clearly scorable and detected amplicons resolved on the polyacrylamide gels were considered for phylogeny analysis (). The three CSS primers generated a total of 175 amplicons, with polymorphic percentages ranging from 98 to 100% (). The number of amplicons per genotype ranged from 7–27 amplicons with a size ranged from 50 bp to 1.2 kb (in Bracelet), 11–33 amplicons ranging in size from 90 bp to 4 kb (in Hybrid), and 8–27 amplicons have a size of 190 bp-2 kb (in Trypsin Inhibitors) (). Notably, the faba bean species showed the lowest number of amplicons in all cyclotide subfamilies. While the tomato species revealed the highest number of amplicons in the Hybrid subfamily, followed by the sesame species in the Bracelet and Trypsin Inhibitors subfamilies ().
Figure 6. Acrylamide gels used in screening of cyclotides and their analogues using cyclotide sub-family specific primers (Bracelet, Hybrid and Trypsin Inhibitors) in different economic plant species: Tomato ‘Solanum lycopersicum’ (T.CV1: LOJAIN, T.CV2: L-276, and T.CV3: 023), Cucumber ‘Cucumis sativus’ (C.CV1: AL NEMS F1, C.CV2: Ruba 73 F1, and C.CV3: OF OFFICER F1), Rice ‘Oryza sativa’ (R.CV1: SK-102, R.CV2: SK-103, and R.CV3: SK-106), Sesame ‘Sesamum indicum’ (S.CV1: Sohag-1, S.CV2: Toshky-1, and S.CV3: Shandwil-3), Wheat ‘Triticum aestivum’ (T.CV1: Bany Swif-4, T.CV2: Gemmeiza-1, and T.CV3: Sakha 95), Faba bean ‘Vicia faba’ (F.CV1: Wadi 1, F.CV2: Nobaria 1, and F.CV3: Nobaria 3), and Viola tricolor.
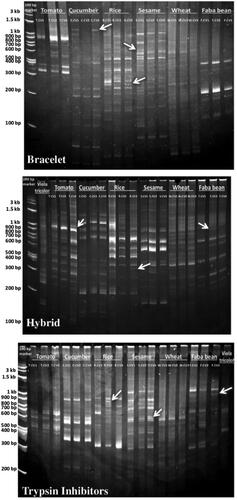
Table 1. Primers and marker efficiency parameters of designed cyclotide primers.
Based on UPGMA analysis, the dendrogram was constructed using the combined data generated from Bracelet, Hybrid and TI markers of the seven plant species and their cultivars/genotypes. Generally, the dendrogram topology of the analyzed genotypes revealed a consistent clustering with the known taxonomic/pedigree information (). The dendrogram comprised two main clusters; the first comprised only all sesame cultivars. The second cluster was divided into two sub-clusters; the first included only all cucumber cultivars. Meanwhile, the second subcluster was subdivided into two separate groups, the first of which comprised only faba bean cultivars. In contrast, the second group clustered four plant species (wheat, rice, tomato and Viola tricolor), with discrete separation for cultivars belonging to each plant species ().
Figure 7. Phylogenetic tree showing the similarity among seven different plant species and their cultivars/genotypes based on Jaccard’s similarity of Bracelet, Hybrid and TI cyclotide combined data.
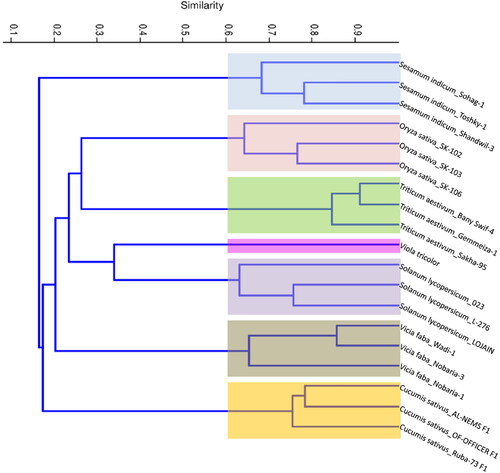
Furthermore, the similarity matrix analysis of the combined data revealed highly similar results to the molecular phylogeny analysis. The results indicated that the highest similarity (within the same species) was observed between the wheat cultivars Bany Swif-4 and Gemmeiza-1 (91.1%), while the lowest similarity was found between the rice cultivars SK-102 and SK-103 (58.2%) (Supplemental Figure S2).
Based on the analysis of the obtained marker results (Bracelet, Hybrid and Trypsin Inhibitor), the following efficiency parameters (Heterozygosity values, Polymorphism Information Content, mean Heterozygosity, Marker Index and Discriminating power) exhibited a narrow range of values. In contrast, the effective multiplex ratio and resolving power parameters showed a moderate range of values ().
Discussion
Cyclotides are short, head-to-tail cyclic peptides that harbour the cyclic cysteine knot (CCK) motif, which plays a key role in plant defence. Additionally, it exhibits a wide range of natural and synthetic activities that contribute to various applications, particularly in the pharmaceutical field. Due to the significance of the rapid development of biodata mining and bioinformatics tools, as well as the clear lack of studies focusing on cyclotide genome mining, a crucial need has been raised to perform a cyclotide genome-wide characterization to understand the abundance of each cyclotide subfamily within diverse plant genome species.
During the last decade, previous efforts have been made to address cyclotide-mining at the genome and transcriptome levels, with some of the following drawbacks: i) lacking the classification of screened cyclotides into subfamilies; ii) no comparative analysis between cyclotide subfamilies and their prevalence; and iii) missing the development of cyclotide subfamily-specific markers. Although regarding the development of specific cyclotides, primers can work efficiently in PCR, it was found to represent a big challenge due to the lower to moderate conservativeness in the sequences of cyclotide precursors (with sequence similarities of nearly 60%). However, the results of this study suggested that developing cyclotide subfamily-specific (CSS) degenerate primers is an effective approach to overcome this obstacle.
Hence, to our knowledge, this study represents the first report focusing on the development of cyclotide subfamily-specific markers as well as in silico mining and profiling of cyclotide subfamilies at the genome/transcriptome level in different plant species. It is noteworthy that our developed primers are considered the most effective for screening cyclotides compared to the previously published primers due to their semi-conservative design nature, targeting a wide range of plant species with the ability to identify certain cyclotide subfamilies. However, there are many limitations to using the previous primers for cyclotide screening, including their inability to determine/characterize each cyclotide subfamily across various plant species. Particularly, they target only a single cyclotide sequence in a certain plant species [Citation45,Citation46] or only one cyclotide subfamily within a certain species [Citation47]. Additionally, some of these primers are designed based on the conserved regions AAFALPA in the ER signal domain in the cyclotide precursors [Citation47], which target all cyclotides regardless of their subfamily. Moreover, several degenerate forward primers were designed to target cyclotide domains in combination with oligo (dT) as a reverse primer [Citation48], but they were also unable to distinguish between different cyclotide subfamilies without sequencing.
Regarding the cyclotide in silico mining of our developed CSS markers across 15 plant species, this study successfully identified a total of 903 CMDs, including 191 Bracelet, 206 Moebius, 49 Hybrid and 457 TI CMDs. A previous study conducted in [Citation39] found that the in silico screening of cyclotides based on the six cysteine-distributed regions (CDRs) identified a total of 510 cyclotide/cyclotide analogues within 34 plant species genomes/proteomes without dissection of their cyclotide subfamilies. Compared to our results, the screening in Arabidopsis thaliana, Cicer arietinum, Brassica napus, Glycine max, Oryza sativa and Solanum lycopersicum identified 0, 3, 9, 4, 4 and 2 cyclotide/cyclotide analogues, respectively. Meanwhile, this study identified a higher number of CMDs in the same plant genomes as 6, 20, 55, 297, 69 and 32 CMDs, respectively. In contrast, Phaseolus vulgaris exhibited a higher number of cyclotides (62 cyclotide/cyclotide analogues) when compared to our results (14 CMDs).
Additionally, a recent study on wheat that combined the in silico and in vitro screening of cyclotide-related sequences (CRS) successfully identified 5 CRSs [Citation49], while our in vitro results exhibited 51 cyclotide targets.
At the proteomic level, a recent study aimed to screen Beta vulgaris, which is known to contain a cyclotide-like peptide, through plant extracts combined with pharmacological analysis [Citation50]. This screening identified only one cyclotide-like peptide (bevuTI-I), which is the Trypsin Inhibitor peptide [Citation50], whereas our findings revealed a total of 24 CMDs containing 9 Trypsin Inhibitor cyclotides.
Notably, the number of cyclotides identified at the transcriptome level was 30 CMDs in Viola pubescens and 3 CMDs in Vicia faba. For V. pubescens, a recent study investigated the in silico mining of cyclotides within its draft genome and identified 81 CMDs [Citation51] without characterization of different cyclotide subfamilies. This limited number of identified CMDs might be attributed to the mining of the transcriptome rather than the genome, which does not include all cyclotide genes or CMDs owing to the fact that the expression of cyclotides occurs in a tissue-specific manner.
Based on the molecular phylogeny of cyclotide in vitro mining results, the constructed dendrogram revealed a relatively high degree of phylogenetic precision (similarity between cultivars of 91.1%), obtained from the utilization of CSS markers. Furthermore, it was noticed that CSS markers efficiently determined the genetic diversity and clustering by grouping all cultivars/genotypes belonging to each species together without any overlapping or outliers between different species. As this study represents the first report assessing the efficiency and precision of developed cyclotide subfamily-based markers in molecular phylogeny studies, we found it logical to compare the efficiency of the CSS maker system against previous functional marker systems. Recently Ref. [Citation52] reported that the CBDP marker system exhibited high genetic diversity among fifty durum wheat genotypes, with a similarity of 96%, which is considered relatively high compared to our results. Moreover Patidar et al. [Citation53] found that SCoT markers exhibited a moderate level of genetic diversity identification and, consequently, molecular phylogeny precision (similarity between lines of 70%) in the NPT lines of rice.
For the CSS marker system efficiency and reliability, our results demonstrated that the PIC, Heterozygosity (H), Effective Multiplex Ratio (EMR), Marker Index (MI), Discrimination Power (D) and Resolving Power (R) parameters revealed relatively positive indications. However, particularly the PIC, EMR and R parameters of RAPD and SSR markers in sesame [Citation54] and the PIC and H parameters of silicoDArT and SNP markers in sesame revealed fewer positive indications when compared to our results [Citation55]. Likewise, the PIC and R parameters of ISSR, SRAP and SSR in faba bean [Citation56], and the EMR, MI, D and R parameters of SCoT and CBDP markers in Centaurea species [Citation43] showed relatively lower efficiency indications compared to our results.
In contrast, the PIC and H parameters showed higher values in faba bean based on SNP markers [Citation57] and in Centaurea species based on SCoT and CBDP markers [Citation43]. Moreover, other studies revealed higher values of PIC in sesame based on InDel markers [Citation58], in addition to the EMR parameter of SCoT markers in rice [Citation53]. The CSS markers developed in this study demonstrated consistent discrimination between different plant genomes.
Ultimately, our study represents the first report to develop a cyclotide subfamily-specific (CSS) marker technique that exhibits a semi-conservative design for targeting and screening cyclotides within a wide range of plant species with the ability to identify each cyclotide subfamily.
Conclusions
Our study presented the CSS marker system with a clear ability to screen and characterize different cyclotide subfamilies through an in silico mining analysis (in silico PCR and in silico profiling analysis) of 15 plant genomes/transcriptomes. Subsequently, the developed CSS markers were validated by PCR in vitro analysis of 19 genotypes belonging to 7 plant species. Additionally, the effectiveness of this marker system was assessed via molecular phylogeny analysis and efficiency parameter evaluation, which showed its precision, reliability, and efficiency in genetic diversity analysis. Eventually, the CSS marker system could be useful in cyclotide subfamily screening within a broad range of plant species without any prior knowledge requirement or sequencing, which can provide a source for cyclotide-based drug design by speeding up the identification of the suitable cyclotide subfamily.
Author contributions
MAMA: conceptualized project and acquired funding. AS: lab work. AS and MAMA: bioinformatics analyses. AS, and EAE: data curation. AS, MAMA, and EAE: data analyses. AS and MAMA: writing—original draft preparation. AS, MAMA, RMG and EAE: writing—review and editing. AS and MAMA: visualization. MAMA, RMG and EAE: supervision. MAMA: project administration. All authors have read and agreed to the published version of the manuscript. All authors contributed to the article and approved the submitted version.
Supplemental Material
Download Zip (1.4 MB)Acknowledgments
The authors would like to sincerely thank the administration of the Agricultural Genetic Engineering Research Institute (AGERI), as well as the administration of the Agricultural Research Center (ARC), Egypt, for their continued support.
Data availability statement
The authors agree to make data and materials supporting the results or analyses presented in their paper available upon reasonable request.
Additional information
Funding
References
- Jagadish K, Camarero JA. Recombinant expression of cyclotides using split inteins. Split inteins. New York (NY): Humana Press; 2017. p. 41–55.
- Gran L. An oxytocic principle found in Oldenlandia affinis DC. Medd nor Farm Selsk. 1970;12(173):80.
- Craik DJ, Daly NL, Bond T, et al. Plant cyclotides: a unique family of cyclic and knotted proteins that defines the cyclic cystine knot structural motif. J Mol Biol. 1999;294(5):1327–1336.
- Park S, Yoo KO, Marcussen T, et al. Cyclotide evolution: insights from the analyses of their precursor sequences, structures and distribution in violets (Viola). Front Plant Sci. 2017;8:2058.
- Weidmann J, Craik DJ. Discovery, structure, function, and applications of cyclotides: circular proteins from plants. J Exp Bot. 2016;67(16):4801–4812.
- Ojeda PG, Cardoso MH, Franco OL. Pharmaceutical applications of cyclotides. Drug Discov Today. 2019;24(11):2152–2161.
- Kaas Q, Craik DJ. Analysis and classification of circular proteins in CyBase. Pept Sci. 2010;94(5):584–591.
- Craik DJ, Mylne JS, Daly NL. Cyclotides: macrocyclic peptides with applications in drug design and agriculture. Cell Mol Life Sci. 2010;67(1):9–16.
- Craik DJ, Čemažar M, Wang CK, et al. The cyclotide family of circular miniproteins: nature’s combinatorial peptide template. Peptide Science: original Research on Biomolecules. 2006;84(3):250–266.
- Pränting M, Lööv C, Burman R, et al. The cyclotide cycloviolacin O2 from Viola odorata has potent bactericidal activity against Gram-negative bacteria. J Antimicrob Chemother. 2010;65(9):1964–1971.
- Ireland DC, Colgrave ML, Nguyencong P, et al. Discovery and characterization of a linear cyclotide from Viola odorata: implications for the processing of circular proteins. J Mol Biol. 2006;357(5):1522–1535.
- Nguyen GKT, Lian Y, Pang EWH, et al. Discovery of linear cyclotides in monocot plant Panicum laxum of Poaceae family provides new insights into evolution and distribution of cyclotides in plants. J Biol Chem. 2013;288(5):3370–3380.
- Ravipati AS, Henriques ST, Poth AG, et al. Lysine-rich cyclotides: a new subclass of circular knotted proteins from Violaceae. ACS Chem Biol. 2015;10(11):2491–2500.
- Daly NL, Clark RJ, Plan MR, et al. Kalata B8, a novel antiviral circular protein, exhibits conformational flexibility in the cystine knot motif. Biochem J. 2006;393(Pt 3):619–626.
- Hernandez JF, Gagnon J, Chiche L, et al. Squash trypsin inhibitors from Momordica cochinchinensis exhibit an atypical macrocyclic structure. Biochemistry. 2000;39(19):5722–5730.
- Colgrave ML, Craik DJ. Thermal, chemical, and enzymatic stability of the cyclotide Kalata B1: the importance of the cyclic cystine knot. Biochemistry. 2004;43(20):5965–5975.
- Cao P, Yang Y, Uche FI, et al. Coupling plant-derived cyclotides to metal surfaces: an antibacterial and antibiofilm study. Int J Mol Sci. 2018;19(3):793.
- Du Q, Huang YH, Wang CK, et al. Mutagenesis of bracelet cyclotide hyen D reveals functionally and structurally critical residues for membrane binding and cytotoxicity. J Biol Chem. 2022;298(4):101822.
- Vilas Boas LCP, Campos ML, Berlanda R, et al. Antiviral peptides as promising therapeutic drugs. Cell Mol Life Sci. 2019;76(18):3525–3542.
- Strömstedt AA, Park S, Burman R, et al. Bactericidal activity of cyclotides where phosphatidylethanolamine-lipid selectivity determines antimicrobial spectra. Biochim Biophys Acta Biomembr. 2017;1859(10):1986–2000.
- Gruber CW, O’Brien M. Uterotonic plants and their bioactive constituents. Planta Med. 2011;77(3):207–220.
- Pinto MEF, Najas JZG, Magalhães LG, et al. Inhibition of breast cancer cell migration by cyclotides isolated from Pombalia calceolaria. J Nat Prod. 2018;81(5):1203–1208.,
- Aboye TL, Ha H, Majumder S, et al. Design of a novel cyclotide-based CXCR4 antagonist with anti-human immunodeficiency virus (HIV)-1 activity. J Med Chem. 2012;55(23):10729–10734.
- Gründemann C, Stenberg KG, Gruber CW. T20K: an immunomodulatory cyclotide on its way to the clinic. Int J Pept Res Ther. 2019;25(1):9–13.
- Camarero JA, Campbell MJ. The potential of the cyclotide scaffold for drug development. Biomedicines. 2019;7(2):31.
- Bobey AF, Pinto MEF, Cilli EM, et al. A cyclotide isolated from Noisettia orchidiflora (Violaceae). Planta Med. 2018;84(12-13):947–952.
- Camarero JA. Rapid screening of cyclotide-based libraries for the selection of potent E3 ligase antagonists. Cancer Research. 2019;79(13_Supplement):4841–4841.
- Kalmankar NV, Venkatesan R, Balaram P, et al. Transcriptomic profiling of the medicinal plant Clitoria ternatea: identification of potential genes in cyclotide biosynthesis. Sci Rep. 2020;10(1):1–20.
- Rajendran S, Slazak B, Mohotti S, et al. Screening for cyclotides in Sri Lankan medicinal plants: discovery, characterization, and bioactivity screening of cyclotides from Geophila repens. J Nat Prod. 2023;86(1):52–65.
- Rizwan Z, Aslam N, Zafar F, et al. Isolation of novel cyclotide encoding genes from some Solanaceae species and evolutionary link to other families. Pakistan Journal of Agricultural Sciences. 2021;58(1):169–177.
- Khoshkam Z, Zarrabi M, Sepehrizadeh Z, et al. Reporting a transcript from Iranian Viola tricolor, which may encode a novel cyclotide-like precursor: molecular and in silico studies. Comput Biol Chem. 2020;84:107168.
- Craik DJ, Malik U. Cyclotide biosynthesis. Curr Opin Chem Biol. 2013;17(4):546–554.
- Deepshikha V, Rajasekharan PV. Synthesis and characterization of Kalata B2 cyclotide (GLPVCGETCFGGTCNTPGCSCTWPICTRD) on wang resin, as solid support. Open J Med Chem. 2020;10(2):46–55.
- Kalmankar NV, Balaram P, Venkatesan R. Mass spectrometric analysis of cyclotides from Clitoria ternatea Xxx-Pro bond fragmentation as convenient diagnostic of pro residue positioning. Chemistry–An Asian Journal, 16(19), pp.2920-2931. lar and in silico Studies. Comput Biol Chem. 2021;84:107168.
- Shams F, Kanwal N, Tariq S, et al. Cyclopeptide Kalata B12 as HCV-NS5A potent inhibitor. Pak BioMed J. 2022;5(5):267–271.
- Gerlach SL, Göransson U, Kaas Q, et al. A systematic approach to document cyclotide distribution in plant species from genomic, transcriptomic, and peptidomic analysis. Pept Sci. 2013;100(5):433–437.
- Rice P, Longden I, Bleasby A. EMBOSS: the European molecular biology open software suite. Trends Genet. 2000;16(6):276–277.
- Kalendar R. A guide to using FASTPCR software for PCR, in silico PCR, and oligonucleotide analysis. PCR primer design. New York (NY): Humana; 2022. p. 223–243.
- Zhang J, Hua Z, Huang Z, et al. Two blast-independent tools, CyPerl and CyExcel, for harvesting hundreds of novel cyclotides and analogues from plant genomes and protein databases. Planta. 2015;241(4):929–940.
- Mauri M, Elli T, Caviglia G, et al. 2017. RAWGraphs: a visualisation platform to create open outputs. Proceedings of the 12th Biannual Conference on Italian SIGCHI chapter. p. 1–5.
- Hammer Ø, Harper DAT, Ryan PD. Past: paleontological statistics software package for education and data analysis. Palaeontol Electronica. 2001;4(1):4–9.
- Jaccard P. Étude comparative de la distribution florale dans une portion des Alpes et des Jura. Bull Soc Vaudoise Sci Nat. 1901;37:547–579.
- Atia MAM, El-Moneim DA, Abdelmoneim TK, et al. Evaluation of genetic variability and relatedness among eight Centaurea species through CAAT-box derived polymorphism (CBDP) and start codon targeted polymorphism (SCoT) markers. Biotechnol Biotechnol Equip. 2021;35(1):1230–1237.
- Tyler KD, Wang G, Tyler SD, et al. Factors affecting reliability and reproducibility of amplification-based DNA fingerprinting of representative bacterial pathogens. J Clin Microbiol. 1997;35(2):339–346.
- Jennings C, West J, Waine C, et al. Biosynthesis and insecticidal properties of plant cyclotides: the cyclic knotted proteins from Oldenlandia affinis. Proc Natl Acad Sci. 2001;98(19):10614–10619.
- Qin Q, McCallum EJ, Kaas Q, et al. Identification of candidates for cyclotide biosynthesis and cyclisation by expressed sequence tag analysis of Oldenlandia affinis. BMC Genomics. 2010;11(1):1–11.
- Bahramnejad B, Kodari N, Rostamzadeh J, et al. Molecular cloning and characterization of a cyclotide gene family in Viola modesta Fenzl. J Agric Sci Technol. 2015;17(6):1637–1649.
- Simonsen SM, Sando L, Ireland DC, et al. A continent of plant defense peptide diversity: cyclotides in Australian Hybanthus (Violaceae). Plant Cell. 2005;17(11):3176–3189.
- Mulvenna JP, Mylne JS, Bharathi R, et al. Discovery of cyclotide-like protein sequences in graminaceous crop plants: ancestral precursors of circular proteins? Plant Cell. 2006;18(9):2134–2144.
- Muratspahić E, Tomašević N, Nasrollahi-Shirazi S, et al. Plant-derived cyclotides modulate κ-opioid receptor signaling. J Nat Prod. 2021;84(8):2238–2248.,
- Sternberger AL, Bowman MJ, Kruse CP, et al. Transcriptomics identifies modules of differentially expressed genes and novel cyclotides in Viola pubescens. Front Plant Sci. 2019;10:156.
- Etminan A, Pour-Aboughadareh A, Mohammadi R, et al. Applicability of CAAT box-derived polymorphism (CBDP) markers for analysis of genetic diversity in durum wheat. Cereal Res Commun. 2018;46(1):1–9.
- Patidar A, Sharma R, Kotu GK, et al. SCoT markers assisted evaluation of genetic diversity in new plant type (NPT) lines of rice. Bangladesh Journal of Botany. 2022;51(2):335–341.
- Dar AA, Mudigunda S, Mittal PK, et al. Comparative assessment of genetic diversity in Sesamum indicum L. using RAPD and SSR markers. 3 Biotech. 2017;7(1):1–12.
- Negash TT, Tesfaye K, Wakeyo GK, et al. Genetic diversity, population structure analysis using ultra-high throughput diversity array technology (DArTseq) in different origin sesame (Sesamum indicum L.). 2020; DOI: 10.21203/rs.3.rs-103763/v1.
- Mahmoud AF, Abd El-Fatah BES. Genetic diversity studies and identification of molecular and biochemical markers associated with fusarium wilt resistance in cultivated faba bean (Vicia faba). Plant Pathol J. 2020;36(1):11.
- Mulugeta B, Tesfaye K, Keneni G, et al. Genetic diversity in spring faba bean (Vicia faba L.) genotypes as revealed by high-throughput KASP SNP markers. Genetic Resour Crop Evol. 2021;68(5):1971–1986.
- Kizil S, Basak M, Guden B, et al. Genome-wide discovery of InDel markers in sesame (Sesamum indicum L.) using ddRADSeq. Plants. 2020;9(10):1262.