ABSTRACT
The main purpose of this paper is efficiency analysis as well as its quantification in the case of emerging capital markets, by building a new measure of market efficiency. The basic assumption of such markets is the lack of correlation between returns, and therefore the existence of low entropy, the lack of randomness, manifestation of fractality and long-term memory, integrated into a single measure, will indicate the distancing from the state of efficient market. This paper proposes five different estimates (for informational entropy, run test, Hurst exponent, long-term correlation coefficient and fractal dimension) to construct a new measure of market efficiency based on a deviation from the ideal state (expressed by the efficient market). The Capital Market Efficiency Exponent is estimated for nine emerging capital markets and, for comparison, for three developed capital markets, at different stages of development over a 16-year time span.
1. Introduction
Testing the efficiency of different capital markets in various regions is, undoubtedly, a popular topic in financial journals. However, the hypothesis of market efficiency is normally either rejected or not and markets are ranked quite infrequently. In addition, the researchers significantly concentrate on a single method and comment on the results. Also, the whole idea of testing or measuring capital market efficiency has been managing the joint-hypothesis issue (i.e., when we reject the efficiency of a particular market, it might be caused by a wrong assumption of the market’s behaviour) since its beginnings. This issue was also touched on by Eugene Fama (Citation1965) himself.
The Efficient Market Hypothesis (EMH) developed by Fama (Citation1965), with its subsequent developments, is a large research area, since the question is to what extent and from what perspective this theory is realistic. From the perspective of tests’ applicability to these markets, the greatest challenges of EMH concern the following elements:
• | despite the simplicity of the assumptions underlying the EMH, it is surprisingly difficult to test and requires considerable attention when it is applied and when the empirical tests are processed; | ||||
• | tests only occasionally showed evidence for the existence of informational efficiency (Borgesa, Citation2010; Chen & Jarrett, Citation2011; Ito, Noda, & Wada, Citation2016; Urquhart, Citation2014); | ||||
• | the limits that exist related to statistical and mathematical models used to test EMH (Malkiel, Citation2003); | ||||
• | the existence of complex non-linear dynamics and chaos in capital markets (Cajueiro & Tabak, Citation2005; Granero, Segovia, & Pérez, Citation2008; Oprean, Tănăsescu, & Brătian, Citation2014; Oprean & Tănăsescu, Citation2014; Reboredo, Rivera-Castro, Miranda, & García-Rubio, Citation2013). |
To overcome all these problems, this paper proposes five different estimates (one estimate of informational entropy, one estimate of run test as a random test, one estimate of the Hurst exponent, one estimate for the long-term correlation coefficient and one estimate for fractal dimension) to construct a new measure of market efficiency – the Capital Market Efficiency Exponent (CMEE), in order to control the possible correlations and complexity using a single measure.
Another reason to apply the study on emerging markets is the fact that most studies so far have focused on stochastic long-memory behaviour of stock returns in developed capital markets. By contrast, the question of long memory in smaller markets has received little attention. Outside the world’s developed economies, many emerging capital markets in recent years have attracted a great deal of attention from investors and investment funds seeking to diversify further their assets.
In this paper, we try to bypass the problem by defining the efficient market as a martingale, because it is less restrictive. Samuelson (Citation1965) provided the first formal economic argument for ‘efficient markets’ and focused on the concept of a martingale, rather than a random walk (as in Fama, Citation1965). His contribution is perfectly summarised by the title of his article: ‘Proof that properly anticipated prices fluctuate randomly’.
Taking into account this definition, we assume that the returns of a financial asset are serially uncorrelated and with finite variance for the efficient market circumstance. Such a simple definition permits us to utilise different measures of market efficiency, which are described in this article. We utilise the fact that the measures are bounded and subsequently can be utilised to develop an informative norm representing the said deviation from the ideal state. In the end, we propose the CMEE, which takes these statistics into consideration and helps to rank different assets according to their efficiency while using different dynamic properties of the time series in this study.
This paper is structured as follows. Section 2 outlines the context of the paper, respectively some views regarding the classical EMH and the implications of complexity and chaos on capital markets. Section 3 contains the literature review. Section 4 discusses the theoretical framework and methodology of the paper. Section 5 presents and validates the data and the empirical results of the study. Conclusions are given in Section 6.
2. The context: from classical EMH to the implications of complexity and chaos on capital markets
EMH, outlined by Eugene Fama (Citation1965, Citation1970), assumes that capital markets process the economic information in a highly sensitively manner and react quickly to adjust the prices of financial assets. The possibility to predict future returns, starting from the returns noticed in the past, is naturally an interesting point in finance, both from the academic point of view and from a practical standpoint.
In finances great interest is shown in the role of chaos in this area, due to the multitude of information and the interest in detecting predictable patterns. Tests have shown that asset prices on the market, although unpredictable, show a certain trend. The capital market is accepted as a self-similar system in the sense that the components are similar or even identical to the whole. In this regard, the association of capital market with a fractal seems appropriate because if one examines the graphs in stock prices over a period, one may see that they have a similar structure. However, like the fractal, the capital market has a sensitive dependence on initial conditions, which makes the dynamic market systems so difficult to predict. It is argued that although a system can be unpredictable in the short term, it can become deterministic in the long term.
The Fractal Market Hypothesis (Peters, Citation1994, Citation1996) is a replica of efficient markets, but the focus lies not on the market efficiency, but on its stability. Thus, the daily randomness of the market and the anomalies occurring on the market are taken into consideration, and it is suggested that the apparent random evolution of the rate can be decomposed into several periods of evolution, similar to each other, the original series thus becoming mouldable.
Regarding entropy, it is a measure both for the uncertainty and for the complexity of a system, with many applications in physics, in information theory, in biology, and in economics (complexity of a system). In other words, high levels of entropy are produced in situations of deep uncertainty and low levels of entropy are associated with a low-uncertainty situation.
3. Literature review
According to Fama’s (Citation1970) early work, the prices on an efficient capital market should follow a random walk and always fully reflect all available and relevant information. From this early work, an entire literature appeared on testing the efficiency of emerging and developed capital markets.
Using traditional statistical tests, such as autocorrelation tests, most of the EMH tests were based on linear prediction of future changes in stock prices (Brătian & Opreana, Citation2010; Oprean, Citation2012). The weak form of market efficiency is disregarded when the return series display long-range dependence. In view of previous information one can make forecasts for future returns. According to Hurst’s methodology (and co-authors) (Hurst, Black, & Simaika, Citation1965), the discovery of long memory should be heuristically possible by evaluating its intensity, namely the value of parameter H, which fluctuates somewhere around 0 and 1. The H value equivalent to 0.5 means non-appearance of long memory.
Among the first studies on long-range dependence are Mandelbrot’s (Citation1972), who applied range/standard deviation (R/S) analysis to returns, and Greene and Fielitz’s (Citation1977), who claimed that many daily stock return series are characterised by long-term dependence. Long-term memory of assets returns was investigated and demonstrated in many recent works (Adel & Lamia, Citation2012; Ahamed, Kalita, & Tiwari, Citation2015; Anoruo & Gil-Alana, Citation2011; Cajueiro & Tabak, Citation2004, Citation2005; Hull & McGroarty, Citation2014; Kale & Butar, Citation2011; Kristoufek & Vosvrda, Citation2013, Citation2014a, Citation2014b; Lilam, Citation2003; Necula & Radu, Citation2012; Oprean & Tănăsescu, Citation2014; Oprean et al., Citation2014; Pele & Tepus, Citation2011; Plesoianu & Todea, Citation2012; Risso, Citation2008; Tan, Chin, & Galagedera, Citation2014; and many others). Moreover, there are some authors who did not find any significant evidence for long-term memory in common stock returns (Grau-Carles, Citation2005; Oh, Um, & Kim, Citation2006).
Previous studies on efficiency have also applied Shannon’s entropy (Adel & Lamia, Citation2012; Mensi, Citation2012, etc.), some efficiency rankings have been presented (for example, Mensi, Citation2012, ranks efficiency for 26 emerging markets applying Shannon’s entropy; and Baciu, Citation2014, ranks efficiency for 20 European markets) and also a similar efficiency index has been already advanced and applied by Kristoufek and Vosdra (Citation2013, Citation2014a, 2014b).
4. Theoretical framework and methodology of constructing the CMEE
The methodology that is used in this paper for constructing a mathematical model, namely the CMEE, consists of the following steps (Bucur, Citation2014).
Step 1. Setting the goal of mathematical modelling.
In this paper, the aim of mathematical modelling is to create an indicator to measure market efficiency. The efficiency measure is taken as a Euclidean distance from an ideal efficient market situation. For the construction of capital market efficiency measure EI, in this paper the procedure of Kristoufek and Vosvrda (Citation2013) is followed, which is equivalent to the Chebyshev measure, and is easy to apply and interpret by experts in financial or economic fields, by managers, etc.
Step 2. Setting input and output variables in the mathematical model.
In this paper, for the construction of CMEE, five partial indicators are used as input variables.
(1) | Shannon entropy. |
To measure efficiency one can follow two steps. First, the returns are represented in order to be less demanding to identify their changes over the analysed period. Second, the Shannon entropy is applied and computed to measure the amount of information contained in the returns series. Using the symbolic time series analysis (Daw et al., Citation2003), it is possible to get more information from an ordinary time series, as the ordinary information with very different values is transformed into a series symbolised by just a few distinct values.
Taking into account that there is no formal method by which time series symbols are characterised, for this paper it was very helpful to find combinations of the positive and negative values of the returns series. Therefore, the negative returns were denoted by (0) and positive ones by (1). In fact, for this situation, the procedure is a sequence of Bernoulli trials ((0) and (1)). In this way, an N-size time series was defined as (R1, R2, R3, ..., RN), Rt being the security returns at time t, where t = 1, 2, 3, ..., N. The returns were measured by using the first difference of logarithmic values of daily prices.
Then, the series of returns (R1, R2, R3, ..., RN) was transformed into a symbolic series (s1, s2, s3, ..., sN ) in accordance with the following relations:(1)
As a measure of informational efficiency, the Shannon entropy (HS) is implemented, which can take a maximum value of 1 when the process is completely random and a minimum value of 0 when alluding to a sure event. The theoretical expression of HS for two events, p (the probability of having negative returns) and 1 − p (the probability of having positive returns) is given as (Risso, Citation2008): (2)
The maximum value (HS = 1) is obtained for p = 1/2 (maximum uncertainty) and the minimum is obtained when one of the events is a sure event (p = 0 or p = 1). In this case, it means that the market is not informationally efficient and the Shannon entropy value (HS) will be less than 1.
(2) | Run test. |
where n represents the total number of observations, nA stands for the number of observations within the first cycle, and nB stands for the number of the second cycle. R is used to designate the observed number of runs.
The test for the run dependence should be performed by comparing the total number of effective runs with the expected number. H0: E(sequences) = E(R) represents the null hypothesis and a random hypothesis is checked for a two-valued data sequence. The value expressed by H0 shows that the changes that follow are not dependent and they are in random walk. In the case of a high number of observations, its distribution becomes practically equivalent to the normal distribution. Along these lines the normal distribution Z might be used to implement a run test. The following formula is the formula used for the standard score:(5)
In the event that the Z value fundamentally contrasts from the critical value (upper tail), one can dismiss the null hypothesis and infer that the tested index might be anticipated. For this situation, the capital market will not meet the low weak form of market efficiency.
(3) | Hurst exponent. |
In the following, the fact that three different values of Hurst exponent can express three different types of time series is examined.
In the case of H = 0.5, the series being no longer correlated, the events become perfectly random. It is standard Brownian motion. Fractional Brownian motion is a well-known stochastic process where the second-order moments of the increments scale as (Kantelhardt et al., Citation2002; Nualart, Citation2006):(6)
with H ∊ [0, 1] (the symbol ‘~’ is used so that they have the same finite joint distribution functions) (Sánchez et al., Citation2015). The Brownian motion represents the particular case where H = 0.5.
In the case of 0 ≤ H < 0.5, the series is negatively correlated. This type of dynamic series represents the antipersistent fractal Brownian motion (a characteristic of self-adjustment systems), therefore any increase in any of the series terms will entail its decrease at the next moment in time.
In the next case, if 0.5 < H ≤ 1, this signifies that the series is positively correlated and a persistent dynamics is thus encountered, that is, if a value increased (decreased) previously, it will very probably continue increasing (decreasing). As one can see, the intensity of persistent behaviour depends on the value of H.
The relationship between long-term memory and potential efficiency has been discussed in numerous studies (Adel & Lamia, Citation2012; Pele & Tepus, Citation2011; Risso, Citation2008; Taback & Cajuiero, Citation2007, 2009; and many others), and in many cases the less-developed markets were characterised by signs of long-term memory. However, it is also necessary to mention the criticism of using the Hurst exponent, as there are authors who argue that such an exponent different from 0.5 does not necessarily mean such long time correlations as those found in fractional Brownian motion (Bassler et al., Citation2006; McCauley et al., Citation2007). So, for financial time series, even an uncorrelated series can yield H different from 0.5 due to several reasons, such as heteroscedasticity, short-term memory and fat tails. The effects of these are discussed in various papers, e.g., Barunik et al. (Citation2012), Barunik and Kristoufek (Citation2010) and Kristoufek (Citation2012). Similarly, Plesoianu and Todea (Citation2012) confirm that thin trading in emerging markets leads to spurious correlations and an overestimation bias of the Hurst exponent.
It must be noted that Hurst exponent estimators are usually biased by the presence of short-term memory in the underlying process (Barunik et al., Citation2012); Kristoufek, Citation2012). However, this is not an issue for the proposed efficiency measure because when short-term memory (which is of course a form of inefficiency as well) biases the Hurst exponent estimate, it is in turn reflected in the efficiency measure.
This study applies the R/S analysis (using rolling window applied on Generalized AutoRegressive Conditional Heteroskedasticity (GARCH) residuals) for the Hurst exponent estimation. A dynamic measurement of this exponent is chosen, taking into account that market efficiency (predictability) appears to evolve over time (Cajueiro & Tabak, Citation2005). A ‘rolling sample’ approach of N = 100 observations is used to evaluate the Hurst exponent and to check for the presence of long-term linear dependence in stock market volatility. Since this approach makes available several Hurst exponents for each stock market and it is impossible to compare all of them, statistical inference with the means, medians and other statistical measures of these Hurst exponents is made in order to compare their market inefficiency degree.
When using the R/S method, i.e., range/standard deviation, an initial dynamic series is required that stands for the evolution of a natural phenomenon or process. Starting from this point one can determine the next dimension (given that the series is divided into subperiods of length t):(7)
where Xt,N represents the cumulative deviation for N periods, xi is the component i of the dynamic series, and mN is the average of the values xi on N periods. N can be equal to the total number of observations and t ≤ N.
Subsequently, the size of the interval R (range) is determined, as the difference between the maximum and the minimum level reached by the dimensions of Xt,N:(8)
In order to compare different dynamic series, Hurst divides the size R to the standard deviation of initial observations, namely:(9)
Then the ratio is determined:(10)
Then, by regression the Hurst coefficient is determined:(11)
Andrew W. Lo (Citation1991) has discovered that the R/S statistic is sensitive to short-range dependence in financial time series. In order to avoid any short-range dependence in mean and variance of the stock returns, the rolling R/S statistic (a rolling window of N = 100 observations) has to be implemented on the adjusted time series. In line with previous studies, including Cajueiro and Tabak (Citation2004, Citation2005) and others, in this article the GARCH (1, 1) model is used to filter the stock return time series. Consequently, the R/S statistic was implemented on the standardised residuals. The GARCH (1, 1) residual can be used to eliminate or reduce linear dependence degree, that is, autocorrelation, because linear dependence will deviate from Hurst exponent H or easily lead to the first kind of error. In the literature this process is usually called the pre-white noise treatment or trend expunction method.
(4) | Long-term correlation coefficient. |
If the Hurst exponent equals 0.5 the series is no longer correlated, and the events are perfectly random (C = 0). It is standard Brownian motion. In other words, the present cannot influence the future.
(5) | Fractal dimension. |
To sum up, the input variables in the mathematical model are: is the ith measure of efficiency (Shannon entropy HS, run test Z, Hurst exponent H, correlation coefficient C and the fractal dimension D, in our case),
is an estimate of the ith measure,
is an expected value of the ith measure for the efficient market and Ri is a range of the ith measure. As ranges of different measures may vary, they are standardised so that the range is equal to one, implying a unit cube as a resulting space. Therefore, the CMEE is defined on a unit n-dimensional cube with the efficient market in the centre, i.e., CMEE = 0 for the efficient market.
The output variable is the indicator CMEE for market efficiency.
Step 3. Setting the interdependence relationships between variables and, respectively, the mathematical model itself.
The mathematical model of CMEE is (after the procedure proposed by Kristoufek & Vosvrda, Citation2013):(13)
In this particular case, the capital market efficiency measure CMEE is based on five estimates (one estimate of informational entropy, one estimate of run test, one estimate of Hurst exponent, one estimate for correlation coefficient and one estimate for the fractal dimension). For our specific case, CMEE is defined as:(14)
where HS is estimated informational entropy, Z is estimated value of run test, HR/S is estimated Hurst exponent based on R/S methodology, C is estimated correlation coefficient and D is estimated fractal dimension.
Step 4. Interpretation of mathematical model results on a scale.
The result of the mathematical model, the output variable CMEE, can be interpreted according to Table .
Table 1. Interpretation of the results.
5. Data and empirical results
The data used in this article represent daily stock market quotes of the most traded indices in the nine emergent capital market countries, respectively, five European Union (EU) countries and the four BRIC countries (Brazil, Russia, India and China; South Africa was not included in the study because its main exchange, JSE Limited, demutualised and listed on its own exchange in 2005). In Table there is a short presentation of these indices.
Table 2. The presentation of indices series.
In this paper, we also investigated the relationship between capital market efficiency and economic development level using the data sample from the emerging markets in the study. According to some authors (Hull & McGroarty, Citation2014) economic development levels and stock market efficiency are closely related. In order to verify this, we use the FTSE (2015) classification of advanced, secondary or frontier emerging markets. FTSE formally reviews country classification within its global equity indices on an annual basis each September using a comprehensive, transparent and consistent methodology. The main criteria for FTSE (2015) classification are: market and regulatory environment, custody and settlement, dealing landscape, derivatives, and size of market. In this study, the countries that meet the ‘advanced’ criteria are Brazil, Czech Republic, Hungary and Greece. The ‘secondary’ emerging markets are China, India and Russia, while Romania and Croatia are considered to be frontier countries (see Table ).
In order to allow more interesting comparisons and an enhanced understanding of stock market efficiency, we applied this study also in the case of developed capital markets, namely in the case of German, French and Spanish capital markets. Information has basically been gathered from the stock markets’ own sites. They are registered daily values over a 16-year time range, January 2000–January 2016. The information comprises an average of 4000 daily values of every index price.
5.1. Applying information entropy to emerging capital markets
Using the methodology outlined earlier to determine the Shannon entropy, these emerging countries are rated on the basis of the entropy value obtained, as shown in Figure .
The results obtained show that, in general, all nine emerging countries and the three developed markets are characterised by weak informational efficiency. However, the most efficient capital market in terms of information seems to be the Croatian market, followed by the French and Hungarian markets. The least effective market in terms of information, using the Shannon entropy as an indicator of appreciation, is the Russian capital market, which has the lowest entropy value.
5.2. Run test analysis
The results of the basic parameters for the run test applied to indices time series are given in Table . It may be concluded that the Romanian capital market does not meet the quality of efficiency. It is not efficient in light of the fact that the stock price changes can be forecast. Additionally, India, Russia, Greece, Germany and France (at a critical-level median) present an unexpected number of sequences lower than the total number, subsequently they clearly reject the hypothesis of random walk. By results, in this period, all the other capital markets: China, Brazil, Hungary, The Czech Republic, Croatia and Spain, are weak-formed.
Table 3. The run test for the emerging capital markets indices.
5.3. Hurst exponent analysis
In order to test the presence of long-term linear dependence in stock market volatility over time, the Hurst exponent is calculated from a rolling window of N = 100 observations to process the extent of inefficiency. The GARCH (1, 1) model is employed in order to filter the stock return time series. In Tables , and ) the descriptive statistics for these Hurst exponents are shown for each of the 12 indices.
Table 4a. Descriptive statistics for Hurst exponent (applied on GARCH residuals, rolling window of 100 observations) – BRIC capital markets.
Table 4b. Descriptive statistics for Hurst exponent (applied on GARCH residuals, rolling window of 100 observations) – European emergent capital markets.
Table 4c. Descriptive statistics for Hurst exponent (applied on GARCH residuals, rolling window of 100 observations) – European developed capital markets.
From these tables, one can see that the mean estimations of the Hurst exponent range from 0.756 (France) to 0.835 (Romania), while the median values of the Hurst exponent range from 0.762 (France) to 0.834 (Romania). It is also pointed out that the Hurst exponents are constantly above 0.5, demonstrating the presence of long-range dependence on stock market returns. The highest standard deviation of the Hurst exponent is attributed to Croatia’s market, whereas the lowest standard deviation is a feature of the French Hurst exponent. Besides, the skewness and kurtosis statistics reveal that the Hurst exponents are not normally distributed. Almost all markets evince significant deviations from normality.
In order to ensure that the variation of the Hurst statistics over time is not due to noise, this preliminary finding is supported by testing normality. In this paper, in order to test the normality of Hurst exponent series, the Kolmogorov–Smirnov test (statistics D) and Shapiro–Wilk test (statistic W) are used initially (shown in Table ).
Table 5. Tests of normality for the series of Hurst exponents.
To interpret the results, the following decision rule is used: if p (Sig) is greater than 0.05, then the hypothesis of normality of distribution is accepted for large samples and the conclusion is considered uncertain in the case of small samples (Wiener et al., Citation2003). The values obtained in Table show that the distribution of the values of Hurst exponent series does not follow the normal distribution.
Another test of normality is also performed through the detrended normal QQ-plot, which charts the observed values against the normal distribution in our case. The results of this normality test are reported in Figure (a–c). The plots show some deviation from the normal distribution, particularly at the lower and higher ends. Our overall conclusion is that this distribution of these Hurst exponents is not normal and therefore one should analyse the medians of the Hurst exponents in order to compare these indices.
Figure 2. (a) Detrended normal QQ-plot for Hurst exponents in BRIC capital markets. (b) Detrended normal QQ-plot for Hurst exponents in European emergent capital markets. (c) Detrended normal QQ-plot for Hurst exponents in European developed capital markets. Source: According to the authors’ calculations.
In Figure , the distancing of each individual capital market from market efficiency is presented graphically (in the figure, the centre point corresponds to a value of 0.5 for the Hurst exponent). Subsequent to the situation presented in this figure, according to the median value of the Hurst exponent, one may notice, as an overall trend, that all rate series are persistent, since the value of the Hurst exponent ranges between 0.5 and 1.
Figure 3. The situation of capital markets according to Hurst exponent values. Source: According to the authors' calculations.
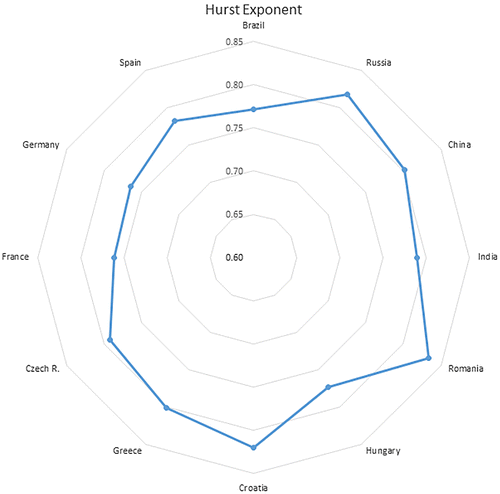
As mentioned earlier, the Hurst exponents’ behaviour exhibits large deviation from the Gaussian distribution. The non-parametric tests could be used (non-parametric tests do not make assumptions about a specific distribution) for equality of medians for the different sample markets in order to test whether these rankings are meaningful. The results are summarised in Table and they suggest significance of the difference between the median values of the Hurst exponents among all the selected non-parametric tests at the 1% significance level, and consequently our ranking is meaningful.
Table 6. Test for equality of medians between series.
5.4. Long-term correlation coefficient and fractal dimension
In Table the long-term correlation coefficient and the fractal dimension are computed for all the indices and these stock markets are ranked according to these indicators, using medians for the calculated Hurst exponents.
Table 7. Indices classification according to the value of Hurst exponent, correlation coefficient and fractal dimension.
Subsequent to the classification displayed in Table , one may see that the developed capital markets in France and Germany, followed by the emerging countries Brazil and Hungary are closest to the random walk, a characteristic of EMH, accordingly it can be said that they are the less inefficient stock markets. On the other hand, the capital markets in Romania, Croatia and Russia prove to be furthest from the random walk, accurately observing the fractal Brownian motion, and thus being the most inefficient markets. These outcomes reinforce our previous findings and suggest that this fractal exponent may be useful in surveying the stage of stock market inefficiency.
5.5. Capital Market Efficiency Exponent
The efficiency of the nine stock market indices is analysed. The results for CMEE are summarised in Figure , which graphically shows the distancing of each individual capital market from market efficiency (in the figure, the centre point corresponds to a value of zero for CMEE). The most efficient markets turn out to be the Hungarian, Brazilian and Spanish capital markets and the least efficient are the Greek and Romanian markets. According to the scale proposed in Section 4 for the interpretation of the mathematical model, the only capital market characterised by high efficiency is the Hungarian market, while the Russian, Indian, Romanian and Greek capital markets are the least efficient ones. All the other markets are characterised by medium efficiency.
In conclusion, the developed capital markets have an average CMEE of 2.42, the advanced emerging markets’ return series have an average CMEE of 2.51, while the secondary emerging markets’ return series have an average CMEE of 3.35. By contrast, the frontier markets have an average CMEE of 4.12, which suggests that the least efficient are these frontier markets. In conclusion, we found that, indeed, economic development levels and stock market efficiency are closely related, the developed capital markets being the most efficient compared with the advanced emerging markets, secondary and frontier emerging countries selected and analysed in this paper.
Following the results, the developed capital markets are more efficient compared with the emerging BRIC capital markets, with an average CMEE of 2.785, and the emerging European countries selected and analysed in this paper (with an average CMEE of 3.442).
Empirical evidence of emerging markets has opened opportunities for portfolio investors to exploit the predictability in these markets, and these results confirm other results in the existing literature (Chong et al., Citation2010; Karamchandani et al., Citation2014).
6. Conclusions
The topic of EMH legitimacy in capital markets has been investigated in much research. The limitations posed on many widely used estimating tools due to the impact of non-linear dynamics in stock markets can be overcome by replacing or supplementing those tools with the ones of econophysics, such as the Hurst exponent or Shannon entropy.
In this paper, a measure of market efficiency has been developed based on deviation from the perfect state (expressed by the efficient market). For this measure of market efficiency five different estimates have been proposed (one estimate of informational entropy, one estimate of run test, one estimate of Hurst exponent, one estimate for correlation coefficient and one estimate for fractal dimension).
Entropy is seen as a measure of informational efficiency of capital markets. Likewise, in order to find out whether the indices exhibit random behaviour or whether they follow a specific growth pattern, the run test is used. This analysis applied the R/S analysis method with rolling window applied on GARCH residuals to estimate the Hurst exponent H. In order to measure the correlation between recent and previous observations (also called long-term memory character), it used the correlation coefficient evinced throughout the time range subject to analysis. It is also used the relation between fractal dimension D (used to identify whether the system is random) and Hurst exponent. To sum up, the tests performed in this paper indicate that the returns of these emerging stock markets are series submitting to fractal distribution. Fractal market means that there is memory, enhancement and continuity in the variation of the stock market. The changes in asset prices increase and continue on the basis of the previous state.
The final obtained results show that the most efficient market, with the lowest value of CMEE, is the Hungarian capital market, and the least efficient, with the highest value of CMEE, is the Greek capital market.
In order to allow more interesting comparisons and an enhanced understanding of stock market efficiency, we also applied this study in the case of developed capital markets, namely the German, French and Spanish capital markets. The results obtained in our study, like the results from most studies already undertaken in this regard (for example, Baciu, Citation2014; Hull & McGroarty, Citation2014; Kitt, Citation2003; Podobnik et al., Citation2006; Wright, Citation2001, show that even though the series of developed markets usually possess only short or no memory, emerging markets exhibit a different behaviour.
Then the question arises: why do emerging markets act unlike developed markets? This phenomenon deserves further study; a possible clarification is the following. In stock markets, short-term moves are described by the psychology of crowd behaviour. In developed markets, investors have seen drops and rises of indices and they behave more rationally: when the index is down, they are entering the market to buy; when the index is up, they are selling. This adjusts the market, yet makes short-term randomness in prices, which leads to lower Hurst exponents. In emerging markets, local investors are not experienced with financial markets and they behave more unpredictably. They can buy when prices are rising and create bubbles, or they can sell when prices are dropping, and create crashes.
Also, the absence of long-memory dynamics can be rationalised for developed stock markets on the grounds that they are informationally efficient. Prices tend to reflect all publicly available information and any new one is fully arbitraged away. Thin markets, on the other hand, are characterised by various institutional rigidities that perpetuate informational inefficiency and asymmetry (Limam, 2003).
Regarding this study, a possible improvement would be in terms of enlarging the sample of companies in the study to obtain more representative results. This research aims in the future to cover indices from North and Latin America, Western Europe, Asia, Oceania and Africa in order to gain in depth and relevance with the inclusion of emerging markets from several continents.
For investors, the implications of trading on BRIC emerging markets, more efficient compared with the European ones analysed in this study, refer to:
(a) | The more efficient the market, the more random and unpredictable the price evolution is on such a market. This is the result of the fact that agents on the market try to take advantage of the available information, and quickly using this information, they will be quickly incorporated into prices, thus eliminating the opportunities to gain from arbitrage. | ||||
(b) | In an efficient market, equity research and valuation would be a costly task giving no advantages, for the chances of finding an undervalued stock ought to be arbitrary. | ||||
(c) | In an efficient market, a strategy of randomly diversifying across stocks or indexing to the market, conveying practically zero data cost and insignificant execution expenses, would be better than any other technique that created larger data and execution costs. | ||||
(d) | In an efficient market, a strategy of minimising trading, i.e., creating a portfolio and not trading unless cash was needed, would be better than a strategy that required regular trading. |
The financial literature is rich in tests proving the presence or not of informational efficiency; fortunately, many studies have also proved the presence of anomalies. These studies have become important foundations to further advances in theory and research in finance. Indeed, frontier econometric, statistical and mathematical methods to test EMH and other stock market issues are currently being applied in all financial research.
Disclosure statement
No potential conflict of interest was reported by the authors.
References
- Adel, B., & Lamia, S. (2012). Crash occurrence probability and stock market efficiency the indie stock exchange via Shannon. International Journal of Accounting and Financial Reporting, 2(2), 157–167.
- Ahamed, N., Kalita, M., & Tiwari, A. (2015). Testing the long-memory features in return and volatility of NSE index. Theoretical Economics Letters, 5, 431–440. doi:10.4236/tel.2015.53050
- Ahmed, E., Rosser, J. B., Jr., & Uppal, J. Y. (2010). Emerging markets and stock market bubbles: Nonlinear speculation? Emerging Markets Finance and Trade, 46, 23–40. doi:10.2753/REE1540-496X460402
- Anoruo, E., & Gil-Alana, L. A. (2011). Mean reversion and long memory in African stock market prices. Journal of Economics and Finance, 35, 296–308. doi:10.1007/s12197-010-9124-0
- Antoniou, A., Ergul, N., Holmes, P., & Priestley, R. (1997). Technical analysis, trading and market efficiency: Evidence from an emerging market. Applied Financial Economics, 7(4), 361–365. doi:10.1080/096031097333475
- Baciu, O. A. (2014). Ranking Capital markets efficiency the case of twenty European stock markets. Journal of Applied Quantitative Methods, 9, 24–33.
- Barunik, J., Aste, T., Di Matteo, T., & Liu, R. (2012). Understanding the source of multifractality in financial markets. Physica A, 391, 4234–4251.10.1016/j.physa.2012.03.037
- Barunik, J., & Kristoufek, L. (2010). On Hurst exponent estimation under heavy-tailed distributions. Physica A, 389(18), 3844–3855.10.1016/j.physa.2010.05.025
- Bassler, K. E., Gunaratne, G. H., & McCauley, J. L. (2006). Hurst exponents, Markov processes, and nonlinear diffusion equations. Physica A, 369, 343–353.10.1016/j.physa.2006.01.081
- Borgesa, M. R. (2010). Efficient market hypothesis in European stock markets. The European Journal of Finance, 16(7), 711–726.10.1080/1351847X.2010.495477
- Brătian, V., & Opreana, C. (2010). Testing the hypothesis of a efficient market in terms of information – the case of capital market in Romania during recession. Studies in Business and Economics, 5, 79–106.
- Bucur, A. (2014). Modelare şi simulare cu privire la calitate şi managementul calităţii [Modeling and simulation on quality and quality management]. Sibiu: Lucian Blaga University of Sibiu Publishing House. 89–90.
- Cajueiro, D. O., & Tabak, B. M. (2004). Evidence of long range dependence in Asian equity markets: The role of liquidity and market restrictions. Physica A: Statistical Mechanics and its Applications, 342(3–4), 656–664.10.1016/j.physa.2004.05.034
- Cajueiro, D. O., & Tabak, B. M. (2005). Ranking efficiency for emerging equity markets. Chaos, Solitons and Fractals, 23(2), 671–675.10.1016/j.chaos.2004.05.009
- Chen, F., & Jarrett, J. E. (2011). Financial crisis and the market efficiency in the Chinese equity markets. Journal of the Asia Pacific Economy, 16(3), 456–463. doi:10.1080/13547860.2011.589632
- Chong, T. T.-L., Cheng, S. H.-S., & Wong, E. N.-Y. (2010). A comparison of stock market efficiency of the BRIC countries. Technology and Investment, 1, 235–238. doi:10.4236/ti.2010.14029
- Daw, C. S., Finney, E. A., & Tracy, E. R. (2003). Symbolic time series analyses. A Review of Symbolic Analysis of Experimental Data, 79(915), 1–18. doi:10.1063/1.1531823
- Fama, E. (1965). The behavior of stock market prices. Journal of Business, 38, 34–105.10.1086/jb.1965.38.issue-1
- Fama, E. (1970). Efficient capital markets: A review of theory and empirical work. Journal of Finance, 25, 383–417.10.2307/2325486
- Granero, S., Segovia, T., & Pérez, G. (2008). Some comments on Hurst exponent and the long memory processes on capital markets. Physica A, 387, 5543–5551.10.1016/j.physa.2008.05.053
- Grau-Carles, Pilar (2005). Tests of long memory: A bootstrap approach. Computational Economics, 25(1–2), 103–113.10.1007/s10614-005-6277-6
- Greene, M. T., & Fielitz, B. D. (1977). Long-term dependence in common stock returns. Journal of Financial Economics, 4, 339–349.10.1016/0304-405X(77)90006-X
- Hasanov, M., & Omay, T. (2008). Nonlinearities in emerging stock markets: Evidence from Europe’s two largest emerging markets. Applied Economics, 40(20), 2645–2658. doi:10.1080/00036840600970310
- Hull, M., & McGroarty, F. (2014). Do emerging markets become more efficient as they develop? Long memory pedrsistence in equity indices. Emerging Markets Review, 18, 45–61.10.1016/j.ememar.2013.11.001
- Hurst, H. E., Black, R. P., & Simaika, Y. M. (1965). Long-term storage: An experimental study. London: Constable.
- Islam, S. M. N., Watanapalachaikul, S., & Clark, C. (2007). Are emerging financial markets efficient? Some evidence from the models of the Thai stock market. Journal of Emerging Market, 6(3), 1–16.
- Ito, M., Noda, A., & Wada, T. (2016). The evolution of stock market efficiency in the US: A non-Bayesian time-varying model approach. Applied Economics, 48(7), 621–635. doi:10.1080/00036846.2015.1083532
- Kale, M., & Butar, F. B. (2011). Fractal analysis of time series and distribution properties of Hurst exponent. Journal of Mathematical Sciences and Mathematics Education, 5(1), 8–19.
- Kantelhardt, J., Zschiegner, S., Koscielny-Bunde, E., Bunde, A., Havlin, S., & Stanley, E. (2002). Multifractal detrended fluctuation analysis of nonstationary time series. Physica A, 316(1–4), 87–114.10.1016/S0378-4371(02)01383-3
- Karamchandani, M., Mohadikar, S., & Jain, S. (2014). Stock indices of BRIC economies: Explored for non linear dynamics and volatility. IOSR Journal of Economics and Finance, 2, 53–65.10.9790/5933
- Kitt, R. (2003). The importance of the Hurst exponent in describing financial time series. Proc. Estonian Acad. Sci. Phys. Math., 52, 198–206.
- Kristoufek, L. (2012). How are rescaled range analyses affected by different memory and distributional properties? A Monte Carlo study. Physica A, 391, 4252–4260.10.1016/j.physa.2012.04.005
- Kristoufek, L., & Vosvrda, M. (2013). Measuring capital market efficiency: Global and local correlations structure. Physica A, 392, 184–193.10.1016/j.physa.2012.08.003
- Kristoufek, L., & Vosvrda, M. (2014a). Commodity futures and market efficiency. Energy Economics, 42, 50–57.10.1016/j.eneco.2013.12.001
- Kristoufek, L., & Vosvrda, M. (2014b). Measuring capital market efficiency: Long-term memory, fractal, dimension and approximate entropy. Eur. Phys. J. B, 87, 34. doi:10.1140/epjb/e2014-50113-6
- Lilam, I. (2003). Is long memory a property of thin stock markets? International evidence using Arab countries. Review of Middle Eastern Economics and Finance, 1, 56–71. doi:10.2202/1475-3693.1015
- Lim, K., & Brooks, R. (2009). Are Chinese stock markets efficient? Further evidence from a battery of nonlinearity tests. Applied Financial Economics, 19(2), 147–155. doi:10.1080/09603100701765182
- Lo, A. W. (1991). Long-term memory in stock market prices. Econometrica, 59, 1279–1313.10.2307/2938368
- Loc, T. D., Lanjouw, G., & Lensink, R. (2010). Stock-market efficiency in thin-trading markets: The case of the Vietnamese stock market. Applied Economics, 42(27), 3519–3532. doi:10.1080/00036840802167350
- Malkiel, B. G. (2003). The efficient market hypothesis and its critics. Journal of Economic Perspectives, 17, 59–82.10.1257/089533003321164958
- Mandelbrot, B. B. (1972). Statistical methodology for non-periodic cycles: From the covariance to R/S analysis. Annals of Economic and Social Measurement, 1, 259–290.
- Mandelbrot, B. B. (1982). The fractal geometry of nature. New York, NY: W. H. Freeman and Company.
- McCauley, J., Gunaratne, G. H., & Bassler, K. E. (2007). Hurst exponents, Markov processes, and fractional Brownian motion. Physica A: Statistical Mechanics and its Applications, 379(1), 1–9.10.1016/j.physa.2006.12.028
- Mensi, W. (2012). Ranking efficiency for twenty-six emerging stock markets and financial crisis: Evidence from the Shannon entropy approach. International Journal of Management Science and Engineering Management, 7, 53–63.
- Necula, C., & Radu, A.-N. (2012). Long memory in Eastern European financial markets returns. Economic Research, 25(2), 361–378.
- Nualart, D. (2006). Fractional Brownian motion: Stochastic calculus and applications. Proceedings of the International Congress of Mathematicians, Madrid, Spain, 2006, 1541–1562.
- Oh, G., Um, C., & Kim, S. (2006). Long-term memory and volatility clustering in daily and high-frequency price changes. Arxiv preprint physics, 0601174, arXiv:physics/0601174v2.
- Oprean, C. (2012). Testing informational efficiency: The case of UE and BRIC emergent markets. Studies in Business and Economics, 7, 94–112.
- Oprean, C. (2016). This is a chapter. In Nicolescu & Lloyd-Reason (Eds.), Performances and tendencies in organisation management: Information and its entropy – A measure of market complexity (pp. 159–166). World Scientific. doi:10.1142/9789814656023_0018
- Oprean, C., & Tănăsescu, C. (2014). Fractality evidence and long-range dependence on capital markets: A Hurst exponent evaluation. Fractals, 22, 1450010. doi:10.1142/S0218348X14500108
- Oprean, C., Tănăsescu, C., & Brătian, V. (2014). Are the capital markets efficient? A fractal market theory approach. Economic Computation and Economic Cybernetics Studies and Research, 48, 190–205.
- Pele, D. T., & Tepus, A.-M. (2011). Information–entropy and efficient market hypothesis. International Conference of Applied Economics, 463–473.
- Peters, E. E. (1994). Fractal market analysis. New York, NY: Wiley.
- Peters, E. E. (1996). Chaos and order in the capital markets: A new view of cycles, prices, and market volatility. New York, NY: John Wiley and Sons, Inc.
- Plesoianu, A., & Todea, A. (2012). Long memory and thin trading: Empirical evidence from Central and Eastern European stock markets. Oeconomica, 1, 21–27.
- Podobnik, B., Fu, D., Jagric, T., Grosse, I., & Stanley, H. E. (2006). Fractionally integrated process for transition economics. Physica A, 362, 465–470.10.1016/j.physa.2005.09.051
- Reboredo, J. C., Rivera-Castro, M. A., Miranda, J. G. V., & García-Rubio, R. (2013). How fast do stock prices adjust to market efficiency? Evidence from a detrended fluctuation analysis. Physica A: Statistical Mechanics and its Applications, 392(7), 1631–1637. doi:10.1016/j.physa.2012.11.038
- Risso, A. W. (2008). The informational efficiency and the financial crashes. Research in International Business and Finance, 22, 396–408.10.1016/j.ribaf.2008.02.005
- Samuelson, P. (1965). Proof that properly anticipated prices fluctuate randomly. Industrial Management Review, 6, 41–49.
- Sánchez, M. Á., Trinidad, J. E., García, J., & Fernández, M. (2015). The effect of the underlying distribution in Hurst exponent estimation. PLoS One, 10, e0127824. doi:10.1371/journal.pone.0127824
- Shannon, C. E. (1948). A mathematical theory of communication. Bell System Technical Journal, 27, 379–423.10.1002/bltj.1948.27.issue-3
- Taback, B. M., & Cajuiero, D. O. (2007). Are the crude oil markets becoming weak efficient over time? A Test for time-varying long-range dependence in prices and volatility. Energy Economics, 29, 28–36.10.1016/j.eneco.2006.06.007
- Tan, P. P., Chin, C. W., & Galagedera, D. U. A. (2014). A wavelet-based evaluation of time-varying long memory of equity markets: A paradigm in crisis. Physica A, 410, 345–358.10.1016/j.physa.2014.05.044
- Urquhart, A. (2014). The Euro and European stock market efficiency. Applied Financial Economics, 24(19), 1235–1248. doi:10.1080/09603107.2014.924292
- Wiener, U., Isaic-Maniu, A., & Vodă, V. (2003). Aplicaţii ale reţelelor probabilistice în tehnică [Applications of probabilistic networks in technical field]. Bucureşti: Ed. Economică.
- Wright, J. H. (2001). Long memory in emerging market stock returns. Emerging Markets Quarterly, 5, 50–55.