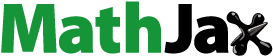
Abstract
Non-life insurance prices may fluctuate due to economic and/or institutional factors; occasionally, the changes are cyclical. While the majority of previous studies relating to insurance price dynamics adopt data from developed economies, this paper uses data from China to provide new evidence. This study tests the long-term and short-term effects of real gross domestic product (GDP), interest rate and rate of stock market return on the prices of different lines of non-life insurance, i.e., property-liability insurance and personal accident insurance. The results indicate that the price dynamics of property-liability insurance are generally similar to those of developed countries, except for the effect of GDP, while price determination of personal accident insurance seems to be affected by a wider range of economic and institutional variables and has its own features. The price dynamics of non-life insurance in China have been identified as being connected to the country-specific economic and institutional environments.
1. Introduction
The dynamics of insurance price (measured as underwriting profits or loss ratio; see Harrington and Niehaus (Citation2000) for a review) in property-liability insurance markets are traditionally observed as the dynamic shifting back and forth of insurance price between ‘hard’ and ‘soft’ markets. The soft market is characterised by readily available insurance coverage and lower insurance prices, while hard markets are characterised by difficulty in obtaining restrictive insurance coverage and higher insurance prices. In practice, a strong form of such a phenomenon is known as the underwriting cycle. Underwriting cycles are widely observed in the non-life insurance industry and are not easy to eliminate from insurance operations. By modelling and predicting such fluctuations, insurance companies might be able to control the operating volatility and, thus, lower the capital costs.
Many theories try to explain the dynamics of insurance price, but no single theory seems capable of explaining all of its aspects. The first explanation is referred to as the rational expectation framework, whereby the insurance price is assumed to only reflect the discounted cash flow of future costs, and expectations are made using all relevant information; thus, insurance prices are the best predictors of future losses and expenses. Accordingly, insurance price is a decreasing function that depends only on the interest rate in both the short- and long-term. Many empirical studies indicate the short-run relationship between insurance price and interest rate (e.g., Chen, Wong, & Lee, Citation1999) and fewer studies focus on the long-term determination (e.g., Grace & Hotchkiss, Citation1995). According to this theory, a price cycle occurs only if insurance companies’ expected costs are cyclical in a perfect insurance market. Cummins and Outreville (Citation1987) provide a more compelling explanation, the rational expectations/institutional intervention hypothesis, for this theory. Based on the U.S. insurance market, they attribute a cyclical pattern to a second-order autoregressive process, which is caused by a filtration of the rational insurance price through institutional lags of the insurance industry; thus, rational acts of insurance companies may appear irrational. They also suggest that the cycle, as observed in the U.S. market, may also be present in other countries through the proliferation of international re-insurance services. Their insurance price dynamics model, which is based on a second-order autoregressive process, has been the standard form for the past two decades.
Another famous school of thought about the fluctuation of insurance prices is based on the irrational behaviour of insurance companies. Winter (Citation1994) proposes a well-known capacity constraint hypothesis, which documents that the imperfect insurance market prevents insurance companies from quickly adjusting their capacity to maintain a long-term equilibrium. Because the cost of external equity is more than internal equity, insurance companies are willing to drive up the insurance price rather than issue external capital to increase capacity when they experience unexpected negative capital shock, such as an unexpected catastrophe claim. Therefore, insurance prices not only depend on interest rates, but also on present and past values of capacity. Doherty and Garven (Citation1995) also note that not only a loss shock, but also an interest rate shock, would affect the assets and liabilities of insurance companies, as well as their capital. The capacity constraint hypothesis can be tested by examining whether capacity is negatively related to insurance price.
The third school of thought is devoted to the correlation between the insurance price and the broad condition of the economy. And they attempt to explain cyclical behaviour through its relationship with cyclical economic variables. Cyclicality is not unique to the insurance industry. Many industries have had upturns and downturns in prices and profits accompanied by variations in commodity quality and supply. Economists have referred to the fluctuations in the overall business activity as the ‘business cycle’ for more than a century. In addition to being a financial asset, an insurance policy may be viewed as a commodity that is related to its insured property. Overall demand for insurance is also expected to vary with economy growth, with expansion and contraction periods corresponding to demand for insurance. Moreover, profitability for insurance companies, which is also a factor in insurance price making, is linked to investment income, as well as the cost of capital, which must be linked to the wider economy. Within this school, several studies use cointegration analysis to attempt to discover the long-term determination (e.g., Grace & Hotchkiss, Citation1995; Lazar & Denuit, Citation2012), while many studies focus on the short-term relationship between insurance price and relevant macroeconomic variables (e.g., Chen et al. Citation1999; Lamm-Tennant & Weiss, Citation1997) or introduce macroeconomic variables into a second-order autoregressive process (e.g., Meier, Citation2006).
Over the past two decades, a considerable number of empirical studies have been performed regarding the dynamics of insurance price based on the above theories. However, many researchers find that the long-run relationship is relatively difficult to observe when addressing insurance price or profit, primarily because the insurance price might be demonstrated to be a stationary process, while the relevant regressors, such as interest rate, are treated as non-stationary processes. Cointegration is constrained under Johansen’s vector error correction model (VECM) framework, which requires that the underlying variables be integrated of order one. For example, Choi, Hardigree, and Thistle (Citation2002) report that the ratio of discounted losses to premiums net of expenses economic loss ratio (ELR) was I(0), but the interest rate and capacity proxy series were I(1). They conclude that underwriting profits are cointegrated with the interest rate simply because the ELR series reveals I(0); however, the long-run relationship between underwriting profits and capacity proxy is not testable in such circumstances. Harrington and Yu (Citation2003) pay more attention to the time series characteristics of insurance price by using a battery of tests for unit roots under the assumption of a deterministic trend. They indicate that insurance prices are stationary both at the entire industry level and within individual insurance lines. They argue that inclusion of any non-stationary regressors will make both least squares regressions and cointegration analysis inappropriate. Therefore, cointegration analysis is neither relevant nor necessary after controlling for deterministic influences. In contrast, Haley (Citation2007) notes that finding a unit root is a sufficient although unnecessary condition. He also argues that controlling for a time trend when addressing insurance price may not be appropriate. Without the necessity for testing unit roots, Jiang and Nieh (Citation2012) propose a more robust empirical methodology and provide further insight into this context by simultaneously assessing the long-term and short-term effects. They provide evidence of the long-term relationship between interest rate, capacity and underwriting profits in the U.S. market during the last half century. Furthermore, Boyer, Jacquier, and Norden (Citation2012) utilise the time series technique of the business cycles and indicate that any evidence of underwriting cycles could simply be spurious. Regardless of whether the cycles exist, there is no way to predict this cycle to obtain profits. In fact, as Weiss (Citation2007) indicated, there are many more mysteries about underwriting cycles that deserve attention, both theoretically and empirically.
Pioneering studies on insurance price fluctuation or underwriting cycles have been widely performed with data from developed economies, focusing in particular on the U.S. and some European countries. To the best of our knowledge, similar studies have rarely been conducted for developing markets or transitional economies. China is well known as a transitional and mixed economy in which the state-owned sector previously dominated the private sector and remains influential in the whole economy at present, which is very different from the typical market economies. The proportion of the state-owned sector has been observed to decline during the process of transition from a highly planned economy to a market economy and more changes are expected to take place in the future. The Chinese insurance industry has been dominated by state-owned enterprises since the first state-owned insurance companies, which were the only insurance companies at that time; the People’s Insurance Company of China (PICC), set up in 1979, is just a miniature of the whole economy of China. The Chinese insurance industry has gone through major changes along with the economic variables during the past three decades. In Chinese insurance markets, the private sector, including foreign insurance companies, has been gradually encouraged to compete with the state-owned firms and has been observed to be gaining a larger market share. In addition, the regulations have also been reformed in accordance with this trend, for instance, the rates for the majority of insurance products are determined by the market rather than being strictly regulated as in the past. Given this unique background, the relationship between macroeconomic variables and the insurance price may assume a different form compared to the cases of mature market economies appearing in the literature. This article explores external impacts on insurance price determination in the Chinese non-life insurance market and attempts to bridge the gap in understanding the relationship between insurance price and external economic factors.
The importance of this research is also derived from the fact that the Chinese insurance industry has begun to play an important role in the global insurance market. The Chinese insurance industry has grown rapidly in the past three decades and has become one of the largest markets in the world. In 2014, the Chinese insurance market was ranked third in the world insurance market in total premium. However, the insurance density and penetration rates are much lower than the developed countries, indicating that the Chinese insurance market is still growing. Our study contributes to the literature by providing more evidence on the dynamics of insurance price and helping to deepen the understanding of the Chinese non-life insurance market, thus providing insights for both researchers and insurance firms.
Compared to the existing literature, the present work is also innovative because the external impacts on insurance price are studied in an autoregressive distributed lag (ARDL) cointegration framework. The advantages of utilising such a methodology in this context are fourfold. First, the arguments about stationary features of insurance price (e.g., Haley, Citation2007; Harrington & Yu, Citation2003) are avoided, as there is no need to examine whether variables possess unit roots in an ARDL cointegration framework. Second, the data generating process (DGP) of this methodology is based on an autoregressive process, which is typically constructed to portray the dynamics of insurance price (Cummins & Outreville, Citation1987; Higgins & Thistle, Citation2000; Winter, Citation1994) and, thus, is appropriate in this context. Third, the ARDL cointegration framework is valid for using small samples to test and estimate the cointegration relationship (35–40 observations are still valid) (see Shahbaz, Lean, & Kalim, Citation2013). The small sample properties of the ARDL approach are superior to those of Johansen’s technique (Pesaran & Shin, Citation1999) and are more favourable in our case, because the number of observations in our study is rather small (~ 50). Finally, for the error-correction representation of the corresponding ARDL model, uneven lag orders and contemporaneous innovations are permitted, and only a unique error-correction term will be present, which avoids confusion from having multiple cointegration vectors. Findings under this framework indicate that the price dynamics of property-liability insurance in China are generally similar to those of developed economies, while the price of personal accident insurance is significantly affected by some country-specific economic and institutional factors, thus providing new evidence to the existing literature.
This paper is organised as follows: Section 1 explains the external variables that could have an effect on insurance price; Section 2 describes the data and methodology employed; Section 3 gives the empirical results; Section 4 presents conclusions.
2. External effects on the insurance price
As discussed above, the theories of the insurance cycle are not inclusive, and the external factors that affect the insurance price are not clearly known. According to the previous studies related to insurance price dynamics, several economic variables may have an impact on insurance price in the short- or long-term.
2.1. Gross domestic product (GDP)
Non-life insurance activities may be linked to the general economic performance of the national economy and may be related to changes in real GDP. The reason to include income variables is not only because of the wealth and income effect on attitudes toward risk, but also the economic growth effect, which creates more insurable risk as a result of the increase in goods, such as houses and automobiles, and affects the demand for insurance. Price is determined by demand and supply; thus, factors affecting the demand side or supply side can have impacts on price determination. Doherty and Kang (Citation1988) develop a structure model including both the demand side and supply side and describe underwriting cycles as a market clearing process with partial adjustment. The supply function is specified with expected excess underwriting profits, and the competitive underwriting profits are modelled by the insurance capital asset pricing model (CAPM) (Doherty & Garven, Citation1986; Fairley, Citation1979), which depends on the risk-free interest rate and capital market return. The demand function is mainly conditioned by aggregate income measured by GDP. Meier (Citation2006) extends Cummins/Outreville’s second-order autoregressive process by inclusion of the general economic variables and reformulates a cointegration analysis by re-parameter procedures. Three developed countries are examined. GDP serves as an indicator for potential losses and mainly influences the demand side of the model. The results indicate that, compared with the U.S. and Switzerland, the Japanese insurance market reveals quite different features for both GDP and interest rate implications. Lamm-Tennant and Weiss (Citation1997) use a generalised least square regression model to analyse the changes in premiums with respect to the changes in lagged losses, interest rates, average stock prices and real gross domestic products of nine developed countries. Many countries follow the theoretical prediction that the changes in interest rates and changes in average stock prices have a negative impact on insurance premiums; however, some countries reveal neutral, even positive, impacts on insurance premiums, including Germany, Japan, Spain, Austria and Switzerland, which is not consistent with the results for the U.S. market. The changes in GDP have neutral or even negative impacts on insurance premiums, as in the cases of Italy, Japan and Switzerland. Chen et al. (Citation1999) focus on Asian countries for the first time and report that the changes in GDP have no impact on insurance premiums in Japan and Taiwan. Because a significant relationship between the premium and real gross domestic product is identified after accounting for the claim paid in Lamm-Tennant and Weiss (Citation1997) and Chen et al. (Citation1999), it is reasonable to assume that GDP is related to insurance price according to our definition of insurance price.
2.2. Interest rate
According to the rational expectation theory, insurance price reflects the discounted cash flow of future costs; thus, insurance price is a decreasing function that depends only on the discount rate in both the short- and long-term. Practically, the interest rate is usually referred to as the discount rate. As mentioned above, interest rate is found to have significant effects on insurance price in some countries in Meier (Citation2006). Similar results are indicated in Lamm-Tennant and Weiss (Citation1997) and Chen et al. (Citation1999) when the dependent variable is insurance premium. Grace and Hotchkiss (Citation1995) applied a cointegration technique to examine the relationship between the insurance price and the general condition of the economy; a bundle of economic variables, including interest rate, are included.
2.3. Rate of market return
Rate of market return reflects how much return can be paid for unit capital invested in the market. Insurance activities are involved with investment in two ways. First, underwriting capacity comes from the investment of equity holders who will ask for a return that is no less than the general market return elsewhere. So, when the outside market return rises, insurance price might also go up to meet the requirement of equity holders. Second, insurance companies usually invest the reserves of the premiums in the markets to earn profit, especially for the case of life insurance. When the rate of market return rises, insurance companies will benefit from the investment and the pressure to increase the price to make more money will be eased, thus helping to lower the price. So, how the rate of market return will affect insurance price when adding these two impacts up is uncertain. Average stock prices are included in Lamm-Tennant and Weiss (Citation1997) and Chen et al. (Citation1999). Jawadi, Bruneau, and Sghaier (Citation2009) report the cointegration relationship between the nominal insurance premium received and financial markets (i.e., interest rates and stock market returns) for five developed countries. They conclude that the adjustment of the insurance premium toward equilibrium in France, Japan and the U.S. is rather discontinuous, asymmetrical and non-linear.
There are still some other variables, such as consumer price index (CPI), surplus of insurance companies and market concentration ratio, that could have a short- or long-term relationship with insurance price; even the cost of distribution channels can have a significant impact on insurance price (Banyár & Regős, Citation2012), but, currently, we focus on real GDP, interest rate and rate of market return in this study due to constraints in data availability. The relationship between insurance price and interest rate (r), real GDP and rate of market return (Rm) is examined in an ARDL cointegration framework.
3. Data and methodology
3.1. Data
This article uses quarterly data for the period 2001Q1–2013Q2 to study the dynamics of insurance price under the influence of external effects of economic variables. The definition of unit insurance price by Cummins and Outreville (Citation1987), the ratio of premiums to loss (or inverse of loss ratio), is used to indicate the insurance price. This measure reflects the loading or transaction costs of insurance, which measures the aggregate economic value of insurance. Much of the underwriting cycle literature adopts the same definition of unit price. To reach a deeper understanding of the different lines of the non-life insurance industry, two lines, i.e., property-liability insurance and personal accident insurance, are examined. The data for written premiums and claims paid for property-liability insurance and personal accident insurance are taken from the website of the China Insurance Regulatory Committee (CIRC); we transform the written premiums into premiums earned to compute the loss ratio. The rate of the 3-month Treasury bill is used to represent the level of the interest rate (r) in China; the data for the interest rate are adopted from the database of the China Stock Market & Accounting Research (CSMAR) Solution. The data for GDP are obtained from the China Economic Information Network (CEIN) and are adjusted with CPI based on 2000Q1 to obtain real quarterly GDP Stock market returns are indicated with the rate of return of the Shanghai Composite Index; the data are also taken from the database of CSMAR Solution.
Table presents relevant descriptive statistics. As indicated in Table , the inconsistency in the integration order of variables in this study encourages the use of the ARDL bounds approach rather than one of the alternative cointegration tests.
Table 1. Descriptive statistics.
3.2. Methodology
To examine the relationship between unit price of insurance and macroeconomic determinants, we employ the ARDL cointegration model (Pesaran, Shin, & Smith, Citation2001) as a framework. Let yt represent the proxy of unit insurance price of the industry at current time t. rt, Rmt and GDPt denote the interest rate, the market return and real gross domestic products, respectively, and define as a 3 × 1 vector of variables. Consider that the data-generating process for unit insurance price, the interest rate, the market return and real gross domestic products is an unrestricted VECM as follows:
(1)
(1)
where the partition is a 4 × 1 vector of variables. Similarly, deterministic term
and error term
are 4 × 1 vectors. The long-term multiplier,
, is a matrix of order 4 × 4, and
is the short-run dynamic coefficient matrices. A critical assumption is that, if vector
, it ensures that there is at most one long-term relationship between the unit insurance price and the determinants, irrespective of the order of integration. There is no feedback from the level of yt and the interest rate and the market return and real gross domestic products could be regarded as long-term forcing variables. Such an assumption is intuitively reasonable because the underwriting activity of the insurance industry has only a modest impact on the macroeconomic system. Equation Equation(1)
(1)
(1) can then be written in terms of the dependent variable yt and the forcing variables
as (Mills, Citation2008):
(2)
(2)
(3)
(3)
Additionally, define the variance matrix of error term as:(4)
(4)
vyt can be expressed conditionally in terms of as:
(5)
(5)
where , ɛt is normally distributed with zero mean and is independent of vyt. A conditional modelling of the unit insurance price, the scalar variable yt, can be constructed by substituting equations (Equation3
(3)
(3) ) and (Equation5
(5)
(5) ) into equation (2), which yields a conditional error correction model (CECM) as:
(6)
(6)
where
It follows from equation Equation(6)(6)
(6) that, if Ayy ≠ 0 and
, there exists a long-run relationship between unit insurance price and the determinants, given by:
(7)
(7)
where is the long-run response parameters and ut is a zero mean stationary process. A CECM can be represented as:
(8)
(8)
where the error correction component, , is the current adjustment due to the deviation from equilibrium at the last period. The absolute value of
can be viewed as the speed back to equilibrium, and, if
, this long-run relationship is stable. The existence of a unique valid long-term relationship among variables and, hence, a sole error-correction term,
, is the basis for estimation and inference. A short-term relationship cannot be supported unless a unique and stable equilibrium relationship holds in a significant statistical sense.
According to Pesaran et al. (Citation2001), the CECM represented in equation (Equation6(6)
(6) ) is used as the basis of the long-run relationship testing procedure. This approach, which separates the long-term (level) relationship and short-term dynamics, could be applied to test the long-term relationship between the variables, irrespective of the order of the underlying variables (I(0) or I(1)), even fractionally integrated (Cavanagh, Elliott, & Stock, Citation1995; Pesaran et al., Citation2001). Such an outstanding characteristic is suitable for studying the underwriting activity in the insurance industry, because the insurance price is usually assumed to be stationary and, thus, not utilised by traditional cointegration analysis. Unlike other cointegration techniques (e.g., Johansen’s procedure), which require certain pre-testing for unit roots as well as underlying variables to be integrated of order one, this CECM provides an alternative test for examining long-term relationships. The unit root testing of variables (e.g., Grace & Hotchkiss, Citation1995) is no longer necessary. Such an important feature of this test reduces the degree of uncertainty arising from the pre-testing stage of each series in the analysis of level relations, which is an important issue in our case. As mentioned, there is much debate as to whether insurance prices are stationary or not (Haley, Citation2007; Harrington & Yu, Citation2003). The bounds testing procedure provided by Pesaran et al. (Citation2001), therefore, could avoid such an argument. Notice that equation (Equation6
(6)
(6) ) can be differentiated between five cases of interest delineated according to how the deterministic components are specified. To make our arguments more convincible, this paper will test all five cases, i.e., no constant, restricted constant, unrestricted constant, restricted constant and trend, and unrestricted constant and trend, to fit the most suitable case into various insurance lines separately.
Once the long-term relationship is determined by the bounds testing procedure, Pesaran et al. (Citation2001) suggest that the augmented ARDL can be estimated. The autoregressive distributed lag model can be rearranged as a CECM and is capable of differentiating lag lengths on the lagged variables in equation Equation(6)(6)
(6) without affecting the asymptotic results of bounds test. According to Mohd Daud, Halim Ahmad, and Azman-Saini (Citation2013), a lag order of 4 could be chosen as the maximum order of the lags in the ARDL model if observations are on a quarterly basis. Therefore, we chose n = 4 in equation Equation(6)
(6)
(6) and search across (n + 1)4 autoregressive distributed lag models via Akaike Information Criterion (AIC). Allowing for differential lag lengths on the lagged variables is more general than other types of CECM of partial systems carried out by Boswijk (Citation1994, 1995).
4. Empirical results
4.1. Bounds testing
As mentioned in the previous section, a critical assumption that has to be tested is whether there is at most one long-term relationship among variables. Irrespective of whether variables are I(0) or I(1), a bounds testing procedure is provided to test null hypotheses of long-term relationships. This study imposes the order of lag length (n) from 1 and calculates the F-statistic and t-statistic. Note that the asymptotic distributions of the F-statistic and t-statistic are non-standard, irrespective of whether the variables are I(0) or I(1). Because the asymptotic distributions of these two statistics are non-standard, Pesaran et al. (Citation2001) provide a bounds testing procedure that has two sets of asymptotic critical values. One set assumes all variables are I(0), and the other assumes that all variables are I(1). If the computed F-statistic and t-statistic fall above the upper limit of the bound critical value, the null hypothesis is rejected, which means the variables are cointegrated. Conversely, if the computed F-statistic and t-statistic fall below the lower bound critical value, the variables are concluded to be not cointegrated and the null hypothesis cannot be rejected. Finally, the case within the band would be inconclusive.
Table indicates that, for the property-liability insurance, the null hypothesis maintaining non-existence of the long-term relationship is rejected for models with intercept and no trend, and with unrestricted intercept and trend. Under the parsimony principle, we chose the model with intercept and no trend for the property-liability insurance. Such a result is consistent with Jiang and Nieh (Citation2012), in which the model with intercept and no trend has been chosen for the U.S. insurance market. As for the personal accident insurance, the null hypothesis is rejected for models with no intercept and no trend. Therefore, we chose the model with no intercept and no trend for the personal accident insurance. To some extent, the feature of no constant reveals the fact about arbitrary pricing decisions of insurance companies who engage in the business of the personal accident insurance. The reason why the trends are not included in this study is not exactly known; one possible explanation is that our sample period is rather short to reflect the trend. The results for all five cases are listed in Table .
Table 2. Bounds testing statistics.
4.2 Estimation
Given the maximum order of lag (n = 4) by the bounds test, one of 125 (=(1+4)3) ARDL models must be selected using the Akaike Information Criterion (AIC) during the second stage. Table then lists the diagnostic statistics used in ARDL estimation. The adjusted R2s for two models are 0.59 and 0.66, respectively. The computed F-statistics clearly reject the null hypothesis that all regressors have zero coefficients, suggesting that the ARDL model fits the data reasonably well. Diagnostic testing is statistically insignificant for all ARDL models, suggesting no misspecification.
Table 3. Diagnostic statistics of ARDL estimations.
Long-term effects estimation in Table indicates that the price of property-liability insurance has a constant of 2.0956, but the price of personal accident insurance does not have one. This might indicate that the price of property-liability insurance is at some certain level in general, while the price of personal accident insurance is quite flexible. In China, automobile insurance accounts for more than two-thirds of insurance premiums in the non-life insurance industry, the price of automobile insurance has been nearly the same across different insurance firms in the past years, and this phenomenon may help to explain the existence of the price constant. In contrast to property-liability insurance, personal accident insurance has undergone a large price adjustment in the past decade. In China, the pricing philosophy of personal accident insurance is more arbitrary based, resulting in more volatile loss ratios of this line of business. Aviation personal accident insurance, for instance, was sold for RMB 20 for an insurance amount of RMB 200,000 before January 2003, but, after that, the insurance amount increased to RMB 400,000, with the price of the policy unchanged. Moreover, when insurance firms discovered that the real cost of personal accident insurance is very low, an increasing number of new personal accident insurance products were designed with a lower price and/or wider coverage. Thus, during the sample period, it is not surprising to see no price constant.
Table 4. Estimated long-term effects of ARDL model.
It is illustrated in Table that real GDP has a significant positive long-term relationship with the price of property-liability insurance, which means that the demand side of the insurance market has a positive effect on insurance price in the long-run. Real GDP also exhibits a positive long-term relationship with the price of personal accident insurance, but the result is not significant. Our findings for property-liability insurance are different from Lamm-Tennant and Weiss (Citation1997), which did not find a significant relationship between real GDP and premiums, but, similar to Chen et al. (Citation1999), in which a significant relationship between the changes in the real GDP and changes in the premiums of all five sample Asian countries (i.e., Japan, Malaysia, Singapore, South Korea, and Taiwan) was identified. The positive effect of GDP growth on insurance price can be attributed to the enlarging demand and capacity constraint on the supply of insurance in developing countries such as China. During the growth process in developing countries, the development of the service industry always lags behind the manufacturing industry, as most capital is allocated to the manufacturing sector in priority, and a scarcity of underwriting capital in the insurance industry is more often the case than not; thus, the capacity constraint increases the price and hard market forms as indicated by Winter (Citation1994). Personal accident insurance follows a special growth path, as discussed above, but we speculate that the intense competition among the insurers eases the effect of the demand side, resulting in an insignificant positive effect. Dragos (Citation2014) also found a similar result that the GDP per capita has a non-significantly positive influence on the demand for non-life insurance in the Asian emerging countries. She argues that the attitude of risk neutrality induced by the planned Chinese economy generates this non-significance of income level over the non-life insurance demand. The accident losses are covered by family financial aid, donation from the community and government assistance, consequently, lowers the level of risk aversion for the personal accident insurance.
As can be seen from Table , the price of property-liability insurance is negatively correlated with interest rate and rate of market return at the 5% and 10% significance levels, respectively. Generally speaking, profit from non-life insurance operation is mainly from two channels: One is underwriting profit, which is the surplus left in premiums after paying for the claim and the cost of operation and the other is investment profit, which is earned by investing the reserve and surplus. When the revenue from investment is considerable, insurance firms may lower the expectation on underwriting profit, which means that insurance firms can underwrite some relatively bad risk that they would not accept otherwise or underwrite standard risk with a lower price. According to our definition of insurance price, insurance price reflects the margin that insurance firms can obtain from selling the policies. Thus, insurance price has a negative relation with investment profitability, a proxy for rate of market return. Premiums are usually thought to be the discounted present value of future costs; thus, it is not surprising to see that the interest rate, a proxy for discount rate, is negatively related with insurance price. Our findings for the property-liability line are consistent with many previous studies (Doherty & Garven, Citation1995; Doherty & Kang, Citation1988; Fields & Venezian, Citation1989; Haley, Citation1993; Lamm-Tennant & Weiss, Citation1997; Smith, Citation1989). In contrast to property-liability insurance, the price of personal accident insurance is identified as being positively correlated with rate of market return and interest rate in Table . This finding is contrary to general expectations, but not rare in the previous literature. Stock index is found to be positively correlated with premiums among four out of five sample Asian countries, and interest rate has a positive effect on premium among three out of five sample Asian countries in Chen et al. (Citation1999). Lamm-Tennant and Weiss (Citation1997) also identified similar results for Japan, indicating that the stock index has a positive relationship with premiums, but an adequate explanation for this result was not given. For the case of China, because the price of personal accident insurance has experienced radical changes during the past decade and the trend in price has been downward, we believe this change is not the result of any single factor, but due to the whole economic environment during the process of marketization. Under such conditions, the price of personal accident insurance decreases along with less price regulation and intense competition among insurance firms. At the same time, interest rate levels have been descending since financial market reform. The rate of market return shares the same feature as interest rate and correlates with personal accident insurance price positively.
In our short-term analysis framework, the coefficient of error correction mode (ECM) can be viewed as the price adjustment speed of different lines of insurance. The results from Table indicate that personal accident insurance has a higher price adjustment speed than property-liability insurance. This means that, when a price shock is sustained by both lines of insurance, causing prices to deviate from the equilibrium level, it will take less time for personal accident insurance than property-liability insurance to return to the former price trend, indicating a shorter cycle. Actually, such results can be well justified with the practice of insurance. Although the duration of many property insurance policies is 1 year, some forms of property insurance, such as construction project insurance, have a more than 1-year term and it is hard to change the price once those contracts are signed. Moreover, even for a 1-year policy, such as environmental pollution liability insurance and medical liability insurance, the settlement of claim may last for several years. The long-tail property of claim settlement may also have a sticky effect on the insurance price. For instance, when insurance companies detect higher risk on pollution liability insurance, they may raise the premiums and, for the sake of safety, the premiums will not go down until the claim settlement provides evidence that the risk is lowered; this may take quite some time. In contrast to property-liability insurance, the duration of personal accident insurance policies is generally short and, moreover, the settlement of claim will generally not last for more than 1 year. Thus, our test in the ARDL cointegration framework provides substantial evidence for the difference in price dynamics for different lines of non-life insurance.
Table 5. Error correction representation of ARDL model.
The error correction model also provides some evidence on the short-term effect of external factors on different lines of insurance (as indicated in Table ). Changes in the rate of market return are identified as being negatively correlated with changes in property-liability insurance price and personal accident insurance price (with one period lag) in the short-term. This might indicate that, although many lines of non-life insurance have short-duration policies, the investment of insurance funds still matters for insurance price setting in the short-run. Changes in interest rate are found to positively affect the changes in price of personal accident insurance. This finding is not in accordance with rational expectation theory, and we speculate that the interest rate is not just a discount operator, as in the rational expectations framework, but is an indicator of the cost of acquiring capital from outside. When the interest rate rises, insurers intend to raise the price of personal accident insurance, for which the price adjustment is much easier, to accumulate capital.
Our by-line analysis finds that the price dynamics of property-liability insurance, which represents a more than 95% share of the non-life insurance industry in China, has a great deal in common with counterparts in developed countries, except for the long-term effect of GDP On the other hand, the findings about the price dynamics of personal accident insurance are quite inconsistent with the existing literature. As Cummins and Danzon (Citation1992) suggests, the impacts of some economic and institutional variables on insurance are line-specific. We believe that the economic and institutional environment of China shaped the special price dynamics for the two non-life insurance lines under study.
Specifically, several important factors are thought to have significant impacts on the dynamics of the non-life insurance price in China according to the empirical results. First, strong demand for insurance as a result of continuous economic development is not only a powerful engine for the development of the insurance industry, but also helps to raise the price of insurance, which is unlikely in developed economies. Second, with an increasingly competitive insurance market – one that is characterised by more competitors and less market share for state-owned companies, the gradual abolishment of monopolies and strict regulation of insurance price setting and decreasing prices for some lines of non-life insurance – different lines of non-life insurance seem to be affected by this process in varying degrees. Third, the interest rate not only serves as an operator when computing the rate of insurance, but also as an indicator of the reform of the financial system for a country transitioning from a planned to a market-oriented economy, which means its relationship with insurance price might be complicated compared with developed economies. Finally, the rate of market return does not contain consistent effects on personal accident insurance price; the reasons for this could be the irrationality of the capital market, the insurance firms, or both. In developing economies, there are some common irrational operations of insurance companies, such as arbitrary or predatory pricing, bad faith on sales and claim management, destructive competition and potential collusion practice. To address these problems, many efforts have to be made by the regulatory authority, not only in maintaining an orderly market, but in playing a positive advisor role on various insurance practice such as underwriting, pricing, marketing and claim management.
5. Conclusion
This article uses the ARDL method to study both the long-term and short-term relationship between external factors and non-life insurance price by employing for the first time data from China, the largest developing country with mixed economic structure and the fourth biggest insurance market in the world. Findings for the long-term effects indicate that the price dynamics for various lines of non-life insurance are different. Specifically, GDP is found to have a significant positive effect on the price of property-liability insurance. Interest rate and rate of market return have a negative effect on property-liability insurance price, which is consistent with the evidence provided in much of the previous literature, while a positive effect is found for personal accident insurance price, which indicates that the price determination for personal accident insurance might be affected by some special factors, such as deregulation and intense competition among insurance firms in China. For instance, many insurance companies, especially for new entrants, still seize market shares by low-price strategy or refund of premium in the period of low interest rates. Moreover, empirical results for short-run effects indicate that property-liability insurance contains a longer cycle than personal accident insurance. In the short-run, the rate of market return negatively correlates with the price of both property-liability insurance and personal accident insurance (with a one-period lead). The interest rate, however, is found to have a positive effect on personal accident insurance price. Test results indicate that the price dynamics of different lines of non-life insurance in China are identified as varying according to the specific feature of the line and the related background of economic and institutional reform.
Our findings not only add new evidence on insurance price determination in Asian developing countries, by employing data from China for the first time, but also have important practical significance. According to our research, insurance companies in the Chinese non-life insurance market should widely consider economic factors in insurance rate determination, rather than solely relying on actuarial models when competing with counterparts. Regulatory authorities should pay more attention to the specific economic and institutional environments in China and adopt flexible regulatory measures when supervising the insurance markets; for specific lines, such as personal accident insurance under discussion here, in which the price dynamics significantly deviate from the traditional mode, special attention should be given to prevent irrational operations within the insurance companies and to protect the consumers. In order to prevent the market irrationality, several measures could be considered to ensure the public interest. First, to ensure the quality and availability of the personal accident insurance, the regulatory authority should strengthen solvency management to insurance companies who engage in such a line of business. Second, rate regulation is also an effective measure to avoid improper price competition, which could ensure prices are not being charged excessively and are within a rational range. In addition, the regulation agency should supervise insurance firms to improve corporate governance structure, creating more rational market practitioners. Finally, in the midst of the transition from planned to market economy, relevant policy interventions could be provided in order to encourage insurance companies to carry out other product development strategies, such as strategies of differentiation, personalization and regionalization, instead of the price competition.
Disclosure statement
No potential conflict of interest was reported by the authors.
References
- Banyár, J., & Regős, G. (2012). Paradoxical price effects on insurance markets. Economic Modelling, 29, 1399–1407.10.1016/j.econmod.2012.02.018
- Boswijk, H. P. (1994). Testing for an unstable root in conditional and structural error correction models. Journal of Econometrics, 63, 37–60.10.1016/0304-4076(93)01560-9
- Boswijk, H. P. (1995). Efficient inference on cointegration parameters in structural error correction models. Journal of Econometrics, 69, 133–158.10.1016/0304-4076(94)01665-M
- Boyer, M. M., Jacquier, E., & Norden, S. V. (2012). Are underwriting cycles real and forecastable? The Journal of Risk and Insurance, 79, 995–1015.10.1111/jori.2012.79.issue-4
- Cavanagh, C. L., Elliott, G., & Stock, J. H. (1995). Inference in models with nearly integrated regressors. Econometric Theory, 11, 1131–1147.10.1017/S0266466600009981
- Chen, R. B., Wong, K. A., & Lee, H. C. (1999). Underwriting cycles in Asia. The Journal of Risk and Insurance, 66, 29–47.10.2307/253876
- Choi, S., Hardigree, D., & Thistle, P. D. (2002). The property/liability insurance cycle: A comparison of alternative models. Southern Economic Journal, 68, 530–548.10.2307/1061716
- Cummins, J. D., & Danzon, P. M. (1992). Price shocks and capital flows in liability insurance. Working Paper. Philadelphia, PA: University of Pennsylvania.
- Cummins, J. D., & Outreville, J. F. (1987). An international analysis of underwriting cycles in property-liability insurance. The Journal of Risk and Insurance, 54, 246–262.10.2307/252856
- Doherty, N. A., & Garven, J. R. (1986). Price regulation in property-liability insurance: A contingent-claims approach. Journal of Finance, 41, 1031–1050.10.1111/j.1540-6261.1986.tb02529.x
- Doherty, N. A., & Garven, J. R. (1995). Insurance cycles: Interest rates and the capacity constraint model. The Journal of Business, 68, 383–404.10.1086/jb.1995.68.issue-3
- Doherty, N. A., & Kang, H. B. (1988). Interest rates and insurance price cycles. Journal of Banking and Finance, 12, 199–214.10.1016/0378-4266(88)90035-0
- Dragos, L. S. (2014). Life and non-life insurance demand: The different effects of influence factors in emerging countries from Europe and Asia. Economic Research- Ekonomska Istraživanja, 27, 169–180.10.1080/1331677X.2014.952112
- Fairley, W. B. (1979). Investment income and profit margins in property-liability insurance: Theory and empirical results. The Bell Journal of Economics, 10, 192–210.10.2307/3003326
- Fields, J. A., & Venezian, E. C. (1989). Interest rates and profit cycles: A disaggregated approach. The Journal of Risk and Insurance, 56, 312–319.10.2307/252992
- Grace, M. F., & Hotchkiss, J. L. (1995). External impacts on the property-liability insurance cycle. The Journal of Risk and Insurance, 62, 738–754.10.2307/253593
- Haley, J. D. (1993). A cointegration analysis of the relationship between underwriting margins and interest rates: 1930–1989. The Journal of Risk and Insurance, 60, 480–493.10.2307/253039
- Haley, J. D. (2007). Further considerations of underwriting margins, interest rates, stability, stationarity, cointegration, and time trends. Journal of Insurance Issues, 30, 62–75.
- Harrington, S. E., & Niehaus, G. (2000). Volatility and underwriting cycle. In G. Dionne (Ed.), The handbook of insurance economics (pp. 657–686). Boston, MA: Kluwer Academic.
- Harrington, S. E., & Yu, T. (2003). Do property-Casualty insurance underwriting margins have unit roots? The Journal of Risk and Insurance, 70, 735–753.
- Higgins, M. L., & Thistle, P. D. (2000). Capacity constraints and the dynamics of underwriting profits. Economic Inquiry, 38, 442–457.10.1111/ecin.2000.38.issue-3
- Jawadi, F., Bruneau, C., & Sghaier, N. (2009). Nonlinear cointegration relationships between non-life insurance premiums and financial markets. The Journal of Risk and Insurance, 76, 753–783.10.1111/jori.2009.76.issue-3
- Jiang, S., & Nieh, C. C. (2012). Dynamics of underwriting profits: Evidence from the U.S. insurance market. International Review of Economics and Finance, 21, 1–15.10.1016/j.iref.2011.03.005
- Lamm-Tennant, J., & Weiss, M. A. (1997). International insurance cycle: Rational expectations/Institutional intervention. The Journal of Risk and Insurance, 64, 415–439.10.2307/253758
- Lazar, D., & Denuit, M. M. (2012). Multivariate analysis of premium dynamics in P&L insurance. The Journal of Risk and Insurance, 79, 431–448.10.1111/jori.2012.79.issue-2
- Meier, U. B. (2006). Multi-national underwriting cycles in property-liability insurance: Part II. The Journal of Risk Finance, 7, 83–97.10.1108/15265940610637825
- Mills, T. C. (2008). The econometric modelling of financial time series. Cambridge: Cambridge University Press.10.1017/CBO9780511817380
- Mohd Daud, N., Halim Ahmad, A., & Azman-Saini, W. N. W. (2013). Does external debt contribute to Malaysia economic growth? Economic Research- Ekonomska Istraživanja, 26, 346–363.
- Pesaran, M. H., & Shin, Y. (1999). An auto regressive distributed-lag modeling approach to cointegration analysis. In S. Strom (Ed.), Econometrics and economic theory in the twentieth century: The Ragnar Frisch centennial symposium (pp. 317–413). Cambridge: Cambridge University Press.
- Pesaran, M. H., Shin, Y., & Smith, R. J. (2001). Bounds testing approaches to the analysis of long-run relationship. Journal of Applied Econometrics, 16, 289–326.10.1002/(ISSN)1099-1255
- Shahbaz, M., Lean, H., & Kalim, R. (2013). The impact of foreign direct investment on stock market development: Evidence from Pakistan. Economic Research- Ekonomska Istraživanja, 26, 17–32.10.1080/1331677X.2013.11517588
- Smith, M. L. (1989). Investment returns and yields to holders of insurance. The Journal of Business, 62, 81–98.10.1086/jb.1989.62.issue-1
- Weiss, A. M. (2007). Underwriting cycles: A synthesis and further directions. Journal of Insurance Issues, 30, 31–45.
- Winter, R. A. (1994). The dynamics of competitive insurance markets. Journal of Financial Intermediation, 3, 379–415.10.1006/jfin.1994.1011