Abstract
This study examines the innovation–growth nexus in the European Union (EU) countries over the period 1993–2011. The system generalised method of moments estimator was used to test whether patent activities and different research and development (R&D) outlays affect economic growth in the old (EU-15) and new (EU-13) member states differently. The authors have found no significant impact of R&D on the growth and positive relationship between patent activities and growth in the EU-13. The results suggest that there may be no single recipe for growth for the EU and raise the question of whether setting common numerical targets in the EU innovation policy makes economic sense.
1. Introduction
The enlargement of the European Union (EU) has far-reaching economic consequences not only for the EU itself but also for the world economy. Measured in terms of goods and services it produces, the EU ranked as the world’s largest single market in 2013 for the first time. Following the recent enlargements, the EU has been transformed from a relatively homogenous organisation of developed and highly developed countries into a rather heterogeneous body. Differences in gross domestic product (GDP) per capita remain significant across European countries. This raises questions about possible measures that can be taken to close this gap. Although the discussion of determinants of economic growth is an evergreen topic, both in theoretical and empirical studies, there is a growing consensus among economists and policy-makers that investment in knowledge, which is at the centre of the endogenous growth process, is a precondition for achieving permanently high growth rates.
After the boom of exogenous growth models in the 1950s and 1960s the growth theory died for almost two decades, leaving a problem of sources of growth unsolved in a ‘black box’ of technology. The work of Romer (Citation1986) and Lucas (Citation1988) began a new strand of research. In these models technological progress is not explicitly modelled and growth goes to infinity due to knowledge spillovers and human capital externalities, which allow escape from diminishing returns present in the exogenous models. By contrast, the endogenous growth models of the first generation developed in the early 1990s by Romer (Citation1990), Grossman and Helpman (Citation1991) and Aghion and Howitt (Citation1992) are based on a basic growth mechanism, which operates through technological progress that is a result of purposeful research and development (R&D) activity. In response to Jones’ (Citation1995) critique those models were altered in various ways. The semi-endogenous models of Kortum (Citation1997), Segerstrom (Citation1998) and Jones (Citation2002) assume diminishing returns to knowledge and require continuous growth of R&D to maintain sustained productivity growth. On the other hand, the Schumpeterian models developed by Aghion and Howitt (Citation1998), Peretto (Citation1998) and Howitt (Citation1999) maintain scale effects from first-generation endogenous growth models. Sustained total factor productivity (TFP) growth is allowed through increasing R&D efforts counteracting the effect of growth in product variety that reduces the productivity effects of R&D activity. Although all endogenous models differ with respect to the nature of innovation, they exhibit similar dynamics and put knowledge at the centre of a never-ending growth process.Footnote1
The nature of knowledge, which was assumed to be both non-rival and non-excludable in exogenous models, naturally leads to questioning why rational agents would devote resources to its development if everyone can use created knowledge freely. To avoid the problem of free-riding, it is assumed that once a design has been produced a firm can obtain an infinitely livedlife patent (Aghion & Howitt, Citation1992; Romer, Citation1990). This means that non-rival designs are at least partially excludable. The owner of a patent gains monopoly power and monopoly profits which provide incentives for further investment in R&D. However, no patent system can provide perfect protection; therefore, some useful knowledge that results from the purposeful actions of profit-seeking firms becomes available to the public. This knowledge reduces the future costs of R&D to other innovators; hence the larger is the stock of R&D today, the cheaper it becomes to conduct R&D in the future. Consequently, endogenous growth models have clear implications for economic policy. Knowledge created as a result of R&D investment helps use existing physical and human capital resources more efficiently. Therefore, R&D expenditures and patent activity should have a positive and persistent effect on growth.
The EU intensifies efforts to strengthen its economic position in the global economy, particularly vis-à-vis the United States, Japan and rapidly growing China. In 2000, the EU adopted the Lisbon strategy, a development plan, the key objective of which was to make Europe ‘the most competitive and dynamic knowledge-based economy in the world’ (CEC, Citation2000). One of the goals set out in the Lisbon agenda was to increase R&D expenditure to 3% of GDP. As it became obvious in 2010 that the strategy failed to meet its self-imposed targets, the ‘Europe 2020’ – new 10-year plan – was designed. The successor to the Lisbon strategy sets out economic growth based on knowledge and innovation as one of its priorities and maintains the 3% objective for R&D intensity.
The aim of this empirical study is to assess the contribution of technological innovation to per capita GDP growth in a panel of EU countries. In light of the above-mentioned facts, we ask and try to answer a series of questions. Is there a relationship between technological innovation and economic growth in the EU? Will the increase in R&D outlays contribute to economic growth? Does the role of technological innovation in fostering growth differ between EU-15 (old member states) and EU-13 (new member states) countries? Does the role of R&D in fostering growth differ when R&D is differentiated by source of funding? We expect the role of innovation in economic growth to be different in old and new member states due to their significant variation in terms of economic development. Figures and show the trends in average GDP per capita growth rates and proxies for technological innovation in the EU-15 and EU-13 countries since 1993.
Figure 2. Average number of European Patent Office applications and R&D intensity. Source: Authors’ calculations.
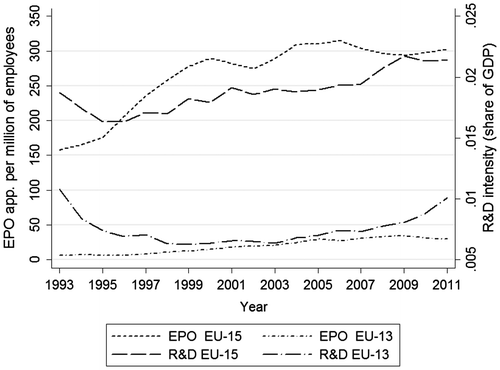
The growth rates are usually higher in the EU-13 than the EU-15. However, R&D intensity is much larger in the case of EU-15 countries, being in the order of two to three times the average levels in the EU-13. The gap in patent activity between old and new member states is even larger.
We investigate the innovation–growth relationship by running separate estimates for old and new member states. Innovation is proxied by R&D outlays and patents. R&D expenditures are used as a proxy for investment in generating new knowledge. Data on patents are an indicator of the creation of new ideas. To account for the fact that knowledge builds up over time, as well as the interaction between the existing stock of knowledge and the costs of future research, we include both current and cumulative R&D and patent activity data in the regressions.
We contribute to the existing literature in several ways. First, to the best of our knowledge this is one of the very few panel data studies on the innovation–growth nexus in the EU at the country level.Footnote2 Second, the originality of our contribution is that we test whether innovation affects economic growth differently in the old and new member states. This is in line with models highlighting that innovation is likely to have a different impact on growth depending on a country’s proximity to the world technology frontier (see, e.g., Acemoglu, Aghion, & Zilibotti, Citation2006; Chu, Cozzi, & Galli, Citation2014). Moreover, we examine the relationship between economic growth and R&D investments differentiated by the source of funding. Finally, part of our contribution lies in paying attention to the endogeneity problem. In order to address this issue properly the system generalised method of moments (GMM) estimator was used in regressions.
The study provides mixed results on the role of innovation in economic growth. Compared with other studies, our contribution differs in one crucial dimension. We did not find any statistically significant relationship between R&D expenditures and economic growth in the EU-15 and EU-13 countries. At the same time, the results concerning patents are partially in line with conventional wisdom. They indicate a significant and positive relationship between patents and growth in the new member states and an insignificant one in the old EU countries. These results suggest that the impact of innovation on economic growth is far more complex than previously assumed, and may have some pertinent implications regarding the EU’s R&D policy. They may also be important with respect to the ongoing process of introducing the unitary European patent, which is intended to provide uniform protection in all 25 participating member states.
The remainder of the paper is organised as follows. Section 2 provides a brief review of the related empirical literature. Section 3 describes the data and methodology. Empirical results and discussion are presented in Section 4. Section 5 concludes.
2. Brief review of the literature
Starting from the early 1990s, numerous empirical analyses based on endogenous growth models have been conducted. An important issue in the empirical work is how to measure innovation at the macroeconomic level. R&D expenditures and patent statistics are often used as proxies for innovation ‘input’ and ‘output’, mainly due to their availability for relatively broad samples of countries. Pioneering work was carried out by Lichtenberg (Citation1992), who examined the relationship between R&D investment and productivity in a cross section of 74 countries from 1964 to 1989. The effect of privately funded R&D investment on economic growth was found to be positive. Government-sponsored R&D had a negative influence on growth in some specifications; however, Lichtenberg indicated that his findings should be interpreted with caution and that the effect of R&D investment may depend more on its objectives than on the source of funding. The other early study on R&D was performed by Goel and Ram (Citation1994), who explored the effect of R&D outlays on output growth from 1960 to 1985 in a cross section of 52 countries. The authors reported estimates for the full sample and for the subsample of less-developed countries. The results suggested that the R&D expenditures are correlated with growth only in less-developed countries. The earliest panel data analyses for a relatively large number of countries were performed by Coe and Helpman (Citation1995), who studied 22 countries in the period 1970–1990, and Park (Citation1995), who studied 10 Organisation for Economic Cooperation and Development (OECD) countries from 1970 to 1987. Park provides evidence that domestic and foreign productivity growth is positively related to domestic private investment in R&D. Coe and Helpman (Citation1995) provide support for a positive relationship between TFP and R&D stocks, including both a country’s own and that of its trade partners. Engelbrecht (Citation1997) assessed the robustness of Coe and Helpman’s results, adding the human capital variable to their model. His findings are consistent with the original results, although the estimated coefficients for R&D are smaller. Guellec and Van Pottelsberghe de la Potterie (Citation2004) used panel data for 16 OECD countries over the period 1980–1998 to examine whether R&D – carried out by the business sector, the public sector and foreign firms – is positively related to TFP. Their results suggest that all types of R&D are significant determinants of productivity growth, although the impact of business R&D increased while the impact of public R&D decreased over the time period analysed. The authors also discuss the reasons why the effect of public R&D on output might be hard to capture directly in empirical analyses. Finally, Ang and Madsen (Citation2011) considered the role of R&D in the growth experiences of the six Asian miracle economies from 1953 to 2006. Their results provide strong evidence that economic growth was driven by R&D intensity over the period analysed.
Another strand of empirical literature tests whether there is a relationship between patent activity and economic growth. Porter and Stern (Citation2000) contribute to a better understanding of endogenous growth by estimating the ideas production function. Using data for 16 OECD countries, the authors examined the determinants of the United States Patent and Trademark Office (USPTO) patents flow. The results obtained show that the ideas production function increases proportionally with the existing stock of knowledge and that aggregate output is positively related to patent stock. However, the worldwide knowledge stock may have a negative effect on the ideas production function since it raises the bar for producing domestic technology that is new at the international level. Ulku (Citation2007) used data from 41 countries to examine the relationship between innovation and per capita output. Analyses were conducted separately for the high-income, low-income, large-market and small-market subsamples for OECD and non-OECD countries. The results show that innovation, proxied by USPTO patent flows, raises per labour GDP only in the high-income OECD countries, while in the case of non-OECD countries it has a significant impact on GDP per labour in all subsamples, except for the low-income one. The findings also suggest that developing countries benefit more from innovation than developed countries in terms of increasing per labour GDP. Madsen (Citation2008) demonstrated that in 16 OECD countries the effect of international patent stock on growth was positive during the period 1883–2004. In another paper Madsen (Citation2010) used both R&D and patents as measures of innovative activity in 21 OECD countries in different periods of time. Change in the flow and stock of patents and patenting intensity had a positive effect on productivity growth. Barcenilla-Visús, Gómez-Sancho, López-Pueyo, Mancebón, and Sanaú (Citation2013) examined data for 15 OECD countries from 1989 to 2004 to assess the role of TFP in explaining economic growth. The results show that technology, proxied by domestic R&D stocks or, alternatively, by the stock of patents, stimulates the variation in technological change.
Although the contribution of innovation to economic growth has been widely analysed for different samples of countries (mainly OECD member states), the overall impression is that the majority of authors made data availability the only criterion for country selection. Such an approach is quite understandable as it expands the number of observations. However, from the policy-making perspective studying the relationship of interest in a group of integrated countries (such as the EU) that share some common characteristics, obey horizontally institutionalised organisational rules and adopt common policies may be more informative and may provide more useful policy prescriptions. This is what we do in the following sections.
3. Data and methodology
3.1. Data
The analysis was performed over the period 1993–2011 at the macroeconomic level for 28 EU countries separated into two subsamples (EU-15 and EU-13). The time period of analysis was chosen to maximise the number of observations, given data availability. Since some of the data is not available, the panel is unbalanced and the number of observations depends on the choice of explanatory variables. Even though most panel data studies of economic growth use averaged data over certain time spans (usually 5 or 10 years), it was not possible to use averages for a panel such as ours given the relatively short time dimension of our data set (T = 19). We use annual data instead, as it provides us with sufficient number of observations to estimate separate models for two relatively small subsamples. Although we acknowledge some limitations of this approach, in particular the fact that growth regressions based on annual data are exposed to the effects of short-term business cycle fluctuations, using stock variables as regressors helps to overcome this problem, at least to some extent.
The standard specification in growth empirics can be written in a panel variant as:(1)
where Δyi,t is the growth rate of GDP per capita between time t – 1 and t, yi,t is the log of real GDP per capita, xi,t is a vector of determinants of economic growth, ηi is a country-specific effect that allows controlling for unobserved time-invariant country heterogeneity, μt is a time-specific effect and εi,t is an error term (Durlauf, Johnson, & Temple, Citation2005).
Equation Equation(1)(1) can be equivalently rearranged as a dynamic model with a lagged dependent variable on the right-hand side:
(2)
Our set of regressors is comprised of ‘robust regressors’ from Levine and Renelt (Citation1992), openness and measures of innovation. Robust regressors include the investment rate as a share of GDP (INVESTMENT) and the log of lagged level of GDP per capita (logGDP(t − 3)).Footnote3 Openness (logOPENNESS), calculated as the log of the sum of exports and imports to GDP, allows controlling for the possibility to import technology embodied in capital goods from neighbouring countries and from the world’s most R&D-intensive countries (Eaton & Kortum, Citation2001). This is particularly important in an integrated area such as the EU where innovation can easily diffuse. By including this measure in regressions, we take into consideration the effects of the restructuring process caused by foreign trade – a country’s innovativeness increases as a result of import competition (Bloom, Draca, & Van Reenen, Citation2016) and the learning-by-exporting effect (Damijan, Kostevc, & Polanec, Citation2010; Liu & Buck, Citation2007). From this point of view, a country’s R&D outlays show their ‘second face’ by facilitating technology transfer from abroad (Griffith, Redding, & Van Reenen, Citation2004). Technological innovation in our model is proxied by the current and cumulative patents (per employee) and R&D expenditures (relative to GDP). Further, we distinguished between private and public sources of R&D funding and tried to answer the question whether private and public R&D investments differ in fostering growth. The data on GDP, population, number of persons engaged, investment and openness are from PWT 8.0 (Feenstra, Inklaar, & Timmer, Citation2015), whereas the data on innovation are from the Eurostat Database.
Finally, we estimate the following model to see whether the data provide support for the innovation–growth relationship:(3)
where Z denotes the ‘innovation’ variable.
The data on innovation expenditures contain total intramural R&D expenditures (RD_EXPENDITURES) as well as R&D by source of funds: gross domestic expenditure on R&D funded by the business enterprise sector (RD_BUSINESS) and gross domestic expenditure on R&D funded by the government sector (RD_GOVERNMENT) (these sectors have the highest shares in total R&D outlays). Data on patent activity include European Patent Office (EPO) (logEPO_FLOW) applications and patents granted by the USPTO (logUSPTO_FLOW) by priority year at the national level.
Stock variables were computed using the perpetual inventory method, employing the formulae:(4)
(5)
where S0 stands for the initial stock, Ft is the flow at time t, r is the compound annual growth rate of flow (computed for each country for the period analysed) and δ is the depreciation rate (20%).Footnote4 Stock variables include R&D total stock (RD_TOTAL), stock of privately/publicly funded R&D (RD_PRIVATE/RD_PUBLIC), EPO applications stock (logEPO_STOCK) and stock of patents granted by the USPTO (logUSPTO_STOCK). The stock of private R&D was calculated using data on R&D expenditures by source of funds, and comprises business enterprises, private non-profit and abroad sectors. Stock of public R&D was calculated as the sum of government and higher education sectors.Footnote5
3.2. Methodology
Modelling the dynamic panel data has several advantages. The most important ones are the increase in the number of observations and the ability to control for individual unobserved heterogeneity. However, the use of such models introduces a set of problems, therefore special attention should be paid to the estimation method. The first method widely used in growth empirics is the fixed-effects estimator (henceforth FE). As Durlauf et al. (Citation2005) point out, the motivation for using this estimator is the fact that the estimates will not be biased by any omitted variables which are constant over time. However, the use of this estimator brings with it certain costs. Measurement error is typically a problem, since the FE estimator ignores the between-country variation and the reduction in bias implies higher standard errors. Moreover, Nickell (Citation1981) proved that the FE estimator is not consistent in autoregressive panel data models with finite T, since inclusion of the lagged dependent variable leads to a correlation between regressors and the error term. Therefore, dynamic panel data estimation suffers from the Nickell bias. Although this bias is a declining function of T (bias of order 1/T), we cannot guarantee that the bias would be eliminated in our case. For this reason we use the bias-corrected least squares dummy variable (LSDV) estimator (henceforth LSDVC) as the first method of estimation. This method, developed for unbalanced dynamic panels by Bruno (Citation2005), helps to overcome the problem mentioned above and estimates a bootstrap variance–covariance matrix for the corrected estimator. We use the Blundell–Bond estimator to initiate bias correction and take the first-order term of the approximation evaluated at the true parameter values, which as Bruno (Citation2005) shows is usually capable of accounting for more than 90% of the actual bias. In order to bootstrap the estimated standard errors we undertook 100 repetitions of the procedure. The two estimation methods outlined above suffer, however, from one major limitation. None of them can be applied in the presence of endogenous regressors. The nature of some of our regressors gives the strong potential for endogeneity, e.g., investment is likely to be an endogenous variable as higher output may lead to higher investment. There is also a similar problem with R&D: the direction of causality in the R&D expenditure–growth relation is not obvious.
The most popular alternative strategy to address potential endogeneity is to use one of the class of generalised method of moments estimators. This is the second method of estimation that we employ. The GMM estimator developed by Arellano and Bond (Citation1991) removes fixed effects, taking first differences of Equation Equation(2)(2) in the first step. Then the differenced right-hand-side variables are instrumented with their lagged levels. A serious drawback of this estimator is that the lagged levels are likely to be weak instruments if series are highly persistent, as output and stock variables are in our case.Footnote6 To deal with this problem, Blundell and Bond (Citation1998) proposed an estimator (henceforth SYS-GMM) that is obtained by adding the original equation in levels to the equation in differences. Instruments in differences are used for the former and instruments in levels for the latter. This helps to solve the problem of weak identification as even persistent series may be valid instruments if their lagged first differences are used. Therefore, SYS-GMM is our preferred estimator since it is found to be more efficient for data such as ours.
4. Estimation results and discussion
4.1. Results
This section contains the results of regression analysis for the two samples (EU-15, EU-13). Tables show the results obtained using LSDVC and SYS-GMM estimators; however, we concentrate on the SYS-GMM estimations as being more reliable. All regressions follow general specification (3) and, for the purpose of comparability, include a constant set of control variables and one proxy of technological innovation at the time to minimise the risk of multicollinearity.Footnote7 In order to control for the effects of financial crisis, time effects for crisis years (2008, 2009, 2010, 2011) are included in all regressions.
Table 1. Total R&D activity and economic growth.
Table 2. Private R&D activity and economic growth.
Table 3. Public R&D activity and economic growth.
Table 4. European Patent Office patent activity and economic growth.
Table 5. United States Patent and Trademark Office patent activity and economic growth.
The first set of variables is comprised of lagged GDP per capita, investment rate and openness. The estimated coefficients on lagged GDP per capita are statistically significant, have expected signs and are in line with the main strand of growth empirics. The share of investments in GDP is a significant and positive determinant of GDP growth in EU-13 countries and insignificant (although positive) in old member states. Coefficients of trade openness are significant and positive in most regressions for the EU-15 sample, and statistically insignificant in most regressions for EU-13.
As shown in Tables , the estimates demonstrate a statistically insignificant relationship between R&D expenditures and GDP per capita growth. This holds true across both samples analysed and regression techniques, even when we distinguish between business (Table ) and government-funded (Table ) flows of R&D. These results may stem from the fact that current expenditures do not capture the cumulative nature of knowledge, and that its impact on economic growth cannot thus be observed when using present investments in R&D. Therefore, we include in our analysis proxies for cumulative R&D efforts (i.e., R&D stocks). As it turned out, even when measured by accumulated R&D outlays, its impact is still insignificant using conventional levels of significance. After discriminating between privately (Table ) and publicly (Table ) funded R&D stocks, the results still lack empirical evidence for the stimulating role of cumulative R&D expenditures. The stock of publicly funded R&D was statistically insignificant in all SYS-GMM specifications in both samples analysed, and significant and negative in the case of EU-15 (LSDVC). In turn, the stock of privately funded R&D was statistically insignificant for the EU-15 sample using both estimation techniques. In the case of EU-13 countries, its impact was negative and significant using the LSDVC technique. Thus, returns on R&D expenditures seem to be questionable, particularly in the new member states.
When it comes to patent activity, according to the SYS-GMM results displayed in Tables and , current and cumulated EPO (Table ) and USPTO (Table ) patents are significant determinants of GDP per capita growth in the new member states, while the coefficients for the EU-15 are insignificant at a 10% level. The results for EU-13 are supported by the LSDVC in the case of USPTO annual flows of patents and stock of USPTO patents.
In sum, the results do not indicate the presence of a positive impact of R&D spending on per capita GDP growth. At the same time, patent activities do matter in fostering economic growth in the case of the EU-13 countries. However, effects of R&D and patents on growth are theoretically plausible, as endogenous growth models imply. Such effects have also been found in studies at the regional level.Footnote8 Therefore, our findings may seem surprising at first glance, hence they call for explanation.
4.2. Discussion
The results concerning R&D may indicate the possibly more complex relationship between R&D expenditures and economic growth. When looking at the data, one might suppose that R&D expenditures in the EU were incapable of generating technological progress because they did not reach the critical mass, as only high R&D cumulative expenditures have a positive impact on GDP per capita growth. At the theoretical level, questions may be raised as to whether there is too little or too much R&D (Alvarez-Pelaez & Groth, Citation2005).Footnote9 With respect to public R&D expenditures, they are mainly aimed at generating basic knowledge that is used in later stages by industry (the business sector) to create technological innovation. As a large part of government-funded R&D is aimed at public missions (health, the environment) that do not directly affect productivity (Guellec & Van Pottelsberghe de la Potterie, Citation2001), it is highly possible that there is no direct link between public R&D and growth. Another likely explanation of the insignificant relationship between government-funded R&D and economic growth may be inefficiency. It should be borne in mind that bureaucrats cannot get access to all decentralised information, therefore they are not able to behave like a benevolent social planner. As Romer (Citation2005) indicates, many people see the endogenous growth theory ‘as a blanket seal of approval for all of their favourite government interventions, many of which are very wrong-headed’ (p. 690). For this reason, government-funded R&D spending does not necessarily have to be effective.
The above findings are of particular importance for developing catching-up strategies of new member states and may have interesting implications concerning the European innovation policy. Are high R&D expenditures indispensable for rising productivity? The Austrian ‘growth puzzle’ shows that there are other possible ways to catch up: capital accumulation, adoption of technologies developed abroad, and reforming the industrial structure (OECD, Citation2007). Setting common targets and a simple call for increases in R&D, without taking into account country-specific characteristics, may not be sufficient as a policy guideline. Another important issue that may influence results is the heterogeneity of European countries. Comparing the old and new EU member states does not fully solve the problem. The EU-15 is comprised of countries with both low R&D intensity (e.g., Greece, Portugal, Spain and Italy) as well as economies with relatively high R&D spending (e.g., Finland, Sweden, Denmark and Germany). Moreover, heterogeneity may also reflect differences in institutions, culture and religion.
As far as patent activity is concerned, the findings are quite surprising as patents have a strong commercial orientation and therefore can be expected to promote growth independently of any analysed group of countries. A possible explanation of these results might be that owing to the relatively high costs of patenting, only the most economically valuable inventions are patented in the new member states, hence its impact on economic growth in EU-13 was positive. This may be related to the costs of applying for a patent in terms of actual fees as well as the relative market-size-per-unit application cost, and the different industrial structure of the new and old EU countries (Arundel & Kabla, Citation1998). The reason for the lack of a correlation between the number of patents awarded and economic growth in the EU-15 may be due to, inter alia, patent-trolling behaviour, where patenting is purely an anti-competitive strategy. This refers to situations where firms seek patents not to produce innovation but to extort patent fees from competitors (Boldrin & Levine, Citation2013). Our results may be of particular interest in the context of the ongoing process of introducing the European patent with unitary effect, a process that is intended to further strengthen patent protection across the EU. Such a strengthening may be an improper policy since it can provide incentives for patent trolls and enhance patent abuse via artificial litigation. As a consequence, increasing patent protection above a certain level may have no impact on economic growth. This is in line with the literature, which argues that stronger patent protection is not always growth-enhancing (e.g., Azevedo, Afonso, & Silva, Citation2014; Furukawa, Citation2007).
Furthermore, the results may also be influenced by other factors. The relatively short time frame prevents us from using averages instead of annual data. The other potential limitation of the study is that it neglects the impact of non-technological innovation on economic growth. In substance, the concept of innovation might be extended to include non-technological innovation embodied in new marketing methods or organisational changes (OECD & Eurostat, Citation2005) in order to obtain a complete picture of the innovativeness of a country. However, the lack of comparable data prevented us from undertaking such an extension.
5. Conclusion
The aim of this paper was to provide empirical evidence in relation to the role of technological innovation in the process of economic growth in a sample of EU countries. The main objective was to test whether the relationship between innovation and economic growth differs between the EU-15 and EU-13 countries. The impact of R&D investments, differentiated by the source of funding, on economic growth was also examined.
The estimated results show that the relation between innovation and growth is not as obvious as the family of endogenous growth models predicts. We found no statistically significant relationship between total R&D outlays and economic growth. This holds true across both samples analysed. The relationship remains insignificant also when R&D is differentiated by the source of funding. On the other hand, patents turned out to be significant determinants of GDP per capita growth in the new member states. Estimated coefficients were positive both for flows and stocks of USPTO and EPO patents.
All in all, our study suggests that there may be no single recipe for growth for all EU countries. In fact, the growth strategies may differ across member states and should address a country’s specific settings and development challenges. This questions the practice of setting common innovation policy objectives in the EU. Furthermore, strengthening patent protection across the EU might be an improper policy and have no impact on economic growth since it can provide incentives for patent-trolling behaviours. Consequently, our results raise questions regarding the ongoing process of extending intellectual property rights protection in the EU by introducing a unitary patent scheme. However, the estimated results should be approached with caution given the relatively short time dimension of the data set and weaknesses of our measures of innovation. A longer time frame could give more reliable estimates; therefore, our findings serve as a pilot and should be confirmed by future studies.
Disclosure statement
No potential conflict of interest was reported by the authors.
Acknowledgements
The authors thank Zbigniew Kuchta and Jan J. Sztaudynger from the University of Łódź, as well as anonymous referees for helpful comments and suggestions. All remaining errors are the authors.
Notes
1. See Aghion and Howitt (Citation2009) for a detailed discussion of endogenous growth models.
2. The number of panel studies addressing the innovation–growth nexus in the EU is limited and those studies that do address this relationship in the EU were usually conducted at the sector and regional levels.
3. Three lags were used, as a one-year lagged level of GDP per capita may be too recent to explain the convergence effect. Moreover, shorter lag lengths were not enough to wipe out autocorrelation.
4. Guellec and Van Pottelsberghe de la Potterie (Citation2001) show in sensitivity analysis that chosen depreciation rates do not change the results of the regressions significantly.
5. Both flows and stocks of R&D are expressed as a share of GDP. Data on patent activity are per million of employees. Owing to the missing data, stocks of privately and publicly funded R&D were computed using the compound growth rate of total R&D expenditures.
6. An additional important advantage of GMM estimators is that by differencing they minimalise the risk of non-stationarity of series.
7. The only exceptions are Models (8) in Tables and that were re-estimated (and denoted as (8a)) with a reduced set of control variables due to an alarming p-value of the Hansen test, which indicates a potentially excessive number of instruments. To avoid instrument proliferation, openness was excluded from the regressions.
8. See, e.g., Sterlacchini (Citation2008), Rodríguez-Pose and Crescenzi (Citation2008) and Capello and Lenzi (Citation2013).
9. The EU-13 sample is comprised mostly of countries with R&D stock below the EU-28 median in 2011. The notable exceptions are Slovenia and Estonia. On the other hand, R&D stocks in EU-15 member states are generally above the EU-28 median, except for Greece, Italy and Spain.
References
- Acemoglu, D., Aghion, P., & Zilibotti, F. (2006). Distance to frontier, selection, and economic growth. Journal of the European Economic Association, 4, 37–74. doi:10.1162/jeea.2006.4.1.37
- Aghion, P., & Howitt, P. (1992). A model of growth through creative destruction. Econometrica, 60, 323–351. doi:10.2307/2951599
- Aghion, P., & Howitt, P. (1998). Endogenous growth. Cambridge: MIT Press.
- Aghion, P., & Howitt, P. (2009). The economics of growth. Cambridge: MIT Press.
- Alvarez-Pelaez, M. J., & Groth, C. (2005). Too little or too much R&D? European Economic Review, 49, 437–456. doi:10.1016/S0014-2921(03)00045-X
- Ang, J. B., & Madsen, J. B. (2011). Can second-generation endogenous growth models explain the productivity trends and knowledge production in the Asian miracle economies? Review of Economics and Statistics, 93, 1360–1373. doi:10.1162/REST_a_00126
- Arellano, M., & Bond, S. (1991). Some tests of specification for panel data: Monte Carlo evidence and an application to employment equations. Review of Economic Studies, 58, 277–297. doi:10.2307/2297968
- Arundel, A., & Kabla, I. (1998). What percentage of innovations are patented? empirical estimates for European firms. Research Policy, 27, 127–141. doi:10.1016/S0048-7333(98)00033-X
- Azevedo, M. L., Afonso, Ó., & Silva, S. T. (2014). Endogenous growth and intellectual property rights: A north–south modeling proposal. Economic Modelling, 38, 112–120. doi:10.1016/j.econmod.2013.12.021
- Barcenilla-Visús, S., Gómez-Sancho, J. M., López-Pueyo, C., Mancebón, M. J., & Sanaú, J. (2013). Technical change, efficiency change and institutions: Empirical evidence for a sample of OECD countries. Economic Record, 89, 207–227. doi:10.1111/1475-4932.12019
- Bloom, N., Draca, M., & Van Reenen, J. (2016). Trade induced technical change? The impact of Chinese imports on innovation, IT and productivity. The Review of Economic Studies, 83, 87–117. doi:10.1093/restud/rdv039
- Blundell, R., & Bond, S. (1998). Initial conditions and moment restrictions in dynamic panel data models. Journal of Econometrics, 87, 115–143. doi:10.1016/S0304-4076(98)00009-8
- Boldrin, M., & Levine, D. K. (2013). The case against patents. Journal of Economic Perspectives, 27, 3–22. doi:10.1257/jep.27.1.3
- Bruno, G. S. F. (2005). Estimation and inference in dynamic unbalanced panel-data models with a small number of individuals. The Stata Journal, 5, 473–500. Retrieved from http://www.stata-journal.com/article.html?article=st0091
- Capello, R., & Lenzi, C. (2013). Territorial patterns of innovation and economic growth in European regions. Growth and Change, 44, 195–227. doi:10.1111/grow.12009
- CEC. (2000). Presidency conclusions of the Lisbon European council. Brussels: Commission of the European Communities: March 23/24.
- Chu, A. C., Cozzi, G., & Galli, S. (2014). Stage-dependent intellectual property rights. Journal of Development Economics, 106, 239–249. doi:10.1016/j.jdeveco.2013.10.005
- Coe, D. T., & Helpman, E. (1995). International R&D spillovers. European Economic Review, 39, 859–887. doi:10.1016/0014-2921(94)00100-E
- Damijan, J. P., Kostevc, Č., & Polanec, S. (2010). From innovation to exporting or vice versa? The World Economy, 33, 374–398. doi:10.1111/j.1467-9701.2010.01260.x
- Durlauf, S. N., Johnson, P. A., & Temple, J. R. W. (2005). Growth econometrics. In P. Aghion, & S. N. Durlauf (Eds.), Handbook of economic growth, Vol. 1A (pp. 555–677). New York, NY: Elsevier. doi:10.1016/S1574-0684(05)01008-7
- Eaton, J., & Kortum, S. (2001). Trade in capital goods. European Economic Review, 45, 1195–1235. doi:10.1016/S0014-2921(00)00103-3
- Engelbrecht, H.-J. (1997). International R&D spillovers, human capital and productivity in OECD economies: An empirical investigation. European Economic Review, 41, 1479–1488. doi:10.1016/S0014-2921(96)00046-3
- Feenstra, R. C., Inklaar, R., & Timmer, M. P. (2015). The next generation of the Penn World Table. American Economic Review, 105, 3150–3182. Retrieved from www.ggdc.net/pwt doi: 10.15141/S5159X
- Furukawa, Y. (2007). The protection of intellectual property rights and endogenous growth: Is stronger always better? Journal of Economic Dynamics and Control, 31, 3644–3670. doi:10.1016/j.jedc.2007.01.011
- Goel, R. K., & Ram, R. (1994). Research and development expenditures and economic growth: A cross-country study. Economic Development and Cultural Change, 42, 403–411. Retrieved from http://www.jstor.org/stable/1154449. 10.1086/452087
- Griffith, R., Redding, S., & Van Reenen, J. (2004). Mapping the two faces of R&D: Productivity growth in a panel of OECD industries. Review of Economics and Statistics, 86, 883–895. doi:10.1162/0034653043125194
- Grossman, G. M., & Helpman, E. (1991). Quality ladders in the theory of growth. The Review of Economic Studies, 58, 43–61. doi:10.2307/2298044
- Guellec, D., & Van Pottelsberghe de la Potterie, B. (2001). R&D and productivity growth: Panel data analysis of 16 OECD countries. OECD Economic Studies, 2001, 103–126. doi:10.1787/eco_studies-v2001-art12-en
- Guellec, D., & Van Pottelsberghe de la Potterie, B. (2004). From R&D to productivity growth: Do the institutional settings and the source of funds of R&D matter? Oxford Bulletin of Economics and Statistics, 66, 353–378. doi:10.1111/j.1468-0084.2004.00083.x
- Howitt, P. (1999). Steady endogenous growth with population and R&D inputs growing. Journal of Political Economy, 107, 715–730. doi:10.1086/250076
- Jones, C. I. (1995). R&D-based models of economic growth. Journal of Political Economy, 103, 759–784. Retrieved from http://www.jstor.org/stable/213858110.1086/262002
- Jones, C. I. (2002). Sources of U.S. economic growth in a world of ideas. American Economic Review, 92, 220–239. doi:10.1257/000282802760015685
- Kortum, S. (1997). Research, patenting, and technological change. Econometrica, 65, 1389–1419. doi:10.2307/2171741
- Levine, R., & Renelt, D. (1992). A sensitivity analysis of cross-country growth regressions. American Economic Review, 82, 942–963. Retrieved from http://www.jstor.org/stable/2117352
- Lichtenberg, F. R. (1992). R&D investment and international productivity differences (Working Paper No. 4161). Cambridge, MA: NBER. doi:10.3386/w4161
- Liu, X., & Buck, T. (2007). Innovation performance and channels for international technology spillovers: Evidence from Chinese high-tech industries. Research Policy, 36, 355–366. doi:10.1016/j.respol.2006.12.003
- Lucas, R. E. (1988). On the mechanics of economic development. Journal of Monetary Economics, 22, 3–42. doi:10.1016/0304-3932(88)90168-7
- Madsen, J. B. (2008). Economic growth, TFP convergence and the world export of ideas: A century of evidence. The Scandinavian Journal of Economics, 110, 145–167. doi:10.1111/j.1467-9442.2008.00530.x
- Madsen, J. B. (2010). The anatomy of growth in the OECD since 1870. Journal of Monetary Economics, 57, 753–767. doi:10.1016/j.jmoneco.2010.05.014
- Nickell, S. (1981). Biases in dynamic models with fixed effects. Econometrica, 49, 1417–1426. Retrieved from http://www.jstor.org/stable/191140810.2307/1911408
- OECD. (2007). OECD economic surveys: Austria 2007. Paris: OECD Publishing.
- OECD & Eurostat. (2005). Guidelines for collecting and interpreting innovation data - The Oslo Manual (3rd ed.). Paris: OECD.
- Park, W. G. (1995). International R&D spillovers and OECD economic growth. Economic Inquiry, 33, 571–591. doi:10.1111/j.1465-7295.1995.tb01882.x
- Peretto, P. (1998). Technological change and population growth. Journal of Economic Growth, 3, 283–311. doi:10.1023/A:1009799405456
- Porter, M. E., & Stern, S. (2000). Measuring the “ideas” production function: Evidence from international patent output (Working Paper No. 7891): Cambridge, MA: NBER. doi:10.3386/w7891.10.3386/w7891
- Rodríguez-Pose, A., & Crescenzi, R. (2008). Research and development, spillovers, innovation systems, and the genesis of regional growth in Europe. Regional Studies, 42, 51–67. doi:10.1080/00343400701654186
- Romer, P. M. (1986). Increasing returns and long-run growth. Journal of Political Economy, 94, 1002–1037 . Retrieved from http://www.jstor.org/stable/183319010.1086/261420
- Romer, P. M. (1990). Endogenous technological change. Journal of Political Economy, 98, S71–S102. doi:10.1086/261725
- Romer, P. M. (2005). Interview with Paul M. Romer. In B. Snowdon & H. R. Vane (Eds.), Modern macroeconomics. Its origins, development and current state (pp. 673–694). Cheltenham: Edward Elgar.
- Segerstrom, P. S. (1998). Endogenous growth without scale effects. American Economic Review, 88, 1290–1310. Retrieved from http://www.jstor.org/stable/116872
- Sterlacchini, A. (2008). R&D, higher education and regional growth: Uneven linkages among European regions. Research Policy, 37, 1096–1107. doi:10.1016/j.respol.2008.04.009
- Ulku, H. (2007). R&D, innovation and output: Evidence from OECD and nonOECD countries. Applied Economics, 39, 291–307. doi:10.1080/00036840500439002